Newly Launched - AI Presentation Maker

AI PPT Maker
Powerpoint Templates
PPT Bundles
Kpi Dashboard
Professional
Business Plans
Swot Analysis
Gantt Chart
Business Proposal
Marketing Plan
Project Management
Business Case
Business Model
Cyber Security
Business PPT
Digital Marketing
Digital Transformation
Human Resources
Product Management
Artificial Intelligence
Company Profile
Acknowledgement PPT
PPT Presentation
Reports Brochures
One Page Pitch
Interview PPT
All Categories


Data Mining Powerpoint Presentation Slides
Data mining combines domain experience, computer abilities, and mathematical and statistical understanding to extract valuable insights from data. Here is a professionally designed Data Mining template that will greatly assist companies in presenting an overview of their current scenario and assessing the need for data mining adoption. Further, this data mining module showcases the gap analysis of the company and the introduction of data mining. In addition, it contains information on the requirements for the data mining adoption, the life cycle and phases of data mining, and critical components of the data mining. Furthermore, this data mining template includes the data mining tools such as SAS, Apache Spark, Excel, Tableau, NLP, and Tensor Flow, along with data mining in decision making. Moreover, this module highlights the difference between data mining and other tools, data mining workflow, job roles in data mining, and top data mining applications such as healthcare, logistic, finance, airlines, and business. Lastly, this template comprises a dashboard and impacts of the data mining integration on the organization. Download it now.
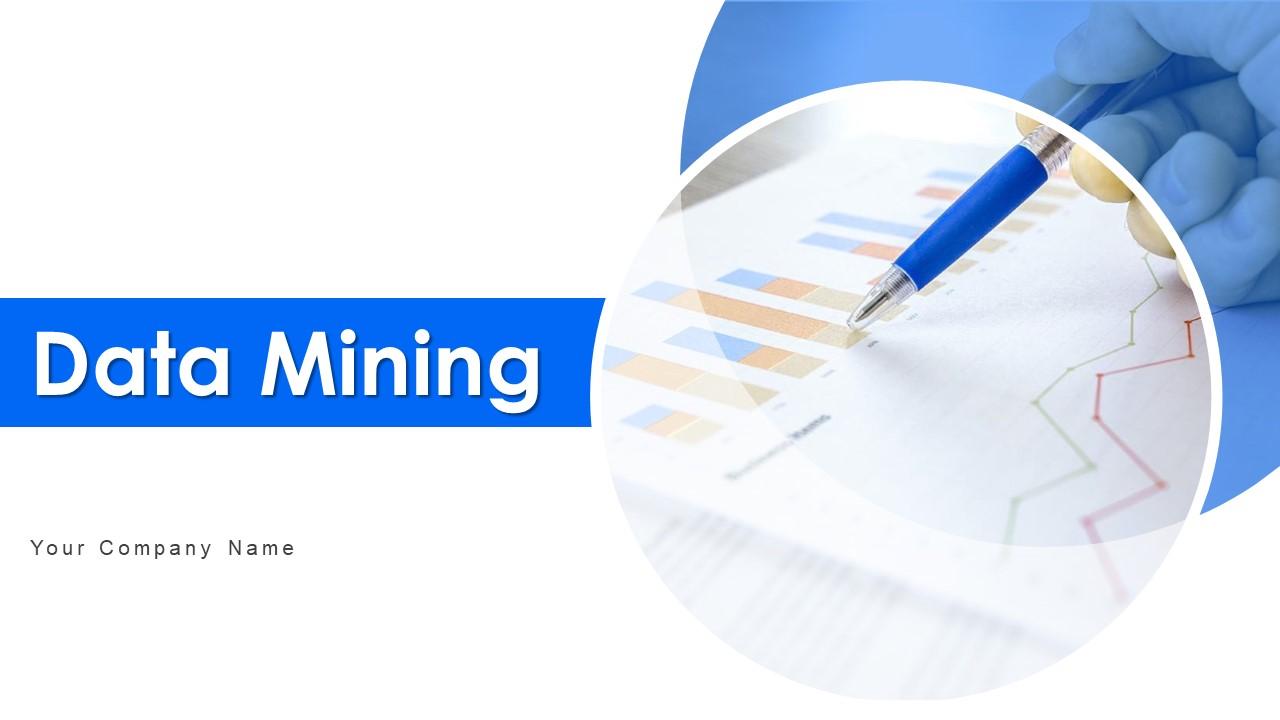
- Add a user to your subscription for free
You must be logged in to download this presentation.
PowerPoint presentation slides
Deliver this complete deck to your team members and other collaborators. Encompassed with stylized slides presenting various concepts, this Data Mining Powerpoint Presentation Slides is the best tool you can utilize. Personalize its content and graphics to make it unique and thought provoking. All the eighty two slides are editable and modifiable, so feel free to adjust them to your business setting. The font, color, and other components also come in an editable format making this PPT design the best choice for your next presentation. So, download now.
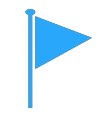
People who downloaded this PowerPoint presentation also viewed the following :
- Complete Decks , All Decks , IT
- Phases Of Data Science ,
- Tools Of Data Science ,
- Job Roles In Data Science
Content of this Powerpoint Presentation
Slide 1 : This slide introduces Data Mining. State Your Company Name and begin. Slide 2 : This is an Agenda slide. State your agendas here. Slide 3 : This slide shows Table of Content for the presentation. Slide 4 : This is another slide continuing Table of Content for the presentation. Slide 5 : This slide highlights title for topics that are to be covered next in the template. Slide 6 : This slide presents current situation of our business by displaying the ratio of unstructured and structured data stored in the database. Slide 7 : This slide shows how unstructured data is causing challenges and how data science will help provide solutions. Slide 8 : This slide highlights title for topics that are to be covered next in the template. Slide 9 : This slide represents the need of the data science in the organization. Slide 10 : This slide shows Benefits of Data Mining to the Organization. Slide 11 : This slide presents the role of data Mining in decision making, and it includes collection & acquisition, storage, cleaning of data, etc. Slide 12 : This slide highlights title for topics that are to be covered next in the template. Slide 13 : This slide displays the prerequisites for data Mining that include knowledge of machine learning, modeling, statistic, database, and programming languages. Slide 14 : This slide represents Data Scientist Must Have Skills Before Implementing Data Science. Slide 15 : This is another slide showing Data Scientist must have Skills before Implementing Data Science. Slide 16 : This slide highlights title for topics that are to be covered next in the template. Slide 17 : This slide describes the life cycle of data science, which includes the stages such as predefined business problems, information acquisition, etc. Slide 18 : This slide displays the first phase of data science that is understanding business problems and the facts that come under this phase. Slide 19 : This slide represents the data preparation phase of data science, including its various stages such as raw data, structure data, data preprocessing, EDA, etc. Slide 20 : This slide shows Information Acquisition in Data Preparation Phase. Slide 21 : This slide presents model planning phase in data science and shows its tools, such as SQL Analysis Service, R, and SAS/ACCESS. Slide 22 : This slide shows exploratory data analysis in the model planning phase of data science and its various stages and reasons. Slide 23 : This slide displays various tools that could help in data modeling such as SAS enterprise miner, SPCS modeler, MATLAB, etc. Slide 24 : This slide represents operational phase of data science and what tasks are performed in this phase. Slide 25 : This slide shows last phase of the data science and in this phase, all the key findings are communicated to stakeholders. Slide 26 : This slide presents how data scientists throughout the project manage data till completion. Slide 27 : This slide highlights title for topics that are to be covered next in the template. Slide 28 : This slide displays top tools that are used in data science which include SAS, Apache Spark, Excel, etc. Slide 29 : This slide represents Statistical Analysis System used in data science for data management and modeling. Slide 30 : This slide shows Apache Spark tool used in data science and its features such as speed, reusability, advanced analytics, etc. Slide 31 : This slide presents excel tool used in data science and its usage along with its features. Slide 32 : This slide shows tool used in data science and its features such as licensing views, subscription of others, etc. Slide 33 : This slide displays Tools for Data Science- Natural Language Toolkit (NLTK). Slide 34 : This slide represents TensorFlow tool used in Data Science, and its features include flexibility, columns, visualizer, etc. Slide 35 : This slide highlights title for topics that are to be covered next in the template. Slide 36 : This slide presents difference between data science and data analytics based on skillset, scope, exploration and goals. Slide 37 : This slide shows difference between Business Intelligence and Data Science based on the factors such as concept, scope, data, etc. Slide 38 : This slide highlights title for topics that are to be covered next in the template. Slide 39 : This slide represents tasks performed by the business analyst and how he will be helpful to improve business operations. Slide 40 : This slide shows data engineers’ responsibilities and skills that they should possess. Slide 41 : This slide presents tasks performed by a Database Administrator and skills that he should possess. Slide 42 : This slide shows machine learning engineer’s tasks and skills, including a deep knowledge of machine learning, ML algorithms, and Python and C++. Slide 43 : This slide displays the tasks performed by data scientists in data science and their skills. Slide 44 : This slide represents the different types of data scientists, including vertical experts, stat DS managers, generalists, etc. Slide 45 : This slide shows data architect’s tasks in data science projects and their skills. Slide 46 : This slide presents tasks performed by a statistician in data science and his skills such as data mining, distributive computing, etc. Slide 47 : This slide shows tasks performed by the business analyst and how he will be helpful to improve business operations. Slide 48 : This slide displays tasks performed by a data and analytics manager and skills he should have. Slide 49 : This slide represents RACI matrix for data Mining and tasks performed by data analysts, data engineers, data scientists, etc. Slide 50 : This slide highlights title for topics that are to be covered next in the template. Slide 51 : This slide presents Checklist for Effective Data Science Integration in Business. Slide 52 : This slide highlights title for topics that are to be covered next in the template. Slide 53 : This slide displays Table of Content highlighting Timeline for Data Mining Implementation in the Organization. Slide 54 : This slide represents Table of Content highlighting Roadmap to Integrate Data Mining in the Organization. Slide 55 : This slide shows Roadmap to Integrate Data Mining in the Organization. Slide 56 : This slide highlights title for topics that are to be covered next in the template. Slide 57 : This slide shows 30-60-90 Days Plan for Data Mining Implementation. Slide 58 : This slide displays Dashboard for Data Mining Implementation. Slide 59 : This slide represents dashboard for data integration in the business, and it is showing real-time details about expenses, profits, margins percentage, etc. Slide 60 : This slide highlights title for topics that are to be covered next in the template. Slide 61 : This slide presents Impacts of Data Mining Integration in the Organization. Slide 62 : This slide highlights title for topics that are to be covered next in the template. Slide 63 : This slide displays Domains where Data Mining is Creating its Impression. Slide 64 : This slide represents data Mining in healthcare departments and its benefits in different ways. Slide 65 : This slide shows Data Mining in Logistics and Transportation Department. Slide 66 : This slide presents data Mining role in airlines and its benefits that cover revenue management and route planning. Slide 67 : This slide shows application of data Mining in financial organizations and its benefits. Slide 68 : This slide displays the data Mining application in business and its benefits. Slide 69 : This slide highlights title for topics that are to be covered next in the template. Slide 70 : This slide shows the meaning of data Mining and how this innovation is helpful in businesses developing AI systems. Slide 71 : This slide presents critical components of data Mining such as data, programming, statistics & probability, etc. Slide 72 : This slide displays Icons for Data Mining. Slide 73 : This slide is titled as Additional Slides for moving forward. Slide 74 : This is Our Mission slide with related imagery and text. Slide 75 : This slide presents Bar chart with two products comparison. Slide 76 : This slide showcases Magnifying Glass to highlight information, specifications etc Slide 77 : This is a Timeline slide. Show data related to time intervals here. Slide 78 : This slide shows Post It Notes. Post your important notes here. Slide 79 : This is an Idea Generation slide to state a new idea or highlight information, specifications etc. Slide 80 : This slide presents Roadmap with additional textboxes. Slide 81 : This slide provides 30 60 90 Days Plan with text boxes. Slide 82 : This is a Thank You slide with address, contact numbers and email address.
Data Mining Powerpoint Presentation Slides with all 87 slides:
Use our Data Mining Powerpoint Presentation Slides to effectively help you save your valuable time. They are readymade to fit into any presentation structure.
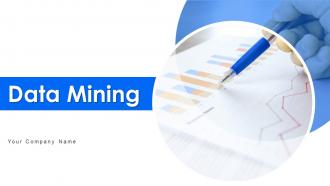
The need for data science arises from the enormous amount of structured and unstructured data that organizations generate. Data science helps in analyzing this data, which leads to insights that can improve business processes, make better decisions, and increase revenue.
Data mining provides a variety of benefits to organizations, such as identifying patterns and trends, reducing costs, increasing revenue, improving customer relationships, and enhancing business operations.
The lifecycle of data science includes several stages, such as defining business problems, data preparation, modeling, testing, and deployment.
The different types of data scientists include vertical experts, statistical data scientists, machine learning engineers, generalists, etc. They possess skills such as deep knowledge of machine learning, programming languages, and analytics.
Data science is a broader field that includes data analytics, machine learning, and deep learning, while data analytics is a subset of data science that focuses on analyzing historical data to uncover insights that can improve business operations.
Ratings and Reviews
by Miller Rogers
April 20, 2022
by Cruz Hayes
April 19, 2022

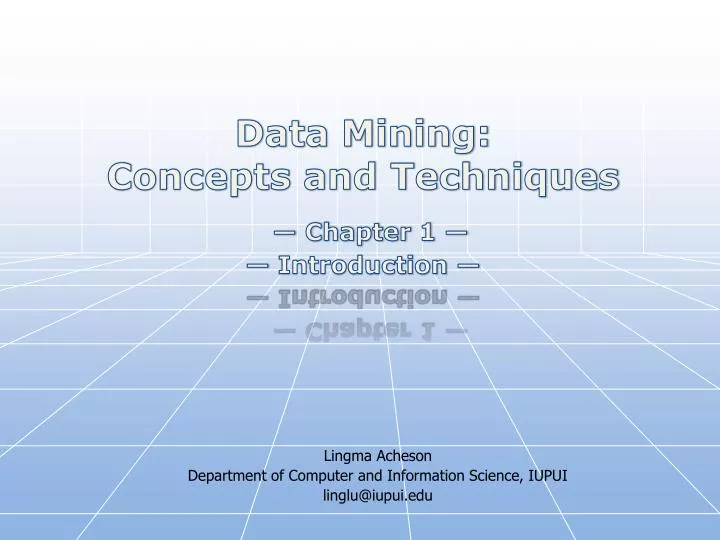
Data Mining: Concepts and Techniques — Chapter 1 — — Introduction —
Sep 07, 2014
560 likes | 1.11k Views
Data Mining: Concepts and Techniques — Chapter 1 — — Introduction —. Lingma Acheson Department of Computer and Information Science, IUPUI [email protected]. Outline. 1.1 Motivation: Why data mining? 1.2 What is data mining? 1.3 Data Mining: On what kind of data?
Share Presentation
- data mining
- data warehouses
- relational databases
- 3 data mining
- data selection data relevant
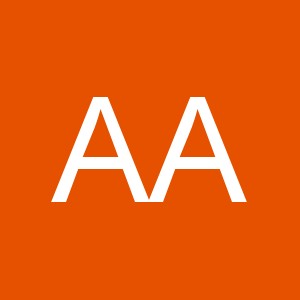
Presentation Transcript
Data Mining: Concepts and Techniques— Chapter 1 —— Introduction — Lingma Acheson Department of Computer and Information Science, IUPUI [email protected]
Outline • 1.1 Motivation: Why data mining? • 1.2 What is data mining? • 1.3 Data Mining: On what kind of data? • 1.4 Data mining functionality: What kinds of Patterns Can Be Mined? • 1.5 Are all the patterns interesting? • 1.6 Classification of data mining systems • 1.7 Data Mining Task Primitives • 1.8 Integration of data mining system with a DB and DW System • 1.9 Major issues in data mining Data Mining: Concepts and Techniques
1.1 Why Data Mining? • The Explosive Growth of Data: from terabytes(10004) to yottabytes(10008) • Data collection and data availability • Automated data collection tools, database systems, web • Major sources of abundant data • Business: Web, e-commerce, transactions, stocks, … • Science: bioinformatics, scientific simulation, medical research … • Society and everyone: news, digital cameras, … • Data rich but information poor! • What does those data mean? • How to analyze data? • Data mining — Automated analysis of massive data sets Data Mining: Concepts and Techniques
Evolution of Database Technology
1.2 What Is Data Mining? • Data mining (knowledge discovery from data) • Extraction of interesting (non-trivial,implicit, previously unknown and potentially useful)patterns or knowledge from huge amount of data • Data mining: a misnomer? • Alternative names • Knowledge discovery (mining) in databases (KDD), knowledge extraction, data/pattern analysis, data archeology, data dredging, information harvesting, business intelligence, etc. Data Mining: Concepts and Techniques
Potential Applications • Data analysis and decision support • Market analysis and management • Target marketing, customer relationship management (CRM), market basket analysis, cross selling, market segmentation • Risk analysis and management • Forecasting, customer retention, improved underwriting, quality control, competitive analysis • Fraud detection and detection of unusual patterns (outliers) • Other Applications • Text mining (news group, email, documents) and Web mining • Stream data mining • Bioinformatics and bio-data analysis Data Mining: Concepts and Techniques
Ex.: Market Analysis and Management • Where does the data come from?—Credit card transactions, loyalty cards, discount coupons, customer complaint calls, surveys … • Target marketing • Find clusters of “model” customers who share the same characteristics: interest, income level, spending habits, etc., • E.g. Most customers with income level 60k – 80k with food expenses $600 - $800 a month live in that area • Determine customer purchasing patterns over time • E.g. Customers who are between 20 and 29 years old, with income of 20k – 29k usually buy this type of CD player • Cross-market analysis—Find associations/co-relations between product sales, & predict based on such association • E.g. Customers who buy computer A usually buy software B Data Mining: Concepts and Techniques
Ex.: Market Analysis and Management (2) • Customer requirement analysis • Identify the best products for different customers • Predict what factors will attract new customers • Provision of summary information • Multidimensional summary reports • E.g. Summarize all transactions of the first quarter from three different branches Summarize all transactions of last year from a particular branch Summarize all transactions of a particular product • Statistical summary information • E.g. What is the average age for customers who buy product A? • Fraud detection • Find outliers of unusual transactions • Financial planning • Summarize and compare the resources and spending Data Mining: Concepts and Techniques
Knowledge Discovery (KDD) Process Data Mining: Concepts and Techniques
KDD Process: Several Key Steps • Learning the application domain • relevant prior knowledge and goals of application • Identifying a target data set: data selection • Data processing • Data cleaning (remove noise and inconsistent data) • Data integration (multiple data sources maybe combined) • Data selection (data relevant to the analysis task are retrieved from database) • Data transformation (data transformed or consolidated into forms appropriate for mining) (Done with data preprocessing) • Data mining (an essential process where intelligent methods are applied to extract data patterns) • Pattern evaluation (indentify the truly interesting patterns) • Knowledge presentation (mined knowledge is presented to the user with visualization or representation techniques) • Use of discovered knowledge Data Mining: Concepts and Techniques
Data Mining and Business Intelligence Increasing potential to support business decisions End User DecisionMaking Business Analyst Data Presentation Visualization Techniques Data Mining Data Analyst Information Discovery Data Exploration Statistical Summary, Querying, and Reporting Data Preprocessing/Integration, Data Warehouses DBA Data Sources Paper, Files, Web documents, Scientific experiments, Database Systems Data Mining: Concepts and Techniques
A typical DM System Architecture • Database, data warehouse, WWW or other information repository (store data) • Database or data warehouse server (fetch and combine data) • Knowledge base (turn data into meaningful groups according to domain knowledge) • Data mining engine (perform mining tasks) • Pattern evaluation module (find interesting patterns) • User interface (interact with the user) Data Mining: Concepts and Techniques
A typical DM System Architecture (2)
Database Technology Statistics Data Mining Information Science Visualization Other Disciplines Confluence of Multiple Disciplines Machine Learning • Not all “Data Mining System” performs true data mining • machine learning system, statistical analysis (small amount of data) • Database system (information retrieval, deductive querying…) Data Mining: Concepts and Techniques
1.3 On What Kinds of Data? • Database-oriented data sets and applications • Relational database, data warehouse, transactional database • Advanced data sets and advanced applications • Object-Relational Databases • Temporal Databases, Sequence Databases, Time-Series databases • Spatial Databases and Spatiotemporal Databases • Text databases and Multimedia databases • Heterogeneous Databases and Legacy Databases • Data Streams • The World-Wide Web Data Mining: Concepts and Techniques
Relational Databases • DBMS – database management system, contains a collection of interrelated databases e.g. Faculty database, student database, publications database • Each database contains a collection of tables and functions to manage and access the data. e.g. student_bio, student_graduation, student_parking • Each table contains columns and rows, with columns as attributes of data and rows as records. • Tables can be used to represent the relationships between or among multiple tables. Data Mining: Concepts and Techniques
Relational Databases (2) – AllElectronics store Data Mining: Concepts and Techniques
Relational Databases (3) • With a relational query language, e.g. SQL, we will be able to find answers to questions such as: • How many items were sold last year? • Who has earned commissions higher than 10%? • What is the total sales of last month for Dell laptops? • When data mining is applied to relational databases, we can search for trends or data patterns. • Relational databases are one of the most commonly available and rich information repositories, and thus are a major data form in our study. Data Mining: Concepts and Techniques
Data Warehouses • A repository of information collected from multiple sources, stored under a unified schema, and that usually resides at a single site. • Constructed via a process of data cleaning, data integration, data transformation, data loading and periodic data refreshing. Data Mining: Concepts and Techniques
Data Warehouses (2) • Data are organized around major subjects, e.g. customer, item, supplier and activity. • Provide information from a historical perspective (e.g. from the past 5 – 10 years) • Typically summarized to a higher level (e.g. a summary of the transactions per item type for each store) • User can perform drill-down or roll-up operation to view the data at different degrees of summarization Data Mining: Concepts and Techniques
Data Warehouses (3)
Transactional Databases • Consists of a file where each record represents a transaction • A transaction typically includes a unique transaction ID and a list of the items making up the transaction. • Either stored in a flat file or unfolded into relational tables • Easy to identify items that are frequently sold together Data Mining: Concepts and Techniques
1.4 Data Mining Functionalities - What kinds of patterns can be mined? • Concept/Class Description: Characterization and Discrimination • Data can be associated with classes or concepts. • E.g. classes of items – computers, printers, … concepts of customers – bigSpenders, budgetSpenders, … • How to describe these items or concepts? • Descriptions can be derived via • Data characterization – summarizing the general characteristics of a target class of data. • E.g. summarizing the characteristics of customers who spend more than $1,000 a year at AllElectronics. Result can be a general profile of the customers, such as 40 – 50 years old, employed, have excellent credit ratings. Data Mining: Concepts and Techniques
1.4 Data Mining Functionalities - What kinds of patterns can be mined? • Data discrimination – comparing the target class with one or a set of comparative classes • E.g. Compare the general features of software products whole sales increase by 10% in the last year with those whose sales decrease by 30% during the same period • Or both of the above • Mining Frequent Patterns, Associations and Correlations • Frequent itemset: a set of items that frequently appear together in a transactional data set (e.g. milk and bread) • Frequent subsequence: a pattern that customers tend to purchase product A, followed by a purchase of product B Data Mining: Concepts and Techniques
1.4 Data Mining Functionalities - What kinds of patterns can be mined? • Association Analysis: find frequent patterns • E.g. a sample analysis result – an association rule: buys(X, “computer”) => buys(X, “software”) [support = 1%, confidence = 50%] (if a customer buys a computer, there is a 50% chance that she will buy software. 1% of all of the transactions under analysis showed that computer and software are purchased together. ) • Associations rules are discarded as uninteresting if they do not satisfy both a minimum support threshold and a minimum confidence threshold. • Correlation Analysis: additional analysis to find statistical correlations between associated pairs Data Mining: Concepts and Techniques
1.4 Data Mining Functionalities - What kinds of patterns can be mined? • Classification and Prediction • Classification • The process of finding a model that describes and distinguishes the data classes or concepts, for the purpose of being able to use the model to predict the class of objects whose class label is unknown. • The derived model is based on the analysis of a set of training data (data objects whose class label is known). • The model can be represented in classification (IF-THEN) rules, decision trees, neural networks, etc. • Prediction • Predict missing or unavailable numerical data values Data Mining: Concepts and Techniques
1.4 Data Mining Functionalities - What kinds of patterns can be mined? Data Mining: Concepts and Techniques
Data Mining Functionalities (2) • Cluster Analysis • Class label is unknown: group data to form new classes • Clusters of objects are formed based on the principle of maximizing intra-class similarity & minimizing interclass similarity • E.g. Identify homogeneous subpopulations of customers. These clusters may represent individual target groups for marketing. Data Mining: Concepts and Techniques
Data Mining Functionalities (2) • Outlier Analysis • Data that do no comply with the general behavior or model. • Outliers are usually discarded as noise or exceptions. • Useful for fraud detection. • E.g. Detect purchases of extremely large amounts • Evolution Analysis • Describes and models regularities or trends for objects whose behavior changes over time. • E.g. Identify stock evolution regularities for overall stocks and for the stocks of particular companies. Data Mining: Concepts and Techniques
1.5 Are All of the Patterns Interesting? • Data mining may generate thousands of patterns: Not all of them are interesting • A pattern is interesting if it is • easily understood by humans • validon newor test data with some degree of certainty, • potentially useful • novel • validates some hypothesis that a user seeks to confirm • An interesting measure represents knowledge ! Data Mining: Concepts and Techniques
1.5 Are All of the Patterns Interesting? • Objective measures • Based on statistics and structures of patterns, e.g., support, confidence, etc. (Rules that do not satisfy a threshold are considered uninteresting.) • Subjective measures • Reflect the needs and interests of a particular user. • E.g. A marketing manager is only interested in characteristics of customers who shop frequently. • Based on user’s belief in the data. • e.g., Patterns are interesting if they are unexpected, or can be used for strategic planning, etc • Objective and subjective measures need to be combined. Data Mining: Concepts and Techniques
1.5 Are All of the Patterns Interesting? • Find all the interesting patterns: Completeness • Unrealistic and inefficient • User-provided constraints and interestingness measures should be used • Search for only interesting patterns: An optimization problem • Highly desirable • No need to search through the generated patterns to identify truly interesting ones. • Measures can be used to rank the discovered patterns according their interestingness. Data Mining: Concepts and Techniques
Database Technology Statistics Data Mining Information Science Visualization Other Disciplines 1.6 Classification of data mining systems Machine Learning
1.6 Classification of data mining systems • Database • Relational, data warehouse, transactional, stream, object-oriented/relational, active, spatial, time-series, text, multi-media, heterogeneous, legacy, WWW • Knowledge • Characterization, discrimination, association, classification, clustering, trend/deviation, outlier analysis, etc. • Multiple/integrated functions and mining at multiple levels • Techniques utilized • Database-oriented, data warehouse (OLAP), machine learning, statistics, visualization, etc. • Applications adapted • Retail, telecommunication, banking, fraud analysis, bio-data mining, stock market analysis, text mining, Web mining, etc.
1.7 Data Mining Task Primitives • How to construct a data mining query? • The primitives allow the user to interactively communicate with the data mining system during discovery to direct the mining process, or examine the findings Data Mining: Concepts and Techniques
1.7 Data Mining Task Primitives • The primitives specify: (1) The set of task-relevant data – which portion of the database to be used • Database or data warehouse name • Database tables or data warehouse cubes • Condition for data selection • Relevant attributes or dimensions • Data grouping criteria Data Mining: Concepts and Techniques
1.7 Data Mining Task Primitives • The primitives specify: (2) The kind of knowledge to be mined – what DB functions to be performed • Characterization • Discrimination • Association • Classification/prediction • Clustering • Outlier analysis • Other data mining tasks Data Mining: Concepts and Techniques
1.7 Data Mining Task Primitives (3) The background knowledge to be used – what domain knowledge, concept hierarchies, etc. (4) Interestingness measures and thresholds – support, confidence, etc. (5) Visualization methods – what form to display the result, e.g. rules, tables, charts, graphs, … Data Mining: Concepts and Techniques
1.7 Data Mining Task Primitives • DMQL – Data Mining Query Language • Designed to incorporate these primitives • Allow user to interact with DM systems • Providing a standardized language like SQL Data Mining: Concepts and Techniques
An Example Query in DMQL (1) (3) (2) (1) (1) (1) (2) (1) (5) Data Mining: Concepts and Techniques
Why Data Mining Query Language? • Automated vs. query-driven? • Finding all the patterns autonomously in a database?—unrealistic because the patterns could be too many but uninteresting • Data mining should be an interactive process • User directs what to be mined • Users must be provided with a set of primitives to be used to communicate with the data mining system • Incorporating these primitives in a data mining query language • More flexible user interaction • Foundation for design of graphical user interface • Standardization of data mining industry and practice Data Mining: Concepts and Techniques
1.8 Integration of Data Mining and Data Warehousing • No coupling • Flat file processing, no utilization of any functions of a DB/DW • system • Not recommended • Loose coupling • Fetching data from DB/DW • Does not explore data structures and query optimization methods provided by DB/DW system • Difficult to achieve high scalability and good performance with • large data sets Data Mining: Concepts and Techniques
1.8 Integration of Data Mining and Data Warehousing • Semi-tight • Efficient implementations of a few essential data mining primitives in a DB/DW system are provided, e.g., sorting, indexing, aggregation, • histogram analysis, multiway join, precomputation of some stat • functions • Enhanced DM performance • Tight • DM is smoothly integrated into a DB/DW system, mining query is • optimized based on mining query analysis, data structures, indexing, query processing methods of a DB/DW system • A uniform information processing environment, highly desirable Data Mining: Concepts and Techniques
1.9 Major Issues in Data Mining • Mining methodology and User interaction • Mining different kinds of knowledge • DM should cover a wide spectrum of data analysis and knowledge discovery tasks • Enable to use the database in different ways • Require the development of numerous data mining techniques • Interactive mining of knowledge at multiple levels of abstraction • Difficult to know exactly what will be discovered • Allow users to focus the search, refine data mining requests • Incorporation of background knowledge • Guide the discovery process • Allow discovered patterns to be expressed in concise terms and different levels of abstraction • Data mining query languages and ad hoc data mining • High-level query languages need to be developed • Should be integrated with a DB/DW query language Data Mining: Concepts and Techniques
1.9 Major Issues in Data Mining • Presentation and visualization of results • Knowledge should be easily understood and directly usable • High level languages, visual representations or other expressive forms • Require the DM system to adopt the above techniques • Handling noisy or incomplete data • Require data cleaning methods and data analysis methods that can handle noise • Pattern evaluation – the interestingness problem • How to develop techniques to access the interestingness of discovered patterns, especially with subjective measures bases on user beliefs or expectations Data Mining: Concepts and Techniques
1.9 Major Issues in Data Mining • Performance Issues • Efficiency and scalability • Huge amount of data • Running time must be predictable and acceptable • Parallel, distributed and incremental mining algorithms • Divide the data into partitions and processed in parallel • Incorporate database updates without having to mine the entire data again from scratch • Diversity of Database Types • Other database that contain complex data objects, multimedia data, spatial data, etc. • Expect to have different DM systems for different kinds of data • Heterogeneous databases and global information systems • Web mining becomes a very challenging and fast-evolving field in data mining Data Mining: Concepts and Techniques
- More by User
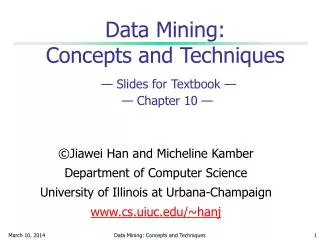
Data Mining: Concepts and Techniques — Slides for Textbook — — Chapter 10 —
Data Mining: Concepts and Techniques — Slides for Textbook — — Chapter 10 —. © Jiawei Han and Micheline Kamber Department of Computer Science University of Illinois at Urbana-Champaign www.cs.uiuc.edu/~hanj. Chapter 10: Applications and Trends in Data Mining. Data mining applications
1.12k views • 55 slides
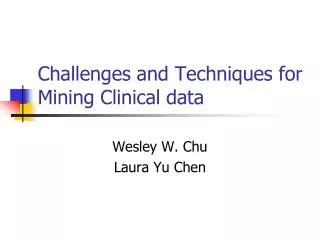
Challenges and Techniques for Mining Clinical data
Challenges and Techniques for Mining Clinical data. Wesley W. Chu Laura Yu Chen. Outline. Introduction of SmartRule association rule mining Case I: mining pregnancy data to discover drug exposure side effects Case II: mining urology clinical data for operation decision making.
613 views • 35 slides
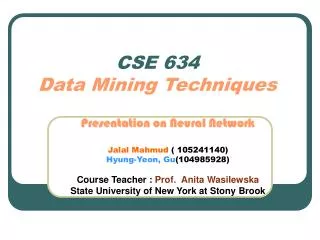
CSE 634 Data Mining Techniques
CSE 634 Data Mining Techniques. Presentation on Neural Network Jalal Mahmud ( 105241140) Hyung-Yeon, Gu (104985928) Course Teacher : Prof. Anita Wasilewska State University of New York at Stony Brook. References. Data Mining Concept and Techniques (Chapter 7.5)
1.17k views • 55 slides
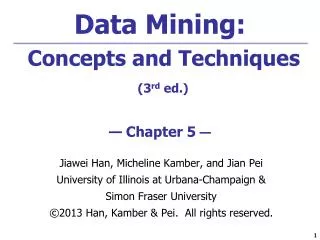
Data Mining: Concepts and Techniques (3 rd ed.) — Chapter 5 —
Data Mining: Concepts and Techniques (3 rd ed.) — Chapter 5 —. Jiawei Han, Micheline Kamber, and Jian Pei University of Illinois at Urbana-Champaign & Simon Fraser University ©2013 Han, Kamber & Pei. All rights reserved. 1. Chapter 5: Data Cube Technology.
2.06k views • 86 slides
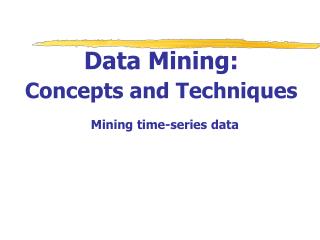
Data Mining: Concepts and Techniques Mining time-series data
Data Mining: Concepts and Techniques Mining time-series data. Time-Series and Sequential Pattern Mining. Regression and trend analysis—A statistical approach Similarity search in time-series analysis Sequential Pattern Mining Markov Chain Hidden Markov Model. Mining Time-Series Data.
1.99k views • 22 slides
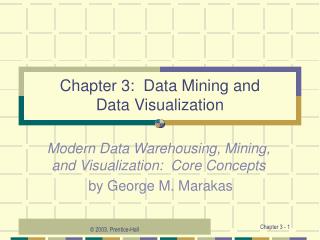
Chapter 3: Data Mining and Data Visualization
Chapter 3: Data Mining and Data Visualization. Modern Data Warehousing, Mining, and Visualization: Core Concepts by George M. Marakas. 3-1: A Picture is Worth a Thousand Words. Data mining is the set of activities used to find new, hidden, or unexpected patterns in data.
829 views • 51 slides
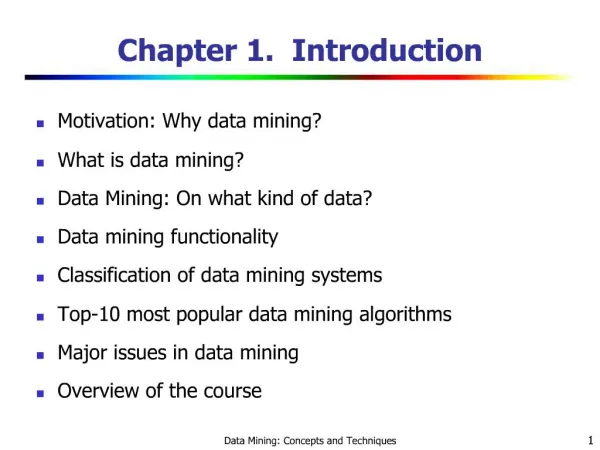

Motivation: Why data mining What is data mining Data Mining: On what kind of data Data mining functionality Classificati
August 26, 2007. Data Mining: Concepts and Techniques. 2. Why Data Mining? . The Explosive Growth of Data: from terabytes to petabytesData collection and data availabilityAutomated data collection tools, database systems, Web, computerized societyMajor sources of abundant dataBusiness: Web, e-co
1.32k views • 46 slides
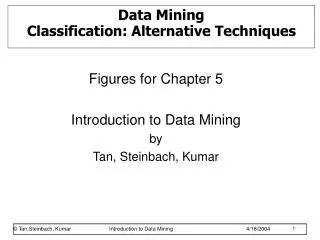
Data Mining Classification: Alternative Techniques
© Tan,Steinbach, Kumar Introduction to Data Mining 4/18/2004 1. Data Mining Classification: Alternative Techniques. Figures for Chapter 5 Introduction to Data Mining by Tan, Steinbach, Kumar.
788 views • 50 slides
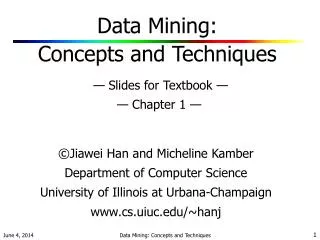
Data Mining: Concepts and Techniques — Slides for Textbook — — Chapter 1 —
Data Mining: Concepts and Techniques — Slides for Textbook — — Chapter 1 —. ©Jiawei Han and Micheline Kamber Department of Computer Science University of Illinois at Urbana-Champaign www.cs.uiuc.edu/~hanj. Data Mining: Concepts and Techniques. Acknowledgements.
1.98k views • 34 slides
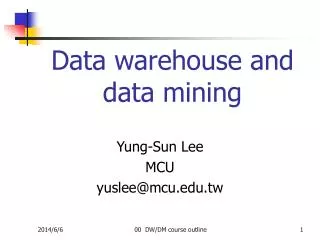
Data warehouse and data mining
Data warehouse and data mining. Yung-Sun Lee MCU [email protected]. Text Book. Data mining concepts and techniques — Jiawei Han, Micheline Kamber, 2007 2e, Morgan Kaufmann Publishers, 新月代理 Reference: 資料探勘 — 曾憲雄等著,旗標, 2005.5
364 views • 4 slides
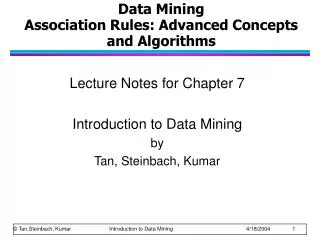
Data Mining Association Rules: Advanced Concepts and Algorithms
© Tan,Steinbach, Kumar Introduction to Data Mining 4/18/2004 1. Data Mining Association Rules: Advanced Concepts and Algorithms. Lecture Notes for Chapter 7 Introduction to Data Mining by Tan, Steinbach, Kumar. Continuous and Categorical Attributes.
982 views • 67 slides
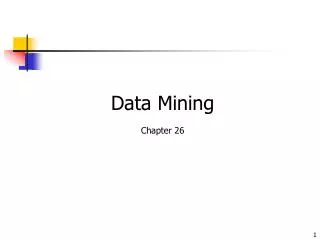
Data Mining Chapter 26
Data Mining Chapter 26. Chapter 1. Introduction. Motivation: Why data mining? What is data mining? Data Mining: On what kind of data? Data mining functionality Are all the patterns interesting? Major issues in data mining. Motivation: “Necessity is the Mother of Invention”.
671 views • 31 slides
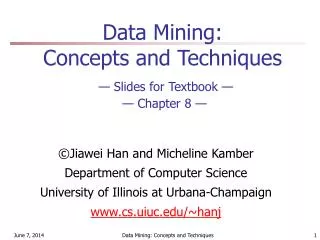
Data Mining: Concepts and Techniques — Slides for Textbook — — Chapter 8 —
Data Mining: Concepts and Techniques — Slides for Textbook — — Chapter 8 —. © Jiawei Han and Micheline Kamber Department of Computer Science University of Illinois at Urbana-Champaign www.cs.uiuc.edu/~hanj. Cluster Analysis. What is Cluster Analysis? Types of Data in Cluster Analysis
506 views • 29 slides
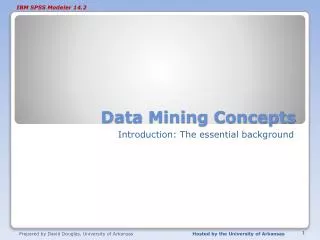
Data Mining Concepts
Data Mining Concepts. Introduction: The essential background. Modules in this Series. The modules in this series are targeted to support using the IBM SPSS Modeler 14.2 using data from DB2 tables on a z10 at the University of Arkansas This module is the introduction to data mining
362 views • 14 slides
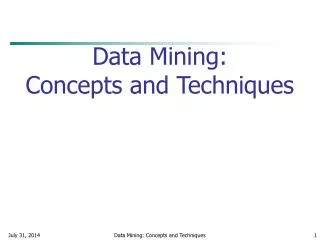
Data Mining: Concepts and Techniques
Data Mining: Concepts and Techniques. Chapter 3: Data Preprocessing. Why preprocess the data? Data cleaning Data integration and transformation Data reduction Discretization and concept hierarchy generation Summary. Why Data Preprocessing?. Data in the real world is dirty
789 views • 50 slides
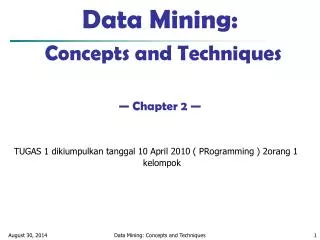
Data Mining: Concepts and Techniques — Chapter 2 —
Data Mining: Concepts and Techniques — Chapter 2 —. TUGAS 1 dikiumpulkan tanggal 10 April 2010 ( PRogramming ) 2orang 1 kelompok. Chapter 2: Data Preprocessing. Karakteristik data secara umum Diskripsi data dan eksplorasi Mengukur kesamaan data Data cleaning
1.37k views • 95 slides
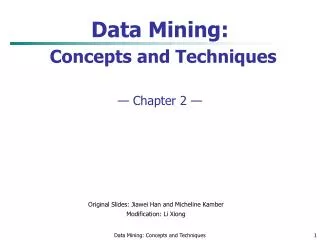
Data Mining: Concepts and Techniques — Chapter 2 —. Original Slides: Jiawei Han and Micheline Kamber Modification: Li Xiong. Chapter 2: Data Preprocessing. Why preprocess the data? Descriptive data summarization Data cleaning Data integration Data transformation Data reduction
3.21k views • 94 slides
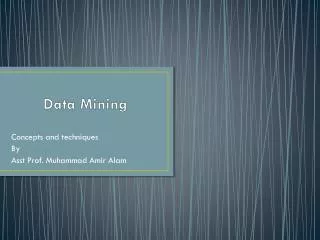
Data Mining
Data Mining. Concepts and techniques By Asst Prof . Muhammad Amir Alam. Data Warehousing and Data Mining. Introduction to Databases. Database A Database is an organized collection of data . Relational Database
414 views • 12 slides
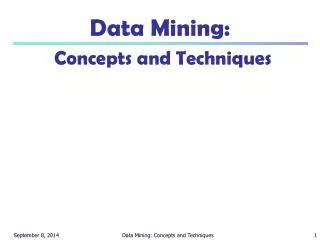
Data Mining: Concepts and Techniques. Chapter 5: Mining Frequent Patterns, Association and Correlations. Basic concepts and a road map Scalable frequent itemset mining methods Mining various kinds of association rules Constraint-based association mining
1.44k views • 107 slides
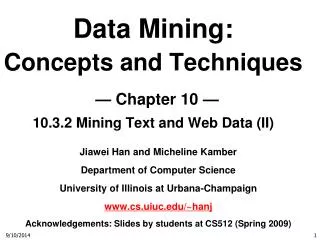
Data Mining: Concepts and Techniques — Chapter 10 — 10.3.2 Mining Text and Web Data (II)
Data Mining: Concepts and Techniques — Chapter 10 — 10.3.2 Mining Text and Web Data (II). Jiawei Han and Micheline Kamber Department of Computer Science University of Illinois at Urbana-Champaign www.cs.uiuc.edu/~hanj Acknowledgements: Slides by students at CS512 (Spring 2009). 9/10/2014.
1.54k views • 75 slides
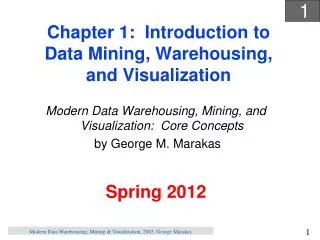
Chapter 1: Introduction to Data Mining, Warehousing, and Visualization
Chapter 1: Introduction to Data Mining, Warehousing, and Visualization. Modern Data Warehousing, Mining, and Visualization: Core Concepts by George M. Marakas Spring 2012. Objectives. What is the purpose and motivation for developing a Data Warehouse (DW)?
600 views • 25 slides
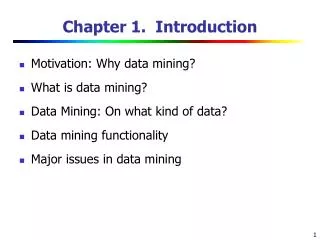
Chapter 1. Introduction
Chapter 1. Introduction. Motivation: Why data mining? What is data mining? Data Mining: On what kind of data? Data mining functionality Major issues in data mining. Why Data Mining?. The Explosive Growth of Data: from terabytes to petabytes Data collection and data availability
4.05k views • 323 slides

COMMENTS
What Is Data Mining? • Data mining (knowledge discovery from data) • Extraction of interesting (non-trivial, implicit, previously unknown and potentially useful) patterns or knowledge from huge amount of data • Alternative names • Knowledge discovery (mining) in databases (KDD), knowledge
Data Mining Powerpoint Presentation Slides with all 87 slides: Use our Data Mining Powerpoint Presentation Slides to effectively help you save your valuable time. They are readymade to fit into any presentation structure.
Data mining is the process of automatically discovering useful information from large data sets. It draws from machine learning, statistics, and database systems to analyze data and identify patterns. Common data mining tasks include classification, clustering, association rule mining, and sequential pattern mining.
This document provides an introduction to data mining and machine learning. It discusses how data mining can extract hidden patterns from large datasets. The document covers common data mining tasks like classification, regression, and clustering.
This document discusses various machine learning techniques for classification and prediction. It covers decision tree induction, tree pruning, Bayesian classification, Bayesian belief networks, backpropagation, association rule mining, and ensemble methods like bagging and boosting.
Outline • 1.1 Motivation: Why data mining? • 1.2 What is data mining? • 1.3 Data Mining: On what kind of data? • 1.4 Data mining functionality: What kinds of Patterns Can Be Mined? • 1.5 Are all the patterns interesting? • 1.6 Classification of data mining systems • 1.7 Data Mining Task Primitives • 1.8 Integration of data ...