Information
- Author Services

Initiatives
You are accessing a machine-readable page. In order to be human-readable, please install an RSS reader.
All articles published by MDPI are made immediately available worldwide under an open access license. No special permission is required to reuse all or part of the article published by MDPI, including figures and tables. For articles published under an open access Creative Common CC BY license, any part of the article may be reused without permission provided that the original article is clearly cited. For more information, please refer to https://www.mdpi.com/openaccess .
Feature papers represent the most advanced research with significant potential for high impact in the field. A Feature Paper should be a substantial original Article that involves several techniques or approaches, provides an outlook for future research directions and describes possible research applications.
Feature papers are submitted upon individual invitation or recommendation by the scientific editors and must receive positive feedback from the reviewers.
Editor’s Choice articles are based on recommendations by the scientific editors of MDPI journals from around the world. Editors select a small number of articles recently published in the journal that they believe will be particularly interesting to readers, or important in the respective research area. The aim is to provide a snapshot of some of the most exciting work published in the various research areas of the journal.
Original Submission Date Received: .
- Active Journals
- Find a Journal
- Proceedings Series
- For Authors
- For Reviewers
- For Editors
- For Librarians
- For Publishers
- For Societies
- For Conference Organizers
- Open Access Policy
- Institutional Open Access Program
- Special Issues Guidelines
- Editorial Process
- Research and Publication Ethics
- Article Processing Charges
- Testimonials
- Preprints.org
- SciProfiles
- Encyclopedia
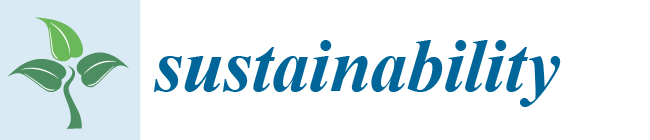
Article Menu
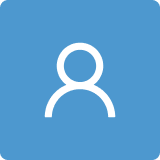
- Subscribe SciFeed
- Recommended Articles
- Google Scholar
- on Google Scholar
- Table of Contents
Find support for a specific problem in the support section of our website.
Please let us know what you think of our products and services.
Visit our dedicated information section to learn more about MDPI.
JSmol Viewer
Causality between technological innovation and economic growth: evidence from the economies of developing countries.

1. Introduction
- It is considered an important applied research design in studying the relationship between technological innovation and economic growth.
- It intensifies the research and development process for the purpose of changing the traditional structures in developing countries, and thus provides new goods that would improve the financial conditions and consequently the economic growth of countries.
- It highlights the transition from that of the traditional economy to the innovative one, by acquiring various skills that enable countries to improve their financial performance.
- The researcher expects, through this study, to motivate researchers to conduct more research in the field of technological innovation, especially on the relationship between innovation, sustainable development, and competitiveness.
2. Theoretical Background
- Supply-leading Hypothesis (SLH) suggests a unidirectional causality between innovation activities and economic growth (see, for example, Yang, [ 62 ]; Guloglu and Tekin, [ 40 ]; Cetin, [ 31 ]; Pradhan et al.) [ 63 ].
- Demand-following Hypotheses (DFH) suggest unidirectional causality from economic growth to innovation activities (see, for example, Sinha, [ 64 ]; Cetin, [ 31 ]; Sadraoui et al., [ 65 ]; Pradhan et al., [ 63 ].
- The Feedback Hypothesis (FBH) suggests a bidirectional causality between economic growth and innovation practices (see, for example, Guloglu and Tekin, [ 40 ]; Cetin, [ 31 ]; Pradhan et al. [ 63 ].
- The Neutrality Hypothesis (NLH) suggests no association between economic growth and innovation activities (see, for example, Cetin, [ 31 ]; Pradhan et al., [ 63 ].
- Throughout history, nations seeking a successful future have relied on the discovery of the next “great idea,” which often follows the accidental discovery of a great idea that propelled the country forward. Nevertheless, for the country to succeed in competition, and for its growth to continue in the current and ever-changing business environment, it must learn how to develop a thriving innovation culture—that is, a continuous ability to generate, accept, and implement creative ideas—within the country, as can be seen in Figure 2 .
3. Research Model
3.1. economic methodology, 3.2. standard methodology.
- ( Y i t X i t ) Study variable vectors.
- Panel data cross-sectional directions ( i = 1, …, N ),
- (N) represents the number of units (people, companies, industries, countries... etc.),
- (t = 1, …, T) time direction.
3.3. Availability of Data and Material
4. estimating and analyzing results.
- The minimum value of the PAR over all the sample is 0.23 while the maximum is 1233, with average = 42.8 and standard deviation = 85.4.
- The minimum value of the PAN over all the sample is 0.91 while the maximum is 1524, with average = 55.3 and standard deviation = 121.7
- The minimum value of the RDE over all the samples is 0 while the maximum is 0.534, with average = 0.022 and standard deviation = 0.041.
- The minimum value of the PRD over all the samples is 0.28 while the maximum is 660.3, with average = 45.2 and standard deviation = 103.6.
- The minimum value of the THE overall the sample is 0.0016 while the maximum is 2.9, with average = 0.28 and standard deviation = 0.48.
- The minimum value of the STJ over all the sample is 0.69 while the maximum is 1966, with average = 153.5 and standard deviation = 207.8.
- The minimum value of the EDU over all the sample is 0 while the maximum is 1.27, with average = 0.135 and standard deviation = 0.157.
- The minimum value of the GDP per capita growth overall the samples is −14.351 while the maximum is 13.636, with average = 2.974 and standard deviation = 3.841. The following figures show the highest and lowest averages of innovation variables for all developing countries during the period 1990–2018, shown in Figure 3 , Figure 4 , Figure 5 , Figure 6 , Figure 7 , Figure 8 , Figure 9 and Figure 10 . We can conclude the following: Mexico has the highest average PAR, followed by Hungary, while Tunisia has the lowest one ( Figure 3 ). The lowest. Figure 4 shows that Argentina had the highest average for PAN. Regarding Figure 5 , Hungary has the highest average RDE, while China and Indonesia have the lowest averages. Figure 6 shows that Hungary has the highest PRD average, while China, Indonesia, and the Philippines have the lowest averages. According to Figure 7 and Figure 8 , Hungary has the highest average for THE and DUE., While China has the lowest average of these two variables. Figure 9 shows that Poland had the highest average for STJ, while the Philippines had the lowest average. China recorded the highest average per capita GDP, while Argentina had the lowest ( Figure 10 ).
- Sargan-Hansen test or Sargan‘s test is a statistical test used in the statistical model to assess over-identifying limitations. In other words, it checks whether the instrument variables used are correct. This test’s null hypothesis is, “no over-identification.” If the null hypothesis is not dismissed, the model is correct.
- The Arellano-Bond method tests whether the errors are correlated. This test’s null hypothesis is “no self-correlation”. If the null hypothesis is not dismissed, the model is correct. The findings are provided in Table 7 and Table 8 and it is possible to infer that there is a significant negative impact of education (percent of expenditure in GDP) on GDP per capita growth, and this effect = −3.5, with 95% confidence, as the p -value of the coefficient is less than 5%. Moreover, findings show a significant positive impact of research development and expenditure on GDP per capita growth, with an effect = 0.00269, and with a 95% confidence as the p -value of the coefficient is less than 5%. While there is a significant positive impact of scientific and technical journal articles on GDP per capita growth, and this effect = 0.004503, with 95% confidence as the p -value of the coefficient is less than 5%. However, there is a significant positive impact of high technology exports on GDP per capita growth, and this effect = 0.740, with 95% confidence as the p -value of the coefficient is less than 5%. Finally, there is an insignificant impact of each of PAN, PAR, and RDE on GDP per capita, with 95% confidence as the p -value for these coefficients are greater than 5%. Regarding the goodness of fit of the model and analysis of the results of the dynamic model estimation for GMM, the empirical results from estimating the dynamic models of panel data by GMM are good if the estimated values of the regression coefficients of these models by this method are consistent and consistency is achieved with the actual values of the regression coefficients. This can be illustrated by the graph in Figure 12 showing the consistency of the actual, estimated, and residual values with each other. Additionally, to determine the validity of these variables, Sargan’s statistical test was used, and it was greater than 5%. Therefore, the null hypothesis was accepted, which states the quality and suitability of the tools used in the model and the validity of the moment conditions used in the estimation. It was also clear through Sargan’s test that the delay variables were valid and that the first-degree differences were statistically acceptable. On the other hand, the statistical value of the Arellano-Bond test for second-order serial correlation between the estimated errors with the first step indicates that the null hypothesis of this test is not rejected, which is the absence of this correlation. This means that the original error term is not sequentially related. This is because the estimation results in Table 7 show that the probability of this test statistic is greater than 5%, equal to (0.9907) that is, accepting the null hypothesis that there is no second-order serial correlation to the random error, and this indicates the validity of the moment constraints used in the estimation.
5. Discussion
6. conclusions and recommendations, author contributions, institutional review board statement, informed consent statement, data availability statement, conflicts of interest.
Pairwise Granger Causality Tests. | |||
---|---|---|---|
Sample: 1990 2018 IF COUNTRY1 = 1 | |||
Null Hypothesis: | Obs | F-Statistic | Prob. |
PAN does not Granger Cause GDP_PER_CAPITA | 27 | 3.45139 | 0.0497 |
GDP_PER_CAPITA does not Granger Cause PAN | 0.65959 | 0.5270 | |
PAR does not Granger Cause GDP_PER_CAPITA | 27 | 0.28866 | 0.7521 |
GDP_PER_CAPITA does not Granger Cause PAR | 0.54733 | 0.5862 | |
PRD does not Granger Cause GDP_PER_CAPITA | 27 | 0.41770 | 0.6637 |
GDP_PER_CAPITA does not Granger Cause PRD | 1.35729 | 0.2781 | |
RDE does not Granger Cause GDP_PER_CAPITA | 27 | 0.19865 | 0.8213 |
GDP_PER_CAPITA does not Granger Cause RDE | 0.11666 | 0.8904 | |
STJ does not Granger Cause GDP_PER_CAPITA | 27 | 0.88593 | 0.4265 |
GDP_PER_CAPITA does not Granger Cause STJ | 0.72180 | 0.4970 | |
THE does not Granger Cause GDP_PER_CAPITA | 27 | 5.55960 | 0.0111 |
GDP_PER_CAPITA does not Granger Cause THE | 0.87955 | 0.4291 | |
EDU does not Granger Cause GDP_PER_CAPITA | 27 | 0.06448 | 0.9377 |
GDP_PER_CAPITA does not Granger Cause EDU | 0.08922 | 0.9150 | |
Pairwise Granger Causality Tests | |||
Sample: 1990 2018 IF COUNTRY1 = 2 | |||
Null Hypothesis: | Obs | F-Statistic | Prob. |
PAN does not Granger Cause GDP_PER_CAPITA | 27 | 1.85640 | 0.1713 |
GDP_PER_CAPITA does not Granger Cause PAN | 1.20757 | 0.3340 | |
PAR does not Granger Cause GDP_PER_CAPITA | 27 | 2.75374 | 0.0709 |
GDP_PER_CAPITA does not Granger Cause PAR | 1.69911 | 0.2010 | |
PRD does not Granger Cause GDP_PER_CAPITA | 27 | 3.75307 | 0.0285 |
GDP_PER_CAPITA does not Granger Cause PRD | 2.52407 | 0.0884 | |
RDE does not Granger Cause GDP_PER_CAPITA | 27 | 3.80148 | 0.0273 |
GDP_PER_CAPITA does not Granger Cause RDE | 1.31620 | 0.2983 | |
STJ does not Granger Cause GDP_PER_CAPITA | 27 | 3.80122 | 0.0273 |
GDP_PER_CAPITA does not Granger Cause STJ | 2.78491 | 0.0689 | |
THE does not Granger Cause GDP_PER_CAPITA | 27 | 2.11193 | 0.1325 |
GDP_PER_CAPITA does not Granger Cause THE | 1.21826 | 0.3303 | |
EDU does not Granger Cause GDP_PER_CAPITA | 27 | 2.50999 | 0.0896 |
GDP_PER_CAPITA does not Granger Cause EDU | 1.46903 | 0.2547 | |
Pairwise Granger Causality Tests | |||
Sample: 1990 2018 IF COUNTRY1 = 3 | |||
Null Hypothesis: | Obs | F-Statistic | Prob. |
PAN does not Granger Cause GDP_PER_CAPITA | 27 | 0.02088 | 0.9794 |
GDP_PER_CAPITA does not Granger Cause PAN | 3.50598 | 0.0477 | |
PAR does not Granger Cause GDP_PER_CAPITA | 27 | 1.05750 | 0.3643 |
GDP_PER_CAPITA does not Granger Cause PAR | 0.92123 | 0.4128 | |
PRD does not Granger Cause GDP_PER_CAPITA | 27 | 0.61420 | 0.5501 |
GDP_PER_CAPITA does not Granger Cause PRD | 3.58595 | 0.0449 | |
RDE does not Granger Cause GDP_PER_CAPITA | 27 | 0.93278 | 0.4085 |
GDP_PER_CAPITA does not Granger Cause RDE | 1.57160 | 0.2302 | |
STJ does not Granger Cause GDP_PER_CAPITA | 27 | 3.85557 | 0.0367 |
GDP_PER_CAPITA does not Granger Cause STJ | 0.82624 | 0.4508 | |
THE does not Granger Cause GDP_PER_CAPITA | 27 | 0.48506 | 0.6221 |
GDP_PER_CAPITA does not Granger Cause THE | 0.26147 | 0.7723 | |
EDU does not Granger Cause GDP_PER_CAPITA | 27 | 3.45232 | 0.0497 |
GDP_PER_CAPITA does not Granger Cause EDU | 3.39050 | 0.0521 | |
Pairwise Granger Causality Tests | |||
Sample: 1990 2018 IF COUNTRY1 = 4 | |||
Null Hypothesis: | Obs | F-Statistic | Prob. |
PAN does not Granger Cause GDP_PER_CAPITA | 27 | 0.55365 | 0.4638 |
GDP_PER_CAPITA does not Granger Cause PAN | 4.24517 | 0.0499 | |
PAR does not Granger Cause GDP_PER_CAPITA | 27 | 0.55365 | 0.4638 |
GDP_PER_CAPITA does not Granger Cause PAR | 4.24517 | 0.0499 | |
PRD does not Granger Cause GDP_PER_CAPITA | 27 | 2.08852 | 0.1608 |
GDP_PER_CAPITA does not Granger Cause PRD | 1.31153 | 0.2630 | |
RDE does not Granger Cause GDP_PER_CAPITA | 27 | 2.25745 | 0.1455 |
GDP_PER_CAPITA does not Granger Cause RDE | 1.08045 | 0.3085 | |
STJ does not Granger Cause GDP_PER_CAPITA | 27 | 24.2234 | 5.E-05 |
GDP_PER_CAPITA does not Granger Cause STJ | 0.47384 | 0.4976 | |
THE does not Granger Cause GDP_PER_CAPITA | 27 | 9.65160 | 0.0047 |
GDP_PER_CAPITA does not Granger Cause THE | 1.68544 | 0.2061 | |
EDU does not Granger Cause GDP_PER_CAPITA | 27 | 0.17195 | 0.6819 |
GDP_PER_CAPITA does not Granger Cause EDU | 2.04251 | 0.1653 | |
Pairwise Granger Causality Tests | |||
Sample: 1990 2018 IF COUNTRY1 = 5 | |||
Null Hypothesis: | Obs | F-Statistic | Prob. |
PAN does not Granger Cause GDP_PER_CAPITA | 27 | 6.42670 | 0.0179 |
GDP_PER_CAPITA does not Granger Cause PAN | 0.16010 | 0.6925 | |
PAR does not Granger Cause GDP_PER_CAPITA | 27 | 6.42670 | 0.0179 |
GDP_PER_CAPITA does not Granger Cause PAR | 0.16010 | 0.6925 | |
PRD does not Granger Cause GDP_PER_CAPITA | 27 | 0.93974 | 0.3416 |
GDP_PER_CAPITA does not Granger Cause PRD | 0.70005 | 0.4107 | |
RDE does not Granger Cause GDP_PER_CAPITA | 27 | 0.25433 | 0.6185 |
GDP_PER_CAPITA does not Granger Cause RDE | 4.69006 | 0.0401 | |
STJ does not Granger Cause GDP_PER_CAPITA | 27 | 0.70643 | 0.4086 |
GDP_PER_CAPITA does not Granger Cause STJ | 8.69955 | 0.0068 | |
THE does not Granger Cause GDP_PER_CAPITA | 27 | 2.18918 | 0.1515 |
GDP_PER_CAPITA does not Granger Cause THE | 5.95758 | 0.0221 | |
EDU does not Granger Cause GDP_PER_CAPITA | 27 | 0.54776 | 0.4661 |
GDP_PER_CAPITA does not Granger Cause EDU | 4.74801 | 0.0390 | |
Pairwise Granger Causality Tests | |||
Sample: 1990 2018 IF COUNTRY1 = 6 | |||
Null Hypothesis: | Obs | F-Statistic | Prob. |
PAN does not Granger Cause GDP_PER_CAPITA | 27 | 0.07652 | 0.9266 |
GDP_PER_CAPITA does not Granger Cause PAN | 4.13486 | 0.0299 | |
PAR does not Granger Cause GDP_PER_CAPITA | 27 | 0.07652 | 0.9266 |
GDP_PER_CAPITA does not Granger Cause PAR | 4.13486 | 0.0299 | |
PRD does not Granger Cause GDP_PER_CAPITA | 27 | 1.59115 | 0.2263 |
GDP_PER_CAPITA does not Granger Cause PRD | 1.71315 | 0.2035 | |
RDE does not Granger Cause GDP_PER_CAPITA | 27 | 0.63068 | 0.5416 |
GDP_PER_CAPITA does not Granger Cause RDE | 6.14284 | 0.0076 | |
STJ does not Granger Cause GDP_PER_CAPITA | 27 | 4.29613 | 0.0266 |
GDP_PER_CAPITA does not Granger Cause STJ | 1.72200 | 0.2019 | |
THE does not Granger Cause GDP_PER_CAPITA | 27 | 5.98152 | 0.0084 |
GDP_PER_CAPITA does not Granger Cause THE | 0.04888 | 0.9524 | |
EDU does not Granger Cause GDP_PER_CAPITA | 27 | 0.08946 | 0.9148 |
GDP_PER_CAPITA does not Granger Cause EDU | 0.13876 | 0.8712 | |
Pairwise Granger Causality Tests | |||
Sample: 1990 2018 IF COUNTRY1 = 7 | |||
Lags: 1 | |||
Null Hypothesis: | Obs | F-Statistic | Prob. |
PAN does not Granger Cause GDP_PER_CAPITA | 27 | 7.85133 | 0.0097 |
GDP_PER_CAPITA does not Granger Cause PAN | 0.05001 | 0.8249 | |
PAR does not Granger Cause GDP_PER_CAPITA | 27 | 7.85133 | 0.0097 |
GDP_PER_CAPITA does not Granger Cause PAR | 0.05001 | 0.8249 | |
PRD does not Granger Cause GDP_PER_CAPITA | 27 | 1.01360 | 0.3237 |
GDP_PER_CAPITA does not Granger Cause PRD | 0.50835 | 0.4825 | |
RDE does not Granger Cause GDP_PER_CAPITA | 27 | 0.12053 | 0.7314 |
GDP_PER_CAPITA does not Granger Cause RDE | 6.87595 | 0.0147 | |
STJ does not Granger Cause GDP_PER_CAPITA | 27 | 0.02424 | 0.8775 |
GDP_PER_CAPITA does not Granger Cause STJ | 1.20223 | 0.2833 | |
THE does not Granger Cause GDP_PER_CAPITA | 27 | 0.06169 | 0.8059 |
GDP_PER_CAPITA does not Granger Cause THE | 1.60651 | 0.2167 | |
EDU does not Granger Cause GDP_PER_CAPITA | 27 | 18.0285 | 0.0003 |
GDP_PER_CAPITA does not Granger Cause EDU | 6.94753 | 0.0142 | |
Pairwise Granger Causality Tests | |||
Sample: 1990 2018 IF COUNTRY1 = 8 | |||
Null Hypothesis: | Obs | F-Statistic | Prob. |
PAN does not Granger Cause GDP_PER_CAPITA | 27 | 0.11664 | 0.7356 |
GDP_PER_CAPITA does not Granger Cause PAN | 1.36300 | 0.2540 | |
PAR does not Granger Cause GDP_PER_CAPITA | 27 | 0.11664 | 0.7356 |
GDP_PER_CAPITA does not Granger Cause PAR | 1.36300 | 0.2540 | |
PRD does not Granger Cause GDP_PER_CAPITA | 27 | 1.23194 | 0.2776 |
GDP_PER_CAPITA does not Granger Cause PRD | 6.58574 | 0.0167 | |
RDE does not Granger Cause GDP_PER_CAPITA | 27 | 4.35234 | 0.0473 |
GDP_PER_CAPITA does not Granger Cause RDE | 53.1925 | 1.E-07 | |
STJ does not Granger Cause GDP_PER_CAPITA | 27 | 4.47252 | 0.0446 |
GDP_PER_CAPITA does not Granger Cause STJ | 2.96970 | 0.0972 | |
THE does not Granger Cause GDP_PER_CAPITA | 27 | 3.48594 | 0.0737 |
GDP_PER_CAPITA does not Granger Cause THE | 1.65908 | 0.2095 | |
EDU does not Granger Cause GDP_PER_CAPITA | 27 | 1.07799 | 0.3091 |
GDP_PER_CAPITA does not Granger Cause EDU | 0.00019 | 0.9892 | |
Pairwise Granger Causality Tests | |||
Sample: 1990 2018 IF COUNTRY1 = 9 | |||
Null Hypothesis: | Obs | F-Statistic | Prob. |
PAN does not Granger Cause GDP_PER_CAPITA | 27 | 0.60294 | 0.5560 |
GDP_PER_CAPITA does not Granger Cause PAN | 5.20243 | 0.0141 | |
PAR does not Granger Cause GDP_PER_CAPITA | 27 | 0.60294 | 0.5560 |
GDP_PER_CAPITA does not Granger Cause PAR | 5.20243 | 0.0141 | |
PRD does not Granger Cause GDP_PER_CAPITA | 27 | 4.82837 | 0.0183 |
GDP_PER_CAPITA does not Granger Cause PRD | 0.11963 | 0.8878 | |
RDE does not Granger Cause GDP_PER_CAPITA | 27 | 0.01557 | 0.9846 |
GDP_PER_CAPITA does not Granger Cause RDE | 0.51660 | 0.6036 | |
STJ does not Granger Cause GDP_PER_CAPITA | 27 | 0.02185 | 0.9784 |
GDP_PER_CAPITA does not Granger Cause STJ | 2.43003 | 0.1113 | |
THE does not Granger Cause GDP_PER_CAPITA | 27 | 1.54441 | 0.2357 |
GDP_PER_CAPITA does not Granger Cause THE | 6.48804 | 0.0061 | |
EDU does not Granger Cause GDP_PER_CAPITA | 27 | 1.45638 | 0.2547 |
GDP_PER_CAPITA does not Granger Cause EDU | 0.39204 | 0.6803 | |
Pairwise Granger Causality Tests | |||
Sample: 1990 2018 IF COUNTRY1 = 10 | |||
Null Hypothesis: | Obs | F-Statistic | Prob. |
PAN does not Granger Cause GDP_PER_CAPITA | 27 | 3.28536 | 0.0819 |
GDP_PER_CAPITA does not Granger Cause PAN | 0.25676 | 0.6168 | |
PAR does not Granger Cause GDP_PER_CAPITA | 27 | 3.28536 | 0.0819 |
GDP_PER_CAPITA does not Granger Cause PAR | 0.25676 | 0.6168 | |
PRD does not Granger Cause GDP_PER_CAPITA | 27 | 8.85677 | 0.0064 |
GDP_PER_CAPITA does not Granger Cause PRD | 0.09699 | 0.7581 | |
RDE does not Granger Cause GDP_PER_CAPITA | 27 | 9.04547 | 0.0059 |
GDP_PER_CAPITA does not Granger Cause RDE | 0.07771 | 0.7827 | |
STJ does not Granger Cause GDP_PER_CAPITA | 27 | 3.93442 | 0.0584 |
GDP_PER_CAPITA does not Granger Cause STJ | 0.50925 | 0.4821 | |
THE does not Granger Cause GDP_PER_CAPITA | 27 | 4.39407 | 0.0463 |
GDP_PER_CAPITA does not Granger Cause THE | 0.07888 | 0.7811 | |
EDU does not Granger Cause GDP_PER_CAPITA | 27 | 2.43955 | 0.1309 |
GDP_PER_CAPITA does not Granger Cause EDU | 3.25965 | 0.0831 | |
Pairwise Granger Causality Tests | |||
Sample: 1990 2018 IF COUNTRY1 = 11 | |||
Null Hypothesis: | Obs | F-Statistic | Prob. |
PAN does not Granger Cause GDP_PER_CAPITA | 27 | 0.33389 | 0.7197 |
GDP_PER_CAPITA does not Granger Cause PAN | 0.11052 | 0.8959 | |
PAR does not Granger Cause GDP_PER_CAPITA | 27 | 0.33389 | 0.7197 |
GDP_PER_CAPITA does not Granger Cause PAR | 0.11052 | 0.8959 | |
PRD does not Granger Cause GDP_PER_CAPITA | 27 | 0.28754 | 0.7529 |
GDP_PER_CAPITA does not Granger Cause PRD | 0.09579 | 0.9090 | |
RDE does not Granger Cause GDP_PER_CAPITA | 27 | 0.31281 | 0.7346 |
GDP_PER_CAPITA does not Granger Cause RDE | 0.18231 | 0.8346 | |
STJ does not Granger Cause GDP_PER_CAPITA | 27 | 0.26862 | 0.7669 |
GDP_PER_CAPITA does not Granger Cause STJ | 1.67510 | 0.2103 | |
THE does not Granger Cause GDP_PER_CAPITA | 27 | 0.29089 | 0.7504 |
GDP_PER_CAPITA does not Granger Cause THE | 0.01289 | 0.9872 | |
EDU does not Granger Cause GDP_PER_CAPITA | 27 | 0.32060 | 0.7290 |
GDP_PER_CAPITA does not Granger Cause EDU | 0.02119 | 0.9791 | |
Pairwise Granger Causality Tests | |||
Sample: 1990 2018 IF COUNTRY1 = 12 | |||
Null Hypothesis: | Obs | F-Statistic | Prob. |
PAN does not Granger Cause GDP_PER_CAPITA | 26 | 0.89077 | 0.4638 |
GDP_PER_CAPITA does not Granger Cause PAN | 3.30388 | 0.0425 | |
PAR does not Granger Cause GDP_PER_CAPITA | 26 | 0.89077 | 0.4638 |
GDP_PER_CAPITA does not Granger Cause PAR | 3.30388 | 0.0425 | |
PRD does not Granger Cause GDP_PER_CAPITA | 26 | 0.45675 | 0.7157 |
GDP_PER_CAPITA does not Granger Cause PRD | 1.24709 | 0.3205 | |
RDE does not Granger Cause GDP_PER_CAPITA | 26 | 3.90725 | 0.0249 |
GDP_PER_CAPITA does not Granger Cause RDE | 0.69901 | 0.5642 | |
STJ does not Granger Cause GDP_PER_CAPITA | 26 | 0.74834 | 0.5367 |
GDP_PER_CAPITA does not Granger Cause STJ | 3.48123 | 0.0363 | |
THE does not Granger Cause GDP_PER_CAPITA | 26 | 1.98610 | 0.1503 |
GDP_PER_CAPITA does not Granger Cause THE | 1.64873 | 0.2117 | |
EDU does not Granger Cause GDP_PER_CAPITA | 26 | 0.59479 | 0.6261 |
GDP_PER_CAPITA does not Granger Cause EDU | 1.69819 | 0.2012 | |
Pairwise Granger Causality Tests | |||
Sample: 1990 2018 IF COUNTRY1 = 13 | |||
Null Hypothesis: | Obs | F-Statistic | Prob. |
PAN does not Granger Cause GDP_PER_CAPITA | 27 | 0.97908 | 0.3914 |
GDP_PER_CAPITA does not Granger Cause PAN | 0.66307 | 0.5253 | |
PAR does not Granger Cause GDP_PER_CAPITA | 27 | 0.97908 | 0.3914 |
GDP_PER_CAPITA does not Granger Cause PAR | 0.66307 | 0.5253 | |
PRD does not Granger Cause GDP_PER_CAPITA | 27 | 0.27895 | 0.7592 |
GDP_PER_CAPITA does not Granger Cause PRD | 0.45400 | 0.6409 | |
RDE does not Granger Cause GDP_PER_CAPITA | 27 | 0.91969 | 0.4134 |
GDP_PER_CAPITA does not Granger Cause RDE | 0.36466 | 0.6986 | |
STJ does not Granger Cause GDP_PER_CAPITA | 27 | 0.09426 | 0.9104 |
GDP_PER_CAPITA does not Granger Cause STJ | 5.11692 | 0.0150 | |
THE does not Granger Cause GDP_PER_CAPITA | 27 | 0.93801 | 0.4065 |
GDP_PER_CAPITA does not Granger Cause THE | 0.91330 | 0.4159 | |
EDU does not Granger Cause GDP_PER_CAPITA | 27 | 0.03313 | 0.9675 |
GDP_PER_CAPITA does not Granger Cause EDU | 0.17291 | 0.8423 | |
Pairwise Granger Causality Tests | |||
Sample: 1990 2018 IF COUNTRY1 = 14 | |||
Null Hypothesis: | Obs | F-Statistic | Prob. |
PAN does not Granger Cause GDP_PER_CAPITA | 27 | 1.32599 | 0.2859 |
GDP_PER_CAPITA does not Granger Cause PAN | 0.14147 | 0.8689 | |
PAR does not Granger Cause GDP_PER_CAPITA | 27 | 1.37618 | 0.2734 |
GDP_PER_CAPITA does not Granger Cause PAR | 1.62187 | 0.2203 | |
PRD does not Granger Cause GDP_PER_CAPITA | 27 | 0.33509 | 0.7189 |
GDP_PER_CAPITA does not Granger Cause PRD | 0.69119 | 0.5115 | |
RDE does not Granger Cause GDP_PER_CAPITA | 27 | 4.55742 | 0.0221 |
GDP_PER_CAPITA does not Granger Cause RDE | 0.67502 | 0.5194 | |
STJ does not Granger Cause GDP_PER_CAPITA | 27 | 0.15912 | 0.8539 |
GDP_PER_CAPITA does not Granger Cause STJ | 0.41448 | 0.6657 | |
THE does not Granger Cause GDP_PER_CAPITA | 27 | 1.78683 | 0.1910 |
GDP_PER_CAPITA does not Granger Cause THE | 0.26876 | 0.7668 | |
EDU does not Granger Cause GDP_PER_CAPITA | 27 | 3.61820 | 0.0438 |
GDP_PER_CAPITA does not Granger Cause EDU | 0.98913 | 0.3878 | |
Pairwise Granger Causality Tests | |||
Sample: 1990 2018 IF COUNTRY1 = 15 | |||
Null Hypothesis: | Obs | F-Statistic | Prob. |
PAN does not Granger Cause GDP_PER_CAPITA | 27 | 3.94050 | 0.0242 |
GDP_PER_CAPITA does not Granger Cause PAN | 0.95690 | 0.4332 | |
PAR does not Granger Cause GDP_PER_CAPITA | 27 | 0.58816 | 0.6302 |
GDP_PER_CAPITA does not Granger Cause PAR | 2.94843 | 0.0590 | |
PRD does not Granger Cause GDP_PER_CAPITA | 27 | 0.26065 | 0.8528 |
GDP_PER_CAPITA does not Granger Cause PRD | 0.80394 | 0.5071 | |
RDE does not Granger Cause GDP_PER_CAPITA | 27 | 1.05090 | 0.3930 |
GDP_PER_CAPITA does not Granger Cause RDE | 3.07919 | 0.0523 | |
STJ does not Granger Cause GDP_PER_CAPITA | 27 | 2.19014 | 0.1226 |
GDP_PER_CAPITA does not Granger Cause STJ | 0.18275 | 0.9068 | |
THE does not Granger Cause GDP_PER_CAPITA | 27 | 0.81335 | 0.5022 |
GDP_PER_CAPITA does not Granger Cause THE | 4.74172 | 0.0124 | |
EDU does not Granger Cause GDP_PER_CAPITA | 27 | 0.04317 | 0.9877 |
GDP_PER_CAPITA does not Granger Cause EDU | 1.17654 | 0.3449 | |
Pairwise Granger Causality Tests | |||
Sample: 1990 2018 IF COUNTRY1 = 16 | |||
Lags: 2 | |||
Null Hypothesis: | Obs | F-Statistic | Prob. |
PAN does not Granger Cause GDP_PER_CAPITA | 27 | 2.56142 | 0.1000 |
GDP_PER_CAPITA does not Granger Cause PAN | 6.73806 | 0.0052 | |
PAR does not Granger Cause GDP_PER_CAPITA | 27 | 2.40498 | 0.1136 |
GDP_PER_CAPITA does not Granger Cause PAR | 0.14059 | 0.8696 | |
PRD does not Granger Cause GDP_PER_CAPITA | 27 | 0.85992 | 0.4369 |
GDP_PER_CAPITA does not Granger Cause PRD | 0.29628 | 0.7465 | |
RDE does not Granger Cause GDP_PER_CAPITA | 27 | 1.02146 | 0.3765 |
GDP_PER_CAPITA does not Granger Cause RDE | 0.13856 | 0.8714 | |
STJ does not Granger Cause GDP_PER_CAPITA | 27 | 9.11119 | 0.0013 |
GDP_PER_CAPITA does not Granger Cause STJ | 19.9675 | 1.E-05 | |
THE does not Granger Cause GDP_PER_CAPITA | 27 | 0.35056 | 0.7082 |
GDP_PER_CAPITA does not Granger Cause THE | 11.8021 | 0.0003 | |
EDU does not Granger Cause GDP_PER_CAPITA | 27 | 2.68070 | 0.0908 |
GDP_PER_CAPITA does not Granger Cause EDU | 2.94423 | 0.0736 | |
Pairwise Granger Causality Tests | |||
Sample: 1990 2018 IF COUNTRY1 = 17 | |||
Null Hypothesis: | Obs | F-Statistic | Prob. |
PAN does not Granger Cause GDP_PER_CAPITA | 27 | 0.13404 | 0.8753 |
GDP_PER_CAPITA does not Granger Cause PAN | 0.34464 | 0.7122 | |
PAR does not Granger Cause GDP_PER_CAPITA | 27 | 1.79948 | 0.1889 |
GDP_PER_CAPITA does not Granger Cause PAR | 9.57892 | 0.0010 | |
PRD does not Granger Cause GDP_PER_CAPITA | 27 | 1.56537 | 0.2314 |
GDP_PER_CAPITA does not Granger Cause PRD | 1.02108 | 0.3767 | |
RDE does not Granger Cause GDP_PER_CAPITA | 27 | 0.27437 | 0.7626 |
GDP_PER_CAPITA does not Granger Cause RDE | 1.95295 | 0.1657 | |
STJ does not Granger Cause GDP_PER_CAPITA | 27 | 0.98285 | 0.3901 |
GDP_PER_CAPITA does not Granger Cause STJ | 0.50109 | 0.6126 | |
THE does not Granger Cause GDP_PER_CAPITA | 27 | 1.00865 | 0.3810 |
GDP_PER_CAPITA does not Granger Cause THE | 1.46224 | 0.2534 | |
EDU does not Granger Cause GDP_PER_CAPITA | 27 | 0.59774 | 0.5587 |
GDP_PER_CAPITA does not Granger Cause EDU | 0.34051 | 0.7151 | |
Pairwise Granger Causality Tests | |||
Sample: 1990 2018 IF COUNTRY1 = 18 | |||
Null Hypothesis: | Obs | F-Statistic | Prob. |
PAN does not Granger Cause GDP_PER_CAPITA | 27 | 4.52198 | 0.0226 |
GDP_PER_CAPITA does not Granger Cause PAN | 1.67446 | 0.2104 | |
PAR does not Granger Cause GDP_PER_CAPITA | 27 | 1.14622 | 0.3361 |
GDP_PER_CAPITA does not Granger Cause PAR | 0.80727 | 0.4589 | |
PRD does not Granger Cause GDP_PER_CAPITA | 27 | 1.54357 | 0.2359 |
GDP_PER_CAPITA does not Granger Cause PRD | 1.21407 | 0.3161 | |
RDE does not Granger Cause GDP_PER_CAPITA | 27 | 0.76778 | 0.4761 |
GDP_PER_CAPITA does not Granger Cause RDE | 2.69477 | 0.0898 | |
STJ does not Granger Cause GDP_PER_CAPITA | 27 | 6.39219 | 0.0065 |
GDP_PER_CAPITA does not Granger Cause STJ | 4.38578 | 0.0249 | |
THE does not Granger Cause GDP_PER_CAPITA | 27 | 8.52687 | 0.0018 |
GDP_PER_CAPITA does not Granger Cause THE | 0.56740 | 0.5751 | |
EDU does not Granger Cause GDP_PER_CAPITA | 27 | 5.85179 | 0.0092 |
GDP_PER_CAPITA does not Granger Cause EDU | 0.02474 | 0.9756 | |
Pairwise Granger Causality Tests | |||
Sample: 1990 2018 IF COUNTRY1 = 19 | |||
Null Hypothesis: | Obs | F-Statistic | Prob. |
PAN does not Granger Cause GDP_PER_CAPITA | 27 | 0.25761 | 0.6162 |
GDP_PER_CAPITA does not Granger Cause PAN | 7.66646 | 0.0104 | |
PAR does not Granger Cause GDP_PER_CAPITA | 27 | 3.59486 | 0.0696 |
GDP_PER_CAPITA does not Granger Cause PAR | 6.67297 | 0.0160 | |
PRD does not Granger Cause GDP_PER_CAPITA | 27 | 0.04494 | 0.8338 |
GDP_PER_CAPITA does not Granger Cause PRD | 2.59188 | 0.1200 | |
RDE does not Granger Cause GDP_PER_CAPITA | 27 | 9.86050 | 0.0043 |
GDP_PER_CAPITA does not Granger Cause RDE | 1.16887 | 0.2900 | |
STJ does not Granger Cause GDP_PER_CAPITA | 27 | 2.20958 | 0.1497 |
GDP_PER_CAPITA does not Granger Cause STJ | 16.5676 | 0.0004 | |
THE does not Granger Cause GDP_PER_CAPITA | 27 | 0.10476 | 0.7489 |
GDP_PER_CAPITA does not Granger Cause THE | 15.6075 | 0.0006 | |
EDU does not Granger Cause GDP_PER_CAPITA | 27 | 0.00675 | 0.9352 |
GDP_PER_CAPITA does not Granger Cause EDU | 7.53871 | 0.0110 | |
Pairwise Granger Causality Tests | |||
Sample: 1990 2018 IF COUNTRY1 = 19 | |||
Null Hypothesis: | Obs | F-Statistic | Prob. |
PAN does not Granger Cause GDP_PER_CAPITA | 27 | 0.25761 | 0.6162 |
GDP_PER_CAPITA does not Granger Cause PAN | 7.66646 | 0.0104 | |
PAR does not Granger Cause GDP_PER_CAPITA | 27 | 3.59486 | 0.0696 |
GDP_PER_CAPITA does not Granger Cause PAR | 6.67297 | 0.0160 | |
PRD does not Granger Cause GDP_PER_CAPITA | 27 | 0.04494 | 0.8338 |
GDP_PER_CAPITA does not Granger Cause PRD | 2.59188 | 0.1200 | |
RDE does not Granger Cause GDP_PER_CAPITA | 27 | 9.86050 | 0.0043 |
GDP_PER_CAPITA does not Granger Cause RDE | 1.16887 | 0.2900 | |
STJ does not Granger Cause GDP_PER_CAPITA | 27 | 2.20958 | 0.1497 |
GDP_PER_CAPITA does not Granger Cause STJ | 16.5676 | 0.0004 | |
THE does not Granger Cause GDP_PER_CAPITA | 27 | 0.10476 | 0.7489 |
GDP_PER_CAPITA does not Granger Cause THE | 15.6075 | 0.0006 | |
EDU does not Granger Cause GDP_PER_CAPITA | 27 | 0.00675 | 0.9352 |
GDP_PER_CAPITA does not Granger Cause EDU | 7.53871 | 0.0110 |
- Grossman, G.M.; Helpman, E. Endogenous innovation in the theory of growth. J. Econ. Perspect. 1994 , 8 , 23–44. [ Google Scholar ] [ CrossRef ] [ Green Version ]
- Tew, J.H.; Lee, K.J.X.; Lau, H.C.; Hoh, Y.C.; Woon, S.P. Linkage between the Role of Knowledge and Economic Growth: A Panel Data Analysis. Ph.D. Thesis, UTAR, Kampar, Malaysia, 2017. [ Google Scholar ]
- Hussaini, N. Economic Growth and Higher Education in South Asian Countries: Evidence from Econometrics. Int. J. High. Educ. 2020 , 9 , 118–125. [ Google Scholar ] [ CrossRef ]
- Dusange, P.; Ramanantsoa, B. Technologie Et Stratégie D’entreprise, Édition International ; Ediscience International: Paris, France, 1994; Volume 1, p. 248. [ Google Scholar ]
- Millier, P. Stratégie Et Marketing De L’innovation Technologique-3ème Édition: Lancer Avec Succès Des Produits Qui N’existent Pas Sur Des Marchés Qui N’existent Pas Encore ; Dunod: Paris, France, 2011; Available online: https://www.dunod.com (accessed on 10 January 2022).
- Diaconu, M. Technological innovation: Concept, process, typology and implications in the economy. Theor. Appl. Econ. 2011 , 18. [ Google Scholar ]
- Dodgson, M.; Gann, D.M.; Salter, A. The Management of Technological Innovation: Strategy and Practice ; Oxford University Press on Demand: Oxford, UK, 2008; Available online: https://www.researchgate.net/publication/43478333 (accessed on 10 January 2022).
- Şener, S.; Sarıdoğan, E. The effects of science-technology-innovation on competitiveness and economic growth. Procedia-Soc. Behav. Sci. 2011 , 24 , 815–828. [ Google Scholar ] [ CrossRef ] [ Green Version ]
- Tidd, J.; Bessant, J.; Pavitt, K. Management De L’innovation: Intégration Du Changement Technologique, Commercial Et Organisationnel ; De Boeck Supérieur: Paris, France, 2006; Available online: https://www.lavoisier.fr/livre/economie/management-de-l-innovation-integration-du-changement-technologique-commercial-et-organisationnel/tidd/descriptif_2157630 (accessed on 10 January 2022).
- Atalay, M.; Anafarta, N.; Sarvan, F. The relationship between innovation and firm performance: An empirical evidence from Turkish automotive supplier industry. Procedia-Soc. Behav. Sci. 2013 , 75 , 226–235. [ Google Scholar ] [ CrossRef ] [ Green Version ]
- Zorrilla, D.M.N.; Gracia, T.J.H.; Velazquez, M.D.R.G.; Gracia, J.F.H.; Duran, J.G.I.; Sevilla, J.A.C. Relevance of technological innovation in the business competitiveness of medium enterprises in Hidalgo State. Eur. Sci. J. 2014 , 10 . Available online: https://eujournal.org/index.php/esj/article/view/3532 (accessed on 10 January 2022).
- Rice, C.F.; Yayboke, E. Innovation-Led Economic Growth: Transforming Tomorrow’s Developing Economies through Technology and Innovation ; Rowman & Littlefield: Washington, DC, USA, 2017; Available online: https://www.amazon.com/Innovation-Led-Economic-Growth-Transforming-Developing (accessed on 10 January 2022).
- Mohamed, M.; Liu, P.; Nie, G. Are technological innovation and foreign direct investment a way to boost economic growth? an egyptian case study using the autoregressive distributed lag (ardl) model. Sustainability 2021 , 13 , 3265. [ Google Scholar ] [ CrossRef ]
- Dincer, O. Does corruption slow down innovation? Evidence from a cointegrated panel of US states. Eur. J. Political Econ. 2019 , 56 , 1–10. [ Google Scholar ] [ CrossRef ]
- Broughel, J.; Thierer, A.D. Technological innovation and economic growth: A brief report on the evidence. Mercatus Res. Pap. 2019 . [ Google Scholar ]
- Smith, K.; Estibals, A. Innovation and Research Strategy for Growth. 2011. Available online: https://assets.publishing.service.gov.uk/government/uploads/system/uploads/attachment_data/file/32445/11-1386-economics-innovation-and-research-strategy-for-growth.pdf (accessed on 10 January 2022).
- Jaumotte, F.; Pain, N. Innovation in the Business Sector. 2005. Available online: https://ideas.repec.org/p/oec/ecoaaa/459-en.html (accessed on 10 January 2022).
- Asheim, B. Localised learning, innovation and regional clusters. Clust. Policies–Clust. Dev. 2001 , 39–58. Available online: https://www.sv.uio.no (accessed on 10 January 2022).
- Bhuiyan, A.A.M. Financing education: A route to the development of a country. J. Educ. Dev. 2019 , 7 , 209–217. [ Google Scholar ]
- Porter, M.E. The competitive advantage of nations harvard business review. Harv. Bus. Rev. 1990 , 91. [ Google Scholar ]
- Cooper, R.G. From experience: The invisible success factors in product innovation. J. Prod. Innov. Manag. 1999 , 16 , 115–133. [ Google Scholar ] [ CrossRef ]
- Romer, P.M. Endogenous technological change. J. Political Econ. 1990 , 98 , S71–S102. [ Google Scholar ] [ CrossRef ] [ Green Version ]
- Aghion, P.; Harmgart, H.; Weisshaar, N. Fostering growth in CEE countries: A country-tailored approach to growth policy. In Challenges for European Innovation Policy ; Edward Elgar Publishing: Cheltenham, UK, 2011; Available online: https://www.ebrd.com/downloads/research/economics/workingpapers/wp0118.pdf (accessed on 10 January 2022).
- Aubert, J.-E. Promoting Innovation in Developing Countries: A Conceptual Framework ; World Bank Publications: Washington, DC, USA, 2005; Volume 3554, Available online: https://openknowledge.worldbank.org/handle/10986/8965 (accessed on 10 January 2022).
- Maradana, R.P.; Pradhan, R.P.; Dash, S.; Gaurav, K.; Jayakumar, M.; Chatterjee, D. Does innovation promote economic growth? Evidence from European countries. J. Innov. Entrep. 2017 , 6 , 1–23. [ Google Scholar ] [ CrossRef ] [ Green Version ]
- Sylwester, K. R&D and economic growth. Knowl. Technol. Policy 2001 , 13 , 71–84. [ Google Scholar ]
- Pala, A. Innovation and economic growth in developing countries: Empirical implication of Swamy’s random coefficient model (RCM). Procedia Comput. Sci. 2019 , 158 , 1122–1130. [ Google Scholar ] [ CrossRef ]
- Sadraoui, T.; Ali, T.B.; Deguachi, B. testing for panel granger causality relationship between international R&D cooperation and economic growth. Int. J. Econom. Financ. Manag. 2014 , 2 , 7–21. [ Google Scholar ]
- Freimane, R.; Bāliņa, S. Research and development expenditures and economic growth in the EU: A panel data analysis. Econ. Bus. 2016 , 29 , 5–11. [ Google Scholar ] [ CrossRef ] [ Green Version ]
- Schumpeter, J.A.; Redvers, O. Theorie Der Wirtschaftlichen Entwicklung. The Theory of Economic Development. An Inquiry into Profits, Capital, Credit, Interest, and the Business Cycle; Redvers Opie. 1934. Available online: https://www.hup.harvard.edu/catalog.php?isbn=9780674879904 (accessed on 10 January 2022).
- ÇETİN, M. The hypothesis of innovation-based economic growth: A causal relationship. Uluslararası İktisadi ve İdari İncelemeler Dergisi 2013 , 1–16. [ Google Scholar ]
- Antonelli, C. The economics of innovation: From the classical legacies to the economics of complexity. Econ. Innov. New Technol. 2009 , 18 , 611–646. [ Google Scholar ] [ CrossRef ]
- Conte, A. The Evolution of the Literature on Technological Change over Time: A Survey. 2006. Available online: https://www.researchgate.net/publication/5018301 (accessed on 10 January 2022).
- Solow, R.M. Technical change and the aggregate production function. Rev. Econ. Stat. 1957 , 39 , 312–320. [ Google Scholar ] [ CrossRef ] [ Green Version ]
- Sofuoğlu, E.; Kizilkaya, O.; Koçak, E. Assessing the impact of high-technology exports on the growth of the turkish economy. J. Econ. Policy Res. 2022 , 9 , 205–229. [ Google Scholar ] [ CrossRef ]
- Idris, J.; Yusop, Z.; Habibullah, M.S. Trade openness and economic growth: A causality test in panel perspective. Int. J. Bus. Soc. 2016 , 17. [ Google Scholar ] [ CrossRef ]
- Zahonogo, P. Trade and economic growth in developing countries: Evidence from sub-Saharan Africa. J. Afr. Trade 2016 , 3 , 41–56. [ Google Scholar ] [ CrossRef ]
- Romer, P.M. Increasing returns and long-run growth. J. Political Econ. 1986 , 94 , 1002–1037. [ Google Scholar ] [ CrossRef ] [ Green Version ]
- Lucas, R.E., Jr. On the mechanics of economic development. J. Monet. Econ. 1988 , 22 , 3–42. [ Google Scholar ] [ CrossRef ]
- Guloglu, B.; Tekin, R.B. A panel causality analysis of the relationship among research and development, innovation, and economic growth in high-income OECD countries. Eurasian Econ. Rev. 2012 , 2 , 32–47. [ Google Scholar ]
- Romer, P.M. The origins of endogenous growth. J. Econ. Perspect. 1994 , 8 , 3–22. [ Google Scholar ] [ CrossRef ] [ Green Version ]
- Jones, C.I. Paul Romer: Ideas, nonrivalry, and endogenous growth. Scand. J. Econ. 2019 , 121 , 859–883. [ Google Scholar ] [ CrossRef ]
- Aghion, P.; Ljungqvist, L.; Howitt, P.; Howitt, P.W.; Brant-Collett, M.; García-Peñalosa, C. Endogenous Growth Theory ; MIT Press: Cambridge, MA, USA, 1998; Available online: https://mitpress.mit.edu/books/endogenous-growth-theory (accessed on 10 January 2022).
- Zeng, J. Reexamining the interaction between innovation and capital accumulation. J. Macroecon. 2003 , 25 , 541–560. [ Google Scholar ] [ CrossRef ]
- Chu, S.-Y. Internet, economic growth and recession. Sci. Res. 2013 , 4 , 3A. [ Google Scholar ] [ CrossRef ] [ Green Version ]
- Nelson, R.R. Why do firms differ, and how does it matter? Strateg. Manag. J. 1991 , 12 , 61–74. [ Google Scholar ] [ CrossRef ]
- Kim, L. Imitation to Innovation: The Dynamics of Korea’s Technological Learning ; Harvard Bus School Press: Boston, MA, USA, 1997. [ Google Scholar ]
- Nelson, R.R. National Innovation Systems: A Comparative Analysis ; Oxford University Press on Demand: Oxford, UK, 1993; Available online: https://www.academia.edu/28567904 (accessed on 10 January 2022).
- Carlo, P.P.; Vandana, C.; Deniz, E. Innovation and Growth Chasing a Moving Frontier: Chasing a Moving Frontier ; OECD Publishing: Washington, DC, USA, 2009; Available online: https://www.oecd.org/innovation/innovationandgrowthchasingamovingfrontier.htm (accessed on 10 January 2022).
- Hargadon, A.; Sutton, R.I. Technology brokering and innovation in a product development firm. Adm. Sci. Q. 1997 , 716–749. [ Google Scholar ] [ CrossRef ]
- Fagerberg, J.; Srholec, M. National innovation systems, capabilities and economic development. Res. Policy 2008 , 37 , 1417–1435. [ Google Scholar ] [ CrossRef ]
- Arocena, R.; Sutz, J. Research and innovation policies for social inclusion: An opportunity for developing countries. Innov. Dev. 2012 , 2 , 147–158. [ Google Scholar ] [ CrossRef ]
- Freeman, C. Continental, national and sub-national innovation systems—Complementarity and economic growth. Res. Policy 2002 , 31 , 191–211. [ Google Scholar ] [ CrossRef ]
- Castellacci, F.; Natera, J.M. The dynamics of national innovation systems: A panel cointegration analysis of the coevolution between innovative capability and absorptive capacity. Res. Policy 2013 , 42 , 579–594. [ Google Scholar ] [ CrossRef ] [ Green Version ]
- Wu, Y. Innovation and Economic Growth in China. Business School the University of Western Australia. DISCUSSION PAPER 10.10. VAL. TECH. 2012. Available online: https://econpapers.repec.org/paper/uwawpaper/10-10.htm (accessed on 10 January 2022).
- Tuna, K.; Kayacan, E.; Bektaş, H. The relationship between research & development expenditures and economic growth: The case of Turkey. Procedia-Soc. Behav. Sci. 2015 , 195 , 501–507. [ Google Scholar ]
- Abdelaoui, T.M.L.; Abdelaoui, O. The impact of innovation on economic development in Arab countries: The Case of Selected Arab Countries from 2007 to 2016. J.N. Afr. Econ. 2020 , 16 , 33–54. [ Google Scholar ]
- Lomachynska, I.; Podgorna, I. Innovation potential: Impact on the national economy’s competitiveness of the EU developed countries. Balt. J. Econ. Stud. 2018 , 4 , 262–270. [ Google Scholar ] [ CrossRef ]
- Pece, A.M.; Simona, O.E.O.; Salisteanu, F. Innovation and economic growth: An empirical analysis for CEE countries. Procedia Econ. Financ. 2015 , 26 , 461–467. [ Google Scholar ] [ CrossRef ] [ Green Version ]
- Solomon, O.; Samuel, J.; Samuel, A. Study of the relationship between economic growth, volatility and innovation for the Eu-27 and ceec countries. J. Inf. Syst. Oper. Manag. 2011 , 5 , 82–90. [ Google Scholar ]
- Economists Understand Little about the Causes of Growth, 2 April 2018. Available online: https://www.economist.com/finance-and-economics/2018/04/12/economists-understand-little-about-the-causes-of-growth (accessed on 12 April 2018).
- Yang, C.-H. Is innovation the story of Taiwan’s economic growth? J. Asian Econ. 2006 , 17 , 867–878. [ Google Scholar ] [ CrossRef ]
- Pradhan, R.P.; Arvin, M.B.; Hall, J.H.; Nair, M. Innovation, financial development and economic growth in Eurozone countries. Appl. Econ. Lett. 2016 , 23 , 1141–1144. [ Google Scholar ] [ CrossRef ]
- Sinha, D. Patents, Innovations and economic Growth in Japan and South Korea: Evidence from individual country and Panel Data. Appl. Econom. Int. Dev. 2008 , 8 . Available online: https://papers.ssrn.com/sol3/papers.cfm?abstract_id=1308261 (accessed on 12 April 2018).
- Sadraoui, T.; Ali, T.B.; Deguachi, B. Economic growth and international R&D cooperation: A panel granger causality analysis. Int. J. Econom. Financ. Manag. 2014 , 2 , 7–21. [ Google Scholar ]
- Fagerberg, J.; Srholec, M.; Knell, M. The competitiveness of nations: Why some countries prosper while others fall behind. World Dev. 2007 , 35 , 1595–1620. [ Google Scholar ] [ CrossRef ]
- Kuo, H.-C.; Tseng, Y.-C.; Yang, Y.-T.C. Promoting college student’s learning motivation and creativity through a STEM interdisciplinary PBL human-computer interaction system design and development course. Think. Ski. Creat. 2019 , 31 , 1–10. [ Google Scholar ] [ CrossRef ]
- Aghion, P.; Howitt, P. A Model of Growth through Creative Destruction ; National Bureau of Economic Research: Cambridge, MA, USA, 1990; pp. 898–2937. Available online: https://dash.harvard.edu/bitstream/handle/1/12490578/A%20Model%20of%20Growth%20through%20Creative%20Destruction.pdf (accessed on 12 April 2018).
- Silva, M.A.P.M.d. Teaching Aghion and Howitt’s model of schumpeterian growth to graduate students: A diagrammatic approach. Australas. J. Econ. Educ. 2012 , 9 , 15–36. [ Google Scholar ]
- Stiglitz, J.E. Capital-market liberalization, globalization, and the IMF. Oxf. Rev. Econ. Policy 2004 , 20 , 57–71. [ Google Scholar ] [ CrossRef ]
- Hsiao, C. Analysis of Panel Data ; Cambridge University Press: Cambridge, UK, 2014; Available online: https://doi.org/10.1017/CBO9781139839327 (accessed on 12 April 2018).
- Baltagi, B.H.; Song, S.H.; Koh, W. Testing panel data regression models with spatial error correlation. J. Econom. 2003 , 117 , 123–150. [ Google Scholar ] [ CrossRef ]
- Narayan, P.K.; Smyth, R. Energy consumption and real GDP in G7 countries: New evidence from panel cointegration with structural breaks. Energy Econ. 2008 , 30 , 2331–2341. [ Google Scholar ] [ CrossRef ]
- Dimitrios, A.; Stephen, G. Hall, Applied Econometrics: A Modern Approach, Revised ; Palgrave Macmillan: London, UK, 2007; Available online: https://www.pdfdrive.com/applied-econometrics-a-modern-approach-using-eviews-and-microfit-revised-edition-d157033339.html (accessed on 12 April 2018).
- MacKinnon, J.G. Numerical distribution functions for unit root and cointegration tests. J. Appl. Econom. 1996 , 11 , 601–618. [ Google Scholar ] [ CrossRef ] [ Green Version ]
- Han, L.; Cui, W.; Zhang, W. A Relevance Study of Economic Time Series Data. In Proceedings of the LISS 2020: The 10th International Conference on Logistics, Informatics and Service Sciences ; Springer: Berlin/Heidelberg, Germany, 2021; p. 405. [ Google Scholar ] [ CrossRef ]
- Dickey, D.A.; Fuller, W.A. Likelihood ratio statistics for autoregressive time series with a unit root. Econom. J. Econom. Soc. 1981 , 83 , 1057–1072. Available online: https://econpapers.repec.org/article/ecmemetrp/v_3a49_3ay_3a1981_3ai_3a4_3ap_3a1057-72.htm (accessed on 12 April 2018). [ CrossRef ]
- Yaya, O.S.; Ogbonna, A.E.; Furuoka, F.; Gil-Alana, L.A. A New Unit Root Test for Unemployment Hysteresis Based on the Autoregressive Neural Network. Oxf. Bull. Econ. Stat. 2021 , 83 , 960–981. [ Google Scholar ] [ CrossRef ]
- Engle, R.F.; Granger, C.W.J. Co-integration and Error Correction: Representation, Estimation, and Testing. Econom. J. Econom. Soc. 1987 , 55 , 176–251. [ Google Scholar ] [ CrossRef ]
- Padder, A.H.; Mathavan, B. The Relationship between Unemployment and Economic Growth in India: Granger Causality Approach. NVEO-Nat. Volatiles Essent. Oils J. NVEO 2021 , 8 , 1265–1271. [ Google Scholar ]
- Naimoğlu, M. Impact on Economic Growth of Energy Consumption and Foreign Direct Investment: The Case of Turkey, Conference: 7. In Proceedings of the International Conference on Economics (IceTea2021): Unpacking the Economic Impacts of COVID-1; Available online: https://teacongress.com/ (accessed on 10 January 2022).
- Granger, C.W. Investigating causal relations by econometric models and cross-spectral methods. Econom. J. Econom. Soc. 1969 , 37 , 424–438. [ Google Scholar ] [ CrossRef ]
- Damodar, N.G. Basic Econometrics ; The Mc-Graw Hill: New York, NY, USA, 2004; Available online: https://www.academia.edu/40263427/BASIC_ECONOMETRICS_FOURTH_EDITION (accessed on 12 April 2018).
- Husain, F.; Abbas, K. Money, Income, Prices, and Causality in Pakistan. A Trivariate Analysis. PIDE-Working Papers 2000:178, Pakistan Institute of Development Economics. 2020. Available online: https://ideas.repec.org/p/pid/wpaper/2000178.html (accessed on 10 January 2022).
- Gujarati, D.N. Basic Econometrics , 4th ed.; McGraw-Hill: Singapore, 2003; Available online: http://zalamsyah.staff.unja.ac.id/wp-content/uploads/sites/286/2019/11/7-Basic-Econometrics-4th-Ed.-Gujarati.pdf (accessed on 12 April 2018).
- Liang, Z. Financial development and income distribution: A system GMM panel analysis with application to urban China. J. Econ. Dev. 2006 , 31 , 1. [ Google Scholar ]
- Pham, T.T.; Dao, L.K.O.; Nguyen, V.C. The determinants of bank’s stability: A system GMM panel analysis. Cogent Bus. Manag. 2021 , 8 , 1963390. [ Google Scholar ] [ CrossRef ]
- Oppong, G.K.; Pattanayak, J.K.; Irfan, M. Impact of intellectual capital on productivity of insurance companies in Ghana: A panel data analysis with system GMM estimation. J. Intellect. Cap. 2019 , 20 , 763–783. [ Google Scholar ] [ CrossRef ]
Click here to enlarge figure
Indices | PAR | PAN | EDU | RDE | PRD | STJ | THE | GDP_PER_CAPITA_GROWT |
---|---|---|---|---|---|---|---|---|
Mean | 42.79799 | 55.28742 | 0.135374 | 0.022152 | 45.21019 | 153.5121 | 0.282907 | 2.974121 |
Median | 15.97560 | 21.55963 | 0.097227 | 0.007254 | 9.439463 | 73.27613 | 0.104898 | 3.253375 |
Maximum | 1232.796 | 1524.110 | 1.269957 | 0.533769 | 660.2663 | 1966.061 | 2.879151 | 13.63634 |
Minimum | 0.227269 | 0.905273 | 0.000000 | 4.14 × 10 | 0.281453 | 0.689034 | 0.001584 | −14.35055 |
Std. Dev. | 85.36316 | 120.7277 | 0.157449 | 0.041215 | 103.6293 | 206.7747 | 0.483565 | 3.840783 |
Skewness | 7.890280 | 7.811967 | 2.788900 | 5.492087 | 3.643645 | 2.860077 | 3.270931 | −0.767087 |
Kurtosis | 93.38687 | 77.77401 | 14.05681 | 54.31703 | 17.32591 | 16.78431 | 14.29189 | 4.782198 |
Jarque-Bera | 203454.6 | 141018.8 | 3706.320 | 66557.17 | 6243.125 | 5382.580 | 4115.652 | 133.6397 |
Probability | 0.000000 | 0.000000 | 0.000000 | 0.000000 | 0.000000 | 0.000000 | 0.000000 | 0.000000 |
Sum | 24822.83 | 32066.70 | 78.51690 | 12.84793 | 26221.91 | 89037.02 | 164.0861 | 1724.990 |
Sum Sq. Dev. | 4219097. | 8439034. | 14.35357 | 0.983534 | 6217899. | 24755592 | 135.3906 | 8541.184 |
Observations | 580 | 580 | 580 | 580 | 580 | 580 | 580 | 580 |
Items | GDP_PER_CAPITA_GROWTH__A | PAR | PAN | RDE | PRD | STJ | THE | EDU |
---|---|---|---|---|---|---|---|---|
GDP_PER_CAPITA_GROWT | 1.000000 | −0.004692 | −0.047889 | 0.011245 | 0.061354 | 0.038487 | 0.056533 | −0.009516 |
PAR | −0.004692 | 1.000000 | 0.746965 | 0.204002 | 0.149051 | 0.324532 | 0.311667 | 0.277678 |
PAN | −0.047889 | 0.746965 | 1.000000 | 0.217452 | 0.142241 | 0.402496 | 0.367943 | 0.441065 |
RDE | 0.011245 | 0.204002 | 0.217452 | 1.000000 | 0.583682 | 0.389245 | 0.494083 | 0.635408 |
PRD | 0.061354 | 0.149051 | 0.142241 | 0.583682 | 1.000000 | 0.539553 | 0.797042 | 0.739735 |
STJ | 0.038487 | 0.324532 | 0.402496 | 0.389245 | 0.539553 | 1.000000 | 0.501469 | 0.552201 |
THE | 0.056533 | 0.311667 | 0.367943 | 0.494083 | 0.797042 | 0.501469 | 1.000000 | 0.685608 |
EDU | −0.009516 | 0.277678 | 0.441065 | 0.635408 | 0.739735 | 0.552201 | 0.685608 | 1.000000 |
Country | PARLV (FD) | PANLV (FD) | RDELV (FD) | PRDLV (FD) | THELV (FD) | STJLV (FD) | EDULV (FD) | GDP per Capita Growth (Annual %) LV (FD) |
---|---|---|---|---|---|---|---|---|
Algeria | 2.158 (20.7 ***) | 2.206 (24.03 ***) | 22.12 *** | 0.217 (34.836) | 8.37 ** | 5.7 × 10 15.7 *** | 12.17 *** | 1.798 (17.034 ***) |
Argentina | 18.42 *** | 18.42 *** | 20.12 *** | 18.79 *** | 19.445 *** | 18.42 *** | 18.46 *** | 0.289 (14.99 ***) |
Brazil | 6.373 ** | 1.127 (13.05 ***) | 1.17 (23.2 ***) | 1.287 (31.22 ***) | 5.298 * (8.62 ***) | 0.00784 (16.87 ***) | 5.64 * (26.29 ***) | 0.573 (8.63 **) |
Bulgaria | 0.911 (17.6 ***) | 0.911 (17.6 ***) | 2.503 (22.9 ***) | 0.031 (14.86 ***) | 0.0237 (15.06 ***) | 0.02188 (20.067 ***) | 1.535 (24.89 ***) | 0.99145 (7.67 **) |
Chile | 7.498 ** | 7.498 ** | 0.212 (15.9 ****) | 1.59 (34.1 ***) | 1.813 (25.85 ***) | 0.0025 (18.42 ***) | 1.36 (7.22 ***) | 0.7916 (6.304 **) |
China | (13.6 ***) | 13.63 *** | 0.036 (19.8 ***) | 0.319 (16.9 ***) | 1.9007 (6.3 ***) | 0.0011 (14.085 ***) | 7.43 ** | 0.3077 (15.12 ***) |
Egypt | 2.52 (16.96 ***) | 5.52 (16.97 ***) | 9.553 *** | 3.96 (18.95 ***) | 12.8 *** | 0.01555 (33.65 ***) | 4.826 * (18.42 ***) | 2.2902 (21.65 ***) |
Hungary | 0.9131 (8.27 **) | 2.37 (8.27 **) | 2.5 (14.47 ***) | 0.227 (19.47 ***) | 2.05 (13.25 ***) | 1.83 (23.47 ***) | 1.79 (9.62 ***) | 0.02018 (19.29 ***) |
Indonesia | 3.011 (23.8 ***) | 3.011 (26.5 ***) | 1.39 (32.5 ***) | 2.9 (29.86 ***) | 2.33 (18.86 ***) | 0.0039 (24.82 ***) | 3.0074 (25.9 ***) | 0.40005 (18.42 ***) |
Iran | 0.544 (19.3 ***) | 0.544 (19.3 ***) | 17.08 *** | 4.97 * (17.47 ***) | 2.24 (21.17 ***) | 0.01927 (6.911 **) | 1.039 (20.83 ***) | 4.568 (11.74 ***) |
Mexico | 16.57 *** | 16.57 *** | 1.79 (22.4 ***) | 17.025 *** | 16.0697 *** | 15.74 *** | 16.56 *** | 2.839 (15.006 ***) |
Morocco | 0.00061 (6.66**) | 0.00061 (6.66 **) | 18.4 *** | 0.00053 (15.73 ***) | 1.56 (11.16 ***) | 2.0E-05 (31.9 ***) | 9.016 ** | 0.233 (11.956 ***) |
Peru | 4.08 (15.9 ***) | 5.212 * (16.18 ***) | 8.1 ** | 3.34 (13.67 ***) | 5.11 * (18.74 ***) | 3.1E09 (34.064 ***) | 5.234 * (30.51 ***) | 0.17077 (9.222 ***) |
Philippines | 0.13564 (8.71 ***) | 9.708 ** | 0.143 8.4 ** | 1.37 (17.6 ***) | 1.28 (9.38 ***) | 5.4E-08 (24.77 ***) | 2.799 (15.55 ***) | 0.1331 (13.0118 ***) |
Poland | 0.5878 (12.94 ***) | 0.859 (12.78 ***) | 0.143 (8.4 ***) | 13.22 *** | 0.0338 (10.33 ***) | 0.024 (16.76 ***) | 5.83 * (11.42 ***) | 2.9957 (13.548 ***) |
Romania | 14.93 *** | 0.45044 (22.47 ***) | 5.62 * (27.7 ***) | 40.25 *** | 1.288 (10.96 ***) | 0.44 (12.8 ***) | 5.38 * (31.14 ***) | 0.10418 (11.128 ***) |
Sri Lanka | 0.816 (33.47 ***) | 2.433 (20.9 ***) | 3.22 (13.8 ***) | 0.618 (13.98 ***) | 4.37 (10.6 ***) | 6.2E-07 (7.06 **) | 1.88 (22.42 ***) | 0.03594 (6.326 **) |
Thailand | 2.05 (26.26 ***) | 6.65 ** | 1.46 (6.003 **) | 3.5E-06 (18.97 ***) | 6.11573** | 0.0002 (13.32 ***) | 11.55 ** | 0.2262 (7.845 ***) |
Tunisia | 0.823 (22.3 ***) | 0.76 (8.196 ***) | 1.066 (9.85 ***) | 0.13077 (11.196 ***) | 0.583 (23.55 ***) | 0.00112 (28.24 ***) | 3.68 (27.87 ***) | 2.050 (11.174 ***) |
Turkey | 0.0064 (12.72**) | 2.841 (7.123 **) | 1.137 (22.7 ***) | 0.01690 (15.05 ***) | 6.077 ** | 0.313 (30.084 ***) | 2.66 (30.33 ***) | 0.528 (16.75 ***) |
Variables | PAR | PAN | RDE | PRD | THE | STJ | EDU | GDP |
---|---|---|---|---|---|---|---|---|
Degree of integration | I (1) | I (1) | I (1) | I (1) | I (1) | I (1) | I (1) | I (1) |
Null Hypothesis: | Algeria | Argentina | Brazil | Bulgaria | Chile | |
---|---|---|---|---|---|---|
PAN | PAN does not Granger Cause GDP_PER_CAPITA | 3.45139 ** | 1.85640 | 0.02088 | 0.55365 | 6.42670 ** |
GDP_PER_CAPITA does not Granger Cause PAN | 0.65959 | 1.20757 | 3.50598 ** | 4.24517 ** | 0.16010 | |
PAR | PAR does not Granger Cause GDP_PER_CAPITA | 0.28866 | 2.75374 * | 1.05750 | 0.55365 | 6.42670 ** |
GDP_PER_CAPITA does not Granger Cause PAR | 0.54733 | 1.69911 | 0.92123 | 4.24517 ** | 0.16010 | |
PRD | PRD does not Granger Cause GDP_PER_CAPITA | 0.41770 | 3.75307 ** | 0.61420 | 2.08852 | 0.93974 |
GDP_PER_CAPITA does not Granger Cause PRD | 1.35729 | 2.52407 * | 3.58595 ** | 1.31153 | 0.70005 | |
RDE | RDE does not Granger Cause GDP_PER_CAPITA | 0.19865 | 3.80148 ** | 0.93278 | 2.25745 | 0.25433 |
GDP_PER_CAPITA does not Granger Cause RDE | 0.11666 | 1.31620 | 1.57160 | 1.08045 | 4.69006 ** | |
STJ | STJ does not Granger Cause GDP_PER_CAPITA | 0.88593 | 3.80122 ** | 3.85557 ** | 24.2234 *** | 0.70643 |
GDP_PER_CAPITA does not Granger Cause STJ | 0.72180 | 2.78491* | 0.82624 | 0.47384 | 8.69955 *** | |
THE | THE does not Granger Cause GDP_PER_CAPITA | 5.55960 ** | 2.11193 | 0.48506 | 9.65160 *** | 2.18918 |
GDP_PER_CAPITA does not Granger Cause THE | 0.87955 | 1.21826 | 0.26147 | 1.68544 | 5.95758 ** | |
EDU | EDU does not Granger Cause GDP_PER_CAPITA | 0.06448 | 2.50999 * | 3.45232 ** | 0.17195 | 0.54776 |
GDP_PER_CAPITA does not Granger Cause EDU | 0.08922 | 1.46903 | 3.39050 * | 2.04251 | 4.74801 ** | |
Null Hypothesis: | China | Egypt | Hungary | Indonesia | Iran | |
PAN | PAN does not Granger Cause GDP_PER_CAPITA | 0.07652 | 7.85133 *** | 0.11664 | 0.60294 | 3.28536 * |
GDP_PER_CAPITA does not Granger Cause PAN | 4.13486 ** | 0.05001 | 1.36300 | 5.20243 ** | 0.25676 | |
PAR | PAR does not Granger Cause GDP_PER_CAPITA | 0.07652 | 7.85133 *** | 0.11664 | 0.60294 | 3.28536 * |
GDP_PER_CAPITA does not Granger Cause PAR | 4.13486 ** | 0.05001 | 1.36300 | 5.20243 ** | 0.25676 | |
PRD | PRD does not Granger Cause GDP_PER_CAPITA | 1.59115 | 1.01360 | 1.23194 | 4.82837 ** | 8.85677 *** |
GDP_PER_CAPITA does not Granger Cause PRD | 1.71315 | 0.50835 | 6.58574 ** | 0.11963 | 0.09699 | |
RDE | RDE does not Granger Cause GDP_PER_CAPITA | 0.63068 | 0.12053 | 4.35234 ** | 0.01557 | 9.04547 *** |
GDP_PER_CAPITA does not Granger Cause RDE | 6.14284 *** | 6.87595 ** | 53.1925 *** | 0.51660 | 0.07771 | |
STJ | STJ does not Granger Cause GDP_PER_CAPITA | 4.29613 ** | 0.02424 | 4.47252 ** | 0.02185 | 3.93442 * |
GDP_PER_CAPITA does not Granger Cause STJ | 1.72200 | 1.20223 | 2.96970 * | 2.43003 | 0.50925 | |
THE | THE does not Granger Cause GDP_PER_CAPITA | 5.98152 ** | 0.06169 | 3.48594 * | 1.54441 | 4.39407 ** |
GDP_PER_CAPITA does not Granger Cause THE | 0.04888 | 1.60651 | 1.65908 | 6.48804 *** | 0.07888 | |
EDU | EDU does not Granger Cause GDP_PER_CAPITA | 0.08946 | 18.0285 *** | 1.07799 | 1.45638 | 2.43955 |
GDP_PER_CAPITA does not Granger Cause EDU | 0.13876 | 6.94753 ** | 0.00019 | 0.39204 | 3.25965 * | |
Null Hypothesis: | Mexico | Morocco | Peru | Philippines | Poland | |
PAN | PAN does not Granger Cause GDP_PER_CAPITA | 0.33389 | 0.89077 | 0.97908 | 1.32599 | 3.94050 ** |
GDP_PER_CAPITA does not Granger Cause PAN | 0.11052 | 3.30388 ** | 0.66307 | 0.14147 | 0.95690 | |
PAR | PAR does not Granger Cause GDP_PER_CAPITA | 0.33389 | 0.89077 | 0.97908 | 1.37618 | 0.58816 |
GDP_PER_CAPITA does not Granger Cause PAR | 0.11052 | 3.30388 ** | 0.66307 | 1.62187 | 2.94843 * | |
PRD | PRD does not Granger Cause GDP_PER_CAPITA | 0.28754 | 0.45675 | 0.27895 | 0.33509 | 0.26065 |
GDP_PER_CAPITA does not Granger Cause PRD | 0.09579 | 1.24709 | 0.45400 | 0.69119 | 0.80394 | |
RDE | RDE does not Granger Cause GDP_PER_CAPITA | 0.31281 | 3.90725 ** | 0.91969 | 4.55742 ** | 1.05090 |
GDP_PER_CAPITA does not Granger Cause RDE | 0.18231 | 0.69901 | 0.36466 | 0.67502 | 3.07919 * | |
STJ | STJ does not Granger Cause GDP_PER_CAPITA | 0.26862 | 0.74834 | 0.09426 | 0.15912 | 2.19014 |
GDP_PER_CAPITA does not Granger Cause STJ | 1.67510 | 3.48123 ** | 5.11692 ** | 0.41448 | 0.18275 | |
THE | THE does not Granger Cause GDP_PER_CAPITA | 0.29089 | 1.98610 | 0.93801 | 1.78683 | 0.81335 |
GDP_PER_CAPITA does not Granger Cause THE | 0.01289 | 1.64873 | 0.91330 | 0.26876 | 4.74172 ** | |
EDU | EDU does not Granger Cause GDP_PER_CAPITA | 0.32060 | 0.59479 | 0.03313 | 3.61820 ** | 0.04317 |
GDP_PER_CAPITA does not Granger Cause EDU | 0.02119 | 1.69819 | 0.17291 | 0.98913 | 1.17654 | |
Null Hypothesis: | Romania | Sri Lanka | Thailand | Tunisia | Turkey | |
PAN | PAN does not Granger Cause GDP_PER_CAPITA | 2.56142 | 0.13404 | 4.52198 ** | 0.25761 | 5.64758 ** |
GDP_PER_CAPITA does not Granger Cause PAN | 6.73806 *** | 0.34464 | 1.67446 | 7.66646 *** | 1.47880 | |
PAR | PAR does not Granger Cause GDP_PER_CAPITA | 2.40498 | 1.79948 | 1.14622 | 3.5948 * | 0.36393 |
GDP_PER_CAPITA does not Granger Cause PAR | 0.14059 | 9.57892 *** | 0.80727 | 6.67297 ** | 2.40591 | |
PRD | PRD does not Granger Cause GDP_PER_CAPITA | 0.85992 | 1.56537 | 1.54357 | 0.04494 | 0.77162 |
GDP_PER_CAPITA does not Granger Cause PRD | 0.29628 | 1.02108 | 1.21407 | 2.59188 | 1.20477 | |
RDE | RDE does not Granger Cause GDP_PER_CAPITA | 1.02146 | 0.27437 | 0.76778 | 9.86050 *** | 0.29961 |
GDP_PER_CAPITA does not Granger Cause RDE | 0.13856 | 1.95295 | 2.69477 * | 1.16887 | 4.26462 ** | |
STJ | STJ does not Granger Cause GDP_PER_CAPITA | 9.11119 *** | 0.98285 | 6.39219 *** | 2.20958 | 8.52400 *** |
GDP_PER_CAPITA does not Granger Cause STJ | 19.9675 *** | 0.50109 | 4.38578 ** | 16.5676 *** | 0.25703 | |
THE | THE does not Granger Cause GDP_PER_CAPITA | 0.35056 | 1.00865 | 8.52687 *** | 0.10476 | 1.62364 |
GDP_PER_CAPITA does not Granger Cause THE | 11.8021 *** | 1.46224 | 0.56740 | 15.6075 *** | 1.12907 | |
EDU | EDU does not Granger Cause GDP_PER_CAPITA | 2.68070 | 0.59774 | 5.85179 *** | 0.00675 | 0.41114 |
GDP_PER_CAPITA does not Granger Cause EDU | 2.94423 | 0.34051 | 0.02474 | 7.53871 ** | 1.06322 |
Variable | Statistic | Asymp. Sig. (Two-Tailed) |
---|---|---|
GDP per capita growth | 12.326 | 0.0021 |
Variable | Coefficient | Standard Error | t-Statistic | Probability |
---|---|---|---|---|
EDU | −3.500127 | 0.390585 | −8.961248 | 0.0000 |
PAN | −0.000345 | 0.001832 | −0.188131 | 0.8508 |
PAR | 0.000147 | 0.001754 | 0.084037 | 0.9331 |
PRD | 0.002690 | 0.001332 | 2.019151 | 0.0440 |
RDE | −0.686891 | 1.316314 | −0.521829 | 0.6020 |
STJ | 0.004503 | 0.001514 | 2.974776 | 0.0031 |
THE | 0.740715 | 0.245014 | 3.023156 | 0.0026 |
Effects Specification | ||||
Cross-section fixed (first differences) | ||||
Mean dependent var | 0.047455 | S.D. dependent var | 0.206800 | |
S.E. of regression | 0.501957 | Sum squared resid | 139.3344 | |
J-statistic | 13.90493 | Instrument rank | 21 | |
Prob (J-statistic) | 0.456818 |
Test order | m-Statistic | rho | SE (rho) | Prob. |
---|---|---|---|---|
AR (2) | −0.011694 | −0.320535 | 27.409643 | 0.9907 |
MDPI stays neutral with regard to jurisdictional claims in published maps and institutional affiliations. |
Share and Cite
Mohamed, M.M.A.; Liu, P.; Nie, G. Causality between Technological Innovation and Economic Growth: Evidence from the Economies of Developing Countries. Sustainability 2022 , 14 , 3586. https://doi.org/10.3390/su14063586
Mohamed MMA, Liu P, Nie G. Causality between Technological Innovation and Economic Growth: Evidence from the Economies of Developing Countries. Sustainability . 2022; 14(6):3586. https://doi.org/10.3390/su14063586
Mohamed, Maha Mohamed Alsebai, Pingfeng Liu, and Guihua Nie. 2022. "Causality between Technological Innovation and Economic Growth: Evidence from the Economies of Developing Countries" Sustainability 14, no. 6: 3586. https://doi.org/10.3390/su14063586
Article Metrics
Article access statistics, further information, mdpi initiatives, follow mdpi.
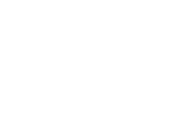
Subscribe to receive issue release notifications and newsletters from MDPI journals
Click through the PLOS taxonomy to find articles in your field.
For more information about PLOS Subject Areas, click here .
Loading metrics
Open Access
Peer-reviewed
Research Article
Re-assessing causality between energy consumption and economic growth
Roles Conceptualization, Data curation, Formal analysis, Funding acquisition, Investigation, Methodology, Project administration, Resources, Software, Supervision, Validation, Visualization, Writing – original draft, Writing – review & editing
Affiliation Newcastle University, Newcastle, United Kingdom
* E-mail: [email protected]
Affiliation Universidad Isabel I. Calle de Fernán González, Burgos, Spain

Affiliation IEI and Universitat Jaume I, Campus del Riu Sec, Castellón, Spain
- Atanu Ghoshray,
- Yurena Mendoza,
- Mercedes Monfort,
- Javier Ordoñez
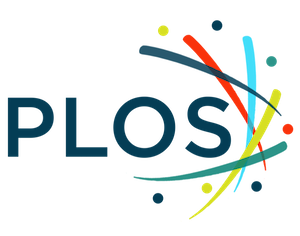
- Published: November 12, 2018
- https://doi.org/10.1371/journal.pone.0205671
- Reader Comments
The energy consumption-growth nexus has been widely studied in the empirical literature, though results have been inconclusive regarding the direction, or even the existence, of causality. These inconsistent results can be explained by two important limitations of the literature. First, the use of bivariate models, which fail to detect more complex causal relations, or the ad hoc approach to selecting variables in a multivariate framework; and, second, the use of linear causal models, which are unable to capture more complex nonlinear causal relationships. In this paper, we aim to overcome both limitations by analysing the energy consumption-growth nexus using a Flexible Fourier form due to Enders and Jones (2016). The analysis focuses on the US over the period 1949 to 2014. From our results we can conclude that, where the linear methodology supports the neutrality hypothesis (no causality between energy consumption and growth), the Flexible Fourier form points to the existence of causality from energy consumption to growth. This is contrary to the linear analysis, suggesting that lowering energy consumption would adversely affect US economic growth. Thus, by employing the Flexible Fourier form we find the conclusions can be quite different.
Citation: Ghoshray A, Mendoza Y, Monfort M, Ordoñez J (2018) Re-assessing causality between energy consumption and economic growth. PLoS ONE 13(11): e0205671. https://doi.org/10.1371/journal.pone.0205671
Editor: María Carmen Díaz Roldán, Universidad de Castilla-La Mancha, SPAIN
Received: April 8, 2018; Accepted: September 29, 2018; Published: November 12, 2018
Copyright: © 2018 Ghoshray et al. This is an open access article distributed under the terms of the Creative Commons Attribution License , which permits unrestricted use, distribution, and reproduction in any medium, provided the original author and source are credited.
Data Availability: All relevant data are available at https://doi.org/10.5061/dryad.4h92834 .
Funding: JO acknowledges financial support from the Spanish Ministry of Science and Innovation through grant ECO2014-58991-C3-2-R and AEI/FEDER ECO2017-83255-C3-3-P. JO acknowledges financial support from PROMETEO project PROMETEO II/2014/053. AG and JO thank the Generalitat Valenciana project AICO/2016/038. JO and MM are grateful for support from the University Jaume I research project P1.1B2014-7 and UJI-B2017-33. YM is grateful for support from the University Isabel I research project. The funders had no role in study design, data collection and analysis, decision to publish, or preparation of the manuscript.
Competing interests: The authors have declared that no competing interests exist.
Introduction
It has been argued that economic growth may exhaust resources and cause environmental degradation [ 1 ], compromising future growth. The fear that today's growth can cause other significant economic problems, especially for future generations, has propelled sustainable growth to the top of the political agenda for the vast majority of developed countries. Sustainability will depend on how the substitutability or complementarity between energy and production factors and the interplay with technical progress and productivity, impact economic growth. Consequently, and with the ultimate aim of assessing whether sustainable growth can be achieved, the causal relationship between economic growth and energy consumption has been widely debated in empirical studies. The energy-growth nexus has important policy implications. If increased energy consumption causes economic growth, sustainability can only be achieved by ensuring access to a cheap, safe, environmentally-friendly energy supply. Alternatively, if economic growth causes increased energy demand, the challenge is to reduce energy demand through market-oriented policies and regulatory instruments. A review of previous studies on growth-energy consumption using vector autoregressive (hereafter VAR) methodology is presented in Table 1 . Till date, from Table 1 , the results are inconsistent on the direction of causality between energy consumption and economic growth and even about the existence of causality [ 2 – 4 ]. Several factors lie behind these conflicting results. First, the data used in previous studies include different countries and time periods. Secondly, there are also differences in the variable selection; for example, most studies use aggregate energy consumption data, whereas a number of others examine various disaggregated measures. Thirdly, the studies also differ in terms of the econometric methodology.
- PPT PowerPoint slide
- PNG larger image
- TIFF original image
https://doi.org/10.1371/journal.pone.0205671.t001
The majority of the early studies confine the analysis to the bivariate causal relationship between energy consumption and real output. A common problem with the bivariate model specification is the possibility of omitted variable bias [ 17 , 18 ], with the consequent loss of information that may be relevant in determining the direction of causality. Over recent years, several authors have attempted to overcome this problem by including additional variables in the causal analysis of the relationship between energy consumption and growth. However, as pointed out by [ 19 ], these additional variables have been selected on a rather ad hoc basis and the results on causality may be influenced by variable selection bias. Related to this problem of selection bias and variable omission, [ 20 ] point out that the absence of a prior theoretical model may cause the causality test to deliver mixed results. To address the lack of statistical motivation when choosing the control variables for the causal analysis, [ 19 ] apply a robust Bayesian probabilistic model to select the explanatory variables to be considered in the causal analysis of the relationship between energy consumption and economic growth. This approach allows for the evaluation of the posterior probability of including in the model a control variable selected from a large group of possible candidates; to the best of our knowledge, it is the first time in the literature that a robust variable selection method has been applied for this purpose. [ 19 ] use this method to select control variables for the analysis of the relationship between energy consumption and growth in the US from 1949 and 2010, for both aggregated and disaggregated data.
Most empirical studies test for causality in a linear framework (for example using Granger-Sims causality tests and/or unit root and cointegration techniques with either time series or panel data), neglecting the possibility of nonlinear causality. However, given the growing evidence of the presence of possible nonlinearity in several macroeconomic time series which could be caused as a result of several structural breaks, there has been an increasing reliance on nonlinear techniques that could capture causal relations between such variables. Some authors argue that the linear approach to causality testing is limited in its capacity to detect certain kinds of nonlinear causal relationships and so recommend the use of nonlinear techniques [ 21 – 23 ].
While the linear VAR model has advantages in incorporating a large number of variables to be analyzed for Granger causality, there remain limitations, particularly relating to the underlying characteristics of the variables chosen in the model. The variables chosen in the present study are subject to structural breaks as documented by various studies. For example, [ 24 ] conclude structural breaks in oil prices, [ 25 ] find evidence of structural breaks in energy consumption and [ 26 ] conclude the presence of breaks in economic growth. Taking account of the recent studies in the area of energy consumption-economic growth nexus, the VAR modelling approach remains popular. However, as shown by [ 27 ] it is not straightforward to control for breaks in a VAR since a break in one variable will manifest itself in other variables of the VAR model, leading to model misspecification [ 28 ]. Accordingly, we propose to adopt a Flexible Fourier Form VAR (FFF-VAR) framework that allows for smooth breaks that increase the power and size properties of the model.
Therefore, our contribution to the extant literature is to add new findings to the energy consumption-economic growth literature using an alternative modelling approach. We test for causality between energy consumption and economic growth in the US in a multivariate framework, including variables such as those with the highest posterior probability of inclusion according to the results reported by [ 19 ], and at the same time, recognise the presence of the variables included in the VAR model to contain structural breaks and thereby choose an appropriate specification, the FFF-VAR to test for causality between the variables. This approach would be more conducive for the type of variables employed given the possibility of several gradual breaks which can be approximated by smooth breaks couched in the FFF-VAR model.
The remainder of this paper is organized as follows: in the next section, we discuss why it is important to consider nonlinearities when analysing the energy-growth nexus; we explain the econometric methodology in section 3 and present the results of our analysis in section 4. Finally, we outline our conclusions in section 5.
Nonlinearities and the energy-growth nexus
In addition to the selection of relevant variables prior to the study of causality, as stated above, another potential cause of ambiguity in the empirical results on causality is the selection of the functional form of the test. The importance of not neglecting the nonlinearity in energy studies has been widely discussed in the literature. Table 2 summarizes the reasons suggested in the energy literature that motivates the use of a nonlinear framework. Specifically, [ 29 ] conclude that “Due to the influences of economic cycle fluctuations, macroeconomic policies, international oil price fluctuations, technological progress, and industrial adjustment, there may be a nonlinear relationship among economic growth, energy consumption, and CO2 emission” (pp. 1153).
https://doi.org/10.1371/journal.pone.0205671.t002
Despite the importance of nonlinearities in energy economics, the visibility of nonlinear econometric methodologies is surprisingly almost non-existent. For example, in a survey of more than fifty studies in the energy literature by [ 35 ] only one paper due to [ 34 ], consider a nonlinear functional form. Similarly, a survey by [ 36 ] cites a single paper by [ 31 ], that uses a nonlinear methodology in the energy-environment-growth nexus analysis. From the extant literature discussed above, both the empirical and the theoretical studies suggest several reasons to expect nonlinear behavior in the relationship between growth and energy consumption. The main arguments are:
a) Energy prices cause different consumption levels
Historical events suggest that a significant and persistent increase in energy prices over time is usually followed by a downward adjustment of economic growth [ 37 ]. However, this adjustment is not instantaneous; there is a delay between the rise in prices and the fall in the level of production. After a time lag, this economic contraction causes a lower level of consumption, which is likely to be maintained until there is a significant change in energy prices, especially the case of oil.
It is important to note that the nonlinear pattern of energy prices could be reflected in the energy consumption-growth ratio, since energy prices may be the cause of certain contractions and expansions in growth, consequently resulting in different levels of energy consumption. The structural breaks in energy prices renders the linear framework unsuitable for capturing the dynamics of this relationship.
b) Pollution haven hypothesis and porter hypothesis
More stringent environmental regulations increase competitive pressure, especially for those firms operating in the most polluting activities. Companies have a number of ways in which to adapt to regulations: first, they can buy emissions rights in order to continue consuming similar levels of energy; second, they can limit their consumption by producing less; a third alternative (called the Porter Hypothesis) is to invest in clean, efficient technologies that enable them to adapt to regulations while simultaneously boosting their competitiveness; or, fourth, they can move to countries with lax environmental regulations. This last strategy is known as the Pollution Haven Hypothesis (PHH), which states that companies in countries forced to comply with strict environmental regulations may eventually relocate to countries with weaker environmental laws.
According to the PHH, emissions in countries subject to regulatory pressure may decrease as a consequence of tightening environmental regulations. Nevertheless, it is unlikely that companies would all of a sudden "migrate" in response to the new regulatory framework. Instead, one would expect to find a gradual change in the deterministic structure of the relationship. On the other hand, the Porter Hypothesis holds that firms will introduce changes in production in order to comply with strict environmental policies and in an attempt to be more efficient and innovative. These changes will in turn affect energy consumption. Structural changes such as these are the result of progressive investment in cleaner, more efficient technologies. Therefore, models that allow for small but several breaks that can be approximated by smooth changes seem more suitable than linear models when it comes to capturing the effects envisaged by the Porter Hypothesis.
c) Changes in sectoral specialization
Changes in the deterministic structure of the energy consumption-growth relationship can also be explained by the changes in the relative contribution different sectors make to GDP as a country experiences economic growth. There is a shift in the early stages of industrialization whereby sectors such as agriculture become less important than manufacturing; while in more advanced stages of development, manufacturing and other consumer goods sectors are replaced by the lower-consumption services sector. This undoubtedly creates a structural change in energy consumption that linear tests may be unable to capture.
d) The environment as a luxury good
The Environmental Kuznets Curve (EKC) describes the change in a country’s emission levels over time as a result of its economic growth. In the early stages of industrialization, energy consumption rises sharply in countries that do not prioritize environmental degradation control. When countries reach a critical income level, their priorities switch to environmental protection, leading to changes in the energy regime.
To sum up, there are several possible reasons for the existence of nonlinearities in the energy consumption-economic growth relationship. Nevertheless, most studies analyze the energy consumption-economic growth relationship using a linear framework, which for reasons discussed earlier, are restrictive. Given the limitations, the most appropriate models for capturing a possible causality relationship between energy consumption and growth would be those that can approximate the small but several structural breaks that are likely to plague the variables chosen in this study. For the sake of comparison, we estimate both linear and non-linear models.
Methodology
It is not unusual to find economic variables that contain multiple structural breaks. A vast plethora of studies have been put forward that test for structural breaks in individual time series variables. However, when the variables are couched in to a VAR model, this leads to a serious problem. For example, if there are structural breaks in just one variable in the VAR model, that can induce structural shifts in the other variables included in the model [ 28 ]. The problem is exacerbated in the VAR model as the breaks in the single variable affect other variables with a lag. [ 28 ] address this problem by building on the FFF-VAR model allowing for the Flexible Fourier Form to deal with possible multiple smooth shifts in the data.
This deterministic form is particularly useful in capturing the nature of the time series process that contains several small structural breaks with the help of low frequency components. In a way, the choice of the appropriate frequencies to include into the Flexible Fourier form controls for the structural breaks in the data. A test for nonlinearity can be conducted by performing a simple F-test for the exclusion restriction that all values of ϕ ik = ψ ik = 0 in (2). This is possible as [ 39 ] show that the ϕ ik and ψ ik in (2) have multivariate normal distributions. An advantage of the Flexible Fourier form is that it can mimic the nature of the breaks without any knowledge of the magnitude, location and the number of break dates. Besides, the Fourier approximation works for structural breaks which can be of either the innovational outlier or the additive outlier type.
The null hypothesis of a unit root is given by H 0 :( θ = 0) and is tested using a Lagrange Multiplier (LM) test statistic given by τ LM . If the null hypothesis is rejected we can conclude that the data series is stationary. In the case of serially correlated errors, lagged values of Δ y t are added to the regression so that the residuals are white noise. Given the limitation of the number of observations that we have, we choose to set n = k = 1. As emphasised by [ 40 ], a fourier form using k = 1 can serve as a reasonable approximation to breaks of unknown form.
The FFF-VAR model has good size and power properties when testing for smooth structural changes in a VAR(1). In particular, with multiple structural breaks not particularly accounted for, the Granger causality tests tend to have poor size properties. The application of the FFF-VAR model to data that may contain multiple structural breaks, obviates these problems leading towards more reliable results.
Data, results and discussion
In this section, we investigate the dynamic relationship between energy consumption and growth in the US. Making use of a Flexible Fourier form due to [ 28 ], we use causality analysis. As mentioned earlier, [ 19 ] develop a Bayesian model to select the variables with the highest posterior probability of explaining US growth; they consequently choose energy consumption (EC), public spending (SPE) and the oil price (OP) as covariates. Growth has been extracted from the US Bureau of Economic Analysis ( http://www.bea.gov/ ) and is measured in million dollars as the ratio between Value Added (VA) and the the Value Added Price Index. Energy Consumption (EC), is measured in billion BTU, and has been obtained from US Energy Information Administration ( http://www.eia.gov/ ). Oil Price (OP) corresponds to real oil prices in dollars per barrel, and has been obtained from InflationData.com ( http://inflationdata.com/ Inflation/Inflation_Rate/ Historical_Oil_Prices_Table.asp), Public Spending (SPE) is measured as total real spending by the Government in million dollars and has been taken from ( http://www.usgovernmentspending.com/spending_chart_1940_2017USk_13s1li011mcn_F0 .
Clearly, excluding the oil price from the analysis of the causal link between energy consumption and growth may cause misleading results, to the extent that energy consumption and oil prices are connected. The inclusion of public spending allows us to control for other demand-side factors in the US growth.
All the data is plotted in Fig 1 . Alongside the graphs of the variables we have the plot of the Flexible Fourier form that approximates the structural breaks in the variables. The data is measured annually and the sample covers the period from 1949 to 2014.
https://doi.org/10.1371/journal.pone.0205671.g001
We conduct a unit root test on the variables chosen for this study. To this end, the LM based Flexible Fourier unit root test due to [ 40 ] is applied. The results of the test are shown in Table 3 below.
https://doi.org/10.1371/journal.pone.0205671.t003
From the table we find that the null hypothesis of a unit root in all the variables can be rejected at least at the 10% significance level, except for the variable GDP t . The variables EC t energy consumption, OP t oil price and SPE t government spending are all found to be stationary I(0) processes. Since GDP t is found to contain a unit root, the variable is differenced to be made stationary. The variable Δ GDP t is therefore enters the FFF-VAR model in differenced logarithmic form, describing economic growth, and hereafter labelled as GROWTH t
Table 4 presentes the results of the nonlinear causality tests using the FFF-VAR model. The first column lists the possible causality relations, while the second column provides the associated p-values to test the null of Granger non-causality.
https://doi.org/10.1371/journal.pone.0205671.t004
The results show that the null hypothesis of Granger non-causality cannot be rejected for almost all possible pairs except for three cases. We can conclude energy consumption Granger causes economic growth; oil prices Granger cause energy consumption and alternatively, energy consumption Granger causes oil prices. This implies a feedback effect between oil prices and energy consumption. The nonlinear causality test provides evidence supporting the growth hypothesis , meaning that policies aimed at limiting energy consumption will in turn diminish economic growth. It is also interesting to note that in the nonlinear framework bidirectional causality is detected between energy consumption and oil prices. The fact that the price of oil causes energy consumption, underlies the fact that the US has not yet managed to divest from one of the most polluting fossil fuels. Also, what we find is that there are no changes in public spending to address the effects of an increase in energy consumption in the case of the US economy.
To test whether the FFF-VAR is a better fit to the data than the standard linear VAR, we conduct an F-test on the trigonometric terms, or in other words, we conduct a test for linearity. We set up the null hypothesis that the trigonometric terms are equal to zero. The results of the test are given in Table 5 below:
https://doi.org/10.1371/journal.pone.0205671.t005
The results show that we can reject the null hypothesis of the trigonometric variable exclusión test. This implies that the trigonometric terms are significant and they do approximate the small but several breaks that may exist in the data. Based on these results we can therefore conclude that the FFF-VAR provides a better fit to the data.
Given the results of the FFF-VAR we proceed to compare the causality results with those of a linear VAR. To determine how our results would compare if we had ignored the possibility of multiple structural breaks that could be gradual, we conduct a bootstrap versión of the [ 41 ] tests by implementing a procedure due to [ 42 ]. This model, in contrast to the FFF-VAR, is linear and takes in to account the order of integration of the variables. Accordingly, as a prelude to the Granger causality tests in a linear VAR framework, we conduct a comprehensive set of unit root tests to determine the order of integration of the variables. The results are shown in Table 6 below:
https://doi.org/10.1371/journal.pone.0205671.t006
In the first two columns we conduct the standard ADF tests in levels and differences. We cannot reject the unit root null in levels, but we can reject in first differences, thereby concluding that the data is I(1). It is well known that the ADF tests suffer from low power and accordingly we conduct a battery of unit root tests being the GLS detrended ADF tests (ADF-GLS) due to [ 43 ], M-type test due to [ 44 ] and the KPSS unit root test where the null is of stationary against the alternative of non-stationarity based on the procedure of [ 45 ]. The results broadly conclude that the data is I(1). The only exception is that of oil prices ( OP t ) where we cannot reject the null of stationarity using the KPSS test. However, in general, we can conclude that all the variables are I(1). We also carried out panel unit root tests, due to [ 46 ] and [ 47 ] for a common unit root, as well as [ 48 ] and [ 49 ] for individual unit roots. The results are the same. The results are not reported for brevity, but are available from the authors on request.
The results of no-break unit root tests stand in stark contrast to the unit root tests using flexible fourier form to approximate smooth breaks. This is not surprising as we know the unit root tests have low power to reject the null, especially in the case where structural breaks are present in the data (e.g. [ 50 ]). This is also true when there are multiple breaks in the data and the breaks can be gradual [ 40 ]. Our results shown in Table 3 where we can reject the unit root null using the Enders and Lee (ibid) method, underscores that we are using more powerful tests where we reject the unit root null taking into account the unknown nature of breaks. The results emphasise that ignoring the possible presence of multiple and gradual structural breaks in the data can lead to under-rejection of the unit root null hypothesis.
Nonetheless, based on our finding that the variables are I(1) in this particular linear form case, we proceed to carry out the [ 42 ] procedure to tests for Granger causality (We thank an anonymous referee for this comment). This procedure extends the [ 41 ] methodology. Since the [ 41 ] procedure includes non-stationary I(1) variables in the VAR making adjustments to the chosen lag length, the variables appear in the VAR in level form. The results are in Table 7 below:
https://doi.org/10.1371/journal.pone.0205671.t007
The modified Wald (MWALD) tests are given in the second column of Table 2 and alongside in the three adjacent columns we tabulate the boostrapped critical values at the 1%, 5% and 10% significance levels respectively. In all the possible pairwise combinations, we find the MWALD test is lower than the bootstrapped critical values, except for the null hypothesis that oil prices ( OP t ) do not Granger cause energy consumption ( EC t ). This implies that except for oil prices causing energy consumption we cannot find any evidence of causality in all the possible pairwise combinations. The results in general show fewer rejections of the null of Granger non-causality in comparison to the results we find using the FFF-VAR. Caution needs to be exercised though, as the GDP data in this case is in levels, whereas in the FFF-VAR model the GDP data was in growth form.
Conclusions
There is extensive literature that analyzes the causality between growth and energy consumption; however, most such studies use a bivariate approach and thus face the problem of the omission of relevant variables. Such an omission could explain the inconclusive results on the relationship between energy and growth. In addition, the studies that use multivariate models in an attempt to overcome this limitation have selected the additional variables on an ad hoc basis, thus introducing bias into the results. What is more, most studies have analyzed the existence of causality between energy and growth in a linear context, despite a broad body of literature that highlights the importance of taking into account the nonlinear dynamics in the variables associated with studies on energy consumption and economic growth.
In this paper, we overcome the limitations in the literature and analyze the relationship between energy consumption and growth in a multivariate context, choosing variables according to the probability of inclusion reported in [ 19 ]. The variables used in the model are growth, energy consumption, public spending and oil prices for the US. Besides, the existence of causality between these variables is analysed using a FFF- VAR, which approximates possible structural breaks in the variables, thereby overcoming the limitations in linear studies.
The linear causality test results indicate that there is no causality between GDP and energy consumption, and therefore a prescriptive policy measure would be to reduce energy consumption in the US without affecting the country’s economic growth. This is referred to in the literature as the ‘neutrality hypothesis’. In contrast, the nonlinear model supports the so-called ‘growth hypothesis’, since causation is found running from energy consumption to growth. This implies that a reduction in energy consumption would in fact adversely affect growth. In light of the above, it can be argued that since linear tests are unable to identify certain causal relationships, they can lead to erroneous conclusions which can have consequences for economic policy. The nonlinear methodology allows us to capture causal relationships between the other variables of the system that the linear approach fails to detect. Thus, based on the results of the linear model we would be inclined to draw the conclusion that the price of oil causes energy consumption; however, the nonlinear procedure indicates more to this relationship that there is mutual causality between the two variables. To sum up, we find the FFF-VAR results depart from those of the linear VAR. Given the evidence of nonlinearity in the data, we would be inclined to rely on the results obtained from the FFF-VAR since the Fourier form has better size and power properties; in which case there is support for the growth hypothesis, rather than the neutrality hypothesis leading to a different set of policy prescriptions.
- View Article
- Google Scholar
- 21. Péguin-Feissolle, A., & Terasvirta, T. (1999) A general framework for testing the Granger noncausality hypothesis, Working Paper Series in Economics and Finance, Stockholm School of Economics, 343.
- 46. Breitung J. The local power of some unit root tests for panel data. Berlin: Humboldt-Universität; 1999.
- DOI: 10.1111/1467-8454.00015
- Corpus ID: 56073565
Causality between Exports and Economic Growth: The Empirical Evidence from Shanghai
- Jordan Shan , G. Tian
- Published 1 June 1998
Figures and Tables from this paper
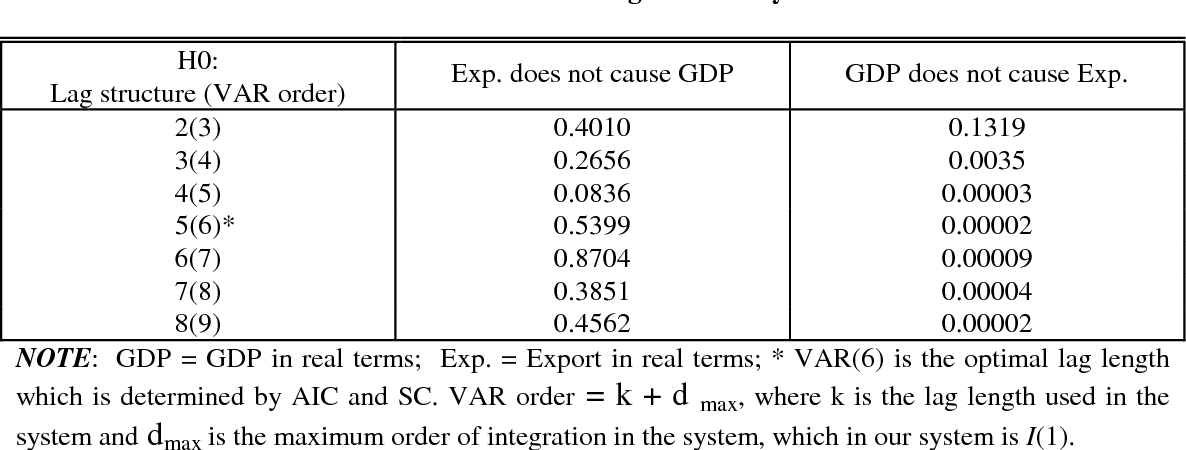
89 Citations
Time-series evidence for balassa’s export-led growth hypothesis, export and economic growth in namibia: a granger causality analysis, exports and economic growth: the causality test for asean countries, an empirical analysis of the asean-4’s causality between exports and output growth, export-led growth in south africa: evidence from the components of exports, causal nexus between economic growth, export and external debt servicing the case of pakistan, the aggregate exports-gdp relation under the prism of infrequent trend breaks and multi-horizon causality, market size and export-led growth hypotheses: new evidence from malaysia, does the export-led growth hypothesis hold for myanmar, exports-growth nexus in pakistan cointegration and causality analysis, 35 references, on the export-led growth hypothesis: the econometric evidence from china, export growth and economic growth: an application of cointegration and error-correction modeling, on the causality between export growth and gdp growth: an empirical reinvestigation, export-led growth or growth-driven exports the canadian case, economic growth and the expanding export sector: china 1952–1985, exports and economic growth: evidence from cross–country analysis, the engine of growth or its handmaiden, export-led growth and the four little dragons, export–led growth hypothesis: some empirical testing, on export-led growth in australia and canada: cointegration, causality and structural stability*, related papers.
Showing 1 through 3 of 0 Related Papers
To read this content please select one of the options below:
Please note you do not have access to teaching notes, the causality relationship between foreign direct investment and economic growth in rcep countries.
Journal of Economic and Administrative Sciences
ISSN : 2054-6238
Article publication date: 11 November 2022
Issue publication date: 29 February 2024
The direction of the causality relationship between Foreign Direct Investment (FDI) and economic growth is a highly controversial issue in the literature. There are two basic approaches advocating different causal directions between FDI and growth, which are called hypotheses of FDI-led Growth and Growth-led FDI. The aim of this study is to analyze the causality relationship between FDI and economic growth in RCEP countries and thus make a new contribution to the discussions in the relevant literature. In addition, the results of the study are expected to provide important implications for the policies to be designed for economic growth based on FDI flows to RCEP countries. Thus, by examining the direction of causality between FDI and economic growth in RCEP countries, we aim to provide a new contribution to related literature and make some implications for the policy design process of economic growth in the RCEP area.
Design/methodology/approach
We empirically examined the direction of a causal link between FDI and economic growth in the context of Regional Comprehensive Economic Partnership (RPEC) countries in order to test the hypothesis of FDI-led growth and Growth-led FDI. Accordingly, as our main variables of interest, we incorporated the inward foreign direct investment stock to gross domestic product ratio (FDI) and gross domestic product per capita (GDP). Hatemi-J (2012) asymmetric causality test has been employed in the investigation of the direction of causality between FDI and GDP over the period of 1980–2020. Thus, unlike most of the studies investigating the direction of causality between FDI and growth using the linear causality analysis method, our study performed a nonlinear causality analysis.
Empirical results reveal that the causal relationship between FDI and national income in RPEC countries is non-linear or asymmetric . The results of the symmetric causality test for both from FDI to national income and from national income to FDI are statistically insignificant for all countries. Therefore, this finding obtained from the study provided an important guide to the econometric methods to be used in other studies to be conducted in the same region in the future. Concerning the asymmetric causality relationship from FDI to growth, positive FDI shocks are an important cause of national income in most RCEP countries. However, the effect of negative FDI shocks on national income is quite weak compared to positive shocks. Regarding the asymmetric causality relationship from growth to FDI, positive national income shocks do not create a significant causal relationship with FDI. Similarly, the effects of negative national income shocks on FDI are statistically insignificant. Overall, asymmetric causality test results reveal that positive FDI shocks have an important causal impact on economic growth in most RCEP countries. Thus, the results of econometric analysis mostly support the argument that the FDI-led growth hypothesis rather than the Growth-led FDI hypothesis in RCEP countries. Accordingly, policy-makers in most of the RCEP countries should continue to provide more incentives and facilities to multinational companies in order to ensure constant economic growth.
Originality/value
Our study brings a significant difference in the econometric method used compared to most of the other studies in the literature. Existing empirical studies on the direction of causality between FDI and growth mostly use standard Granger-linear causality-type tests to detect the direction of causality among FDI and growth. Unlike most of the studies in the literature, our study adopted a different methodological approach, namely the Hatemi J test to detect the non-linear causality between FDI and economic growth in RCEP countries. Therefore, this paper made a new methodological contribution significantly to the literature focusing on the causal relationship between FDI and economic growth by using a non-linear causality method rather than a linear causality one.
- Foreign direct investment
- Economic growth
- Hatemi-J non-linear causality test
- RPEC countries
Karahan, Ö. and Çolak, O. (2024), "The causality relationship between foreign direct investment and economic growth in RCEP countries", Journal of Economic and Administrative Sciences , Vol. 40 No. 1, pp. 95-110. https://doi.org/10.1108/JEAS-04-2022-0112
Emerald Publishing Limited
Copyright © 2022, Emerald Publishing Limited
Related articles
All feedback is valuable.
Please share your general feedback
Report an issue or find answers to frequently asked questions
Contact Customer Support
The political economy of development: A cross-national causality test of development-democracy-growth hypothesis
- Published: August 1988
- Volume 58 , pages 123–141, ( 1988 )
Cite this article
- Abbas Pourgerami 1
742 Accesses
57 Citations
Explore all metrics
Concluding remarks
The empirical investigation of the development-democracy-growth hypothesis estimates positive correlations and causal associations between development and democracy and democracy and growth. Among the preconditions for the emergence of DEMOCRACY, MARKET and CULTURE show strong and highly significant positive effects which are largely transmitted directly. DEVELOPMENT affects DEMOCRACY directly and indirectly via EDUCATION and INVESTMENT. The positive causal association between DEMOCRACY and GROWTH is transmitted both directly and indirectly via LABOR and WELFARE. Accordingly, the hypothesized compatibility between economic development and political democracy is confirmed, while rejecting the trade-off between political democracy and economic growth.
The developmental implications of these findings emphasize a need for continuous efforts on the part of governments and people to initiate and/or accelerate a process of establishing preconditions for the emergence of democracy via improvements in the allocative role of markets, enrichment of cultural values in such a way that more diversity and compromise are tolerated in the decision making processes, and betterment of the standard of living by investment in human and physical capital. The establishment of a genuine democracy and expansion of individual freedom will enable the people to actively participate in the development process in order to continuously improve the quality of life for the entire population.
This is a preview of subscription content, log in via an institution to check access.
Access this article
Subscribe and save.
- Get 10 units per month
- Download Article/Chapter or Ebook
- 1 Unit = 1 Article or 1 Chapter
- Cancel anytime
Price includes VAT (Russian Federation)
Instant access to the full article PDF.
Rent this article via DeepDyve
Institutional subscriptions
Similar content being viewed by others
Perplexing Coexistence of Democracy, Transparency and Development in the Contemporary Era
Democracy as a Driver of Post-Communist Economic Development
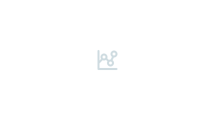

Democracy and Economic Growth: a Literature Review
Adelman, I., and Morris, C.T. (1967). Society, politics, and economic development . Baltimore: Johns Hopkins University Press.
Google Scholar
Alwin, D.F., and Hauser, R.M. (1975). The decomposition of effects in path analysis. American Sociological Review 40 (February): 37–47.
Amnesty International report (1984–1986). London: Amnesty International Publications.
Andreski, S. (1969). Parasitism and subversion: The case of Latin America . New York: Schocken.
Bauer, P.T. (1981). Equality, the third world and economic delusion . London: Weidenfeld and Nicholson.
Berg-Schlosser, D. (1985). African political systems: Typology and performance. Comparative Political Studies 17: 1984/85.
Bhagwati, J. (1966). The economics of underdeveloped countries . New York: McGraw Hill.
Bloch, P.C. (1986). The politico-economic behavior of authoritarian governments. Public Choice 51: 117–128.
Bollen, K.A. (1980). Issues in the comparative measurement of political democracy. American Sociological Review 45 (June): 370–390.
Bruton, H.J. (1977). Industrialization policy and income distribution. In C.R. Frank and R.C. Webb (Eds.), Income distribution and growth in less developed countries , 79–125. Washington DC: Brookings.
Coleman, J.S. (1960). Conclusion. In G.A. Almond and J.S. Coleman (Eds.), The politics of the developing areas , 538. Princeton: Princeton University Press.
Cutright, P. (1963). National political development: Measurement and analysis. American Sociological Review 28: 253–264.
Dick, G.W. (1974). Authoritarian versus nonauthoritarian approaches to economic development. Journal of Political Economy 82: 817–827.
Emerson, R. (1971). The prospects of democracy in Africa. In M.F. Lofchie (Ed.), The state of the nations . Berkeley: University of California Press.
Finer, S.E. (1962). The man on horseback: The role of the military in politics . Harmondsworth, England: Penguin Books.
Finer, S.E. (1971). Comparative government . New York: Basic Book.
Flanigan, W., and Fogelman, E. (1971). The introduction of democracy in underdeveloped countries. In M. Palmer and L. Stern (Eds.), Political development in changing societies . Lexington, MA: Health Lexington Books.
Frank, C.R., and Webb, R.C., Eds. (1977). Income distribution and growth in less developed countries . Washington, DC: Brookings.
Gastil, B.D. (1980). Freedom in the world . New York: Freedom House.
Heilbroner, G. (1963). The great ascent . New York: Harper & Row.
Huntington, S.P. (1984). Will more countries become democratic? Political Science Quarterly 99: 193–218.
Huntington, S.P., and Dominguez, J.I. (1975). Political development. In F.I. Greenstein and N.W. Polsby (Eds.), Handbook of political science: Micropolitical theory , 1–114. Reading, MA: Addison-Wesley.
Huntington, S.P., and Nelson, J.M. (1976). No easy choice: Political participation in developing countries . Cambridge, MA: Harvard University Press.
Kahn, H. (1979). World economic development . London: Croom and Helm.
Laband, D.L. (1984). Is there a relationship between economic conditions and political structure? Public Choice 42 (1): 25–37.
Lewis, W.A. (1970). The theory of economic growth . New York: Harper & Row.
Lipset, S.M. (1959). Some social requisites of democracy: Economic development and political legitimacy. American Political Science Review 53: 69–105.
Lipset, S.M. (1981). Political man: The social bases of politics , 2nd ed. Baltimore: Johns Hopkins University Press.
Lipton, M. (1977). Why poor people stay poor . London: Temple Smith.
Loewenthal, R. (1963). Staatsfunktionen und Staatsform in den Entwicklungsländern. In R. Loewenthal (Ed.), Die Demokratie im Wandel der Gesellschaft . Berlin: Colloquium Verlag.
Marsh, R.M. (1979). Does democracy hinder economic development in the late-comer developing nations? Comparative Social Research 2: 215–248.
Newbold, P. (1984). Statistics for business and economics . Englewood Cliffs, NJ: Prentice-Hall.
O'Donnell, G.A. (1973). Modernization and bureaucratic-authoritarianism . Berkeley: University of California Press.
Pourgerami, A. (1985). Export growth and economic development: A comparative logit analysis. Journal of Economic Development 10 (1): 117–127.
Rustow, D.A. (1970). Transitions to democracy: Toward a dynamic model. Comparative Politics 2: 337–367.
Tullock, G. (1974). The social dilemma: The economics of war and revolution . Blacksburg, VA: University Publications.
Verba, S. (1965). Comparative political culture. In W.P. Lucian and S. Verba (Eds.), Political culture and political development . Princeton: Princeton University Press.
Weber, M. (1906). Zur Lage der bürgerlichen Demokratie in Russland. Archiv für Sozialwissenschaft und Sozialpolitik 22: 346ff.
Weede, E. (1983). The impact of democracy on economic growth: Some evidence from cross-national analysis. Kyklos 36: 21–39.
Download references
Author information
Authors and affiliations.
Economics Institute, University of Colorado, Boulder, 1030 13th Street, 80302, Boulder, CO
Abbas Pourgerami
You can also search for this author in PubMed Google Scholar
Rights and permissions
Reprints and permissions
About this article
Pourgerami, A. The political economy of development: A cross-national causality test of development-democracy-growth hypothesis. Public Choice 58 , 123–141 (1988). https://doi.org/10.1007/BF00125718
Download citation
Issue Date : August 1988
DOI : https://doi.org/10.1007/BF00125718
Share this article
Anyone you share the following link with will be able to read this content:
Sorry, a shareable link is not currently available for this article.
Provided by the Springer Nature SharedIt content-sharing initiative
- Economic Growth
- Economic Development
- Development Process
- Political Economy
- Public Finance
- Find a journal
- Publish with us
- Track your research
Watch CBS News
Trump says he'll end the "inflation nightmare." Economists say Trumponomics could drive up prices.
By Aimee Picchi
Edited By Alain Sherter
July 19, 2024 / 5:00 AM EDT / CBS News
Former President Donald Trump is campaigning on a pledge to end the "inflation nightmare," vowing that if he wins a second term, he'll bring down prices "very quickly." And if that scenario came to pass, it would be cheered by the millions of Americans who say higher costs remain a major problem.
There's only one problem: Key policies that undergird so-called Trumponomics — a combination of tariffs, tax cuts and a crackdown on immigration — are likely to cause a flare-up in inflation, according to many Wall Street economists. That would be a painful outcome for consumers and businesses sapped by more than two years of surging prices. More broadly, renewed inflationary pressures would also come as inflation is finally inching closer to the Federal Reserve's goal of 2% per year.
But experts warn that Trump's economic policies could cause such progress to stall, and even reverse. They note that tariffs effectively act as a consumption tax , increasing the cost of goods imported into the U.S. — costs that businesses typically pass on to consumers.
Meanwhile, the sharp corporate tax cuts Trump has floated would act as an inflationary fiscal stimulus. And another central plank — deporting immigrants — could force employers to pay higher wages to attract a shrinking pool of workers, also adding to pricing pressures, economists told CBS MoneyWatch.
In such a case, the Fed could be forced to keep its benchmark rate higher for longer, causing more pain for consumers and businesses seeking mortgages, auto loans or other credit products.
If consumers "are upset now, they will be hopping mad a year from now" about inflation if Trump wins and enacts his policies, Mark Zandi, chief economist of Moody's Analytics and co-author of a June report on the macroeconomic impacts of either a Trump or Biden win in November, told CBS MoneyWatch.
Zandi, who has previously advised presidential candidates from both parties, said he's not advising either campaign in the current race.
Where will inflation go in 2025?
Under a Republican election sweep that helps Trump implement his economic agenda, the annual inflation rate would increase to 3.6% in 2025, Moody's forecasted in its June analysis. By comparison, the most recent inflation data showed that prices rose 3% in June .
If Biden wins and can continue with his platform, inflation could dip to 2.4% next year, Moody's projected.
- CBS News price tracker shows how much food, utility and housing costs are rising
To be sure, not everyone agrees with such projections. EJ Antoni, a research fellow and public finance economist at the conservative-leaning Heritage Foundation, told CBS MoneyWatch that some economists are making their conclusions "without looking at the broader picture."
"As far as what [Trump] has said in public, it is to reduce federal income taxes and corporate taxes, and essentially replace that with tariffs," Antoni said. "I understand the argument that tariffs are a tax on trade, but so is the income tax. If all these things are taxes on trade, do you want a tax on domestic trade or international trade?"
Under this view, additional tax cuts will offset inflation caused by stiffer levies on foreign goods. Trump has floated a 10% across-the-board tariff on all U.S. imports, as well as a 60% tax on products from China. As the 2024 Republican platform puts it, "As tariffs on foreign producers go up, taxes on American workers, families and businesses can come down."
Although Trump is advocating for a reduction in the corporate tax rate, telling Bloomberg News that he wants to slice it to 15% from the existing 21%, his plans for individual income taxes remain murky. Trump has generally pledged to lower taxes, although many experts believe he would want to extend his 2017 Tax Cuts and Jobs Act, given that many of its tax cuts expire at the end of 2025.
During his presidency, Trump relied on his executive powers to enact tariffs, meaning that he didn't have to wait for Congress to pass legislation. Tax cuts, however, would need to be passed by both the House and Senate, a bigger hurdle for Trump to pursue that side of his platform.
Who pays tariffs?
The typical middle-class household in the U.S. would face an estimated $1,700 a year in additional costs if Trump enacts these proposed tariffs, according to the non-partisan Peterson Institute for International Economics.
But economists note that low- and middle-income households would feel the impact much more than high-income families. That's because lower income earners spend a large share of their money on goods and services, so tariffs have a disproportionate impact on them compared with wealthy families.
"The burden of it is very unequal," Julia Coronado, founder of MacroPolicy Perspectives and a former Fed economist, told CBS MoneyWatch.
Antoni of the Heritage Foundation pointed out that after Trump levied tariffs on some imports during his presidency, inflation remained low. Indeed, inflation sat at an average rate of about 2% from 2017 to 2019 . Yet economists say the scale of his latest plan dwarfs his earlier tariffs.
"What he's proposing on tariffs is way beyond anything he did in his first administration," Coronado noted. "He's proposing blanket tariffs on all imports, and especially severe tariffs on China — that passes onto consumers immediately."
Immigration: Costs and benefits
A surge in immigration has expanded the U.S. labor force at a time when it's facing demographic challenges, such as the aging baby boomer population. That's helped buoy the labor market, which in turn has helped temper wage increases.
But Republicans want to deport millions of immigrants, an action that could disrupt the economy and labor market, Coronado said. "Deporting people is going to be a hit to both demand and supply — you would deport people who are working and spending money in the economy, so growth will slow," she said.
Antoni notes that immigrants drive up costs for both the federal government and at the local level, which would be eliminated if they were deported.
Of course, Trump has yet to detail many of his economic plans, such as his policies for individual income taxes. For now, what is known about his platform suggests it could create conditions that boosts inflation, rather than slow it as he has promised, economists said.
"Trump wants a combination of things that economists know don't add up," Coronado told CBS MoneyWatch. "He's promising, 'I can have my cake and eat it too'."
- Biden Administration
- Donald Trump
Aimee Picchi is the associate managing editor for CBS MoneyWatch, where she covers business and personal finance. She previously worked at Bloomberg News and has written for national news outlets including USA Today and Consumer Reports.
More from CBS News
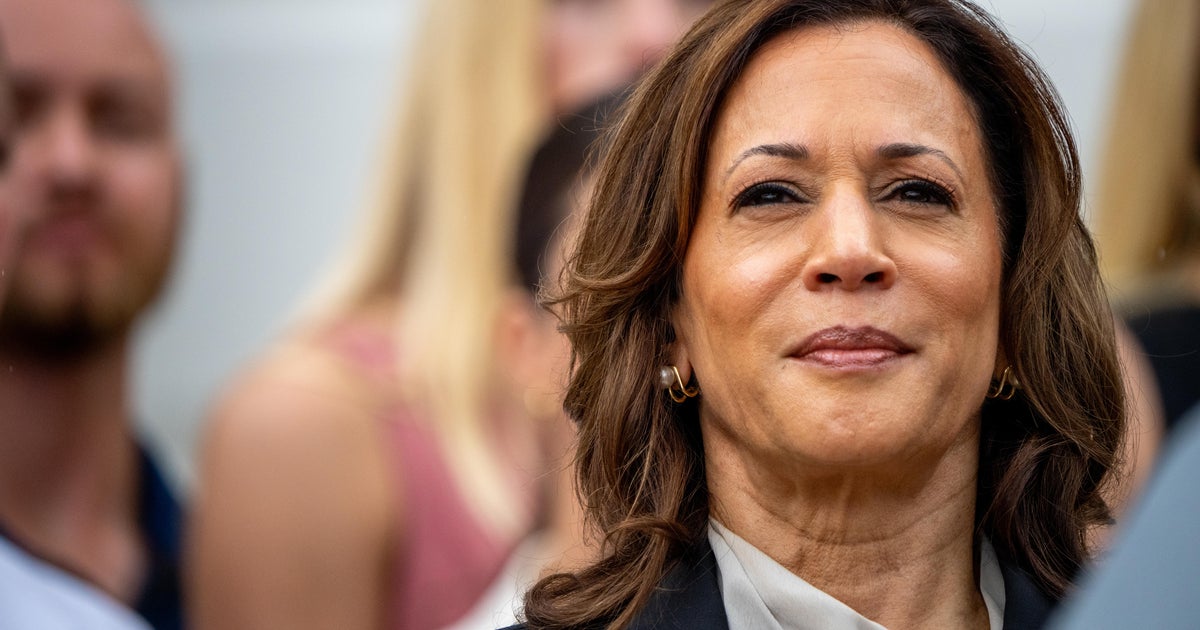
Poll analysis: Harris slightly ahead of Biden in hypothetical Trump matchup
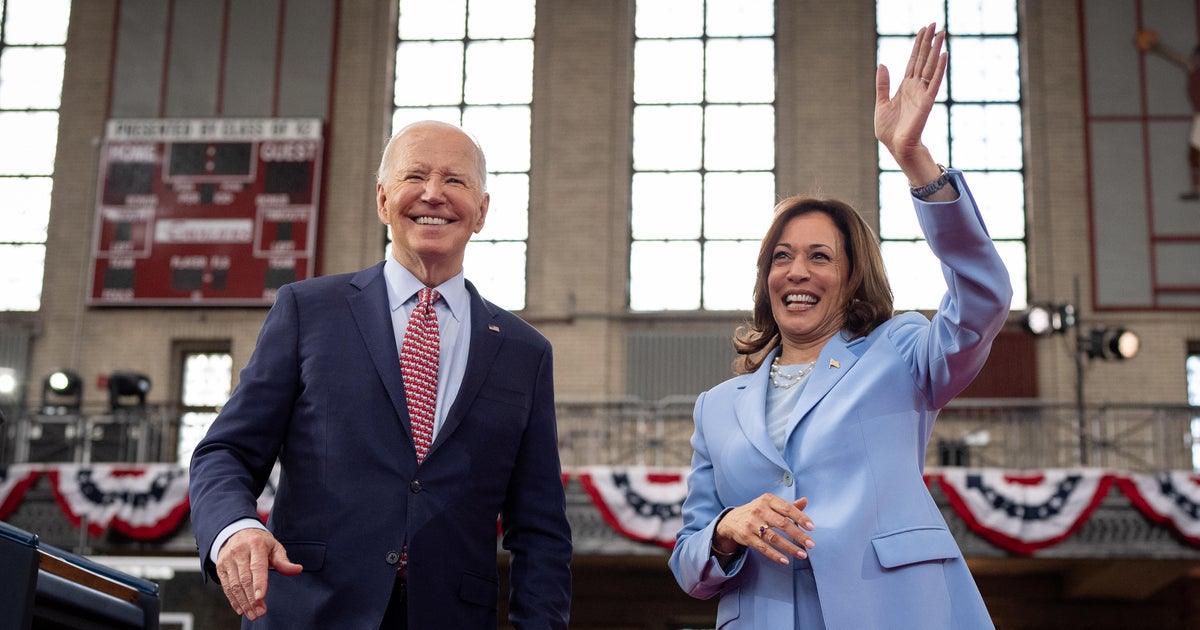
Some Republicans threaten lawsuits to keep Biden on ballot. Will they work?
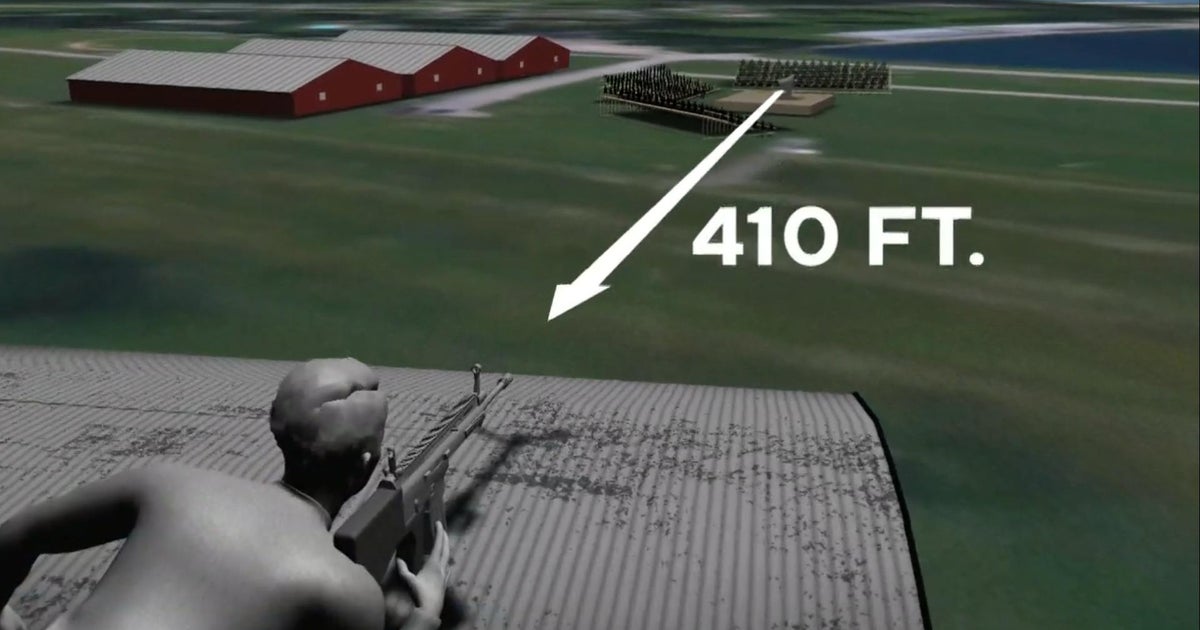
Trump rally gunman fired 8 shots in under 6 seconds, analysis shows
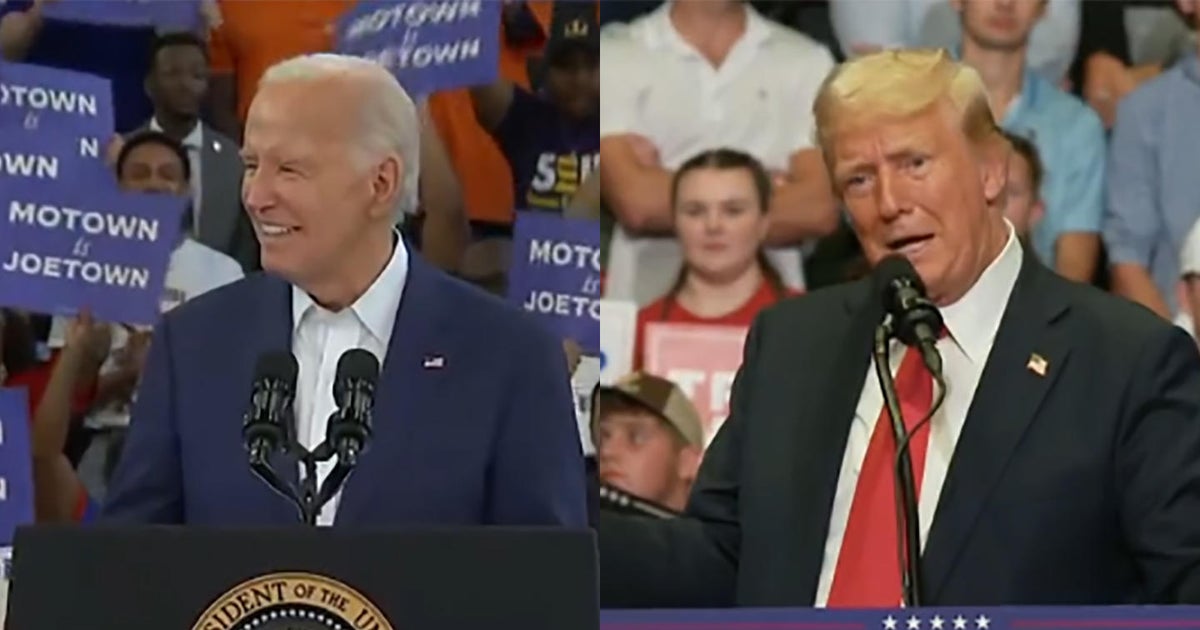
An unconventional week in presidential politics
Trump's promise of tariffs and deportations could pop the stock market bubble if he wins a 2nd term, Capital Economics says
- An AI-driven stock market bubble could burst early if Trump wins the 2024 election, according to Capital Economics.
- Trump's proposed policies on tariffs and immigration could slow economic growth and spark higher inflation, the firm said.
- Higher inflation would restrict the Federal Reserve's ability to cut interest rates.

The ongoing stock market bubble that has been inflated by a strong rally in AI stocks could pop prematurely if former President Donald Trump wins the 2024 election.
That's according to Capital Economics chief markets economist John Higgins, who said in a note on Thursday that Trump's proposed policies regarding tariffs and immigration would spell disaster for the economy.
A second Trump Presidency "could potentially result in the imposition of universal tariffs and reductions in immigration," Higgins said. "Universal tariffs and less immigration would cause growth to be slower and inflation to be higher than otherwise."
Such a scenario would be a nightmare for the Federal Reserve, as higher inflation via potential tariffs would limit their ability to cut interest rates, even if economic growth slows.
"Despite the threats to growth from such trade and immigration policies, the Fed might be less inclined, if at all, to loosen policy in such circumstances. This is because the policies would jeopardise its victory against inflation. That, in turn, could undermine equity valuations, because higher expected interest rates would drive up Treasury yields all else equal," Higgins said.
Capital Economics has held the view that the stock market will continue to hit records to as high as 7,000 by the end of 2025 before the bubble pops, similar to what happened in the 2000 dot-com bubble.
"It's worth recalling that the dotcom bubble burst in the wake of tighter Fed policy and higher Treasury yields," Higgins said.
What's more, if Trump wins a second term as President and Republicans gain control of Congress, the potential for a surge in fiscal spending would also put a cap on the Fed's decision to cut interest rates.
"The public finances are in a more parlous state than in 2016. So, conservative lawmakers in the party would presumably oppose a wider budget deficit, for fear of falling foul of the bond vigilantes. And even if they didn't, a big fiscal expansion would give the Fed even more cause to reassess the appropriate stance of monetary policy given the threat that it would pose to price stability," Higgins said.
And in the event that Trump doesn't win the 2024 election, Higgins said the stock market bubble will still be at risk of a potential slowdown in corporate earnings, lagging Fed policy finally hitting economic growth, or something "out of left field to rock the boat, perhaps geopolitical in nature."
Despite the risk of Trump prematurely popping the stock market bubble if he wins the 2024 election, Higgins is standing by his call for now that the S&P 500 will soar to 7,000 through 2025 before it ultimately crashes back down.
- Main content
La. DOTD under the crosshairs of state legislature
“untrustworthy, insufficient and a contributing cause to the state’s slowed economic growth”.
BATON ROUGE, La. (KALB) - On July 22, the Louisiana Legislature released an official announcement declaring the contracting of the Boston Consulting Group (BCG) to optimize Louisiana’s Department of Transportation and Development (DOTD) .
Under the contract, BCG is tasked with performing an extensive assessment of La. DOTD’s operations and general effectiveness. The assessment’s tasked goal is to provide a concise set of data for state officials to consider during the DOTD’s phase of reorganization/optimization, something Governor Jeff Landry has openly advocated for.
“The collaboration between private industry, both chambers of the legislature and my office to address this issue is a positive sign for Louisiana, and a harbinger of success.” Governor Jeff Landry, (R) Louisiana
The state’s legislative leaders, Senate President Cameron Henry and Speaker of the House Phillip DeVillier openly voiced their agreement with Governor Landry’s calls in the state’s contracting announcement, stating they are dedicated to reforming the department.
“Our members are dedicated to getting this right, and we would be remiss to not fully engage in this process to reform and restructure DOTD.” Speaker of the House Phillip DeVillier, (R) District 41
“As the legislative bodies responsible for overseeing and ensuring funding for DOTD, we believe it is crucial to be deeply involved in this process. Legislators are closest to the people, and it is our duty to engage now to ensure DOTD’s future success.” J. Cameron Henry, Jr., (R) District 9
On May 1 , Gov. Landry signed an executive order declaring the reformation of the state DOTD. Throughout his executive order, Landry refers to the current iteration of the department as untrustworthy, insufficient and a contributing cause to the state’s slowed economic growth.
An audit report released on May 8 by the Louisiana Legislative Auditor (LLA) determined the department was not adhering to federal regulations on the quality assurance of construction projects and failed to comply with certain wage rate requirements, potentially resulting in “contractors not paying laborers and mechanics the [existing] wage rates.”
The assessment phase being conducted by the Boston Consulting Group is set to begin in the fall of 2024.
The secretary of the La. DOTD is required under Landry’s executive order to submit a reorganization plan for approval by the state legislature by January 15, 2025.
Click here to report a typo. Please provide the title of the article in your email.
Copyright 2024 KALB. All rights reserved.

Traffic Alert: Fire on I-49 in Natchitoches Parish

Turkey Creek man arrested for growing marijuana, firearms charges

Natchez sex offender sentenced in child porn case from 2023
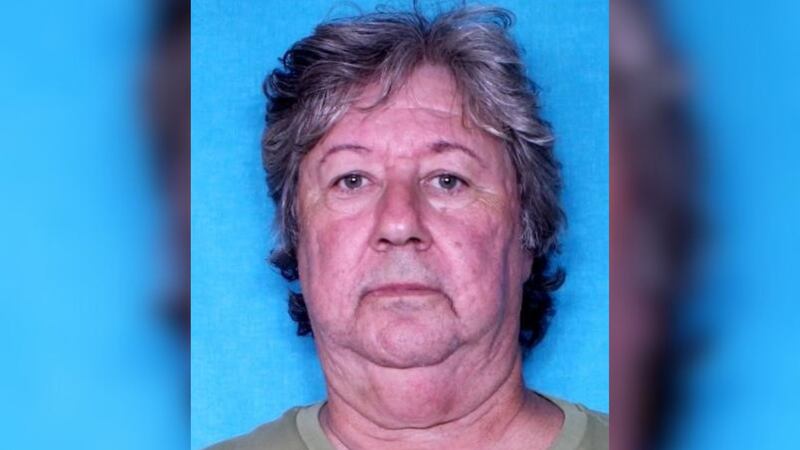
APSO seeking public’s help in death investigation

Taking a look at La. Congressional District 6 before the November Election
Buckeye native and retired major general inducted to La. Guard Hall of Fame
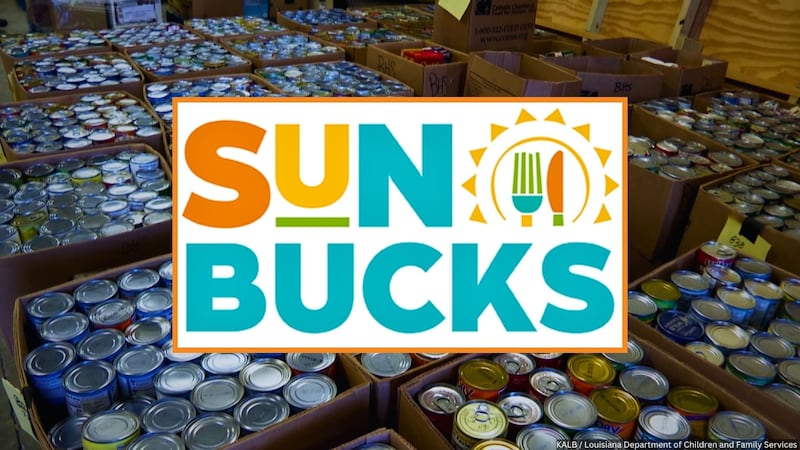
Phase 2 SUN Bucks benefits start going out this week
Latest news.

City of Alexandria approves over $446K to replace lights on MacArthur Drive

Louisiana to ban cell phone usage in school; some teachers question enforcement

James Genovese introduced as NSU’s new president

CLTCC entering 9th year of having a tuition freeze

Laborde Earles Injury Lawyers to host 3rd annual Project Backpack giveaway event
- Do Not Sell My Personal Info
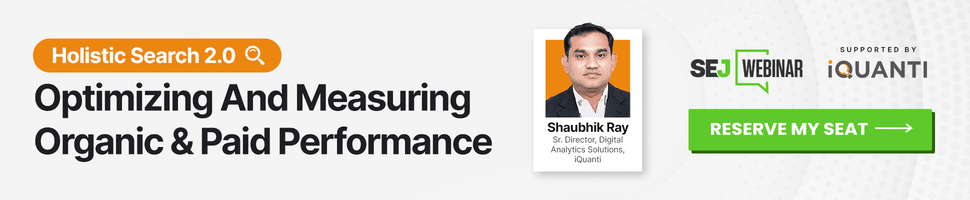
- ⋅
Google Cautions: Exponential Content Growth Causes Re-Evaluation
Google's John Mueller says that an exponential growth in content will cause it to be seen as a new site and be re-evaluated
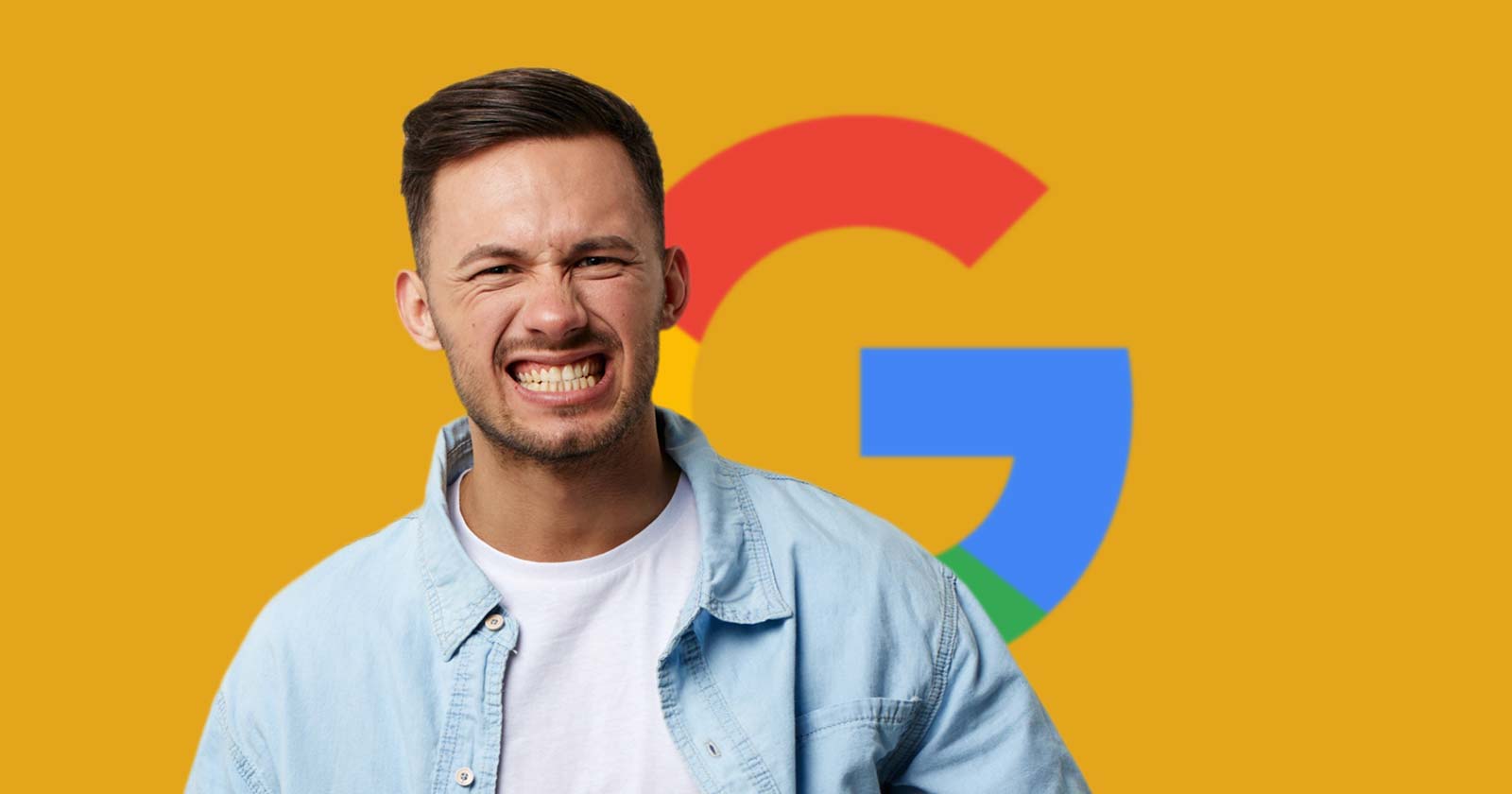
Google’s John Mueller answered a question about the impact of increasing a website’s size by ten times its original size. Mueller’s answer should give pause to anyone considering making their site dramatically larger, as it will cause Google to see it as a brand new website and trigger a re-evaluation.
Impact Of Making A Site Bigger
One of the reasons for a site migration is joining two websites into one website, which can cause a site to become even larger. Another reason for an increase in size is the addition of a massive amount of new products.
This is the question that was asked in the SEO Office Hours podcast:
“What’s the impact of a huge expansion of our product portfolio on SEO Performance, for example going from 10,000 to products to 100,000?”
It must be pointed out that the question is about a site growing ten times larger.
This is is Mueller’s answer:
“I don’t think you have to look for exotic explanations. If you grow a website significantly, in this case, by a factor of 10, then your website will overall be very different. By definition, the old website would only be 10% of the new website. This means it’s only logical to expect search engines to re-evaluate how they show your website. It’s basically a new website after all. It’s good to be strategic about changes like this, I wouldn’t look at it as being primarily an SEO problem.”
Re-Evaluate How Google Shows A Website
Mueller said it’s not primarily an SEO problem but it’s possible most SEOs would disagree because anything that affects how a search engine shows a site is an SEO problem. Maybe Mueller meant that it should be seen as a strategic problem?
Regardless, John Mueller’s answer means that growing a site exponentially in a short amount of time could cause Google to re-evaluate a site because it’s essentially an an entirely new website, which might be an undesirable scenario.
Although Mueller didn’t specify how long a re-evaluation can take, he has indicated in the past that it can take months. Maybe things have changed but this is what he said four years ago about how long a sitewide evaluation takes :
“It takes a lot of time for us to understand how a website fits in with regards to the rest of the Internet. …And that’s something that can easily take, I don’t know, a couple of months, a half a year, sometimes even longer than a half a year, for us to recognize significant changes in the site’s overall quality.”
The implication of a sitewide evaluation triggered by an exponential growth in content is that the optimized way to approach content growth is to do it in phases. It’s something to consider.
Listen to the Google SEO Office Hours podcast at the 4:24 minute mark:
Featured Image by Shutterstock/ShotPrime Studio
I have 25 years hands-on experience in SEO and have kept on top of the evolution of search every step ...
Subscribe To Our Newsletter.
Conquer your day with daily search marketing news.
Luxury brands feel the sting as Chinese growth slows
- Medium Text
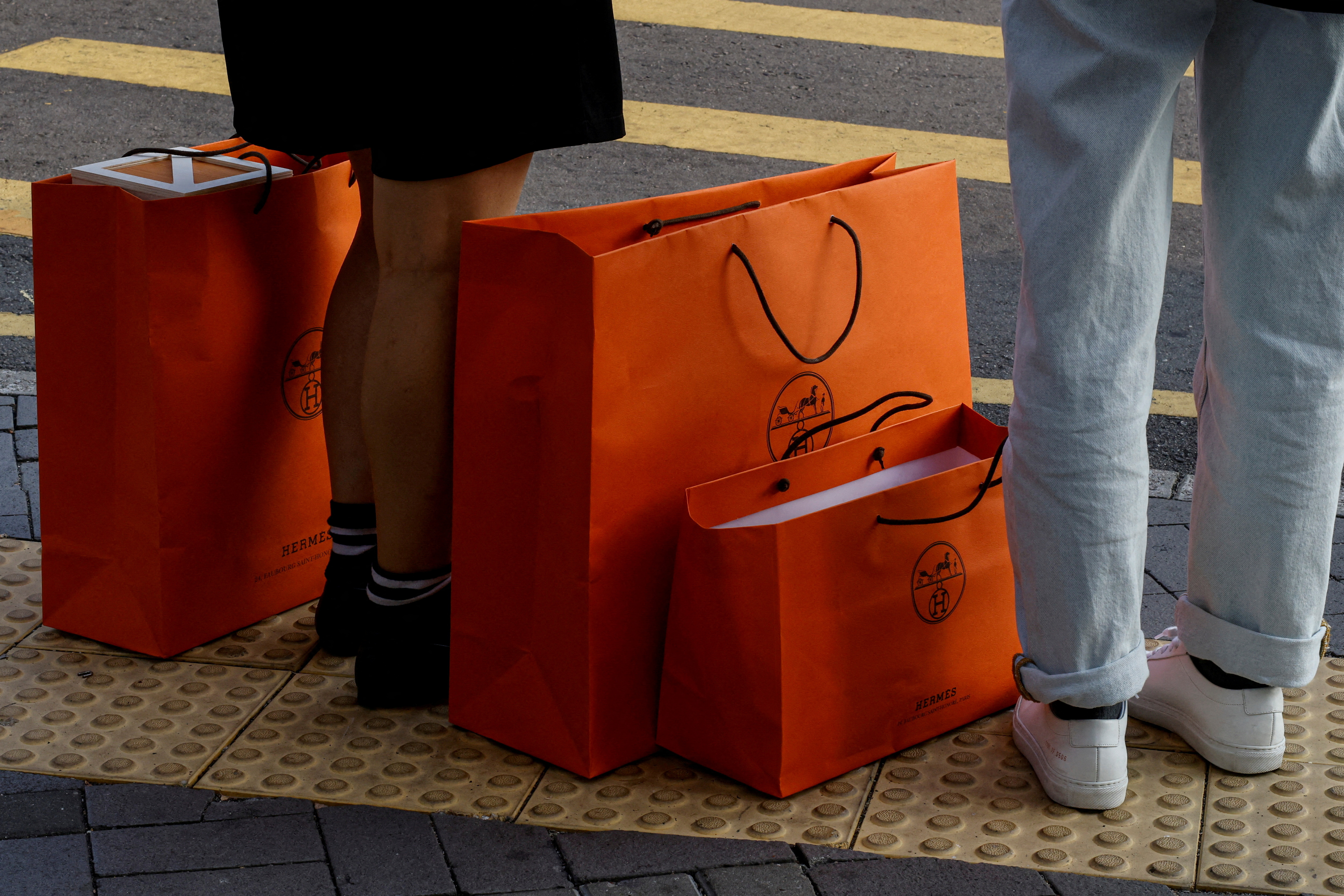
PROLONGED DOWNTURN
Sign up here.
Reporting by Mimosa Spencer; Additional reporting by Danilo Masoni in Milan and Samuel Indyk in London; Editing by Josephine Mason and Jan Harvey
Our Standards: The Thomson Reuters Trust Principles. , opens new tab
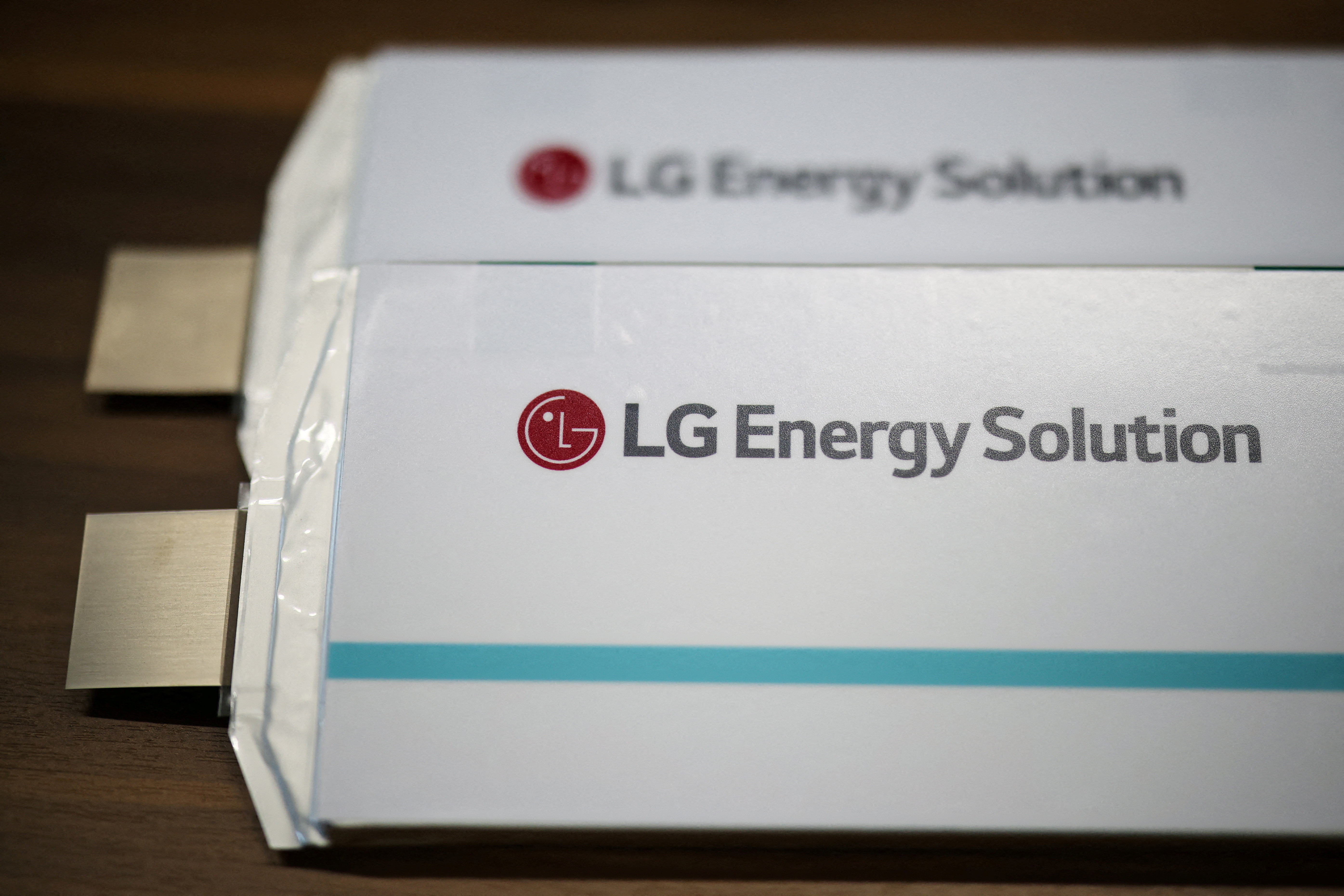
Thai court to decide embattled PM Srettha's fate on August 14
Thailand's Constitutional Court said on Wednesday it will hand down its verdict on Aug. 14 in a case that seeks the dismissal of Prime Minister Srettha Thavisin for appointing to his cabinet a lawyer who served time in jail.


IMAGES
VIDEO
COMMENTS
We study the cointegration and causality between finance and growth for 22 advanced economies. Our time series analysis suggests that causality patterns depend on whether countries' financial development stems from the stock market or the banking sector. We show that stock market development tends to cause economic development, while a ...
The objective of this study was to examine the causal links between exports. economic growth in COMESA countries so as to test the export-led growth driven export hypotheses. Following Dumitrescu and Hurlin (2012), the Granger causality tests in heterogeneous panels to test for Homogeneous Non hypothesis.
In theory, the causal relation between FDI and GDP growth can run in either direction. On the one hand, according to the “FDI-led growth hypothesis†, FDI inflows can stimulate growth for the host countries by increasing the capital stock, creating new job opportunities, and easing the transfer of technology (Borensztein et al., 1998 ...
(2007) conducted a panel-data analysis of the export-driven growth theory in 81 countries for the period 1960-2003. Exports were found to positively cause output growth. Similarly, Bahmani-Oskooee and Oyolola (2007), moving from traditional cointegration techniques of analysis, introduced the bounds test to examine the exports-growth nexus.
Theoretically, the innovation-based growth hypothesis suggests that there is a positive linkage between innovation and economic growth. According to this hypothesis, ... The hypothesis of innovation-based economic growth: A causal relationship. Uluslararası İktisadi ve İdari İncelemeler Dergisi 2013, 1-16. [Google Scholar]
This paper empirically investigates the relationship between tourism and economic growth by using a panel data cointegration test, Granger causality test and Wavelet coherence analysis at the global level. This analysis examines 105 nations utilising panel data from 2003 to 2020. The findings indicates that in most regions, tourism contributes significantly to economic growth and vice versa.
GROWTH HYPOTHESIS IN NIGERIA: A CO-INTEGRATION, CAUSALITY, AND IMPULSE RESPONSE ANALYSIS Mamit Deme ... variables that show a causal link to economic growth have optimal impact during the third quarter; their impact decays after the third quarter. INTRODUCTION For Nigeria, export earnings make up over 25% of the country's gross domestic ...
The results show strong evidence in favor of Granger causality from GDP growth toward CO \(_2\) emissions. For all groups of countries and all tests (except for high-income countries in Table 18.4), the null hypothesis of non-causality is indeed rejected at the 10% level and often with very small p-values.
The current research explores the dynamic causality relationship between a country's economic growth and its environmental, social, and governance performance in a global sample of 118 countries. Using annual data from 1999 to 2015, this study makes use of Granger causality test for panel data models developed by Dumitrescu and Hurlin (Economic Modelling 29(4), 1450-1460, 2012) and Han et ...
growth as evident in the theories put forward in the various growth models - the classical, the neo-classical and the endogenous theory. For example, the Harrod-Domar (1946) classical growth model for a closed economy puts forth that the ratios of national savings and national capital-output stimulate the growth rate of gross national product ...
The literature on the relationship between export and growth is inconsistent in its conclusions regarding the direction of causality. Most recent contributions argue that inconclusive results among studies are likely to be related to the instability of causal relationships over time or over frequencies.
The linear causality test results indicate that there is no causality between GDP and energy consumption, and therefore a prescriptive policy measure would be to reduce energy consumption in the US without affecting the country's economic growth. This is referred to in the literature as the 'neutrality hypothesis'.
The export-led growth hypothesis is tested using monthly time series data for Shanghai (one of the major exporting provinces in China) using the Granger no-causality procedure developed by Toda and Yamamoto (1995) in a vector autoregresion (VAR) model. Three distinct features in this paper stand out: first, the study of the export-led growth hypothesis using the case of Shanghai is the first ...
"Growth hypothesis": unidirectional causality running form energy to economic growth; increases in energy may contribute to growth process; (4) "Feedback hypothesis": bidirectional causality between energy consumption and economic growth; it implies that energy consumption and economic growth are jointly determined and affected at the ...
For example, for Iran, the Fstatistic of 0.59 implies that one cannot reject the null hypothesis that export growth does not cause output growth at the 10% level of significance. The Box-Pierce statistic of 5.67 indicates that one cannot reject the hypothesis of no residual autocorrelation. On the other hand, the F-statistic of 5.20 for Iran ...
Our contribution is to investigate the causal relationship between the ratio of FDI to GDP (FDIG) and economic growth (GDPG) using a battery of cutting-edge methods and an extensive data set. We employ the heterogeneous-panel tests of the Granger non-causality hypothesis based on the works of Hurlin, C. 2004a.
Overall, asymmetric causality test results reveal that positive FDI shocks have an important causal impact on economic growth in most RCEP countries. Thus, the results of econometric analysis mostly support the argument that the FDI-led growth hypothesis rather than the Growth-led FDI hypothesis in RCEP countries.
GROWTH: A CAUSAL RELATIONSHIP Murat ÇETİN1 ABSTRACT Theoretically, the innovation-based growth hypothesis suggests that there is a positive linkage between innovation and economic growth. R&D plays a major role in innovation, raising productivity and increasing economic growth. In this study, this hypothesis is tested empiricially.
The empirical investigation of the development-democracy-growth hypothesis estimates positive correlations and causal associations between development and democracy and democracy and growth. Among the preconditions for the emergence of DEMOCRACY, MARKET and CULTURE show strong and highly significant positive effects which are largely transmitted directly. DEVELOPMENT affects DEMOCRACY directly ...
Although Donald Trump vows to bring U.S. prices down "very quickly," some economists say his policies could rekindle inflation. Here's what to know.
14 Our analysis also finds that the null hypothesis of joint non-causality from LK t, LHC t, LX t and LIMP t to economic growth is rejected at the five percent level for Bahrain, and at one per cent for Kuwait and UAE. At the same time, the null hypothesis of the joint non-causality from LY t, LK t, LHC t and LIMP t to exports is rejected at conventional levels for all countries except Oman.
The ongoing stock market bubble that has been inflated by a strong rally in AI stocks could pop prematurely if former President Donald Trump wins the 2024 election.
Throughout his executive order, Landry refers to the current iteration of the department as untrustworthy, insufficient and a contributing cause to the state's slowed economic growth.
The political economy of development: A cross-national causality test of development-democracy-growth hypothesis ABBAS POURGERAMI Economics Institute, University of Colorado, Boulder, 1030 13th Street, Boulder, CO 80302 1. Background The development-democracy-growth hypothesis has received much attention from social scientists.
Gearing Up Inclusive Growth. First, we need to address the underlying problem of slow growth. Most of the decline in growth in recent decades has been driven by a slump in productivity. A big reason for the slump is that labor and capital aren't flowing to the most dynamic firms. But a smart mix of reforms could jumpstart medium-term growth.
In some ways, Thomas Crooks seems similar to other young men who've wreaked havoc across the US with high-powered assault-style rifles, officials say.
1. Introduction. The nexus between human capital and economic growth constitutes a longstanding focal point in economic inquiry. The trajectory set by new growth theory underscores the pivotal role of human capital accumulation as a fundamental catalyst for a nation's wealth and prosperity (see for instance: Barro, Citation 1991; Benhabib & Spiegel, Citation 1994; Romer, Citation 1990).
Google's John Mueller says that an exponential growth in content will cause it to be seen as a new site and be re-evaluated. SEJ STAFF Roger Montti . 6 hours ago ; ⋅; 3 min read ...
It is expected to post 13% sales growth for the second quarter. Italian luxury label Brunello Cucinelli , opens new tab has also defied the industry slowdown. Last week it reported first-half ...
The export-led growth hypothesis is tested by estimating an augmented growth equation on the basis of times series data from China. The Granger no-causality procedure developed by Toda and Yamamoto (Journal of Econometrics, 66, 1995) was applied to test the causality link between exports and economic growth in a VAR system. Three distinct ...