- Subject List
- Take a Tour
- For Authors
- Subscriber Services
- Publications
- African American Studies
- African Studies
- American Literature
- Anthropology
- Architecture Planning and Preservation
- Art History
- Atlantic History
- Biblical Studies
- British and Irish Literature
- Childhood Studies
- Chinese Studies
- Cinema and Media Studies
- Communication
- Criminology
- Environmental Science
- Evolutionary Biology
- International Law
- International Relations
- Islamic Studies
- Jewish Studies
- Latin American Studies
- Latino Studies
- Linguistics
- Literary and Critical Theory
- Medieval Studies
- Military History
- Political Science
- Public Health
- Renaissance and Reformation
- Social Work
- Urban Studies
- Victorian Literature
- Browse All Subjects

How to Subscribe
- Free Trials
In This Article Expand or collapse the "in this article" section Data Collection in Educational Research
Introduction, general overviews.
- General Quantitative Overviews
- Questionnaires
- Quantitative Interviewing
- Quantitative Observation
- Technical Properties
- General Qualitative Overviews
- In-Depth Interviewing
- Focus Groups
- Qualitative Observation
- Qualitative Document Analysis
- Visual Analysis
Related Articles Expand or collapse the "related articles" section about
About related articles close popup.
Lorem Ipsum Sit Dolor Amet
Vestibulum ante ipsum primis in faucibus orci luctus et ultrices posuere cubilia Curae; Aliquam ligula odio, euismod ut aliquam et, vestibulum nec risus. Nulla viverra, arcu et iaculis consequat, justo diam ornare tellus, semper ultrices tellus nunc eu tellus.
- Case Study in Education Research
- Grounded Theory
- Methodologies for Conducting Education Research
- Mixed Methods Research
- Qualitative Research Design
- Statistical Assumptions
- Using Ethnography in Educational Research
Other Subject Areas
Forthcoming articles expand or collapse the "forthcoming articles" section.
- Gender, Power, and Politics in the Academy
- Girls' Education in the Developing World
- Non-Formal & Informal Environmental Education
- Find more forthcoming articles...
- Export Citations
- Share This Facebook LinkedIn Twitter
Data Collection in Educational Research by James H. McMillan , Laura P. Gogia LAST REVIEWED: 05 August 2020 LAST MODIFIED: 30 June 2014 DOI: 10.1093/obo/9780199756810-0087
Data collection methods in educational research are used to gather information that is then analyzed and interpreted. As such, data collection is a very important step in conducting research and can influence results significantly. Once the research question and sources of data are identified, appropriate methods of data collection are determined. Data collection includes a broad range of more specific techniques. Historically, much of the data collection performed in educational research depended on methods developed for studies in the field of psychology, a discipline which took what is termed a “quantitative” approach. This involves using instruments, scales, Tests , and structured observation and interviewing. By the mid- to late twentieth centuries, other disciplines such as anthropology and sociology began to influence educational researchers. Forms of data collection broadened to include what is now called “qualitative” methods, with an emphasis on narratives, participant perspectives, and less structured observation and interviewing. As contemporary educational researchers also draw from fields such as business, political science, and medicine, data collection in education has become a multidisciplinary phenomenon. Because data collection is such a broad topic, General Overviews that attempt to cover all or most techniques tend to offer introductory treatments. Few texts, however, provide comprehensive coverage of every data collection technique. Instead, some cover techniques appropriate for either quantitative or qualitative research approaches. Even more focus on one or two data collection methods within those two research contexts. Consequently, after presenting general overviews, this entry is categorized by data collection appropriate for quantitative and Qualitative Data Collection . These sections, in turn, are subdivided into the major types of quantitative and qualitative data collection techniques. While there are some data collection techniques specific to mixed method research design, which implies a combination of qualitative and quantitative research methodologies, these specific procedures are not emphasized in the present article—readers are referred to the Oxford Bibliography article Mixed Methods Research by Nancy Leech for a comprehensive treatment of mixed method data collection techniques. To locate sources for this article, extensive searches were performed using general-use Internet search engines and educational, psychological, and social science research databases. These searches included keywords around data collection and research methods, as well as specific data collection techniques such as surveys, Tests , Focus Groups , and observation. Frequently cited texts and articles, most recent editions at the time, and sources specific to educational research were given priority. Once these sources were identified, their suggested readings and reference lists were mined for other potential sources. Works or scholars found in multiple reference lists were investigated. When applicable, book reviews in peer-reviewed journals were located and taken into account when curating sources. Sources that demonstrated a high level of impact or offered unique coverage of the topic were included.
General educational research overviews typically include several chapters on data collection, organized into qualitative and quantitative approaches. As a rule they are updated frequently so that they offer timely discussions of methodological trends. Most of them are introductory in nature, written for student researchers. Because of the influence of psychology and other social sciences on the development of data collection in educational research, representative works of psychology ( Trochim 2006 ) and of general social sciences ( Robson 2011 ) are included. Available online, Trochim 2006 is a reader-friendly introduction that provides succinct explanations of most quantitative and qualitative approaches. Olsen 2012 is helpful in showing how data collection techniques used in other disciplines have implications for educational studies. Specific to education, Gall, et al. 2007 is a frequently cited text that contains most educational data collection techniques, although it tends to emphasize more traditional quantitative approaches. Johnson and Christensen 2014 offers a more balanced treatment meant for novice researchers and educational research consumers. Cohen, et al. 2011 also provides a balanced approach, but from a British perspective. Fielding, et al. 2008 offer practical advice on recently developed forms of online data collection, with special attention given to the ethical ramifications of Internet-based data collection. Finally, Arthur, et al. 2012 is unique in this section in that it is an edited work offering short overviews of data collection techniques authored by contemporary leading experts.
Arthur, James, Michael Waring, Robert Coe, and Larry Hedges, eds. 2012. Research methods and methodologies in education . London: SAGE.
A diverse edited text discussing trends in study designs, data collection, and data analysis. It includes twelve chapters devoted to different forms of data collection, written by authors who have recently published extensively on the topic. Annotated bibliographies found at the end of each chapter provide guidance for further reading.
Cohen, Louis, Lawrence Manion, and Keith Morrison. 2011. Research methods in education . 7th ed. London: Routledge.
This long-running, bestselling, comprehensive source offers practical advice with clear theoretical foundations. The newest edition has undergone significant revision. Specific to data collection, revisions include new chapters devoted to data collection via the Internet and visual media. Slides highlighting main points are available on a supplementary website.
Fielding, Nigel, Raymond Lee, and Grant Blank. 2008. The SAGE handbook of online research methods . Thousand Oaks, CA: SAGE.
This extensive handbook presents chapters on Internet research design and data collection written by leading scholars in the field. It discusses using the Internet as an archival resource and a research tool, focusing on the most recent trends in multidisciplinary Internet research.
Gall, Meredith, Joyce Gall, and Walter Borg. 2007. Educational research: An introduction . 8th ed. White Plains, NY: Pearson.
A long-standing, well-respected, nuts-and-bolts perspective on data collection meant to prepare students for conducting original research. Although it tends to emphasize quantitative research methodologies, it has a uniquely rich chapter on historical document analysis.
Johnson, Burke, and Larry Christensen. 2014. Educational research: Quantitative, qualitative, and mixed approaches . 5th ed. Thousand Oaks, CA: SAGE.
A comprehensive introductory text for the consumer and the would-be researcher, with extensive lists of additional resources for gathering all types of data. It discusses quantitative and qualitative research methodologies and data collection evenly but provides extended coverage of questionnaire construction.
Olsen, Wendy. 2012. Data collection: Key debates and methods in social research . London: SAGE.
This recently published toolkit of quantitative, qualitative, and mixed method approaches to data collection provides a more contemporary introduction for both students and research professionals. It offers a helpful overview of data collection as an integral part of research in several different fields of study.
Robson, Colin. 2011. Real world research: A resource for users of social research methods in applied settings . West Sussex, UK: Wiley
This introductory text is intended for all social science. There is an applied, integrated emphasis on contemporary quantitative and qualitative data collection techniques in a separate section of the book, including individual and focus group observations, surveys, unstructured and structured interviewing, and tests.
Trochim, William. 2006. Research methods knowledge base
A free online hypertext textbook on applied social research methods. Data collection techniques associated with qualitative and quantitative research are covered comprehensively. Foundational information appropriate for undergraduates and early graduate students is presented through a series of easy-to-navigate and intuitively ordered webpages. Printed editions are available for purchase in an edition written with James Donnelly (Atomic Dog/Cengage Learning, 2008).
back to top
Users without a subscription are not able to see the full content on this page. Please subscribe or login .
Oxford Bibliographies Online is available by subscription and perpetual access to institutions. For more information or to contact an Oxford Sales Representative click here .
- About Education »
- Meet the Editorial Board »
- Academic Achievement
- Academic Audit for Universities
- Academic Freedom and Tenure in the United States
- Action Research in Education
- Adjuncts in Higher Education in the United States
- Administrator Preparation
- Adolescence
- Advanced Placement and International Baccalaureate Courses
- Advocacy and Activism in Early Childhood
- African American Racial Identity and Learning
- Alaska Native Education
- Alternative Certification Programs for Educators
- Alternative Schools
- American Indian Education
- Animals in Environmental Education
- Art Education
- Artificial Intelligence and Learning
- Assessing School Leader Effectiveness
- Assessment, Behavioral
- Assessment, Educational
- Assessment in Early Childhood Education
- Assistive Technology
- Augmented Reality in Education
- Beginning-Teacher Induction
- Bilingual Education and Bilingualism
- Black Undergraduate Women: Critical Race and Gender Perspe...
- Blended Learning
- Changing Professional and Academic Identities
- Character Education
- Children’s and Young Adult Literature
- Children's Beliefs about Intelligence
- Children's Rights in Early Childhood Education
- Citizenship Education
- Civic and Social Engagement of Higher Education
- Classroom Learning Environments: Assessing and Investigati...
- Classroom Management
- Coherent Instructional Systems at the School and School Sy...
- College Admissions in the United States
- College Athletics in the United States
- Community Relations
- Comparative Education
- Computer-Assisted Language Learning
- Computer-Based Testing
- Conceptualizing, Measuring, and Evaluating Improvement Net...
- Continuous Improvement and "High Leverage" Educational Pro...
- Counseling in Schools
- Critical Approaches to Gender in Higher Education
- Critical Perspectives on Educational Innovation and Improv...
- Critical Race Theory
- Crossborder and Transnational Higher Education
- Cross-National Research on Continuous Improvement
- Cross-Sector Research on Continuous Learning and Improveme...
- Cultural Diversity in Early Childhood Education
- Culturally Responsive Leadership
- Culturally Responsive Pedagogies
- Culturally Responsive Teacher Education in the United Stat...
- Curriculum Design
- Data Collection in Educational Research
- Data-driven Decision Making in the United States
- Deaf Education
- Desegregation and Integration
- Design Thinking and the Learning Sciences: Theoretical, Pr...
- Development, Moral
- Dialogic Pedagogy
- Digital Age Teacher, The
- Digital Citizenship
- Digital Divides
- Disabilities
- Distance Learning
- Distributed Leadership
- Doctoral Education and Training
- Early Childhood Education and Care (ECEC) in Denmark
- Early Childhood Education and Development in Mexico
- Early Childhood Education in Aotearoa New Zealand
- Early Childhood Education in Australia
- Early Childhood Education in China
- Early Childhood Education in Europe
- Early Childhood Education in Sub-Saharan Africa
- Early Childhood Education in Sweden
- Early Childhood Education Pedagogy
- Early Childhood Education Policy
- Early Childhood Education, The Arts in
- Early Childhood Mathematics
- Early Childhood Science
- Early Childhood Teacher Education
- Early Childhood Teachers in Aotearoa New Zealand
- Early Years Professionalism and Professionalization Polici...
- Economics of Education
- Education For Children with Autism
- Education for Sustainable Development
- Education Leadership, Empirical Perspectives in
- Education of Native Hawaiian Students
- Education Reform and School Change
- Educational Statistics for Longitudinal Research
- Educator Partnerships with Parents and Families with a Foc...
- Emotional and Affective Issues in Environmental and Sustai...
- Emotional and Behavioral Disorders
- Environmental and Science Education: Overlaps and Issues
- Environmental Education
- Environmental Education in Brazil
- Epistemic Beliefs
- Equity and Improvement: Engaging Communities in Educationa...
- Equity, Ethnicity, Diversity, and Excellence in Education
- Ethical Research with Young Children
- Ethics and Education
- Ethics of Teaching
- Ethnic Studies
- Evidence-Based Communication Assessment and Intervention
- Family and Community Partnerships in Education
- Family Day Care
- Federal Government Programs and Issues
- Feminization of Labor in Academia
- Finance, Education
- Financial Aid
- Formative Assessment
- Future-Focused Education
- Gender and Achievement
- Gender and Alternative Education
- Gender-Based Violence on University Campuses
- Gifted Education
- Global Mindedness and Global Citizenship Education
- Global University Rankings
- Governance, Education
- Growth of Effective Mental Health Services in Schools in t...
- Higher Education and Globalization
- Higher Education and the Developing World
- Higher Education Faculty Characteristics and Trends in the...
- Higher Education Finance
- Higher Education Governance
- Higher Education Graduate Outcomes and Destinations
- Higher Education in Africa
- Higher Education in China
- Higher Education in Latin America
- Higher Education in the United States, Historical Evolutio...
- Higher Education, International Issues in
- Higher Education Management
- Higher Education Policy
- Higher Education Research
- Higher Education Student Assessment
- High-stakes Testing
- History of Early Childhood Education in the United States
- History of Education in the United States
- History of Technology Integration in Education
- Homeschooling
- Inclusion in Early Childhood: Difference, Disability, and ...
- Inclusive Education
- Indigenous Education in a Global Context
- Indigenous Learning Environments
- Indigenous Students in Higher Education in the United Stat...
- Infant and Toddler Pedagogy
- Inservice Teacher Education
- Integrating Art across the Curriculum
- Intelligence
- Intensive Interventions for Children and Adolescents with ...
- International Perspectives on Academic Freedom
- Intersectionality and Education
- Knowledge Development in Early Childhood
- Leadership Development, Coaching and Feedback for
- Leadership in Early Childhood Education
- Leadership Training with an Emphasis on the United States
- Learning Analytics in Higher Education
- Learning Difficulties
- Learning, Lifelong
- Learning, Multimedia
- Learning Strategies
- Legal Matters and Education Law
- LGBT Youth in Schools
- Linguistic Diversity
- Linguistically Inclusive Pedagogy
- Literacy Development and Language Acquisition
- Literature Reviews
- Mathematics Identity
- Mathematics Instruction and Interventions for Students wit...
- Mathematics Teacher Education
- Measurement for Improvement in Education
- Measurement in Education in the United States
- Meta-Analysis and Research Synthesis in Education
- Methodological Approaches for Impact Evaluation in Educati...
- Mindfulness, Learning, and Education
- Motherscholars
- Multiliteracies in Early Childhood Education
- Multiple Documents Literacy: Theory, Research, and Applica...
- Multivariate Research Methodology
- Museums, Education, and Curriculum
- Music Education
- Narrative Research in Education
- Native American Studies
- Note-Taking
- Numeracy Education
- One-to-One Technology in the K-12 Classroom
- Online Education
- Open Education
- Organizing for Continuous Improvement in Education
- Organizing Schools for the Inclusion of Students with Disa...
- Outdoor Play and Learning
- Outdoor Play and Learning in Early Childhood Education
- Pedagogical Leadership
- Pedagogy of Teacher Education, A
- Performance Objectives and Measurement
- Performance-based Research Assessment in Higher Education
- Performance-based Research Funding
- Phenomenology in Educational Research
- Philosophy of Education
- Physical Education
- Podcasts in Education
- Policy Context of United States Educational Innovation and...
- Politics of Education
- Portable Technology Use in Special Education Programs and ...
- Post-humanism and Environmental Education
- Pre-Service Teacher Education
- Problem Solving
- Productivity and Higher Education
- Professional Development
- Professional Learning Communities
- Program Evaluation
- Programs and Services for Students with Emotional or Behav...
- Psychology Learning and Teaching
- Psychometric Issues in the Assessment of English Language ...
- Qualitative Data Analysis Techniques
- Qualitative, Quantitative, and Mixed Methods Research Samp...
- Quantitative Research Designs in Educational Research
- Queering the English Language Arts (ELA) Writing Classroom
- Race and Affirmative Action in Higher Education
- Reading Education
- Refugee and New Immigrant Learners
- Relational and Developmental Trauma and Schools
- Relational Pedagogies in Early Childhood Education
- Reliability in Educational Assessments
- Religion in Elementary and Secondary Education in the Unit...
- Researcher Development and Skills Training within the Cont...
- Research-Practice Partnerships in Education within the Uni...
- Response to Intervention
- Restorative Practices
- Risky Play in Early Childhood Education
- Scale and Sustainability of Education Innovation and Impro...
- Scaling Up Research-based Educational Practices
- School Accreditation
- School Choice
- School Culture
- School District Budgeting and Financial Management in the ...
- School Improvement through Inclusive Education
- School Reform
- Schools, Private and Independent
- School-Wide Positive Behavior Support
- Science Education
- Secondary to Postsecondary Transition Issues
- Self-Regulated Learning
- Self-Study of Teacher Education Practices
- Service-Learning
- Severe Disabilities
- Single Salary Schedule
- Single-sex Education
- Single-Subject Research Design
- Social Context of Education
- Social Justice
- Social Network Analysis
- Social Pedagogy
- Social Science and Education Research
- Social Studies Education
- Sociology of Education
- Standards-Based Education
- Student Access, Equity, and Diversity in Higher Education
- Student Assignment Policy
- Student Engagement in Tertiary Education
- Student Learning, Development, Engagement, and Motivation ...
- Student Participation
- Student Voice in Teacher Development
- Sustainability Education in Early Childhood Education
- Sustainability in Early Childhood Education
- Sustainability in Higher Education
- Teacher Beliefs and Epistemologies
- Teacher Collaboration in School Improvement
- Teacher Evaluation and Teacher Effectiveness
- Teacher Preparation
- Teacher Training and Development
- Teacher Unions and Associations
- Teacher-Student Relationships
- Teaching Critical Thinking
- Technologies, Teaching, and Learning in Higher Education
- Technology Education in Early Childhood
- Technology, Educational
- Technology-based Assessment
- The Bologna Process
- The Regulation of Standards in Higher Education
- Theories of Educational Leadership
- Three Conceptions of Literacy: Media, Narrative, and Gamin...
- Tracking and Detracking
- Traditions of Quality Improvement in Education
- Transformative Learning
- Transitions in Early Childhood Education
- Tribally Controlled Colleges and Universities in the Unite...
- Understanding the Psycho-Social Dimensions of Schools and ...
- University Faculty Roles and Responsibilities in the Unite...
- Value of Higher Education for Students and Other Stakehold...
- Virtual Learning Environments
- Vocational and Technical Education
- Wellness and Well-Being in Education
- Women's and Gender Studies
- Young Children and Spirituality
- Young Children's Learning Dispositions
- Young Children's Working Theories
- Privacy Policy
- Cookie Policy
- Legal Notice
- Accessibility
Powered by:
- [66.249.64.20|91.193.111.216]
- 91.193.111.216
Advertisement
Data science for analyzing and improving educational processes
- Published: 29 October 2021
- Volume 33 , pages 545–550, ( 2021 )
Cite this article
- Shadi Aljawarneh 1 &
- Juan A. Lara 2
5792 Accesses
14 Citations
1 Altmetric
Explore all metrics
In this full review paper, the recent emerging trends in Educational Data Science have been reviewed and explored to address the recent topics and contributions in the era of Smart Education. This includes a set of rigorously reviewed world-class manuscripts addressing and detailing state-of-the-art, frameworks and techniques research projects in the area of Data Science applied to Education, using different approaches such as Information Fusion, Soft Computing, Machine Learning, and Internet of Things, among others. Based on this systematic review, we have put some recommendations and suggestions for researchers, practitioners and scholars to improve their research quality in this area.
Avoid common mistakes on your manuscript.
Introduction to the domain of research
The term Data Science (DS) refers to an interdisciplinary field that involves a series of methods, processes and systems, with the aim of extracting knowledge from data. DS, which is a discipline very related to Computing, has proved to be of great application in very different domains, particularly Education (Klašnja-Milićević et al., 2017 ). In Educational environment, there are lots of learning-related processes involved, and great amounts of potential rich data are generated in educational institutions continuously. In order to extract knowledge from those data for a better understanding or learning-related processes, the use of DS approach seems to be useful and necessary (Mitrofanova et al., 2019 ).
The application of DS in the field of Education may result of great interests for involved stakeholders (students, instructors, institutions, …) since the extracted knowledge from educational data would be useful to deal with educational problems such as students’ performance improvement, high churning rates in educational institutions, learning delays, and so on. There are a series of disciplines related to Educational Data Science, such as Educational Data Mining and Learning Analytics (Romero & Ventura, 2020 ), and all of them are of importance for this special issue.
In this introductory paper, Sect. 2 includes the summaries of the selected papers. Section 3 includes a set of recommendations for researchers, practitioners and scholars to improve their research quality in this area. In Sect. 4 , the conclusions have been dawn.
Related work: the selected papers
The purpose of this special issue is to present original contributions of studies on the application of DS techniques in order to extract knowledge of interest for educational stakeholders as long as the analysed data represent a particular educational process and the knowledge extracted is used to improve that process in some way. We have considered papers that include discussions of the implementation of software and/or hardware approaches that also focus on the implications for the improvement of any learning process. Priority has been be given to papers that demonstrate a strong grounding in learning theory and/or rigorous educational research design. We have considered studies focused on tertiary and further education of any type (e-learning, blended and traditional education). All accepted works include an exhaustive validation and include extraordinarily new ideas in the area.
The special issue includes 10 papers, which have been subject to a rigorous peer-review process. Each paper has been reviewed by three independent experts. The rest of this section includes a summary of the selected papers.
The research presented in “Multilayered-Quality Education Ecosystem (MQEE): An Intelligent Education Modal for Sustainable Quality Education”, by Verma et al., intends to unfold some hidden parameters that are affecting the quality education ecosystem (QEE). Academic loafing, unawareness, non-participation, dissatisfaction, and incomprehensibility are the main parameters under this study. A set of hypothesis and surveys are exhibited to study the behaviour of these parameters on quality education at the institution level. The bidirectional weighted sum method is deployed for precise and accurate results regarding boundary value analysis of the survey. The association between parameters understudy and quality education is illustrated with correlation and scatter diagrams. Academic loafing, the hidden and unintended rudiment that affects the QEE is also defined, intended and explored in this work.
In the paper “Improving prediction of students’ performance in Intelligent Tutoring Systems using attribute selection and ensembles of different multimodal data sources”, Chango et al. intend to predict university students’ learning performance using different sources of performance and multimodal data from an Intelligent Tutoring System. They collected and preprocessed data from 40 students from different multimodal sources: learning strategies from system logs, emotions from videos of facial expressions, allocation and fixations of attention from eye tracking, and performance on post-tests of domain knowledge. Their objective is to test whether the prediction could be improved by using attribute selection and classification ensembles.
In “Automated Text Detection from Big Data Scene Videos in Higher Education”, Manasa et al. employed a novel approach to clean up the video frames to feed a neural network model based on region proposal network (RPN) with convolutional neural networks by finding appropriate anchor ratios to extract the text candidates. The trained their model with extracted frames to predict for the test videos. The proposed method is evaluated on ICDAR Video text benchmark datasets and few publicly available test datasets to achieve high recall.
In the paper “Improve teaching with modalities and collaborative groups in an LMS: an analysis of monitoring using visualisation techniques”, by Sáiz-Manzanares et al., the main objective is to test the effectiveness of three teaching modalities (all using Online Project-based Learning -OPBL- and Flipped Classroom experiences and differing in the use of virtual laboratories and Intelligent Personal Assistant -IPA-) on Moodle behaviour and student performance taking into account the covariate "collaborative group". Both quantitative and qualitative research methods were used. With regard to the quantitative analysis, differences were found in student behaviour in Moodle and in learning outcomes, with respect to teaching modalities that included virtual laboratories. Similarly, the qualitative study also analysed the behaviour patterns found in each collaborative group in the three teaching modalities studied.
The study titled “Fuzzy-based Active Learning for Predicting Student Academic Performance using autoML: a step-wise approach”, by Tsiakmaki et al., introduces a fuzzy-based active learning method for predicting students' academic performance which combines, in a modular way, autoML practices. A lot of experiments were carried out, revealing the efficiency of the proposed method for the accurate prediction of students at risk of failure. These insights may have the potential to support the learning experience and be useful the wider science of learning.
In the paper “Peer Assessment Using Soft Computing Techniques”, by Pinargote-Ortega et al., a peer assessment scenario was applied at the Universidad Técnica de Manabí (Ecuador). Students and professors evaluate some works through rubrics, assign a numerical score, and textual feedback grounding the reasons why such numerical score is determined. Interesting scenario to detect inaccuracy between both assessments. It is proposed a model with soft computing techniques to detect inaccuracy and reduce the workload of the professor in the correction process.
In “A Novel Automated Essay Scoring Approach for Reliable Higher Educational Assessments”, Beseiso et al. present a transformer-based neural network model for improved Automatic Essay Scoring performance using Bi-LSTM (Bidirectional Long Short-Term Memory) and RoBERTa language model based on Kaggle’s ASAP (Automated Student Assessment Prize) dataset. The proposed model uses Bi-LSTM model over pre-trained RoBERTa language model to address the coherency issue in essays that is ignored by traditional essay scoring methods, including traditional Natural Language Processing pipelines, deep learning-based methods, a mixture of both. The comparison of the experimental results on essay scoring with human raters concludes that the proposed model outperforms the existing methods in essay scoring in terms of QWK (Quadratic Weighted Kappa) score.
The main goal of the research presented in the paper “Personalized training model for organizing blended and lifelong distance learning courses and its effectiveness in Higher Education”, by Bekmanova et al., is to improve the personification of learning in higher education. The proposed flexible model for organizing blended and distance learning in higher education involves the creation of an individual learning path through testing students before the start of training. Based on the learning outcomes, the student is credited to the learning path. The training path consists of mandatory and additional modules for training; additional modules can be skipped after successfully passing the test, without studying these modules. The paper examines the composition of intelligent learning systems: student model, learning model and interface model.
In the paper “IoT Text Analytics in Smart Education and Beyond”, Mohammed et al. highlight the main components of IoT analytics, along with a comprehensive background of text analytics used techniques and applications. This paper provides a comprehensive survey and comparison of the leveraged IoT Text Analytics models and methods in Smart Education and many other applications.
Finally, in “A Framework to Capture the Dependency between prerequisite and Advanced Courses in Higher Education”, Hriez & Al-Naymat propose a new graph mining algorithm combined with statistical analysis to reveal the dependency relationships between Course Learning Outcomes (CLOs) of prerequisite and advanced courses. In addition, a new model is built to predict students’ performance in the advanced courses based on prerequisites. The evaluation proves that the proposed algorithm is accurate, efficient, effective, and applicable to real-world graphs more than the traditional algorithm.
Discussions and recommendations
A number of recommendations have been suggested to improve the research in this field as follows:
The papers selected for inclusion in this special issue have described a number of data science techniques for extracting knowledge for educational data. However, the knowledge extracted is only applicable to the problem addressed. It is desirable to obtain general models that can be applied in other scenarios (López-Zambrano et al., 2021 ).
Most research is focused on analyzing only one source of educational data. However, in current smart classrooms, a lot of different multi-source and multi-modal data are recorded and it can be very interesting to fuse those data in order to obtain richer and more accurate models.
Many DS approaches generate models that are hard to interpret, in spite of the fact that they can obtain very accurate results. However, interpretability is a requirement in Education sometimes, since it helps understand the learning processes and, therefore, improve them by interventions.
Current educational models are designed on the premise of ubiquity, particularly in the event of emergencies such as the one caused by the Covid-19 pandemic (Maatuk et al., 2021 ). In this scenario, the student needs to be able to self-regulate his or her learning, which is hard sometimes. It is very important to count on tools for personalized learning that adapt to each student depending on his or her emotions at a certain moment. The use of virtual affective agents is a promising line nowadays.
Conclusions
In this special issue, 10 selected papers have been included that present important advancements in the area of Educational Data Science. The selected papers include interesting studies about the development of this area, works about promising existing technologies and outstanding research about theories and methods that will play a crucial role in the future of this discipline.
As guest editors, we are aware of the fact that this issue cannot completely cover all the advancements in this area, but we expect that this special issue can stimulate further research in the domain of Educational Data Science.
Klašnja-Milićević, A., Ivanović, M., & Budimac, Z. (2017). Data science in education: Big data and learning analytics. Computer Applications in Engineering Education, 25 , 1066–1078. https://doi.org/10.1002/cae.21844
Article Google Scholar
López-Zambrano, J., Lara, J. A., & Romero, C. (2021). Improving the portability of predicting students’ performance models by using ontologies. Journal of Computing in Higher Education . https://doi.org/10.1007/s12528-021-09273-3
Maatuk, A. M., Elberkawi, E. K., Aljawarneh, S., Rashaideh, H., & Alharbi, H. (2021). The COVID-19 pandemic and E-learning: Challenges and opportunities from the perspective of students and instructors. Journal of Computing in Higher Education . https://doi.org/10.1007/s12528-021-09274-2
Mitrofanova, Y. S., Sherstobitova, A. A., & Filippova, O. A. (2019). Modeling smart learning processes based on educational data mining tools. In V. Uskov, R. Howlett, & L. Jain (Eds.), Smart Education and e-Learning 2019. Smart Innovation, Systems and Technologies. (Vol. 144). Springer. https://doi.org/10.1007/978-981-13-8260-4_49
Chapter Google Scholar
Romero, C., & Ventura, S. (2020). Educational data mining and learning analytics: An updated survey. Wires Data Mining and Knowledge Discovery, 10 , e1355. https://doi.org/10.1002/widm.1355
Download references
Acknowledgements
We would like to thank the referees who have reviewed the papers for providing a valuable feedback to authors. We would also like to thank the authors for their manuscripts that represent an important contribution to the existing knowledge in the area. Finally, we would like to thank Professor Stephanie L. Moore, Editor-in-Chief, and editorial assistants of Journal of Computing in Higher Education for their support during the preparation of this issue.
Author information
Authors and affiliations.
Software Engineering Department, Jordan University of Science and Technology, Irbid, Jordan
Shadi Aljawarneh
Escuela de Ciencias Técnicas e Ingeniería, Madrid Open University, UDIMA, Ctra. de la Coruña, km 38.500 – Vía de Servicio, 15 - 28400, Collado Villalba, Madrid, Spain
Juan A. Lara
You can also search for this author in PubMed Google Scholar
Corresponding author
Correspondence to Shadi Aljawarneh .
Additional information
Publisher's note.
Springer Nature remains neutral with regard to jurisdictional claims in published maps and institutional affiliations.
Rights and permissions
Reprints and permissions
About this article
Aljawarneh, S., Lara, J.A. Data science for analyzing and improving educational processes. J Comput High Educ 33 , 545–550 (2021). https://doi.org/10.1007/s12528-021-09299-7
Download citation
Accepted : 20 October 2021
Published : 29 October 2021
Issue Date : December 2021
DOI : https://doi.org/10.1007/s12528-021-09299-7
Share this article
Anyone you share the following link with will be able to read this content:
Sorry, a shareable link is not currently available for this article.
Provided by the Springer Nature SharedIt content-sharing initiative
- Educational data science
- Learning analytics
- Educational data mining
- Educational processes
- Find a journal
- Publish with us
- Track your research
- Review Article
- Open access
- Published: 22 June 2020
Teaching analytics, value and tools for teacher data literacy: a systematic and tripartite approach
- Ifeanyi Glory Ndukwe 1 &
- Ben Kei Daniel 1
International Journal of Educational Technology in Higher Education volume 17 , Article number: 22 ( 2020 ) Cite this article
26k Accesses
40 Citations
14 Altmetric
Metrics details
Teaching Analytics (TA) is a new theoretical approach, which combines teaching expertise, visual analytics and design-based research to support teacher’s diagnostic pedagogical ability to use data and evidence to improve the quality of teaching. TA is now gaining prominence because it offers enormous opportunities to the teachers. It also identifies optimal ways in which teaching performance can be enhanced. Further, TA provides a platform for teachers to use data to reflect on teaching outcome. The outcome of TA can be used to engage teachers in a meaningful dialogue to improve the quality of teaching. Arguably, teachers need to develop their teacher data literacy and data inquiry skills to learn about teaching challenges. These skills are dependent on understanding the connection between TA, LA and Learning Design (LD). Additionally, they need to understand how choices in particular pedagogues and the LD can enhance their teaching experience. In other words, teachers need to equip themselves with the knowledge necessary to understand the complexity of teaching and the learning environment. Providing teachers access to analytics associated with their teaching practice and learning outcome can improve the quality of teaching practice. This research aims to explore current TA related discussions in the literature, to provide a generic conception of the meaning and value of TA. The review was intended to inform the establishment of a framework describing the various aspects of TA and to develop a model that can enable us to gain more insights into how TA can help teachers improve teaching practices and learning outcome. The Tripartite model was adopted to carry out a comprehensive, systematic and critical analysis of the literature of TA. To understand the current state-of-the-art relating to TA, and the implications to the future, we reviewed published articles from the year 2012 to 2019. The results of this review have led to the development of a conceptual framework for TA and established the boundaries between TA and LA. From the analysis the literature, we proposed a Teaching Outcome Model (TOM) as a theoretical lens to guide teachers and researchers to engage with data relating to teaching activities, to improve the quality of teaching.
Introduction
Educational institutions today are operating in an information era, where machines automatically generate data rather than manually; hence, the emergence of big data in education ( Daniel 2015 ). The phenomenon of analytics seeks to acquire insightful information from data that ordinarily would not be visible by the ordinary eyes, except with the application of state-of-the-art models and methods to reveal hidden patterns and relationships in data. Analytics plays a vital role in reforming the educational sector to catch up with the fast pace at which data is generated, and the extent to which such data can be used to transform our institutions effectively. For example, with the extensive use of online and blended learning platforms, the application of analytics will enable educators at all levels to gain new insights into how people learn and how teachers can teach better. However, the current discourses on the use of analytics in Higher Education (HE) are focused on the enormous opportunities analytics offer to various stakeholders; including learners, teachers, researchers and administrators.
In the last decade, extensive literature has proposed two weaves of analytics to support learning and improve educational outcomes, operations and processes. The first form of Business Intelligence introduced in the educational industry is Academic Analytics (AA). AA describes data collected on the performance of academic programmes to inform policy. Then, Learning Analytics (LA), emerged as the second weave of analytics, and it is one of the fastest-growing areas of research within the broader use of analytics in the context of education. LA is defined as the "measurement, collection, analysis and reporting of data about the learner and their learning contexts for understanding and optimising learning and the environments in which it occurs" ( Elias 2011 ). LA was introduced to attend to teaching performance and learning outcome ( Anderson 2003 ; Macfadyen and Dawson 2012 ). Typical research areas in LA, include student retention, predicting students at-risk, personalised learning which in turn are highly student-driven ( Beer et al. 2009 ; Leitner et al. 2017 ; Pascual-Miguel et al. 2011 ; Ramos and Yudko 2008 ). For instance, Griffiths ( Griffiths 2017 ), employed LA to monitor students’ engagements and behavioural patterns on a computer-supported collaborative learning environment to predict at-risk students. Similarly, Rienties et al. ( Rienties et al. 2016 ) looked at LA approaches in their capacity to enhance the learner’s retention, engagement and satisfaction. However, in the last decade, LA research has focused mostly on the learner and data collections, based on digital data traces from Learning Management Systems (LMS) ( Ferguson 2012 ), not the physical classroom.
Teaching Analytics (TA) is a new theoretical approach that combines teaching expertise, visual analytics and design-based research, to support the teacher with diagnostic and analytic pedagogical ability to improve the quality of teaching. Though it is a new phenomenon, TA is now gaining prominence because it offers enormous opportunities to the teachers.
Research on TA pays special attention to teacher professional practice, offering data literacy and visual analytics tools and methods ( Sergis et al. 2017 ). Hence, TA is the collection and use of data related to teaching and learning activities and environments to inform teaching practice and to attain specific learning outcomes. Some authors have combined the LA, and TA approaches into Teaching and Learning Analytics (TLA) ( Sergis and Sampson 2017 ; Sergis and Sampson 2016 ). All these demonstrate the rising interest in collecting evidence from educational settings for awareness, reflection, or decision making, among other purposes. However, the most frequent data that have been collected and analysed about TA focus on the students (e.g., different discussion and learning activities and some sensor data such as eye-tracking, position or physical actions) ( Sergis and Sampson 2017 ), rather than monitoring teacher activities. Providing teachers access to analytics of their teaching, and how they can effectively use such analytics to improve their teaching process is a critical endeavour. Also, other human-mediated data gathering in the form of student feedback, self and peer observations or teacher diaries can be employed to enrich TA further. For instance, visual representations such as dashboards can be used to present teaching data to help teachers reflect and make appropriate decisions to inform the quality of teaching. In other words, TA can be regarded as a reconceptualisation of LA for teachers to improve teaching performance and learning outcome. The concept of TA is central to the growing data-rich technology-enhanced learning and teaching environment ( Flavin 2017 ; Saye and Brush 2007 ). Further, it provides teachers with the opportunity to engage in data-informed pedagogical improvement.
While LA is undeniably an essential area of research in educational technology and the learning sciences, automatically extracted data from an educational platform mainly provide an overview of student activities, and participation. Nevertheless, it hardly indicates the role of the teacher in these activities, or may not otherwise be relevant to teachers’ individual needs (for Teaching Professional Development (TPD) or improvement of their classroom practice). Many teachers generally lack adequate data literacy skills ( Sun et al. 2016 ). Teacher data literacy skill and teacher inquiry skill using data are the foundational concepts underpinning TA ( Kaser and Halbert 2014 ). The development of these two skills is dependent on understanding the connection between TA, LA and Learning Design (LD). In other words, teachers need to equip themselves with knowledge through interaction with sophisticated data structures and analytics. Hence, TA is critical to improving teachers’ low efficacy towards educational data.
Additionally, technology has expanded the horizon of analytics to various forms of educational settings. As such, the educational research landscape needs efficient tools for collecting data and analyzing data, which in turn requires explicit guidance on how to use the findings to inform teaching and learning ( McKenney and Mor 2015 ). Increasing the possibilities for teachers to engage with data to assess what works for the students and courses they teach is instrumental to quality ( Van Harmelen and Workman 2012 ). TA provides optimal ways of performing the analysis of data obtained from teaching activities and the environment in which instruction occurs. Hence, more research is required to explore how teachers can engage with data associated with teaching to encourage teacher reflection, improve the quality of teaching, and provide useful insights into ways teachers could be supported to interact with teaching data effectively. However, it is also essential to be aware that there are critical challenges associated with data collection. Moreover, designing the information flow that facilitates evidence-based decision-making requires addressing issues such as the potential risk of bias; ethical and privacy concerns; inadequate knowledge of how to engage with analytics effectively.
To ensure that instructional design and learning support is evidence-based, it is essential to empower teachers with the necessary knowledge of analytics and data literacy. The lack of such knowledge can lead to poor interpretation of analytics, which in turn can lead to ill-informed decisions that can significantly affect students; creating more inequalities in access to learning opportunities and support regimes. Teacher data literacy refers to a teachers’ ability to effectively engage with data and analytics to make better pedagogical decisions.
The primary outcome of TA is to guide educational researchers to develop better strategies to support the development of teachers’ data literacy skills and knowledge. However, for teachers to embrace data-driven approaches to learning design, there is a need to implement bottom-up approaches that include teachers as main stakeholders of a data literacy project, rather than end-users of data.
The purpose of this research is to explore the current discusses in the literature relating to TA. A vital goal of the review was to extend our understanding of conceptions and value of TA. Secondly, we want to contextualise the notion of TA and develop various concepts around TA to establish a framework that describes multiple aspects of TA. Thirdly, to examine different data collections/sources, machine learning algorithms, visualisations and actions associated with TA. The intended outcome is to develop a model that would provide a guide for the teacher to improve teaching practice and ultimately enhance learning outcomes.
The research employed a systematic and critical analysis of articles published from the year 2012 to 2019. A total of 58 publications were initially identified and compiled from the Scopus database. After analysing the search results, 31 papers were selected for review. This review examined research relating to the utilisation of analytics associated with teaching and teacher activities and provided conceptual clarity on TA. We found that the literature relating to conception, and optimisation of TA is sporadic and scare, as such the notion of TA is theoretically underdeveloped.
Methods and procedures
This research used the Tripartite model ( Daniel and Harland 2017 ), illustrated in Fig. 1 , to guide the systematic literature review. The Tripartite model draws from systematic review approaches such as the Cochrane, widely used in the analyses of rigorous studies, to provide the best evidence. Moreover, the Tripartite model offers a comprehensive view and presentation of the reports. The model composes of three fundamental components; descriptive (providing a summary of the literature), synthesis (logically categorising the research based on related ideas, connections and rationales), and critique (criticising the novel, providing evidence to support, discard or offer new ideas about the literature). Each of these phases is detailed fully in the following sections.
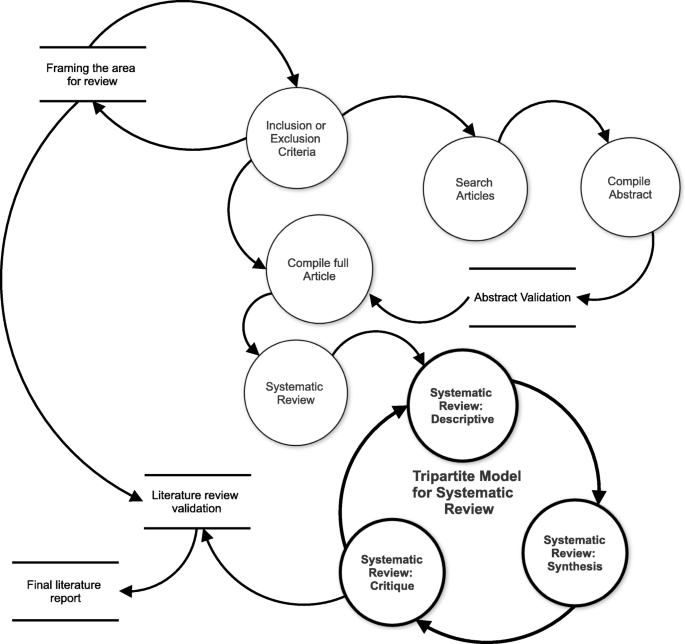
Tripartite Model. The Tripartite Model: A Systematic Literature Review Process ( Daniel and Harland 2017 )
To provide clarity; the review first focused on describing how TA is conceptualised and utilised. Followed by the synthesis of the literature on the various tools used to harvest, analyse and present teaching-related data to the teachers. Then the critique of the research which led to the development of a conceptual framework describing various aspects of TA. Finally, this paper proposes a Teaching Outcome Model (TOM). TOM is intended to offer teachers help on how to engage and reflect on teaching data.
TOM is a TA life cycle which starts with the data collection stage; where the focus is on teaching data. Then the data analysis stage; the application of different Machine Learning (ML) techniques to the data to discover hidden patterns. Subsequently, the data visualisation stage, where data presentation is carried out in the form of a Teaching Analytics Dashboard (TAD) for the teacher. This phase is where the insight generation, critical thinking and teacher reflection are carried out. Finally, the action phase, this is where actions are implemented by teachers to improve teaching practice. Some of these actions include improving the LD, changing teaching method, providing appropriate feedback and assessment or even carrying out more research. This research aims to inform the future work in the advancement of TA research field.
Framing research area for review
As stated in the introduction, understanding current research on TA can be used to provide teachers with strategies that can help them utilise various forms of data to optimise teaching performance and outcome. Framing the review was guided by some questions and proposed answers to address those questions (see Table 1 )
Inclusion and exclusion criteria
The current review started with searching through the Scopus database using the SciVal visualisation and analytical tool. The rationale for choosing the Scopus database is that it contains the largest abstract and citation database of peer-reviewed research literature with diverse titles from publishers worldwide. Hence, it is only conceivable to search for and find a meaningful balance of the published content in the area of TA. Also, the review included peer-reviewed journals and conference proceedings. We excluded other documents and source types, such as book series, books, editorials, trade publications on the understanding that such sources might lack research on TA. Also, this review excluded articles published in other languages other than English.
Search strategy
This review used several keywords and combinations to search on terms related to TA. For instance: ’Teaching Analytics’ AND ’Learning Analytics’ OR ’Teacher Inquiry’ OR ’Data Literacy’ OR ’Learning Design’ OR ’Computer-Supported Collaborative Learning’ OR ’Open Learner Model’ OR ’Visualisation’ OR ’Learning Management System’ OR ’Intelligent Tutoring System’ OR ’Student Evaluation on Teaching’ OR ’Student Ratings’.
This review searched articles published between 2012 to 2019. The initial stage of the literature search yielded 58 papers. After the subsequent screening of previous works and removing duplicates and titles that did not relate to the area of research, 47 articles remained. As such, a total of 36 studies continued for full-text review. Figure 2 , shows the process of finalising the previous studies of this review.
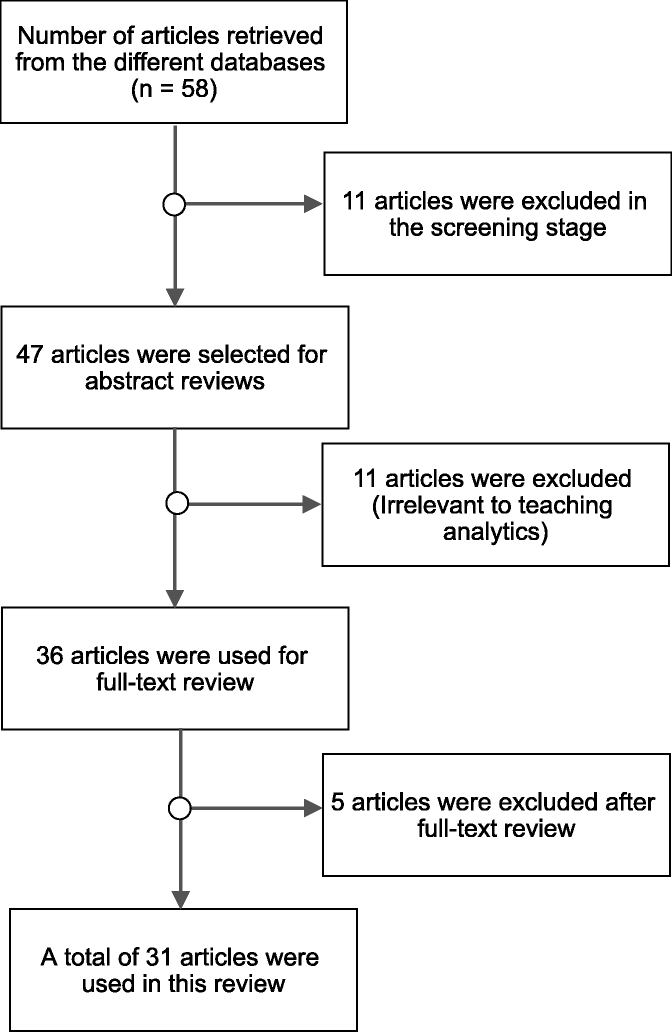
Inclusion Exclusion Criteria Flowchart. The selection of previous studies
Compiling the abstracts and the full articles
The review ensured that the articles identified for review were both empirical and conceptual papers. The relevance of each article was affirmed by requiring that chosen papers contained various vital phrases all through the paper, as well as, title, abstract, keywords and, afterwards, the entire essay. In essence, were reviewed giving particular cognisance and specific consideration to those section(s) that expressly related to the field of TA. In doing as such, to extract essential points of view on definitions, data sources, tools and technologies associated with analytics for the teachers. Also, this review disregarded papers that did not, in any way, relate to analytics in the context of the teachers. Finally, 31 articles sufficed for this review.
Systematic review: descriptive
Several studies have demonstrated that TA is an important area of inquiry ( Flanders 1970 ; Gorham 1988 ; Pennings et al. 2014 ; Schempp et al. 2004 ), that enables researchers to explore analytics associated with teaching process systematically. Such analytics focus on data related to the teachers, students, subjects taught and teaching outcomes. The ultimate goal of TA is to improve professional teaching practice ( Huang 2001 ; Sergis et al. 2017 ). However, there is no consensus on what constitutes TA. Several studies suggest that TA is an approach used to analyse teaching activities ( Barmaki and Hughes 2015 ; Gauthier 2013 ; KU et al. 2018 ; Saar et al. 2017 ), including how teachers deliver lectures to students, tools usage pattern, or dialogue. While various other studies recognise TA as the ability to applying analytical methods to improve teacher awareness of student activities for appropriate intervention ( Ginon et al. 2016 ; Michos and Hernández Leo 2016 ; Pantazos et al. 2013 ; Taniguchi et al. 2017 ; Vatrapu et al. 2013 ). A hand full of others indicate TA as analytics that combines both teachers and students activities ( Chounta et al. 2016 ; Pantazos and Vatrapu 2016 ; Prieto et al. 2016 ; Suehiro et al. 2017 ). Hence, it is particularly problematic and challenging to carry out a systematic study in the area of analytics for the teachers to improve teaching practice, since there is no shared understanding of what constitutes analytics and how best to approach TA.
Researchers have used various tools to automatically harvest important episodes of interactive teacher and student behaviour during teaching, for teacher reflection. For instance, KU et al. ( 2018 ), utilised instruments such as; Interactive Whiteboard (IWB), Document Camera (DC), and Interactive Response System (IRS) to collect classroom instructional data during instruction. Similarly, Vatrapu et al. ( 2013 ) employed eye-tracking tools to capture eye-gaze data on various visual representations. Thomas ( 2018 ) also extracted multimodal features from both the speaker and the students’ audio-video data, using digital devices such as cameras and high-definition cameras. Data collected from some of these tools not only provide academics with real-time data but also attract more details about teaching and learning than the teacher may realise. However, the cost of using such digital tools for large-scale verification is high, and cheaper alternatives are sort after. For instance, Suehiro et al. ( 2017 ) proposed a novel approach of using e-books to extract teaching activity logs in a face-to-face class efficiently.
Vatrapu ( 2012 ) considers TA as a subset of LA dedicated to supporting teachers to understand the learning and teaching process. However, this definition does not recognise that both the learning and teaching processes are intertwined. Also, most of the research in LA collects data about the student learning or behaviour, to provide feedback to the teacher ( Vatrapu et al. 2013 ; Ginon et al. 2016 ; Goggins et al. 2016 ; Shen et al. 2018 ; Suehiro et al. 2017 ), see, for example, the iKlassroom conceptual proposal by Vatrapu et al. ( 2013 ), which highlights a map of the classroom to help contextualise real-time data about the learners in a lecture. Although, a few research draw attention to the analysis of teacher-gathering and teaching practice artefacts, such as lesson plans. Xu and Recker ( 2012 ) examined teachers tool usage patterns. Similarly, Gauthier ( 2013 ) extracted the analysis of the reasoning behind the expert teacher and used such data to improve the quality of teaching.
Multimodal analytics is an emergent trend used to complement available digital trace with data captured from the physical world ( Prieto et al. 2017 ). Isolated examples include the smart school multimodal dataset conceptual future proposal by Prieto et al. ( 2017 ), which features a plan of implementing a smart classroom to help contextualise real-time data about both the teachers and learners in a lecture. Another example, Prieto et al. ( 2016 ), explored the automatic extraction of orchestration graphs from a multimodal dataset gathered from only one teacher, classroom space, and a single instructional design. Results showed that ML techniques could achieve reasonable accuracy towards automated characterisation in teaching activities. Furthermore, Prieto et al. ( 2018 ) applied more advanced ML techniques to an extended version of the previous dataset to explore the different relationships that exist between datasets captured by multiple sources.
Previous studies have shown that teachers want to address common issues such as improving their TPD and making students learn effectively ( Charleer et al. 2013 ; Dana and Yendol-Hoppey 2019 ; Pennings et al. 2014 ). Reflection on teaching practice plays an essential role in helping teachers address these issues during the process of TPD ( Saric and Steh 2017 ; Verbert et al. 2013 ). More specifically, reflecting on personal teaching practice provides opportunities for teachers to re-examine what they have performed in their classes ( Loughran 2002 ; Mansfield 2019 ; Osterman and Kottkamp 1993 ). Which, in turn, helps them gain an in-depth understanding of their teaching practice, and thus improve their TPD. For instance, Gauthier ( 2013 ), used a visual teach-aloud method to help teaching practitioners reflect and gain insight into their teaching practices. Similarly, Saar et al. ( 2017 ) talked about a self-reflection as a way to improve teaching practice. Lecturers can record and observe their classroom activities, analyse their teaching and make informed decisions about any necessary changes in their teaching method.
The network analysis approach is another promising field of teacher inquiry, especially if combined with systematic, effective qualitative research methods ( Goggins et al. 2016 ). However, researchers and teacher who wish to utilise social network analysis must be specific about what inquiry they want to achieve. Such queries must then be checked and validated against a particular ontology for analytics ( Goggins 2012 ). Goggins et al. ( 2016 ), for example, aimed at developing an awareness of the types of analytics that could help teachers in Massive Open Online Courses (MOOCs) participate and collaborate with student groups, through making more informed decisions about which groups need help, and which do not. Network theory offers a particularly useful framework for understanding how individuals and groups respond to each other as they evolve. Study of the Social Network (SNA) is the approach used by researchers to direct analytical studies informed by network theory. SNA has many specific forms, each told by graph theory, probability theory, and algebraic modelling to various degrees. There are gaps in our understanding of the link between analytics and pedagogy. For example, which unique approaches to incorporating research methods for qualitative and network analysis would produce useful information for teachers in MOOCs? A host of previous work suggests a reasonable path to scaling analytics for MOOCs will involve providing helpful TA perspectives ( Goggins 2012 ; Goggins et al. 2016 ; Vatrapu et al. 2012 ).
Teacher facilitation is considered a challenging and critical aspect of active learning ( Fischer et al. 2014 ). Both educational researchers and practitioners have paid particular attention to this process, using different data gathering and visualisation methods, such as classroom observation, student feedback, audio and video recordings, or teacher self-reflection. TA enables teachers to perform analytics through visual representations to enhance teachers’ experience ( Vatrapu et al. 2011 ). As in a pedagogical environment, professionals have to monitor several data such as questions, mood, ratings, or progress. Hence, dashboards have become an essential factor in improving and conducting successful teaching. Dashboards are visualisation tools enable teachers to monitor and observe teaching practice to enhance teacher self-reflection ( Yigitbasioglu and Velcu 2012 ). While a TAD is a category of dashboard meant for teachers and holds a unique role and value [62]. First, TAD could allow teachers to access students learning in an almost real-time and scalable manner ( Mor et al. 2015 ), consequently, enabling teachers to improve their self-knowledge by monitoring and observing students activities. TAD assists the teachers in obtaining an overview of the whole classroom as well as drill down into details about individual and groups of students to identify student competencies, strengths and weaknesses. For instance, Pantazos and Vatrapu ( 2016 ) described TAD for repertory grid data to enable teachers to conduct systematic visual analytics of classroom learning data for formative assessment purposes. Second, TAD also allows for tracking on teacher self-activities ( van Leeuwen et al. 2019 ), as well as students feedback about their teaching practice. For example,Barmaki and Hughes ( 2015 ) explored a TAD that provides automated real-time feedback based on speakers posture, to support teachers practice classroom management and content delivery skills. It is a pedagogical point that dashboards can motivate teachers to reflect on teaching activities, help them improve teaching practice and learning outcome ( 2016 ). The literature has extensively described extensively, different teaching dashboards. For instance, Dix and Leavesley ( 2015 ), broadly discussed the idea of TAD and how they can represent visual tools for academics to interface with learning analytics and other academic life. Some of these academic lives may include schedules such as when preparing for class or updating materials, or meeting times such as meeting appointments with individual or collective group of students. Similarly, Vatrapu et al. ( 2013 ) explored TAD using visual analytics techniques to allow teachers to conduct a joint analysis of students personal constructs and ratings of domain concepts from the repertory grids for formative assessment application.
Systematic review: synthesis
In this second part of the review process, we extracted selected ideas from previous studies. Then group them based on data sources, analytical methods used, types of visualisations performed and actions.
Data sources and tools
Several studies have used custom software and online applications such as employing LMS and MOOCs to collect online classroom activities ( Goggins et al. 2016 ; KU et al. 2018 ; Libbrecht et al. 2013 ; Müller et al. 2016 ; Shen et al. 2018 ; Suehiro et al. 2017 ; Vatrapu et al. 2013 ; Xu and Recker 2012 ). Others have used modern devices including eye-tracker, portable electroencephalogram (EEG), gyroscope, accelerometer and smartphones ( Prieto et al. 2016 ; Prieto et al. 2018 ; Saar et al. 2017 ; Saar et al. 2018 ; Vatrapu et al. 2013 ), and conventional instruments such as video and voice recorders ( Barmaki and Hughes 2015 ; Gauthier 2013 ; Thomas 2018 ), to record classroom activities. However, some authors have pointed out several issues with modern devices such as expensive equipment, high human resource and ethical concerns ( KU et al. 2018 ; Prieto et al. 2017 ; Prieto et al. 2016 ; Suehiro et al. 2017 ).
In particular, one study by Chounta et al. ( 2016 ) recorded classroom activities using humans to code tutor-student dialogue manually. However, they acknowledged that manual coding of lecture activities is complicated and cumbersome. Some authors also subscribe to this school of thought and have attempted to address this issue by applying Artificial Intelligence (AI) techniques to automate and scale the coding process to ensure quality in all platforms ( Prieto et al. 2018 ; Saar et al. 2017 ; Thomas 2018 ). Others have proposed re-designing TA process to automate the process of data collection as well as making the teacher autonomous in collecting data about their teaching ( Saar et al. 2018 ; Shen et al. 2018 ). Including using technology that is easy to set up, effortless to use, does not require much preparation and at the same time, not interrupting the flow of the class. In this way, they would not require researcher assistance or outside human observers. Table 2 , summarises the various data sources as well as tools that are used to harvest teaching data with regards to TA.
The collection of evidence from both online and real classroom practice is significant both for educational research and TPD. LA deals mostly with data captured from online and blended learning platforms (e.g., log data, social network and text data). Hence, LA provides teachers with data to monitor and observe students online class activities (e.g., discussion boards, assignment submission, email communications, wiki activities and progress). However, LA neglects to capture physical occurrences of the classroom and do not always address individual teachers’ needs. TA requires more adaptable forms of classroom data collection (e.g., through video- recordings, sensor recording or by human observers) which are tedious, human capital intensive and costly. Other methods have been explored to balance the trade-off between data collected online, and data gathered from physical classroom settings by implementing alternative designs approach ( Saar et al. 2018 ; Suehiro et al. 2017 ).
Analysis methods
Multimodal analytics is the emergent trend that will complement readily available digital traces, with data captured from the physical world. Several articles in the literature have used multimodal approaches to analyse teaching processes in the physical world ( Prieto et al. 2016 ; Prieto et al. 2017 ; Prieto et al. 2018 ; Saar et al. 2017 ; Thomas 2018 ). In university settings, unobtrusive computer vision approaches to assess student attention from their facial features, and other behavioural signs have been applied ( Thomas 2018 ). Most of the studies that have ventured into multimodal analytics applied ML algorithms to their captured datasets to build models of the phenomena under investigation ( Prieto et al. 2016 ; Prieto et al. 2018 ). Apart from research areas that involve multimodal analytics, other areas of TA research have also applied in ML techniques such as teachers tool usage patterns ( Xu and Recker 2012 ), online e-books ( Suehiro et al. 2017 ), students written-notes ( Taniguchi et al. 2017 ). Table 3 outlines some of the ML techniques applied from previous literature in TA.
Visualisation methods
TA allows teachers to apply visual analytics and visualisation techniques to improve TPD. The most commonly used visualisation techniques in TA are statistical graphs such as line charts, bar charts, box plots, or scatter plots. Other visualisation techniques include SNA, spatial, timeline, static and real-time visualisations. An essential visualisation factor for TA is the number of users represented in a visualisation technique. Serving single or individual users allows the analyst to inspect the viewing behaviour of one participant. Visualising multiple or group users at the same time can allow one to find strategies of groups. However, these representations might suffer from visual clutter if too much data displays at the same time. Here, optimisation strategies, such as averaging or bundling of lines might be used, to achieve better results. Table 4 represents the visualisation techniques mostly used in TA.
Systematic review: critique
Student evaluation on teaching (set) data.
Although the literature has extensively reported various data sources used for TA, this study also draws attention to student feedback on teaching, as another form of data that originates from the classroom. The analytics of student feedback on teaching could support teacher reflection on teaching practice and add value to TA. Student feedback on teaching is also known as student ratings, or SET is a form of textual data. It can be described as a combination of both quantitative and qualitative data that express students opinions about particular areas of teaching performance. It has existed since the 1920s ( Marsh 1987 ; Remmers and Brandenburg 1927 ), and used as a form of teacher feedback. In addition to serving as a source of input for academic improvement ( Linse 2017 ), many universities also rely profoundly on SET for hiring, promoting and firing instructors ( Boring et al. 2016 ; Harland and Wald 2018 ).
Technological advancement has enabled institutions of Higher Education (HE) to administer course evaluations online, forgoing the traditional paper-and-pencil ( Adams and Umbach 2012 ). There has been much research around online teaching evaluations. Asare and Daniel ( 2017 ) investigated the factors influencing the rate at which students respond to online SET. While there is a verity of opinions as to the validity of SET as a measure of teaching performance, many teaching academics and administrators perceive that SET is still the primary measure that fills this gap ( Ducheva et al. 2013 ; Marlin Jr and Niss 1980 ). After all, who experiences teaching more directly than students? These evaluations generally consist of questions addressing the instructor’s teaching, the content and activities of the paper, and the students’ own learning experience, including assessment. However, it appears these schemes gather evaluation data and pass on the raw data to the instructors and administrators, stopping short of deriving value from the data to facilitate improvements in the instruction and the learning experiences. This measure is especially critical as some teachers might have the appropriate data literacy skills to interpret and use such data.
Further, there are countless debates over the validity of SET data ( Benton and Cashin 2014 ; MacNell et al. 2015 ). These debates have highlighted some shortcomings of student ratings of teaching in light of the quality of instruction rated ( Boring 2015 ; Braga et al. 2014 ). For Edström, what matters is how the individual teacher perceives an evaluation. It could be sufficient to undermine TPD, especially if the teachers think they are the subjects of audit ( Edström 2008 ). However, SET is today an integral part of the universities evaluation process ( Ducheva et al. 2013 ). Research has also shown that there is substantial room for utilising student ratings for improving teaching practice, including, improving the quality of instruction, learning outcomes, and teaching and learning experience ( Linse 2017 ; Subramanya 2014 ). This research aligns to the side of the argument that supports using SET for instructional improvements, to the enhancement of teaching experience.
Systematically, analytics of SET could provide valuable insights, which can lead to improving teaching performance. For instance, visualising SET can provide some way, a teacher can benchmark his performance over a while. Also, SET could provide evidence to claim for some level of data fusion in TA, as argued in the conceptualisation subsection of TA.
Transformational TA
The growing research into big data in education has led to renewed interests in the use of various forms of analytics ( Borgman et al. 2008 ; Butson and Daniel 2017 ; Choudhury et al. 2002 ). Analytics seeks to acquire insightful information from hidden patterns and relationships in data that ordinarily would not be visible by the natural eyes, except with the application of state-of-the-art models and methods. Big data analytics in HE provides lenses on students, teachers, administrators, programs, curriculum, procedures, and budgets ( Daniel 2015 ). Figure 3 illustrates the types of analytics that applies to TA to transform HE.
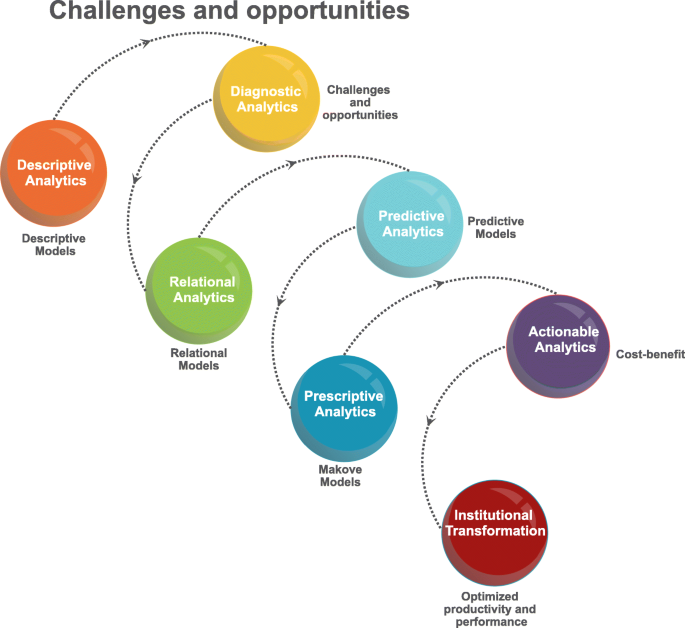
Types of analytics in higher education ( Daniel 2019 )
Descriptive Analytics Descriptive analytics aims to interpret historical data to understand better organisational changes that have occurred. They are used to answer the "What happened?" information regarding a regulatory process such as what are the failure rates in a particular program ( Olson and Lauhoff 2019 ). It applies simple statistical techniques such as mean, median, mode, standard deviation, variance, and frequency to model past behaviour ( Assunção et al. 2015 ; ur Rehman et al. 2016 ). Barmaki and Hughes ( 2015 ) carried out some descriptive analytics to know the mean view time, mean emotional activation, and area of interest analysis on the data generated from 27 stimulus images to investigate the notational, informational and emotional aspect of TA. Similarly, Michos and Hernández-Leo ( 2016 ) demonstrated how descriptive analytics could support teachers’ reflection and re-design their learning scenarios.
Diagnostic Analytics Diagnostic analytics is higher-level analytics that further diagnoses descriptive analytics ( Olson and Lauhoff 2019 ). They are used to answer the "Why it happened?". For example, a teacher may need to carry out diagnostic analytics to know why there is a high failure rate in a particular programme or why students rated a course so low for a specific year compared to the previous year. Diagnostic analytics uses some data mining techniques such as; data discovery, drill-down and correlations to further explore trends, patterns and behaviours ( Banerjee et al. 2013 ). Previous research has applied the repertory grid technique as a pedagogical method to support the teachers perform knowledge diagnostics of students about a specific topic of study ( Pantazos and Vatrapu 2016 ; Vatrapu et al. 2013 ).
Relational Analytics Relational analytics is the measure of relationships that exists between two or more variables. Correlation analysis is a typical example of relational analytics that measures the linear relationship between two variables ( Rayward-Smith 2007 ). For instance, Thomas ( 2018 ) applied correlation analysis to select the best features from the speaker and audience measurements. Some researchers have also referred to other forms of relational analytics, such as co-occurrence analysis to reveal students hidden abstract impressions from students written notes ( Taniguchi et al. 2017 ). Others have used relational analytics to differentiate critical formative assessment futures of an individual student to assist teachers in the understanding of the primary components that affect student performance ( Pantazos et al. 2013 ; Michos and Hernández Leo 2016 ). A few others have applied it to distinguish elements or term used to express similarities or differences as they relate to their contexts ( Vatrapu et al. 2013 ). Insights generated from this kind of analysis can be considered to help improve teaching in future lectures and also compare different teaching styles. Sequential pattern mining is also another type of relational analytics used to determine the relationship that exists between subsequent events ( Romero and Ventura 2010 ). It can be applied in multimodal analytics to cite the relationship between the physical aspect of the learning and teaching process such as the relationship between ambient factors and learning; or the investigation of robust multimodal indicators of learning, to help in teacher decision-making ( Prieto et al. 2017 ).
Predictive Analytics Predictive analytics aims to predict future outcomes based on historical and current data ( Gandomi and Haider 2015 ). Just as the name infers, predictive analytics attempts to predict future occurrences, patterns and trends under varying conditions ( Joseph and Johnson 2013 ). It makes use of different techniques such as regression analysis, forecasting, pattern matching, predictive modelling and multi-variant statistics ( Gandomi and Haider 2015 ; Waller and Fawcett 2013 ). In prediction, the goal is to predict students and teachers activities to generate information that can support decision-making by the teacher ( Chatti et al. 2013 ). Predictive analytics is used to answer the "What will happen". For instance, what are the interventions and preventive measures a teacher can take to minimise the failure rate? Herodotou et al. ( Herodotou et al. 2019 ) provided evidence on how predictive analytics can be used by teachers to support active learning. An extensive body of literature suggests that predictive analytics can help teachers improve teaching practice ( Barmaki and Hughes 2015 ; Prieto et al. 2016 ; Prieto et al. 2018 ; Suehiro et al. 2017 ) and also to identify group of students that might need extra support to reach desired learning outcomes ( Goggins et al. 2016 ; Thomas 2018 ).
Prescriptive Analytics Prescriptive analytics provides recommendations or can automate actions in a feedback loop that might modify, optimise or pre-empt outcomes ( Williamson 2016 ). It is used to answer the "How will it best happen?". For instance, how will teachers make the right interventions for students that have been perceived to be at risk to minimise the student dropout rate or what kinds of resources are needed to support students who might need them to succeed? It determines the optimal action that enhances the business processes by providing the cause-effect relationship and applying techniques such as; graph analysis, recommendation engine, heuristics, neural networks, machine learning and Markov process ( Bihani and Patil 2014 ; ur Rehman et al. 2016 ). For example, applying curriculum Knowledge graph and learning Path recommendation to support teaching and learners learning process ( Shen et al. 2018 ).
Actionable Analytics Actionable analytics refers to analytics that prompt action ( Gudivada et al. 2016 ; Gudivada et al. 2018 ; Winkler and Söllner 2018 ). Norris et al. ( 2008 ) used the term action analytics to describe "the emergence of a new generation of tools, solutions, and behaviours that are giving rise to more powerful and effective utilities through which colleges and universities can measure performance and provoke pervasive actions to improve it". The educational sector can leverage some of these innovative, new and cutting edge technologies and techniques such as Natural Language Processing (NLP) ( Sergis and Sampson 2016 ; Taniguchi et al. 2017 ), big data analytics ( Goggins et al. 2016 ) and deep learning ( Prieto et al. 2018 ) to support teacher in both the teaching and learning processes.
Institutional Transformation Data in themselves are not useful; they only become valuable if they can be used to generate insight. In other words, analytics can be applied to institutional data to optimise productivity and performance of the institutional operations, thereby providing value that can transform the institutional practices. In education, there are various purposes of analytics, ranging from those that provide institutions with an overview or deep-down microscopic view of individual students, faculty, curriculum, programs, operations and budgets, to those capable of predicting future trends. Unveiling the value of TA empowers the teachers to identify issues and transform difficulties into opportunities. These opportunities can be employed to optimises the institutional processes, enhance learner experiences and improve teaching performance. TA and LA both play a vital role in effectively reforming and transforming the educational sector to catch up with the fast pace at which data generates. For example, with the extensive use of online and blended learning platforms, the application of analytics will enable institutional stakeholders at all levels to gain new insights into educational data. Today, the HE sector is at crossroads, where there is a need for synergies in learning research and data analytics to transform the way teaching and learning are fundamentally carried out.
The link between TA, LA and LD
Primarily, TA aims to link the centrepiece of LA and remodel them to address teaching challenges. More specifically, TA argues that connecting and analysing insights generated from LA methods and tools with those generated from in-class methods and tools, through TA tools could support teacher reflection and improve TPD based on evidence. Hence, this concept is presented further in the next subsection.
Conceptual framework of TA
Based on the different perceptions of TA described in previous reviews, this study proposes a conceptual framework for TA to model the complex interaction existing around TA. Three nodes (LA, TA and LD) are interconnected to each other forming a triadic network with the teacher at the centre, performing value-added interactions to make informed based decisions. Each part of this interconnection forms a triangle, totalling three triangles (A, B and C) (see Fig. 4 ).
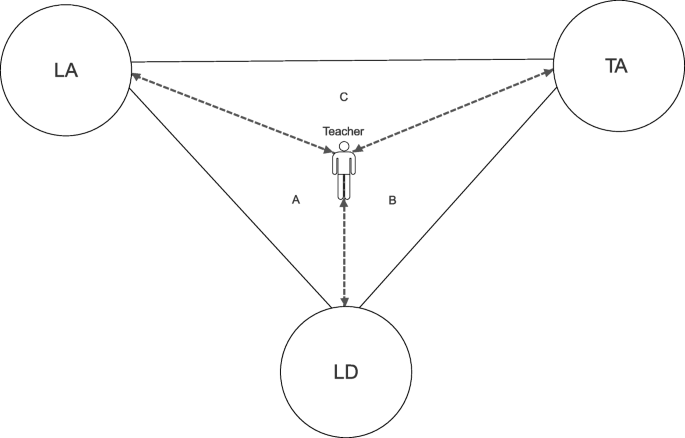
Conceptualisation of TA. Triadic TA Conceptual Framework
The proposed framework is not bound to any particular implementation of learning or design technology. Instead, the point is to describe the elements of analytics and data sources that are key for each domain to guide the use of analytical methods, tools and technology to support the multiple dimensions of learning design successfully.
This triad illustrates the interaction occurring between the teacher, the LA and the LD, to inform TPD. Hernández-Leo et al. ( 2019 ) argued that LD could contribute to structuring and orchestrating the design intent with learners digital trace patterns, advancing the knowledge and interpretation of LA. LA tailored to fit the design intent could be considered by teachers as contributing to the enhancement of the LD in subsequent design interactions. For example, LA could be an information tool to inform the tutors or designers of pedagogical decision making ( Persico and Pozzi 2015 ). Hence, a teacher may want to utilise LA to make just-in-time pedagogical decisions, such as grouping students based on their performance.
Similarly, a teacher may want to investigate if the estimated time taken for students to carry out learning tasks is reasonable or whether adjustments need to be made to the course design ( Hernández-Leo et al. 2019 ; Pozzi and Persico 2013 ). This domain can also provide teachers with analytics regarding the challenges and difficulties students face in the problem-solving phase while performing a task. In return, they give the teacher information in the form of TAD summarising the various challenges students encountered with that activity. They may also provide solutions on how to address them. For example, an early alert system that instantiates a dashboard for instructors using some metrics calculations such as login counts and page views ( Thille and Zimmaro 2017 ). The data sources in the LA node can improve teachers’ awareness, which could also lead to the improvement of LD and help to distinguish design elements that could modify future designs. Data collection in this domain is mostly automatic through virtual learning environments (e.g., LMS, MOOCs). Other forms of data collection may include social media platforms (e.g., Facebook, Tweeter), wearable sensors (e.g., eye-trackers, EEG), software tools that support and collect data related to specific student activities and attendance ( Bakharia et al. 2016 ; Bos and Brand-Gruwel 2016 ).
This triangle represents the relationship between the teacher, the LD and TA. While experiencing LD, TA endeavours to handle continues teachers’ engagement, progression, achievement and learners satisfaction ( Bakharia et al. 2016 ; Sergis and Sampson 2017 ). For example, exploring the impact of video shot on instructor performance and student learning. Using MOOC AB testing, teachers could experiment whether a difference in video production setting would have any impact on the instructors acting performance, or whether any changes in format and instructors performance will result in detectable differences in student viewing behaviour ( Chen et al. 2016 ).
Further, data sources in TA could assist teacher reflection on the impacts of their LD. Data collection could also be automatic by the use of wearable sensors on the teachers while performing teaching activities, also known as in-class analytics. Several institutions now record video contents of their face-to-face classes. Some others even go a step further by collecting their physiological data. These datasets, as mentioned earlier, have a way of exemplifying and illustrating things that ordinarily, a book of pedagogy cannot convey, in providing systematic feedback for the teachers. It involves capturing data during a traditional in-class, face-to-face teacher-centric instruction or teacher-student interaction (where students learn by directly or indirectly interacting with instructors in a lab or lecture hall) and analysing data to identify areas of possible improvements. The kind of data usually captured in this setting are audio, video, body movement, brain activity, cortex activity, to mention just a few. For example, a teacher can perform diagnostic analysis on class recorded videos to expose what is intrinsic during his lecture. This kind of diagnostic analysis could help teachers understand more about their teaching and discover areas of further improvement. SET is another form of data about the teachers; they are collected via the institutional application platforms ( Hernández-Leo et al. 2019 ) and can be visualised to improve teaching performance..
Analytics that happens in the LD involves the visualisation of teaching design to facilitate teacher reflection on the lesson plan, visualisation of the extent to which the lesson plan aligns with the educational objectives, and finally, validation of the lesson plan to highlight potential inconsistencies in the teaching design. For example, a teacher can visualise the number of assessment activities of the lesson plan or the various types of educational resources used in the lesson plan, to know if they are still valid or obsolete. Similarly, a teacher could analyse the time allocated for each lesson activity, to find out if the time allocated for each activity is good enough, or visualise the level of inconsistencies of time misappropriations and imbalances between the overall lesson plan and the individual lesson activities.
This area presents the communication between the teacher, the LA and the TA. Chinchu Thomas ( 2018 ) explored the correlation between student ratings on teaching and student physiological data. Similarly, Schmidlin ( 2015 ) established how to analyse and cross-reference data without decrypting the data sources. Hence, we argue that SET could be linked with LA such as student digital traces from LMS ( Stier et al. 2019 ) and other forms of data (such as attendance data), without compromising privacy. This claim for data fusion could support the teachers to make informed-decisions in new ways. For example, analytics performed on linked datasets could quickly reveal those student opinions that may not count at the end of the semester courses.
Visualisations that could quickly realise students with low participation rates and link it to their opinions, without revealing any identity. Additionally, teachers may be interested in comparing the view of students with low participation rate with those of high participation rate. This kind of information may lead teachers towards making explicit judgements with evidence. A tutor may choose to disregard the opinions of those students that participated less than 20 per cent in-class activities and assignments, as well as had a low attendance rate. Hence, narrowing concentration more on the opinions of students that participated in improving teaching practice.
However, considering ethical concerns, data fusion at the individual level still requires explicit and informed consent from the students whose data are collected ( Menchen-Trevino 2016 ). Other issues such as privacy concerns, data fusion can be problematic as this usually requires that the teachers know student identities. However, from a programmatic perspective, extra measures can be put in place to address this concern. Algorithms can be interfaced to mask student identities to some other unique identities to make them anonymous but linked ( Schmidlin et al. 2015 ) to provide a richer set of data for the teacher to make informed decisions.
Teachers can get a better picture towards improving the context in which learning happens, only if they can be informed about both how they teach and how students learn. Hence, this framework aims to continually provide teachers with interesting information from intelligent feedback based on data generated from users and learning context to improve their learning design and teaching outcome continuously.
Teaching Outcome Model (TOM)
Design-based research advances instructional design work, theory, and implementation as iterative, participatory, and located rather than processes "owned and operated" by designers of instructions ( Wang and Hannafin 2005 ). TOM is an iterative process that follows a design-based research approach to guide teachers, researchers, faculty and administrators on how to utilise data to improve the quality of teaching and learning outcome. This model enables teachers to investigate and evaluate their work using data. Consequently, improving the teacher use of data to inform teaching practice. To build more awareness with regards to teaching data, TOM models TA through iterative cycles of data collection, data analysis, data visualisation and action stages which are interdependent of each other (see Fig. 5 ). Design-based research, as a pragmatic methodology, can guide TOM while generating insights that can support teacher reflections on teaching and student learning. Conversely, TOM ensures that design-based research methodologies can be operational and systemised. Following the various stages outlined in the model, teachers can regularly identify, match and adjust teaching practice, and learning design to all the learners need.
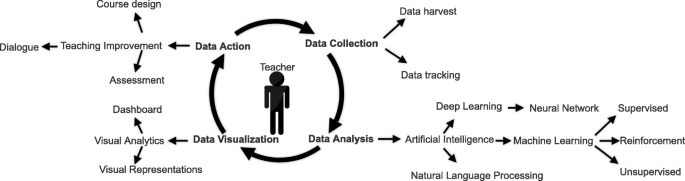
Teaching Outcome Model. TA Life cycle
In the data collection stage, a constant stream of data accumulates from the digital traces relating to teaching daily activities and engagements, including structured and unstructured data, visual and non-visual data, historical and real-time data. It is also important to note that the rate at which diverse data accumulates in our educational system will keep growing. According to Voithofer and Golan ( 2018 ), there are several ways to mine teaching and learning data without professional knowledge that is beyond the necessary teacher training experience in data literacy, administering learning design and class orchestration. Subscribing to this school of thought, adopting Big data infrastructure in our institutions will guarantee easy access to data by the various stakeholders, this will also mitigate the bottleneck of disparate data points existing in our educational sector. Therefore, enabling educators to focus more attention on instruction, setting up interactive class activities, and participating more on discussions that will create more data for evidence-based decision making. Also, the misuse of data is a broad primary concern ( Roberts et al. 2017 ). One critical matter is identifying the types of data that can be collected, analysed and visualized; to ensure that the right people have access to the data for the right purpose. As such, implementing data governance policies around institutional data such as; ’open definition of purpose, scope and boundaries, even if that is broad and in some respects, open-ended’ is critical ( Kay et al. 2012, p 6 ). This sort of measure will introduce clarity and address issues around who controls what data as well as security and privacy issues around data.
Analysis stage
This step involves the different ways of working with data to ensure data quality. Professionals such as data scientists, programmers, engineers and researchers need to work together with the teachers at this level. They can apply data mining techniques, statistical methods, complex algorithms, and AI techniques (such as NLP, AI, ML, deep learning) to adequately transform data into the useful analytical process. Analytics in the education space presents in diverse forms including, descriptive, diagnostic, predictive and prescriptive. These different forms of analytics can be utilised to offer a high-level view or fine-grained view of individual learners, teacher, faculty and their various activities, engagements and behaviours. Unravelling the value of data analytics empowers teachers and researchers to identify problems and transform challenges into opportunities that can be utilised to support teacher reflection and enrich teacher data-literacy experiences. For example, teachers can apply NLP on text data to gather topics from discussion posts, contributions participants have made within collaborative projects and their sentiments.
Furthermore, ML techniques could be combined with TA to enhance teaching outcome. For instance, chatbots could support the teacher by acting as a teacher assistant in large classes. An essential consideration in analytics, however, is that data can be easily de-identified ( Roberts et al. 2017 ; Cumbley and Church 2013 ), especially when data sets increase in size and scope and are combined to generate big data. To resolve these concerns, a particular university introduced a two-stage method of data de-identification coupled with data governance to restrict data access ( De Freitas et al. 2015 ).
Visualisation stage
This stage ensures data presentation in useful and meaningful ways to teachers. Empowering teachers with interactive visual interfaces and dashboards that facilitate teacher cognition and promote reflection about pre-processed and fine-grained teaching and learning activities. Through TAD, can project real-time and historical information from different data sources that might not be necessarily interoperable, and results summarised ( Moore 2018 ). However, visualisation is "what you see is what you get"; meaning that information presentation method may affect its interpretation, and consequently, may influence decision-making. Hence, it is necessary to address issues around visualisations in diverse forms such as; visual analytics and exploratory data analysis to create room for visual interactivity, exploratory visualisation to discover trends, patterns, relationships and behaviours. For example, a teacher can use a TAD to monitor student engagement. When the student engagement is poor, it may prompt the teacher to take necessary actions such as; changing teaching material and making it more interactive. Additionally, there are also questions around privacy, such as who has access to visualisations relevant to an instructor, such as other faculty members participating in the course, directly or indirectly, administrators, researchers, potential employees of other institutions.
Action stage
At this stage, informed-decision leads to action and actions unavoidably reshape our environment; subsequently, regenerate new data. Additionally, there is a to create tools that will be useful to the teacher to understand and make meaning of data quickly. Actions taken by teachers can be used to improve the course design and assessment (value-added formative assessment). In any case, predictive analytics prompts an epistemological question; how should we ensure effective action by the teacher based on flawed predictions such that the system does not collapse?
Discussion and conclusion
This article presents the result of a systematic literature review aimed at describing the conception, and synthesis of the current research on the notion of TA, to provide insight into how TA can be used to improve the quality of teaching. The first part of the article described what is meant by TA to consolidate the divergent discourse on TA. The review showed that TA applies to analytics on teaching activities as well as methods of improving teachers’ awareness on students’ activities, including supporting the teachers to understand student learning behaviours to provide adequate feedback to teachers. In essence, the primary goal of TA is to improve teaching performance. The literature also revealed the several tools and methods are available for extracting digital traces associated with teaching in addition to traditional student evaluation tools. However, one of the main challenges recognised was the cost associated with some devices used to capture in-class activities, and ML techniques have been proposed to minimise this challenge.
The literature has also recognised teacher inquiry as a promising area of research in TA and came to a consensus that methods, like multimodal analytics and SNA, could help promote teacher inquiry and teacher reflection. Visualisations and visual analytics techniques are very significant in TA and also encourage teacher inquiry. The use of visualisation dashboards and TAD are essential tools that the modern-day teachers require to carry out a continuous and efficient reflection on teaching practice.
The emphasis of the synthesis of TA was clearly on data collection, analysis and visualisation, as illustrated in Fig. 6 . In the literature, the various kinds of data collected and used to improve teaching practice, include:
Digital trace data; "records of activity (trace data) undertaken through an online information system (thus, digital)" [119]. They incorporate various activities generated from custom applications and learning environments that leave digital footprints.
Image data are photographic or trace objects that represent the underlying pixel data of an area of an image element.
Physiological data are body measurement based on body-mounted sensors ( Lazar et al. 2017 ), used to extract data from teachers while performing classroom teaching activities.
Audio-video stream data or recorded lecturer data with captured physical teaching activities and students learning activities. Hence, attainable with mounted cameras, computer or mobile cameras connected to applications like Zoom and Skype, eye tracks with recording capabilities and digital cameras connected to learning environments such as Eco365.
Social data are data with online social activities, including utilising the repertory grid technique to collect students’ assessment data from social media sites.
Text data, including quantitative and qualitative data, data generated from text documents such as discussion forums, students essay or articles, emails and chat messages.
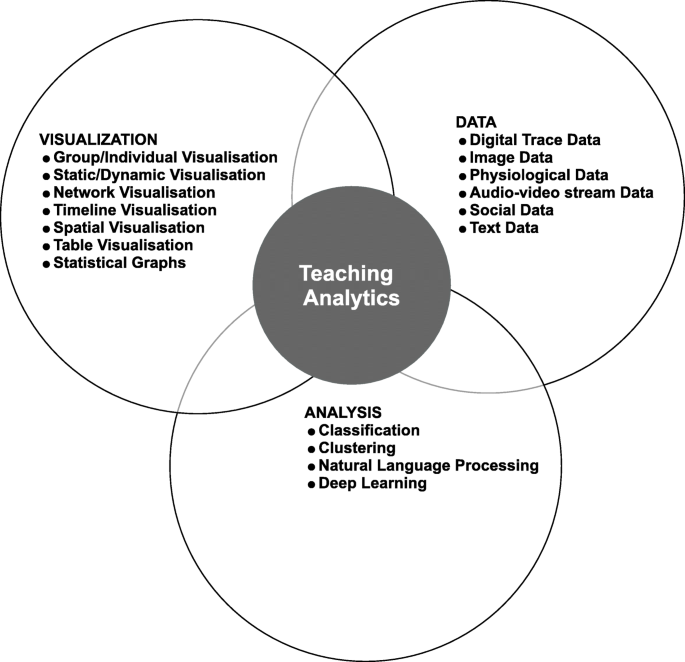
Dimensions of TA. Illustration of TA based on the literature
Analysis in this context refers to the application of Educational Data Mining (EDM) and deep learning techniques mostly used to process data. EDM approaches is a complicated process that requires an interweaving of various specialised knowledge and ML algorithms, especially to improve teaching and learning ( Chen 2019 ). NLP and classification are the two main EDM techniques applied in TA. However, the review also recognised the use of other methods such as clustering and deep learning techniques, to support teachers.
As commonly said, a picture is worth more than a thousand words; visualisation can effectively communicate and reveal structures, patterns and trends in variables and their interconnections. Research in TA has applied several visualisation techniques including Network, Timeline, Spatial, Table and Statistical Graphs. For instance, SNA is a form of visual analytics that is used to support teachers to determine how different groups interact and engage with course resources. Identifying differences in interaction patterns for different groups of students may result in different learning outcomes, such as, how access patterns of successful groups of students differ from that of unsuccessful students. Applying visualisation techniques can support teachers in areas such as advising underperforming students about effective ways to approach study. Visualisation can enable teachers to identify groups of students that might need assistance and discover new and efficient means of using collaborative systems to achieve group work that can be taught explicitly to students.
However, while acknowledging the incomplete nature of data and complexities associated with data collection, analysis and use, teachers should take caution to avoid bais. Data collected in one context may not be directly applicable to another or have both benefits and cost for individuals or groups from which data was harvested. Therefore, key stakeholders, including teachers, course directors, unit coordinators and researchers must pay proper attention to predictive models and algorithms and take extra care to ensure that the contexts of data analysed are carefully considered. There are also privacy concerns, such as who has access to view analytics relating to a teacher, including other faculty members both directly or indirectly involved in the course, administrators, researchers, future employees of other institutions. It will be useful for institutions to have clear guidelines as to who has access to what and who views what. Other issues around data include how long should data remain accessible ( Siemens 2013 ), with big data technology and infrastructure, data should be kept for as long as it can exist. Pardo and Siemens ( 2014 ) acknowledged that the use of analytics in higher education research has no clear interpretation of the right to privacy. They seem opposed to the need for absolute privacy, on the basis that the use of historical data enhances research with potential rewards for the future of teaching professional development and student outcome.
The review provided in the current article highlighted the significant limitations in the existing literature on teaching analytics. The TAD is proposed to guide teachers, developers, and researchers to understand and optimise teaching and the learning environments. The critical aspect of this review is establishing the link between LA, TA and LD and its value in informing teachers’ inquiry process. Also, the review describes the relationship between LA, TA and LD. Finally, the article proposes TOM, which draws from a research-based approach to guide teachers on how to utilise data to improve teaching. The outcome of this model is a TAD that provides actionable insights for teacher reflection and informed decision-making. Therefore, showing the value that TA brings to pedagogic interventions and teacher reflection.
Theoretical implications
The analysis of data collected from the interaction of teachers with technology and students is a promising approach for advancing our understanding of the teaching process and how it can be supported. Teachers can use data obtained from their teaching to reflect on their pedagogical design and optimise the learning environment to meet students’ diverse needs and expectations.
Teacher-centric learning design can improve the utility of new technologies and subsequent acceptance of the use of these technologies to improve the quality of teaching and enhance students learning experience. TAD is one class of tools that can be designed in such a way that will improve teaching practice.
Research on learning analytics has revealed useful insights about students’ learning and the context in which they learn. While the ability to track, harvest and analyse various forms of learning analytics can reveal useful insights about learners’ engagement with learning environments, our review suggests that there is limited focus on analytics relating to the teacher, their teaching approaches and activities. Also, there has been increasing advances in the design of learner and teaching dashboards. However, many teachers still struggle with understanding and interpreting dashboards partly because they lack data literacy skills, and mostly because most the design of many of the tools does not include teachers as partners.
Although, TAD enable teachers to inspect, and understand the processes and progress relating to their teaching, the current implementations of TAD in general, does not adequately provide teachers with the details they need or want in a readily usable format. Educational technology developers can utilise our proposed model to design better tools for improving teaching practice. For example, a TAD can be designed to perform text analytics on students qualitative comments about a course taught, and results presented to the teacher in the form of themes, sentiments and classification; such that it will support the instructor’s needs and preferences for insight generation and reflection.
Teachers monitor, observe and track both teaching and learning activities to make appropriate decisions. Moreover, it is also important to note that visualisations can be misrepresented, misinterpreted or misused by the viewer [122]. Hence, perception and cognition remain a significant challenge in TAD. Consequently, it becomes necessary to design and write algorithms that extract information visualisation, in such a way that allows adequate understanding by teachers. It is also crucial for dashboards to integrate multiple sources such as combining both the learning and teaching activities into a TAD, to create room for teachers to comprehend, reflect on and act upon the presented information quickly.
Also, the current state of technology shows little progress in taking TA, raising concerns about the accurate validity and scalability of innovations such as predictive analytics and TAD. Furthermore, the ethical issues of data use are not considered sufficient to establish institutional policies which incorporate TA as part of quality education models.
Finally, consideration of the framework’s three layers as a whole raises new questions and opportunities. For example, linking educational performance and satisfaction to specific learning design involves consideration of elements of all three layers. This review has shown that TA is a new and essential area of analytics in education. The study also suggests that the conceptualisation of teaching analytics is still at its infancy. However, the practical and successful use of teaching analytics is highly dependent on the development of conceptual and theoretical foundations into consideration.
Implications for practice
This review has uncovered the value of TA and its role in fostering data literacy skills in teachers to support evidence-based teaching. The purpose of TOM is to guide the development of teaching dashboard, and for researchers to develop strategies that help meaningful ways in which data can be presented to teachers. Teacher dashboards can empower the teachers with tools that create new opportunities to make data-informed strategic decisions, utilising the power of analytics and visualisation techniques. Consequently, increasing the efficiency and effectiveness of the institution, including, improving teaching practice, curriculum development and improvement, active learning engagement and improved students’ success. TOM also presents a platform for teaching academics who may have the best understanding of their course contexts, to provide a significant contribution to a culture of data-informed teaching practice within an institution.
The responsibility for managing the systems that provide the analytics usually falls within the control and supervision of the institution’s information technology (IT) department, and often, they have little to no knowledge of their pedagogical applications to teaching and learning. Likewise, academics and their fields of learning support are often deprived of IT skills and have little to no professional understanding of how software systems work. TOM provides opportunities for the teachers to be involved in the design of TA by providing significant interaction and collaboration between the IT and the other sectors that interpret and act upon the information flow.
Additionally, institutions need to provide teaching staff with the necessary training that fosters the development of data literacy skills, and in the use of data and analytical or visualisation dashboards to monitor their teaching practice. Based on some of the challenges identified in the present review, it is imperative institutions ensure that data is collected transparently, with the awareness of all the stakeholders involved, and informed consent of individuals where appropriate. With the advancements in computing technology, data collection, analysis and use have significantly increased, large amounts of data can be continually pulled from different sources and processed at fast speeds. Big data offers institutions the opportunity to implement big data infrastructures and utilise the full potential of data analytics and visualisation. However, institutions also need to consider implementing a data governance framework to guide the implementation and practice of analytics.
The conceptual framework of TA was established to demonstrate the relationship between LA, TA and LD, which can be useful knowledge to various institutional stakeholders, including the learners, teachers, researchers and administrators. However, there are also issues around data ownership, intellectual property rights, and licensing for data re-use (the students, the instructor, the researcher or the institution). For instance, the same data sources can be shared amongst the various stakeholders, but with different level of access, as such data sharing agreement would be needed to guide sharability without infringing on rights, violating privacy or disadvantaging individuals. The implementation of data sharing agreement would require the building of institutional, group as well as individual trust, which would include guidelines on sharing data within the institution and between third parties, such as external organisations and other institutions. In general, stricter data management policies that guide data collection, analysis and use is essential for every institution.
Limitations and future research
Teaching analytics is an emergent phenomenon in the learning analytics and data science literature, with a limited body of published work in the area, as such conclusions drawn from the review are limited to the databases interrogated and articles reviewed. Further, findings in the review are likely to be influenced by our interpretation of the literature and untestable assumptions. For example, linking LA, TA and LD and their underlying assumptions is not grounded in empirical work. The review serves as an advocacy for teacher data literacy and the ability to work with various forms of data. However, working with a single data point may not be publicly accessible to teachers.
Moreover, the combination of analytics on the several data points may lead to some level of identification, and this would require navigating issues around access, protecting privacy, and obtaining appropriate consents. Therefore, it is almost impossible for individual teachers to comprehend not only the scope of data collected, analysed and used but also the consequences of the different layers of collection, analysis and use. Consequently, making it challenging for teachers to make use of the full potentials of data to make informed choices in learning design. No matter how straightforward or transparent institutional policies around data are, the sheer complexity of the collection, analysis and use has made it impossible, posing a fundamental issue for the stakeholders trying to use analytics to enhance teaching practice and learning outcome across an institution.
In future research, we hope to carry out more extensive empirical research on how TOM could be applied to address issues with regards to ethical and privacy concerns about the utilization of TA. We are currently exploring how teaching analytics dashboards can be used to support teacher data literacy and use analytics to improve teaching practice and learning outcome.
Availability of data and materials
Not applicable.
Abbreviations
Academic analytics
Artificial intelligence
Educational data mining
Higher education
Interactive whiteboard
- Learning analytics
Learning design
Learning management system
Machine learning
Massive open online courses
Natural language processing
Open learners model
Student evaluation of teaching
Social network analysis
- Teaching analytics
Teaching analytics dashboard
Term frequency inverse document frequency
- Teaching and learning analytics
- Teaching outcome model
Technology, pedagogy, and content knowledge
Teacher professional development
Adams, M.J., & Umbach, P.D. (2012). Nonresponse and online student evaluations of teaching: Understanding the influence of salience, fatigue, and academic environments. Research in Higher Education , 53 (5), 576–591.
Article Google Scholar
Anderson, T. (2003). Getting the mix right again: An updated and theoretical rationale for interaction. The International Review of Research in Open Distributed Learning , 4 (2).
Asare, S., & Daniel, B.K. (2017). Factors influencing response rates in online student evaluation systems: A systematic review approach. In E-Learn: World Conference on E-Learning in Corporate, Government, Healthcare, and Higher Education . Association for the Advancement of Computing in Education (AACE), (pp. 537–541).
Assunção, M.D., Calheiros, R.N., Bianchi, S., Netto, M.A., Buyya, R. (2015). Big data computing and clouds: Trends and future directions. Journal of Parallel and Distributed Computing , 79 , 3–15.
Bakharia, A., Corrin, L., De Barba, P., Kennedy, G., Gašević, D., Mulder, R., Williams, D., Dawson, S., Lockyer, L. (2016). A conceptual framework linking learning design with learning analytics. In Proceedings of the Sixth International Conference on Learning Analytics & Knowledge . ACM, (pp. 329–338).
Banerjee, A., Bandyopadhyay, T., Acharya, P. (2013). Data analytics: Hyped up aspirations or true potential? Vikalpa , 38 (4), 1–12.
Barmaki, R., & Hughes, C.E. (2015). Providing real-time feedback for student teachers in a virtual rehearsal environment. In Proceedings of the 2015 ACM on International Conference on Multimodal Interaction . ACM, (pp. 531–537).
Beer, C., Jones, D., Clark, K. (2009). The indicators project identifying effective learning: Adoption, activity, grades and external factors. In Ascilite . Citeseer.
Benton, S.L., & Cashin, W.E. (2014). Student ratings of instruction in college and university courses , (pp. 279–326): Springer.
Bihani, P., & Patil, S. (2014). A comparative study of data analysis techniques. International Journal of Emerging Trends & Technology in Computer Science , 3 (2), 95–101.
Google Scholar
Borgman, C.L., Abelson, H., Dirks, L., Johnson, R., Koedinger, K.R., Linn, M.C., Lynch, C.A., Oblinger, D.G., Pea, R.D., Salen, K. (2008). Fostering learning in the networked world: The cyberlearning opportunity and challenge. a 21st century agenda for the national science foundation. https://doi.org/10.1037/e532532011-001 .
Boring, A. (2015). Gender biases in student evaluation of teachers . Paris. https://doi.org/10.1016/j.jpubeco.2016.11.006 .
Boring, A., Ottoboni, K., Stark, P.B. (2016). Student evaluations of teaching are not only unreliable, they are significantly biased against female instructors. Impact of Social Sciences Blog . The London School of Economics and Political Science.
Bos, N., & Brand-Gruwel, S. (2016). Student differences in regulation strategies and their use of learning resources: implications for educational design. In Proceedings of the Sixth International Conference on Learning Analytics & Knowledge . ACM, (pp. 344–353).
Braga, M., Paccagnella, M., Pellizzari, M. (2014). Evaluating students’ evaluations of professors. Economics of Education Review , 41 , 71–88.
Butson, R., & Daniel, B. (2017). The Rise of Big Data and Analytics in Higher Education , (pp. 127–140): Auerbach Publications.
Charleer, S., Klerkx, J., Odriozola, S., Luis, J., Duval, E. (2013). Improving awareness and reflection through collaborative, interctive visualizations of badges. In ARTEL13: Proceedings of the 3rd Workshop on Awareness and Reflection in Technology-enhanced Learning, vol. 1103 . CEUR Workshop Proceedings, (pp. 69–81).
Chatti, M.A., Dyckhoff, A.L., Schroeder, U., Thüs, H. (2013). A reference model for learning analytics. 5-6 , 4 , 318–331.
Chen, L.-L. (2019). Enhancing teaching with effective data mining protocols. Journal of Educational Technology Systems , 47 (4), 500–512.
Chen, Z., Chudzicki, C., Palumbo, D., Alexandron, G., Choi, Y.-J., Zhou, Q., Pritchard, D.E. (2016). Researching for better instructional methods using ab experiments in moocs: results and challenges. Research and Practice in Technology Enhanced Learning , 11 (1), 9.
Choudhury, S., Hobbs, B., Lorie, M., Flores, N. (2002). A framework for evaluating digital library services. D-Lib magazine , 8 (7/8), 1082–9873.
Chounta, I.-A., McLaren, B.M., Albacete, P.L., Jordan, P.W., Katz, S. (2016). Analysis of human-to-human tutorial dialogues: Insights for teaching analytics. In IWTA@ EC-TEL , (pp. 9–17).
Cumbley, R., & Church, P. (2013). Is “big data” creepy? Computer Law & Security Review , 29 (5), 601–609.
Dana, N.F., & Yendol-Hoppey, D. (2019). The Reflective Educator’s Guide to Classroom Research: Learning to Teach and Teaching to Learn Through Practitioner Inquiry : Corwin.
Daniel, B. (2015). Big data and analytics in higher education: Opportunities and challenges. British Journal of Educational Technology , 46 (5), 904–920. https://doi.org/10.1111/bjet.12230 .
Daniel, B., & Harland, T. (2017). Higher Education Research Methodology: A Step-by-Step Guide to the Research Process . Routledge London. https://doi.org/10.4324/9781315149783 .
Daniel, B.K. (2019). Artificial reality: The practice of analytics and big data in educational research. In: Pedersen, J.S., & Wilkinson, A. (Eds.) In Big data: Promise, application and pitfalls . https://doi.org/10.4337/9781788112352.00018 . Edward Elgar, Cheltenham, (pp. 287–300).
Chapter Google Scholar
De Freitas, S., Gibson, D., Du Plessis, C., Halloran, P., Williams, E., Ambrose, M., Dunwell, I., Arnab, S. (2015). Foundations of dynamic learning analytics: Using university student data to increase retention. British Journal of Educational Technology , 46 (6), 1175–1188.
Dix, A.J., & Leavesley, J. (2015). Learning analytics for the academic: An action perspective. J. UCS , 21 (1), 48–65.
Ducheva, Z., Pehlivanova, M., Dineva, S. (2013). Possibilities for students to evaluate and improve electronic courses. In The 8th International Conferemnce on Virtual Learning ICVL .
Edström, K. (2008). Doing course evaluation as if learning matters most. Higher Education Research & Development , 27 (2), 95–106.
Elias, T. (2011). Learning analytics. Learning , 1–22.
Ferguson, R. (2012). Learning analytics: drivers, developments and challenges. International Journal of Technology Enhanced Learning , 4 (5/6), 304–317.
Fischer, F., Wild, F., Sutherland, R., Zirn, L. (2014). Grand Challenge Problems from the Alpine Rendez-Vous , (pp. 3–71): Springer.
Flanders, N.A. (1970). Analyzing Teacher Behavior . Addison-Wesley P.C.
Flavin, M. (2017). Disruptive Technology Enhanced Learning: The Use and Misuse of Digital Technologies in Higher Education : Springer. https://doi.org/10.1057/978-1-137-57284-4 .
Gandomi, A., & Haider, M. (2015). Beyond the hype: Big data concepts, methods, and analytics. International Journal of Information Management , 35 (2), 137–144.
Gauthier, G. (2013). Using teaching analytics to inform assessment practices in technology mediated problem solving tasks. In IWTA@ LAK .
Ginon, B., Johnson, M.D., Turker, A., Kickmeier-Rust, M. (2016). Helping Teachers to Help Students by Using an Open Learner Model. https://doi.org/10.1007/978-3-319-45153-4_69 .
Goggins, S.P. (2012). Group informatics: A multi-domain perspective on the development of teaching analytics. In Proceedings of the TaPTA Workshop at EC-TEL .
Goggins, S.P., Galyen, K., Petakovic, E., Laffey, J.M. (2016). Connecting performance to social structure and pedagogy as a pathway to scaling learning analytics in moocs: an exploratory study. Journal of Computer Assisted Learning , 32 (3), 244–266.
Gorham, J. (1988). The relationship between verbal teacher immediacy behaviors and student learning. Communication Education , 37 (1), 40–53.
Griffiths, D. (2017). The use of models in learning design and learning analytics. Interaction Design and Architecture(s) Journal , 33 , 113–133.
Gudivada, V.N., Irfan, M., Fathi, E., Rao, D. (2016). Cognitive analytics: Going beyond big data analytics and machine learning (Vol. 35, pp. 169–205).
Gudivada, V.N., Rao, D.L., Ding, J. (2018). 2. Evolution and Facets of Data Analytics for Educational Data Mining and Learning Analytics , (pp. 16–42). New York. https://doi.org/10.4324/9780203728703-3 .
Harland, T., & Wald, N. (2018). Vanilla teaching as a rational choice: the impact of research and compliance on teacher development. Teaching in Higher Education , 23 (4), 419–434.
Hernández-Leo, D., Martinez-Maldonado, R., Pardo, A., Muñoz-Cristóbal, J.A., Rodríguez-Triana, M.J. (2019). Analytics for learning design: A layered framework and tools. British Journal of Educational Technology , 50 (1), 139–152.
Herodotou, C., Hlosta, M., Boroowa, A., Rienties, B., Zdrahal, Z., Mangafa, C. (2019). Empowering online teachers through predictive learning analytics. British Journal of Educational Technology . https://doi.org/10.1111/bjet.12853 .
Huang, C.-W. (2001). Educlick: A computer-supported formative evaluation system with wireless devices in ordinary classroom. In Proceedings of Int. Conference on Computers in Education, 2010 , (pp. 1462–1469).
Joseph, R.C., & Johnson, N.A. (2013). Big data and transformational government. IT Professional , 15 (6), 43–48.
Kaser, L., & Halbert, J. (2014). Creating and sustaining inquiry spaces for teacher learning and system transformation. European Journal of Education , 49 (2), 206–217.
Kay, D., Korn, N., Oppenheim, C. (2012). Legal, risk and ethical aspects of analytics in higher education. Analytics Series . JISC Cetis (Centre for educational technology and interoperability standards).
KU, O., LIANG, J.-K., CHANG, S.-B., WU, M. (2018). Sokrates teaching analytics system (stas): An automatic teaching behavior analysis system for facilitating teacher professional development. In Proceedings of the 26th International Conference on Computers in Education. Philippines: Asia-Pacific Society for Computers in Education .
Laney, D. (2001). 3d data management: Controlling data volume, velocity and variety. META Group Research Note. META group research note , 6 (70), 1.
Lazar, J., Feng, J.H., Hochheiser, H. (2017). Research Methods in Human-computer Interaction : Morgan Kaufmann.
Leitner, P., Khalil, M., Ebner, M. (2017). Learning analytics in higher education—a literature review , (pp. 1–23): Springer.
Libbrecht, P., Kortenkamp, U., Rebholz, S., Müller, W. (2013). Tales of a companion teacher analytics. In IWTA@ LAK .
Linse, A.R. (2017). Interpreting and using student ratings data: Guidance for faculty serving as administrators and on evaluation committees. Studies in Educational Evaluation , 54 , 94–106.
Loughran, J.J. (2002). Effective reflective practice: In search of meaning in learning about teaching. Journal of teacher education , 53 (1), 33–43.
Macfadyen, L.P., & Dawson, S. (2012). Numbers are not enough. why e-learning analytics failed to inform an institutional strategic plan. Journal of Educational Technology Society , 15 (3).
MacNell, L., Driscoll, A., Hunt, A.N. (2015). What’s in a name: Exposing gender bias in student ratings of teaching. Innovative Higher Education , 40 (4), 291–303.
Mansfield, J. (2019). Pedagogical Equilibrium: The Development of Teachers’ Professional Knowledge : Routledge.
Marlin Jr, J.W., & Niss, J.F. (1980). End-of-course evaluations as indicators of student learning and instructor effectiveness. The Journal of Economic Education , 11 (2), 16–27.
Marsh, H.W. (1987). Students’ evaluations of university teaching: Research findings, methodological issues, and directions for future research. International Journal of Educational Research , 11 (3), 253–388.
McKenney, S., & Mor, Y. (2015). Supporting teachers in data–informed educational design. British journal of educational technology , 46 (2), 265–279.
Menchen-Trevino, E. (2016). Web historian: Enabling multi-method and independent research with real-world web browsing history data. In IConference 2016 Proceedings . https://doi.org/10.9776/16611 .
Michos, K., & Hernández Leo, D. (2016). Towards understanding the potential of teaching analytics within educational communities. In: Vatrapu, R.G.B.B.S., & Kickmeier-Rust, M. (Eds.) In Vatrapu R, Kickmeier-Rust M, Ginon B, Bull S. IWTA 2016 International Workshop on Teaching Analytics. Proceedings of the Fourth International Workshop on Teaching Analytics, in Conjunction with EC-TEL 2016; 2016 Sept 16; Lyon, France.[place Unknown]: CEUR Workshop Proceedings , (pp. 1–8).
Moore, B.L. (2018). 6. The Role of Data Analytics in Education Possibilities and Limitations, 1st edn.https://doi.org/10.4324/9780203728703-8.
Mor, Y., Ferguson, R., Wasson, B. (2015). Learning design, teacher inquiry into student learning and learning analytics: A call for action. British Journal of Educational Technology , 46 (2), 221–229.
Müller, W., Rebholz, S., Libbrecht, P. (2016). Automatic inspection of e-portfolios for improving formative and summative assessment. In International Symposium on Emerging Technologies for Education . Springer, (pp. 480–489).
Norris, D., Baer, L., Leonard, J., Pugliese, L., Lefrere, P. (2008). Action analytics: Measuring and improving performance that matters in higher education. EDUCAUSE Review , 43 (1), 42.
Olson, D.L., & Lauhoff, G. (2019). Descriptive data mining , (pp. 129–130): Springer.
Osterman, K.F., & Kottkamp, R.B. (1993). Reflective Practice for Educators: Improving Schooling Through Professional Development : ERIC.
Pantazos, K., & Vatrapu, R. (2016). Enhancing the professional vision of teachers: A physiological study of teaching analytics dashboards of students’ repertory grid exercises in business education. In System Sciences (HICSS), 2016 49th Hawaii International Conference On . IEEE, (pp. 41–50).
Pantazos, K., Vatrapu, R.K., Hussain, A. (2013). Visualizing repertory grid data for formative assessment. In IWTA@ LAK .
Papamitsiou, Z., & Economides, A.A. (2016). Learning analytics for smart learning environments: A meta-analysis of empirical research results from 2009 to 2015. Learning, Design, and Technology: An International Compendium of Theory, Research, Practice, and Policy , 1–23. https://doi.org/10.1007/978-3-319-17727-4_15-1 .
Pardo, A., & Siemens, G. (2014). Ethical and privacy principles for learning analytics. British Journal of Educational Technology , 45 (3), 438–450.
Pascual-Miguel, F., Chaparro-Pelaez, J., Hernandez-Garcia, A., Iglesias-Pradas, S. (2011). A characterisation of passive and active interactions and their influence on students’ achievement using moodle lms logs. International Journal of Technology Enhanced Learning , 3 (4), 403–414.
Pennings, H.J., Brekelmans, M., Wubbels, T., van der Want, A.C., Claessens, L.C., van Tartwijk, J. (2014). A nonlinear dynamical systems approach to real-time teacher behavior: Differences between teachers. Nonlinear Dynamics, Psychology, and Life Sciences , 18 (1), 23–45.
Persico, D., & Pozzi, F. (2015). Informing learning design with learning analytics to improve teacher inquiry. British Journal of Educational Technology , 46 (2), 230–248.
Pozzi, F., & Persico, D. (2013). Sustaining learning design and pedagogical planning in cscl. Research in Learning Technology , 21 . https://doi.org/10.3402/rlt.v21i0.17585 .
Prieto, L.P., Magnuson, P., Dillenbourg, P., Saar, M. (2017). Reflection for action: Designing tools to support teacher reflection on everyday evidence. https://doi.org/10.31219/osf.io/bj2rp .
Prieto, L.P., Rodriguez-Triana, M.J., Kusmin, M., Laanpere, M. (2017). Smart school multimodal dataset and challenges. In Joint Proceedings of the Sixth Multimodal Learning Analytics (MMLA) Workshop and the Second Cross-LAK Workshop Co-located with 7th International Learning Analytics and Knowledge Conference, vol. 1828 . CEUR, (pp. 53–59).
Prieto, L.P., Sharma, K., Dillenbourg, P., Jesús, M. (2016). Teaching analytics: towards automatic extraction of orchestration graphs using wearable sensors. In Proceedings of the Sixth International Conference on Learning Analytics & Knowledge . ACM, (pp. 148–157).
Prieto, L.P., Sharma, K., Kidzinski, Ł., Rodríguez-Triana, M.J., Dillenbourg, P. (2018). Multimodal teaching analytics: Automated extraction of orchestration graphs from wearable sensor data. Journal of Computer Assisted Learning , 34 (2), 193–203.
Ramos, C., & Yudko, E. (2008). "hits"(not "discussion posts") predict student success in online courses: a double cross-validation study. Computers & Education , 50 (4), 1174–1182.
Rayward-Smith, V.J. (2007). Statistics to measure correlation for data mining applications. Computational Statistics & Data Analysis , 51 (8), 3968–3982.
Article MathSciNet MATH Google Scholar
Remmers, H.H., & Brandenburg, G. (1927). Experimental data on the purdue rating scale for instructors. Educational Administration and Supervision , 13 (6), 399–406.
Rienties, B., Boroowa, A., Cross, S., Farrington-Flint, L., Herodotou, C., Prescott, L., Mayles, K., Olney, T., Toetenel, L., Woodthorpe, J. (2016). Reviewing three case-studies of learning analytics interventions at the open university uk. In Proceedings of the Sixth International Conference on Learning Analytics & Knowledge . ACM, (pp. 534–535).
Roberts, L.D., Chang, V., Gibson, D. (2017). Ethical considerations in adopting a university-and system-wide approach to data and learning analytics , (pp. 89–108): Springer. https://doi.org/10.1007/978-3-319-06520-5_7 .
Romero, C., & Ventura, S. (2010). Educational data mining: a review of the state of the art. IEEE Transactions on Systems, Man, and Cybernetics, Part C (Applications and Reviews) , 40 (6), 601–618.
Saar, M., Kusmin, M., Laanpere, M., Prieto, L.P., Rüütmann, T. (2017). Work in progress–semantic annotations and teaching analytics on lecture videos in engineering education. In Global Engineering Education Conference (EDUCON), 2017 IEEE . IEEE, (pp. 1548–1551).
Saar, M., Prieto, L.P., Rodríguez-Triana, M.J., Kusmin, M. (2018). Personalized, teacher-driven in-action data collection: technology design principles. In 2018 IEEE 18th International Conference on Advanced Learning Technologies (ICALT) . IEEE, (pp. 58–62).
Saric, M., & Steh, B. (2017). Critical reflection in the professional development of teachers: Challenges and possibilities. CEPS Journal , 7 (3), 67–85.
Saye, J.W., & Brush, T. (2007). Using technology-enhanced learning environments to support problem-based historical inquiry in secondary school classrooms. Theory Research in Social Education , 35 (2), 196–230.
Schempp, P., McCullick, B., Pierre, P.S., Woorons, S., You, J., Clark, B. (2004). Expert golf instructors’ student-teacher interaction patterns. Research Quarterly for Exercise and Sport , 75 (1), 60–70.
Schmidlin, K., Clough-Gorr, K.M., Spoerri, A. (2015). Privacy preserving probabilistic record linkage (p3rl): a novel method for linking existing health-related data and maintaining participant confidentiality. BMC Medical Research Methodology , 15 (1), 46.
Sergis, S., & Sampson, D.G. (2016). Towards a teaching analytics tool for supporting reflective educational (re) design in inquiry-based stem education. In Advanced Learning Technologies (ICALT), 2016 IEEE 16th International Conference On . https://doi.org/10.1109/icalt.2016.134 . IEEE, (pp. 314–318).
Sergis, S., & Sampson, D.G. (2017). Teaching and learning analytics to support teacher inquiry: A systematic literature review , (pp. 25–63): Springer. https://doi.org/10.1007/978-3-319-52977-6_2 .
Sergis, S., Sampson, D.G., Rodríguez-Triana, M.J., Gillet, D., Pelliccione, L., de Jong, T. (2017). Using educational data from teaching and learning to inform teachers’ reflective educational design in inquiry-based stem education. Computers in Human Behavior . https://doi.org/10.1016/j.chb.2017.12.014 .
Shen, J., Chen, H., Jiang, J. (2018). A research on techniques for data fusion and analysis of cross-platform mooc data. In 2018 17th International Conference on Information Technology Based Higher Education and Training (ITHET) . IEEE, (pp. 1–8).
Siemens, G. (2013). Learning analytics: The emergence of a discipline. American Behavioral Scientist , 57 (10), 1380–1400.
Stier, S., Breuer, J., Siegers, P., Thorson, K. (2019). Integrating Survey Data and Digital Trace Data: Key Issues in Developing an Emerging Field. https://doi.org/10.1177/0894439319843669 .
Subramanya, S. (2014). Toward a more effective and useful end-of-course evaluation scheme. Journal of Research in Innovative Teaching , 7 (1).
Suehiro, D., Taniguchi, Y., Shimada, A., Ogata, H. (2017). Face-to-face teaching analytics: Extracting teaching activities from e-book logs via time-series analysis. In Advanced Learning Technologies (ICALT), 2017 IEEE 17th International Conference On . IEEE, (pp. 267–268).
Sun, J., Przybylski, R., Johnson, B.J. (2016). A review of research on teachers’ use of student data: From the perspective of school leadership. Educational Assessment, Evaluation and Accountability , 28 (1), 5–33.
Taniguchi, Y., Suehiro, D., Shimada, A., Ogata, H. (2017). Revealing hidden impression topics in students’journals based on nonnegative matrix factorization. In Advanced Learning Technologies (ICALT), 2017 IEEE 17th International Conference On . IEEE, (pp. 298–300).
Thille, C., & Zimmaro, D. (2017). Incorporating learning analytics in the classroom. New Directions for Higher Education , 2017 (179), 19–31.
Thomas, C. (2018). Multimodal teaching and learning analytics for classroom and online educational settings. In Proceedings of the 2018 on International Conference on Multimodal Interaction . ACM, (pp. 542–545).
ur Rehman, M.H., Chang, V., Batool, A., Wah, T.Y. (2016). Big data reduction framework for value creation in sustainable enterprises. International Journal of Information Management , 36 (6), 917–928.
Van Harmelen, M., & Workman, D. (2012). Analytics for learning and teaching. CETIS Analytics Series , 1 (3), 1–40.
van Leeuwen, A., Rummel, N., van Gog, T. (2019). What information should cscl teacher dashboards provide to help teachers interpret cscl situations? International Journal of Computer-Supported Collaborative Learning , 1–29. https://doi.org/10.1007/s11412-019-09299-x .
Vatrapu, R.K. (2012). Towards semiology of teaching analytics. In Workshop Towards Theory and Practice of Teaching Analytics, at the European Conference on Technology Enhanced Learning, TAPTA, vol. 12 .
Vatrapu, R.K., Kocherla, K., Pantazos, K. (2013). iklassroom: Real-time, real-place teaching analytics. In IWTA@ LAK .
Vatrapu, R., Reimann, P., Bull, S., Johnson, M. (2013). An eye-tracking study of notational, informational, and emotional aspects of learning analytics representations. In ACM International Conference Proceeding Series . https://doi.org/10.1145/2460296.2460321 . https://www.scopus.com/inward/record.uri?eid=2-s2.0-84876499638&doi=10.1145%2f2460296.2460321&partnerID=40&md5=e7b4d83a3e33e7a1c3c5b5f56d5ebe7d , (pp. 125–134).
Vatrapu, R., Reimann, P., Hussain, A., Kocherla, K. (2013). Towards teaching analytics: Repertory grids for formative assessment (rgfa). In CSCL 2013 Conference Proceedings, vol 2 , (pp. 422–426).
Vatrapu, R., Tanveer, U., Hussain, A. (2012). Towards teaching analytics: communication and negotiation tool (coneto). In Proceedings of the 7th Nordic Conference on Human-Computer Interaction: Making Sense Through Design . ACM, (pp. 775–776).
Vatrapu, R., Teplovs, C., Fujita, N., Bull, S. (2011). Towards visual analytics for teachers’ dynamic diagnostic pedagogical decision-making. In Proceedings of the 1st International Conference on Learning Analytics and Knowledge . ACM, (pp. 93–98).
Verbert, K., Duval, E., Klerkx, J., Govaerts, S., Santos, J.L. (2013). Learning analytics dashboard applications. American Behavioral Scientist , 57 (10), 1500–1509.
Voithofer, R., & Golan, A.M. (2018). 5. Data Sources for Educators, 1st edn.https://doi.org/10.4324/9780203728703-7, (p. 18).
Waller, M.A., & Fawcett, S.E. (2013). Data science, predictive analytics, and big data: a revolution that will transform supply chain design and management. Journal of Business Logistics , 34 (2), 77–84.
Wang, F., & Hannafin, M.J. (2005). Design-based research and technology-enhanced learning environments. Educational Technology Research and Development , 53 (4), 5–23.
Williamson, B. (2016). Digital education governance: data visualization, predictive analytics, and ’real-time’ policy instruments. Journal of Education Policy , 31 (2), 123–141.
Winkler, R., & Söllner, M. (2018). Unleashing the potential of chatbots in education: A state-of-the-art analysis. In Academy of Management Annual Meeting (AOM) . https://doi.org/10.5465/ambpp.2018.15903abstract .
Xu, B., & Recker, M. (2012). Teaching analytics: A clustering and triangulation study of digital library user data. Educational Technology & Society , 15 (3), 103–115.
Yigitbasioglu, O.M., & Velcu, O. (2012). A review of dashboards in performance management: Implications for design and research. International Journal of Accounting Information Systems , 13 (1), 41–59.
Download references
Acknowledgements
The research reported is part of an ongoing PhD research study in the area of Big Data Analytics in Higher Education. We also want to thank members of the Technology Enhanced Learning and Teaching (TELT) Committee of the University of Otago, New Zealand for support and for providing constructive feedback.
This research project was fully sponsored by Higher Education Development Centre, University of Otago, New Zealand.
Author information
Authors and affiliations.
Higher Education Development Centre, University of Otago, Dunedin, New Zealand
Ifeanyi Glory Ndukwe & Ben Kei Daniel
You can also search for this author in PubMed Google Scholar
Contributions
IGN conceived and presented the Conceptualisation of Teaching Analytics and Teachingv Outcome Model. BKD developed the Tripartite Approach that was utilised in this research. BKD encouraged IGN to perform a systematic review of teaching analytics that was guided by the Tripartite Approach. BKD supervised the findings of this work. IGN took the lead in writing the manuscript. All authors discussed the results, provided critical feedback and contributed to the final manuscript.
Corresponding author
Correspondence to Ifeanyi Glory Ndukwe .
Ethics declarations
Competing interests.
The authors declare that they have no competing interests. All authors have approved the manuscript and agree with its submission to the International Journal of Education Technology in Higher Education. This manuscript has not been published and is not under consideration for publication elsewhere.
Additional information
Publisher’s note.
Springer Nature remains neutral with regard to jurisdictional claims in published maps and institutional affiliations.
Rights and permissions
Open Access This article is licensed under a Creative Commons Attribution 4.0 International License, which permits use, sharing, adaptation, distribution and reproduction in any medium or format, as long as you give appropriate credit to the original author(s) and the source, provide a link to the Creative Commons licence, and indicate if changes were made. The images or other third party material in this article are included in the article’s Creative Commons licence, unless indicated otherwise in a credit line to the material. If material is not included in the article’s Creative Commons licence and your intended use is not permitted by statutory regulation or exceeds the permitted use, you will need to obtain permission directly from the copyright holder. To view a copy of this licence, visit http://creativecommons.org/licenses/by/4.0/ .
Reprints and permissions
About this article
Cite this article.
Ndukwe, I.G., Daniel, B.K. Teaching analytics, value and tools for teacher data literacy: a systematic and tripartite approach. Int J Educ Technol High Educ 17 , 22 (2020). https://doi.org/10.1186/s41239-020-00201-6
Download citation
Received : 29 October 2019
Accepted : 01 April 2020
Published : 22 June 2020
DOI : https://doi.org/10.1186/s41239-020-00201-6
Share this article
Anyone you share the following link with will be able to read this content:
Sorry, a shareable link is not currently available for this article.
Provided by the Springer Nature SharedIt content-sharing initiative

An official website of the United States government
The .gov means it’s official. Federal government websites often end in .gov or .mil. Before sharing sensitive information, make sure you’re on a federal government site.
The site is secure. The https:// ensures that you are connecting to the official website and that any information you provide is encrypted and transmitted securely.
- Publications
- Account settings
Preview improvements coming to the PMC website in October 2024. Learn More or Try it out now .
- Advanced Search
- Journal List
- MedEdPORTAL


A Guide to Basic Statistics for Educational Research
Donna m. windish.
1 Associate Professor of General Internal Medicine, Department of Internal Medicine, Yale School of Medicine; Director, Resident Research, Yale Primary Care Residency Program, Yale School of Medicine; Program Director, General Internal Medicine Medical Education Fellowship, Yale School of Medicine; Director, Advancement of Clinician-Educator Scholarship (ACES) Faculty Development Program, Department of Internal Medicine, Yale School of Medicine
Associated Data
- Guide to Basic Statistics for Educational Research.mp4
- Educational Examples Worksheet.doc
- Educational Examples Answer Sheet.doc
- Session Evaluation.docx
- Facilitator Guide.docx
All appendices are peer reviewed as integral parts of the Original Publication.
Introduction
Clinician-educators often need to produce scholarship for academic promotion. While some programs exist to help with faculty development skills, few provide adequate statistical training to help educators evaluate their work.
From January 2020 through January 2021, faculty at three academic centers attended one of five in-person or virtual seminars with dedicated statistical training for medical education interventions. These 90-minute seminars included a 45-minute PowerPoint presentation of common statistical tests used for educational interventions followed by small breakout groups to help attendees work on additional practice examples. After each seminar, surveys were distributed in person or virtually to obtain feedback.
Forty-three faculty attended the five seminars, with a range of surgical and nonsurgical specialties represented. Of these attendees, 38 (88%) completed session evaluations. The majority of respondents ( n = 34, 90%) rated the session as extremely useful in helping them know how to use statistics in their scholarly work. Most participants agreed or strongly agreed they had adequate time to practice skills ( n = 30, 79%). Self-rated confidence in using statistics was significantly higher after the session compared to before (3.00 post vs. 1.97 pre, p < .0001). Most participants ( n = 32, 84%) rated the session as excellent and the small-group practice as most useful ( n = 16, 42%), but many ( n = 26, 69%) wanted more skills practice.
This intervention shows that dedicated training on biostatistics used in educational interventions can help clinician-educators improve self-rated confidence and knowledge in choosing statistical tests in educational scholarship.
Educational Objectives
By the end of this activity, learners will be able to:
- 1. Describe the application of the following statistical areas to educational interventions: study designs, variable types, exploratory data analysis, confirmatory (inferential) data analysis, and basic interpretation of results.
- 2. Use a four-step approach to choosing a statistical test for educational cases.
Producing scholarship is often a key determinant to academic advancement for clinician-educators regardless of home institution. 1 – 3 The value of educational scholarship is well recognized and is receiving increased support. 4 To help clinician-educators cultivate faculty development skills, some institutions have established academies of medical educators 5 or education scholarship units. 6 Despite these initiatives, many institutions may not provide adequate statistical or methodological support to help with development and evaluation of educators' work. 3
A recent Association of American Medical Colleges survey showed that a majority of medical schools lack specific biostatistics training. 7 This lack of training can contribute to low statistical knowledge among resident trainees 8 – 11 and subsequently to low statistical literacy among faculty. 11 – 13 A recent scoping review of clinician-educator faculty development programs found that few programs focus on research or scholarship skills. 14 Without statistical knowledge, clinician-educators may be at a disadvantage in publishing their scholarly work and thus potentially miss opportunities to be promoted.
Resources exist that address understanding statistical concepts and evidence-based medicine. The JAMA Guide to Statistics and Medicine contains a series of articles addressing statistical techniques used in clinical research. 15 The goal of the series is to help clinicians understand and learn how to critically appraise the medical literature. One article in the series reviews the reporting guidelines for survey studies. 16 Since survey research is a common tool used in educational interventions, educators might find this particular article helpful in their work. A recent publication in MedEdPORTAL describes a module for teaching students basic biostatistics and evidence-based medicine. 17 The authors of that resource review study design strengths and weaknesses, how to appraise the literature, and how to assess the clinical importance of published studies. Another workshop in MedEdPORTAL contains an interactive review of basic biostatistics and discusses how to apply Bayes' theorem to testing and decision-making. 18 It uses a flipped classroom approach with quizzes to assess knowledge gained. Each of the three publications just described can aid educators in understanding basic statistical concepts, evidence-based medicine, and reading of the literature. None provide a dedicated guide that would aid educators in choosing statistical tests when analyzing their own educational interventions.
In 2006, Windish and Diener-West developed a guide to help clinician-educators understand and choose statistical tests. 19 Since then, little has been published that provides specific training on statistics for educational interventions with detailed examples. The resource presented here is a unique contribution to the literature aimed at building knowledge of biostatistics using educational examples that clinician-educators will find germane to their educational scholarship. The resource includes an instructional video identical to content presented in faculty development seminars across multiple institutions taught to medical educators. It also provides active learning opportunities through additional educational examples to practice and apply what has been learned from the video. This resource can be used as a seminar at other institutions in addition to serving as an everlasting resource for individuals when conducting educational research.
Five faculty development seminars were offered at three different schools of medicine from January 2020 through January 2021. Seminars were either in person or virtual via Zoom, with a range of six to 12 participants, and lasted 90 minutes. Each seminar was led by the author and included a 45-minute PowerPoint presentation that reviewed study designs, variable types, exploratory data analysis, confirmatory data analysis, basic interpretation of results, and a four-step approach to choosing a statistical test. 19 Statistical content was determined based on the low literacy regarding these concepts seen in prior studies of residents and educators. 8 – 13 A video of the PowerPoint presentation contained in this seminar is available in Appendix A . All figures in the presentation were created by the author using Stata statistical software version 14.2 (StataCorp) from fabricated data for illustrative purposes only. The photographs in the apple-pie analogy for regression analysis are author owned. In each seminar, statistical concepts were introduced and interwoven throughout the presentation using an example of an educational intervention aimed at improving second-year medical students' counseling skills, confidence in medical interviewing, professionalism skills, and pass rate. This example was designed to address how to evaluate a curriculum using different evaluation strategies, including the broad categories of assessing knowledge, attitudes, and skills. Statistical concepts included continuous, ordinal, and dichotomous outcome variables, parametric tests, nonparametric tests, and paired analyses.
After the PowerPoint presentation, faculty divided into smaller groups of two to five people who worked together for 20 minutes on additional practice examples provided on worksheets ( Appendix B ). This small-group practice allowed participants to apply the statistical knowledge learned in the presentation. All figures in the worksheets were created by the author from manufactured data and used for illustrative purposes. Half of the small groups completed questions from case 1, and the other half completed questions from case 2. Case 1 addressed the following statistical concepts: Student t test, correlation, and multiple logistic regression. Case 2 had participants work through examples that used a paired t test and analysis of variance. The last 15 minutes of the seminar featured a debrief of the practice examples with answers provided in the larger group ( Appendix C ).
Seminars were held at the Yale School of Medicine, the Washington University School of Medicine in St. Louis, and the University of Wisconsin School of Medicine and Public Health. The two sessions held at Yale were hosted by the Department of Medicine and were open to all faculty in the department, with one in-person session and one virtual session. The two in-person sessions held at the Washington University in St. Louis were hosted by the Academy of Educators and were open to all faculty in any discipline throughout the university. One seminar was hosted virtually for the University of Wisconsin and was open to educators in graduate and undergraduate medical education.
At the end of each seminar, faculty were asked to complete a session evaluation ( Appendix D ). Questions asked participants to rate the following:
- 1. The usefulness of the session in helping know them how to use statistics in their current scholarly work (5-point scale: 1 = extremely useful, 5 = extremely useless ),
- 2. The adequacy of the faculty facilitator (5-point scale: 1 = extremely adequate, 5 = extremely inadequate ),
- 3. The adequacy of time to practice skills (5-point scale: 1 = strongly disagree, 5 = strongly agree ),
- 4. Their confidence in using statistics before and after the seminar (4-point scale: 1 = very unconfident, 4 = very confident ), and
- 5. The overall session (5-point scale: 1 = poor, 5 = excellent ).
Open-ended questions were also asked to elucidate the most useful part of the session and recommendations for change. A paired t test was used to compare self-rated confidence before and after the session.
Appendix E is a facilitator guide providing a step-by-step approach to replicating the previously described seminar. It features information on how to develop a successful session, including what to do prior to the session, how to use the video in Appendix A , how to execute the small-group breakout session, and how to review the answers to the small-group work. The video can be stopped at various times to discuss each area covered. Educational and statistical topics with corresponding video start times are as follows:
- • Start time: 0:03:02—educational example question 1: counseling skills.
- • Start time: 0:03:25—study designs.
- • Start time: 0:04:43—paired data versus unpaired data.
- • Start time: 0:07:40—types of outcome research variables.
- • Start time: 0:11:31—exploratory data analysis.
- • Start time: 0:14:27—parametric tests.
- • Start time: 0:16:06—nonparametric tests.
- • Start time: 0:17:55—confirmatory data analysis.
- • Start time: 0:18:46—regression analysis.
- • Start time: 0:25:34—hypothesis testing.
- • Start time: 0:29:30—educational example question 2: confidence in skills.
- • Start time: 0:32:33—educational example question 3: professionalism skills.
- • Start time: 0:35:25—educational example question 4: pass rate.
- • Start time: 0:36:01—dichotomous outcomes.
In total, 43 faculty attended the five sessions, with a range of surgical and nonsurgical specialties represented, including general surgery, orthopedics, radiology, internal medicine, nephrology, pulmonology, cardiology, geriatrics, gastroenterology, ophthalmology, pediatrics, physical therapy, genomics, psychiatry, pathology, and dermatology. Of the 43 faculty, 38 (88%) completed session evaluations. Most respondents ( n = 34, 90%) rated the session as extremely useful in helping them know how to use statistics in their current scholarly work. All 38 respondents (100%) rated the facilitator as extremely adequate at teaching the statistical concepts. Most participants agreed or strongly agreed that they had adequate time to practice skills in the small-group breakout session ( n = 30, 79%). Self-rated confidence in using statistics was significantly higher after the session compared to before (3.00 post vs. 1.97 pre, p < .0001). Most participants ( n = 32, 84%) rated the session as excellent, with the remainder rating it as very good ( n = 6, 16%).
All respondents provided at least one comment on the most useful part of the session. The majority of comments listed the small-group practice as most useful ( n = 16, 42% of all comments), followed by the usefulness of the flowcharts to determine which statistical test to use ( n = 14, 36%). Other faculty felt the session helped demystify statistics ( n = 7, 18%) or found the descriptions of when to use statistical tests most useful ( n = 2, 5%).
Thirty-two faculty (84%) provided recommendations for change. Having more examples ( n = 12, 38%) and more time for practice ( n = 10, 31%) were the top two recommendations. Remaining suggestions for change included providing an opportunity for hands-on practice with statistical software ( n = 4, 12%) and having a specific slide that included a link to the Windish and Diener-West reference 19 ( n = 3, 9%).
This educational seminar shows that dedicated training on statistics using educational interventions can provide guidance to clinician-educators in conducting and analyzing their work. The seminars were well received by faculty across a spectrum of specialties, disciplines, and institutions, with universal agreement regarding the sessions' usefulness in helping participants in their educational scholarly work. The versatility of the seminars was also demonstrated as they were done both in person and virtually.
In thinking about lessons learned, I realize that although the educational examples worksheet ( Appendix B ) allows participants to practice with additional examples, some faculty may wish to practice and obtain feedback using their own scholarship or might want a review of how to employ statistical software. I initially contemplated having such activities as part of the seminar but feel that participants need time to digest the material and think about how to apply what they have learned to their own work. Consequently, to help solidify the concepts, in the future I will be offering an optional follow-up 90-minute session a few weeks after each seminar. One goal of this additional session will be to provide feedback to any participant who wants to demonstrate how they have used the statistical approach taught with their own educational scholarship. Faculty will be asked to use the last page of the educational examples worksheet to guide them in their own initiatives and in presenting their thought process. Another goal of this follow-up session will be to review how to utilize SPSS and Microsoft Excel for statistical analyses using participant data. I have chosen these two platforms as they contain easy-to-use analytic software. If faculty do not have their own data to analyze, I will provide a small sample educational database for them to work through.
Certain limitations to this method of training should be considered. First, the detailed video may not answer all questions that viewers have on statistics despite multiple examples and practice. While the video is comprehensive and covers many statistical tests that clinician-educators can use, it does not cover all possible statistical tests, qualitative assessment, curriculum development, or how to choose evaluation instruments. Some of these limitations can be offset by using Appendix 1 in the Windish and Diener-West article 19 and textbooks that address curriculum development 20 and educational research. 21 In addition, some of the original wording of the evaluation tool may not have captured all participants' opinions. Thus, changes to the session evaluation ( Appendix D ) have been made. Question 2 now reads, “How would you rate the Statistics facilitator in presenting the content materials covered?” Question 4 now asks, “Was there a part of the Statistics session that was most useful for you? If so, please describe what and why.” This is in place of asking what part of the seminar the participant felt was most useful. Questions 6 and 7, which rate participant confidence, now contain a neutral category to be consistent with other questions featuring 5-point Likert-scale responses. Finally, given the immediate-post design of the session evaluation, it is unclear if the seminars foster actual long-term improvement in subsequent clinician-educator work.
The video and educational examples provided here can be used as a lasting reference for dedicated teaching and practice of evaluating educational initiatives. This resource can be used by institutions that need ways to help their faculty in their educational scholarship pursuits or by individuals who need a guide in analyzing their work. With more faculty reviewing these materials and using them in their educational work, more long-term outcomes can be assessed.
Disclosures
None to report.
Funding/Support
Ethical approval.
Reported as not applicable.
Imperial College London Imperial College London
Latest news.

Imperial academic wins €2.4m European funding to improve solar harvesting tech

Meningococcal vaccine is cost effective at protecting men against gonorrhoea

Shape-shifting cancer cell discovery reveals potential skin cancer drug targets
- Educational Development Unit
- Teaching toolkit
- Educational research methods
- Analysing and writing up your research
Types of data analysis
The means by which you analyse your data are largely determined by the nature of your research question , the approach and paradigm within which your research operates, the methods used, and consequently the type of data elicited. In turn, the language and terms you use in both conducting and reporting your data analysis should reflect these.
The list below includes some of the more commonly used means of qualitative data analysis in educational research – although this is by no means exhaustive. It is also important to point out that each of the terms given below generally encompass a range of possible methods or options and there can be overlap between them. In all cases, further reading is essential to ensure that the process of data analysis is valid, transparent and appropriately systematic, and we have provided below (as well as in our further resources and tools and resources for qualitative data analysis sections) some recommendations for this.
If your research is likely to involve quantitative analysis, we recommend the books listed below.
Types of qualitative data analysis
- Thematic analysis
- Coding and/or content analysis
- Concept map analysis
- Discourse or narrative analysis
- Grouded theory
- Phenomenological analysis or interpretative phenomenological analysis (IPA)
Further reading and resources
As a starting point for most of these, we would recommend the relevant chapter from Part 5 of Cohen, Manion and Morrison (2018), Research Methods in Education. You may also find the following helpful:
For qualitative approaches
Savin-Baden, M. & Howell Major, C. (2013) Data analysis. In Qualitative Research: The essential guide to theory and practice . (Abingdon, Routledge, pp. 434-450).
For quantitative approaches
Bors, D. (2018) Data analysis for the social sciences (Sage, London).
Data Analysis for Educational Research in R
Joshua m. rosenberg, chapter 1 introduction, 1.1 background: data analysis in educational research.
Educational research is hard to do (Berliner, 2002). This is because many educational phenomena are part of a complex system, with multiple, nested levels, and, well, people, many of them developing. Data analysis in education reflects some of the challenges of educational research writ large. In short, both educational research and analysis of educational data is hard. The goal of this book is to share how to make these difficulties less challenging using R, the open-source, free programming language and software.
1.2 Why a book on data analysis in R for educational research
There are at least three reasons why data analysis in educational research is hard:
Educational researchers have unique methods: an emphasis on multi-level models, networks, and measurement are just some examples.
Educational researchers face unique challenges: coming from myriad backgrounds, and working in fields with greater or lesser emphases on different aspects of data analysis.
Finally, there are training challenges. Educational research features some great methodologists: Many advances in the fields mentioned earlier in this session have been made by those working primarily in educational research. Nevertheless, few quantitative classes teach data analysis.
- Open access
- Published: 15 April 2024
Medical, dental, and nursing students’ attitudes and knowledge towards artificial intelligence: a systematic review and meta-analysis
- Hamidreza Amiri 1 na1 ,
- Samira Peiravi 2 na1 ,
- Seyedeh sara rezazadeh shojaee 3 na1 ,
- Motahareh Rouhparvarzamin 4 ,
- Mohammad Naser Nateghi 5 ,
- Mohammad Hossein Etemadi 6 ,
- Mahdie ShojaeiBaghini 7 ,
- Farhan Musaie 8 ,
- Mohammad Hossein Anvari 9 &
- Mahsa Asadi Anar 10
BMC Medical Education volume 24 , Article number: 412 ( 2024 ) Cite this article
197 Accesses
1 Altmetric
Metrics details
Nowadays, Artificial intelligence (AI) is one of the most popular topics that can be integrated into healthcare activities. Currently, AI is used in specialized fields such as radiology, pathology, and ophthalmology. Despite the advantages of AI, the fear of human labor being replaced by this technology makes some students reluctant to choose specific fields. This meta-analysis aims to investigate the knowledge and attitude of medical, dental, and nursing students and experts in this field about AI and its application.
This study was designed based on PRISMA guidelines. PubMed, Scopus, and Google Scholar databases were searched with relevant keywords. After study selection according to inclusion criteria, data of knowledge and attitude were extracted for meta-analysis.
Twenty-two studies included 8491 participants were included in this meta-analysis. The pooled analysis revealed a proportion of 0.44 (95%CI = [0.34, 0.54], P < 0.01, I2 = 98.95%) for knowledge. Moreover, the proportion of attitude was 0.65 (95%CI = [0.55, 0.75], P < 0.01, I2 = 99.47%). The studies did not show any publication bias with a symmetrical funnel plot.
Average levels of knowledge indicate the necessity of including relevant educational programs in the student’s academic curriculum. The positive attitude of students promises the acceptance of AI technology. However, dealing with ethics education in AI and the aspects of human-AI cooperation are discussed. Future longitudinal studies could follow students to provide more data to guide how AI can be incorporated into education.
Peer Review reports
Introduction
The term "artificial intelligence (AI)" was coined nearly 70 years ago to refer to using of computers to imitate human reasoning [ 1 ]. The first application of AI was in mathematics in 1956 when it was utilized for proving theorems [ 2 ]. Integrating of AI in medicine was a gradual process [ 3 ] that began with the development of a software program that guided doctors on appropriate antimicrobial therapy [ 4 ].
AI is a trending topic that is currently at the forefront of technological advancements [ 5 ] and has the potential to influence the healthcare industry significantly [ 6 ]. The term AI refers to a scientific and engineering discipline that deals with developing computer-based systems capable of exhibiting intelligent behavior, as well as understanding and replicating human-like cognitive processes [ 7 ]. Recent advancements in computer and informatics technologies have paved the way for integrating of AI technologies, such as machine learning and deep learning, into healthcare information systems [ 8 , 9 ]. AI has been extensively integrated into decision support systems (DSSs) in data-intensive medical specialties like radiology, pathology, and ophthalmology [ 10 ].
Several experts have expressed their opinions on the future of radiology in light of AI's emergence [ 11 , 12 ]. Radiological societies have also published white papers promoting their views [ 13 , 14 ]. Studies have indicated that medical students do not express significant concern or fear about being replaced by AI in their profession [ 15 ]. However, some students may experience anxiety related to the possibility of being displaced by AI, which may discourage them from considering certain medical specialties [ 16 ]. Indeed, there are positive and negative perspectives on the impact of AI on daily human life. Pessimistic views suggest that AI may replace humans in various sectors. On the other hand, optimistic views highlight that individuals with AI support will have increased opportunities to leverage future advancements [ 17 ]. To the best of our knowledge, this study aimed to evaluate the attitudes, knowledge, and skills of medical, dental, and nursing students toward AI and to gather information about their opinions on the use of AI.
This systematic review and meta-analysis study was based on the PRISMA (Preferred Reporting Items for Systematic Reviews and Meta-Analysis) guidelines. The protocol of this study was registered on PROSPERO with the ID of CRD42024521006.
Literature search
A structured literature search was applied up to 12th September 2023 to collect appropriate articles from PubMed /MEDLINE, Scopus, and Google Scholar databases. Search tactics included two main subgroups of keywords. One subgroup was the concepts related to artificial intelligence, and the other group was the perspective of health care and dentists; then, Subgroups were mixed by using ‘AND.’ More specifically, we searched the above databases for (artificial intelligence or machine learning) and (Medical or dentistry or nursing) (Table 1 ). The search process was done according to the query options of each database. In addition, we searched the reference lists of appropriate systematic reviews to prevent missing data. Two reviewers accomplished all strategies in a solitary state, and any controversy between the reviewers was resolved by negotiation.
Criteria for selecting studies
The main goal was to evaluate the attitudes of students and graduates working in dentistry, nursing, or medical (health care providers) fields toward AI and machine learning. We didn't use any restrictions on date and language, but to make the search more specific, we restricted the keyword search to the title. Articles with irrelevant subject matter and studies utilizing animal models were excluded during the initial phase of document selection. Additionally, duplicate documents were eliminated.
Data extraction and study quality assessment
Two reviewers independently assessed the title and abstract of each study to ascertain its suitability for inclusion in this meta-analysis. We excluded studies that didn’t fulfill our criteria. The complete text of the remaining studies was reviewed, and studies that met the criteria were included in the data extraction step. After that, the subsequent items were acquired for extraction and divided into four sets:
1. Study characteristics include authors, type of study, year, location, and follow-up duration.
2. Participant variables (average age, gender).
3. Research Methodology (e.g., participant sample size).
4. Results and outcomes (the attitude, knowledge, and skill toward artificial intelligence).
Two previously mentioned reviewers utilized the critical appraisal checklists for cohort, case–control, and analytical cross-sectional studies created by the Joanna Briggs Institute (JBI). The checklists can be found at the following website: https://jbi.global/critical-appraisal-tools . If there were any inconsistencies, a third author was involved in the process.
Statistical analysis
Our data analysis was conducted using the STATA 13.1 software developed by StataCorp LP in College Station, TX, USA. The findings were presented as combined odds ratios (ORs) and a 95% confidence interval displayed in a forest plot. Heterogeneity among the eligible studies was assessed using the I2 statistic. The random effects model was employed when significant heterogeneity was observed (I2 > 50%). In addition, we performed a sensitivity analysis by systematically excluding one study at a time and repeating the meta-analysis. This allowed us to guarantee the consistency of our conclusions. To assess the possibility of publication bias, we visually examined the symmetry of the funnel plot and conducted Egger’s regression analysis.
Search strategy
We obtained 2426 from PubMed/MEDLINE, Scopus, and Google Scholar in the initial search. Seventeen studies were found by manual search. After the automatic removal of duplicated reports, 2292 studies remained. Two thousand sixty-five studies were excluded in the title and abstract evaluation. Two hundred twenty-seven remaining studies underwent additional assessment through full-text, causing 205 papers to be excluded due to ineligibility to inclusion criteria. Finally, 22 studies were included in this systematic review and meta-analysis (Fig. 1 ).
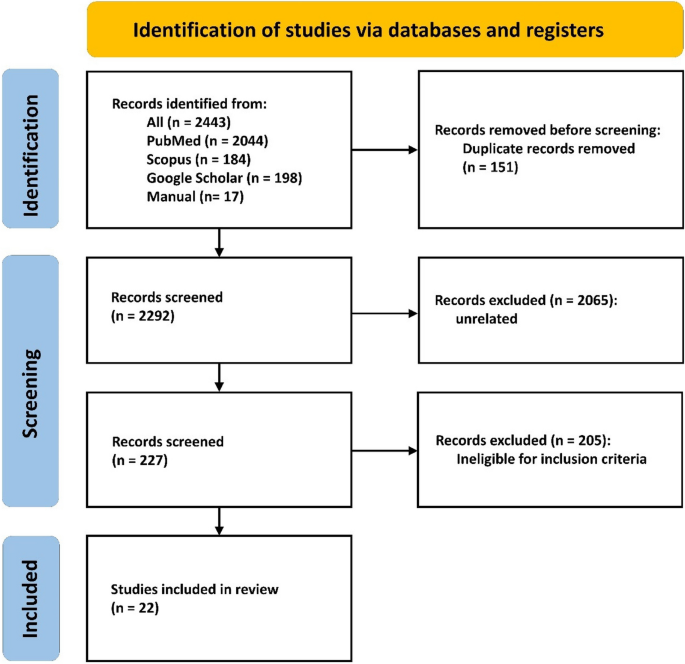
Prisma diagram for study selection process in this study
Baseline characteristic
This systematic review and meta-analysis evaluated the attitude, knowledge, and skills of medical, dental, and nursing students toward artificial intelligence. We included 22 original articles published from 2020–2023. These studies were performed in several countries, including the U.S.A [ 18 ], Germany [ 19 , 20 ], Lebanon [ 21 ], Pakistan [ 22 ], Canada [ 23 ], The U.K. [ 24 ], United Arab Emirates [ 25 ], Nigeria [ 26 ], Turkey [ 27 , 28 ], Spain [ 29 ] Saudi Arabia [ 30 ], India [ 31 , 32 , 33 ], Egypt [ 34 ], Peru [ 35 ], Nepal [ 36 ], Kuwait [ 37 ], Syria [ 38 ], and multiple countries [ 39 ]. The study design of 19 studies was cross-sectional [ 20 , 21 , 22 , 23 , 24 , 25 , 26 , 27 , 28 , 29 , 30 , 31 , 32 , 33 , 34 , 35 , 36 , 37 , 38 ], and the rest followed a mixed methodology [ 18 , 19 , 39 ]. This study included 8491 participants, with a mean age of 19–30 years (Table 2 ).
We performed a meta-analysis on 22 studies for attitude of students toward AI. The proportion for attitude was 0.65 (95%CI = [0.55, 0.75], P < 0.01) according to 22 studies. This means that 65% of all students were agree with the use of AI in medicine and had a favorable view. Similarly, the heterogeneity was severe with I2 of 99.47%, and H2 = 189.47 (Fig. 2 ).
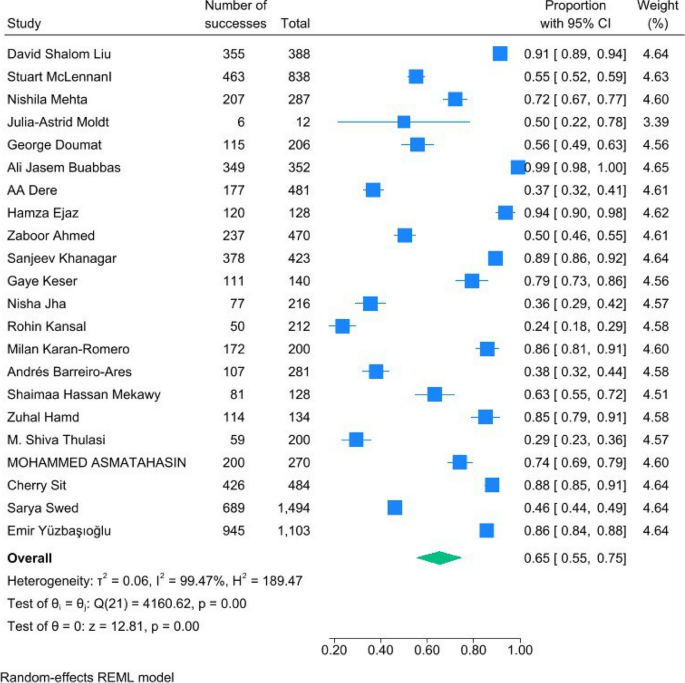
Forest plot of proportion of attitude showed a significant effect of 0.44 (0.34, 0.54)
In comparison between various countries, students in the U.S.A. Kuwait, Saudi Arabia, Turkey, and England showed a higher rate of attitude toward AI than those in Germany, Lebanon, Nigeria, Pakistan, and India. Additionally, the Attitudes of Spanish and United Arab Emirates students varied in different studies. Finally, students in Canada and Egypt displayed a medium rate of positive attitude (Fig. 3 ).
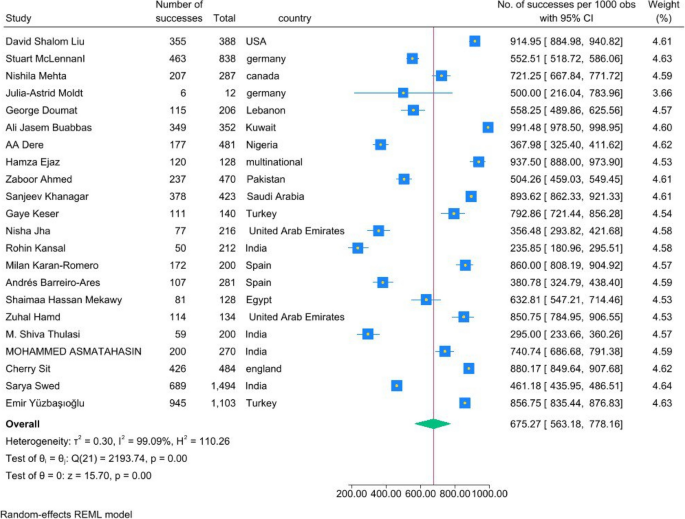
Forest plot for comparing countries in terms of their students' attitudes toward AI
A total of 17 studies had provided the knowledge data. The pooled analysis showed a proportion for knowledge of 0.44 (95%CI = [0.34, 0.54], P < 0.01). This shows that 44% of the total population of included students had a relatively good knowledge about AI, either in the field of theory or practical. The studies showed a high heterogeneity with an I2 of 98.95% and H2 of 93.35 (Fig. 4 ).
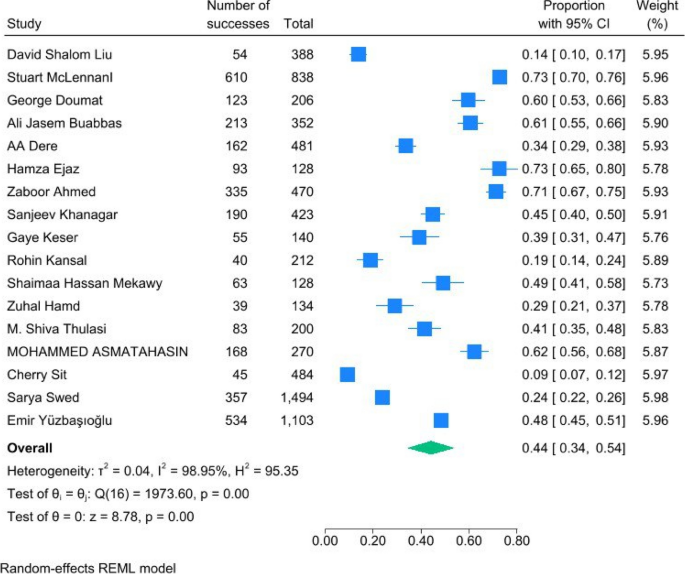
Forest plot of proportion of knowledge showed a significant effect of 0.65 (0.55, 0.75)
Students from Germany, Lebanon, Kuwait, and Pakistan had higher levels of knowledge in the field of AI. In contrast, students from the U.S.A., Nigeria, the United Arab Emirates, and England showed a relatively lower knowledge level. Additionally, the level of knowledge in Indian students varied across different studies. Finally, students from Egypt, Saudi Arabia, and Turkey showed moderate knowledge (Fig. 5 ).
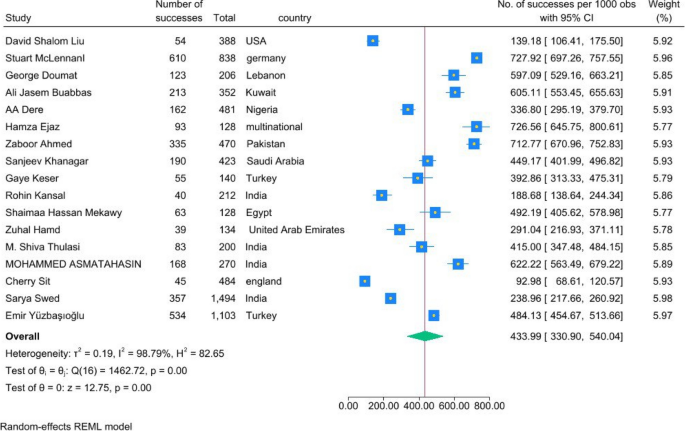
Forest plot for comparing countries in terms of their students' knowledge of AI
Publication bias
The publication bias was evaluated through the funnel plot and Egger’s test. The funnel plot (Fig. 6 ) showed a symmetrical pattern, indicating no publication bias. This was supported by Egger’s test result ( P = 0.75).
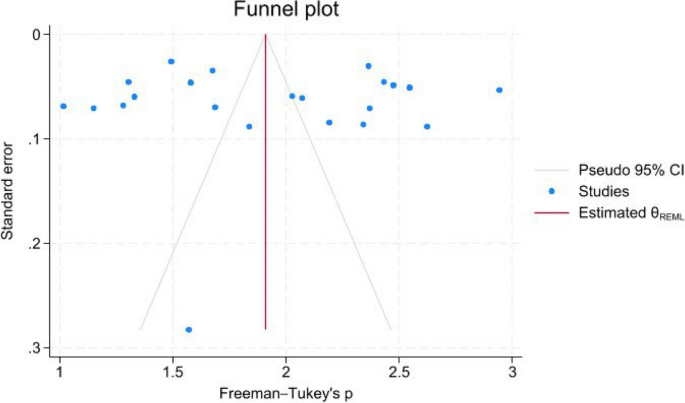
Funnel plot of included studies showed a symmetrical pattern including no publication bias (Egger’s test P -value = 0.75)
This systematic review and meta-analysis aimed to provide evidence on medical, dental, and nursing students’ attitudes, knowledge, and skills regarding AI. Across 24 studies with 5789 participants, students demonstrated moderate knowledge but generally positive attitudes towards AI.
Overall, 44% of students exhibited medium to high knowledge of AI principles and applications. Knowledge encompassed theoretical understanding of AI algorithms, practical abilities to implement AI systems, and programming proficiency. However, the majority of students had limited AI knowledge. This knowledge gap signals an urgent need to incorporate comprehensive AI education into healthcare curricula. Studies show that students support this idea [ 40 , 41 ]. Curricula should cover foundational concepts like machine learning and neural networks as well as applied skills in utilizing AI tools for tasks like diagnostic imaging interpretation. Hands-on experiential learning with real-world case examples could prove highly effective. Other reason is that lack of knowledge is an important barrier to the use of AI [ 42 ]. Notably, students from developed countries demonstrated greater AI knowledge than peers in developing nations. This has been shown in previous studies as well [ 43 ]. This discrepancy highlights concerning global digital divides in accessing AI skills training. Targeted investments and capacity building programs are critical to ensuring students worldwide can gain applied AI competencies.
In contrast to their variable knowledge, 65% of students expressed positive attitudes regarding AI utilization in education and clinical practice. This was also showed in previous studies that most of healthcare students have a positive attitude towards AI [ 19 , 44 , 45 , 46 , 47 ]. Students recognized potential benefits of AI for enhancing diagnostic accuracy, improving healthcare access, and relieving clinical workloads. In contrast there are negative perceptions too [ 44 , 48 , 49 ].
Attitudinal measures had substantial heterogeneity, reflecting divergent perceptions across student subgroups. In particular, developing world students held more skeptical views, fearing AI could dehumanize care or render healthcare jobs obsolete. Curricula must address these valid ethical and social concerns through discussions of AI bias, transparency, and impacts on healthcare roles. It should be noted that patient privacy and autonomy, informed consent, transparency, equality and biases are some of major concerns [ 50 ]. Refining attitudinal measures with more granular subsets and exploring predictors of AI acceptance would further inform targeted educational initiatives based on students’ specific concerns.
Enthusiasm and optimism vs. expertise gaps
Overall students showed enthusiasm and optimism about AI's role in medicine, yet the majority lacked substantial expertise and practical abilities in utilizing AI technology. A similar pattern exists in other majors too. A study by Busch et al. involving 387 pharmacy students from 12 countries found that 58% of students held positive attitudes towards AI in medicine, while 63% reported limited general knowledge of AI [ 51 ]. Bridging these attitude-knowledge gaps represents a key challenge for AI readiness. Curricula must not only transfer technical knowledge but also address values, ethics, and societal impacts. Education should emphasize AI as a collaborative tool to augment human capabilities rather than replace them. Again, having students directly experience AI’s benefits for care quality could show its potential for enhancing work rather than displacing workers. Additionally, equitable access to AI upskilling is imperative, particularly for students from disadvantaged regions who may have heightened concerns about AI’s risks.
Strength and limitations
The strength of our study is the review of articles from three large databases, including PubMed, Scopus, and Google Scholar. Also, we used the random effect model to ensure the robustness of the results. Also, our study had some limitations. We included only studies in English. In addition, most of the included studies used their own questionnaires to evaluate the knowledge and approach of the participants toward artificial intelligence. Finally, it is necessary to mention that there were not enough studies to extract the skill results and perform a meta-analysis.
Future research directions
Future research should investigate the long-term knowledge and attitudinal trajectories of students after graduation. As AI becomes further embedded into real-world practice, how do provider perspectives evolve? Do knowledge gaps persist or does on-the-job exposure improve understanding? How do early attitudinal concerns translate to technology adoption patterns? Longitudinal data tracking cohorts of students into practice could provide pivotal insights to guide continuing education and change management interventions.
Follow-up studies should also assess the durability of AI skills training. Can one-time education produce lasting competencies or is ongoing reinforcement needed? Comparisons of different pedagogical approaches for AI instruction could illuminate best practices as well. And crucially, future work must evaluate links from AI education to concrete improvements in clinical processes and patient outcomes. Demonstrating benefits to care quality represents the strongest incentive for curriculum reform.
AI is rapidly transforming healthcare and medical education. However, the extent to which healthcare students are prepared for this transformation remains unclear. The moderate knowledge levels indicate substantial room for improvement through curricular enhancement. Hands-on experiential learning focused on applied AI skills shows promise for durably improving competencies. Positive baseline attitudes bode well for acceptance, but targeted education around AI ethics, impacts, and human-AI collaboration will be key to realizing this potential.
Important gaps remain in understanding long-term knowledge retention, optimal pedagogies, impacts of improved education on clinical processes and outcomes, and equitable global access. Follow-up longitudinal studies tracking cohorts of students into practice could offer pivotal data to guide continuing education. Comparisons of instructional approaches may illuminate best practices.
Availability of data and materials
The datasets generated and analyzed during the current study are not publicly available but are available from the corresponding author on reasonable request.
Abbreviations
- Artificial intelligence
Larentzakis A, Lygeros N. Artificial intelligence (AI) in medicine as a strategic valuable tool. Pan Afr Med J. 2021;38:184.
Article Google Scholar
Russell S, Norvig P. Artificial Intelligence, A Modern Approach. Second Edition. 2003. p 1081.
Kaul V, Enslin S, Gross SA. History of artificial intelligence in medicine. Gastrointest Endosc. 2020;92(4):807–12.
Shortliffe EH, et al. Computer-based consultations in clinical therapeutics: Explanation and rule acquisition capabilities of the MYCIN system. Comput Biomed Res. 1975;8(4):303–20.
Morris K, Schlenoff C, Srinivasan V. A Remarkable Resurgence of Artificial Intelligence and Its Impact on Automation and Autonomy. IEEE Trans Autom Sci Eng. 2017;14:407–9.
Hinton G. Deep Learning-A Technology With the Potential to Transform Health Care. JAMA. 2018;320(11):1101–2.
Shapiro, S.C., Artificial intelligence (AI), in Encyclopedia of Computer Science. 2003, John Wiley and Sons Ltd. p. 89–93.
Miotto R, et al. Deep learning for healthcare: review, opportunities and challenges. Brief Bioinform. 2018;19(6):1236–46.
Xiao C, Choi E, Sun J. Opportunities and challenges in developing deep learning models using electronic health records data: a systematic review. J Am Med Inform Assoc. 2018;25(10):1419–28.
Yu KH, Kohane IS. Framing the challenges of artificial intelligence in medicine. BMJ Qual Saf. 2019;28(3):238–41.
Moehrle A. “Radiology” Is Going Away . . . and That’s Okay: Titles Change, A Profession Evolves. J Am Coll Radiol. 2018;15(3):499–500.
Schier R. Artificial Intelligence and the Practice of Radiology: An Alternative View. J Am Coll Radiol. 2018;15(7):1004–7.
Beregi JP, et al. Radiology and artificial intelligence: An opportunity for our specialty. Diagn Interv Imaging. 2018;99(11):677–8.
Tang A, et al. Canadian Association of Radiologists White Paper on Artificial Intelligence in Radiology. Can Assoc Radiol J. 2018;69(2):120–35.
Pinto Dos Santos D, et al. Medical students’ attitude towards artificial intelligence: a multicentre survey. Eur Radiol. 2019;29(4):1640–6.
Gong B, et al. Influence of Artificial Intelligence on Canadian Medical Students’ Preference for Radiology Specialty: ANational Survey Study. Acad Radiol. 2019;26(4):566–77.
Johnston SC. Anticipating and Training the Physician of the Future: The Importance of Caring in an Age of Artificial Intelligence. Acad Med. 2018;93(8):1105–6.
Liu DS, et al. Perceptions of US medical students on artificial intelligence in medicine: mixed methods survey study. JMIR Medical Education. 2022;8(4): e38325.
Moldt J-A, et al. Chatbots for future docs: exploring medical students’ attitudes and knowledge towards artificial intelligence and medical chatbots. Med Educ Online. 2023;28(1):2182659.
McLennan S, et al. German medical students´ views regarding artificial intelligence in medicine: A cross-sectional survey. PLOS Digital Health. 2022;1(10): e0000114.
Doumat G, et al. Knowledge and attitudes of medical students in Lebanon toward artificial intelligence: A national survey study. Frontiers in artificial intelligence. 2022;5:1015418.
Ahmed Z, et al. Knowledge, attitude, and practice of artificial intelligence among doctors and medical students in Pakistan: A cross-sectional online survey. Annals of Medicine and Surgery. 2022;76: 103493.
Mehta N, et al. Knowledge and Attitudes on Artificial Intelligence in Healthcare: A Provincial Survey Study of Medical Students. MedEdPublish. 2021;10:75.
Sit C, et al. Attitudes and perceptions of UK medical students towards artificial intelligence and radiology: a multicentre survey. Insights Imaging. 2020;11:1–6.
Hamd Z, et al. A closer look at the current knowledge and prospects of artificial intelligence integration in dentistry practice: A cross-sectional study. Heliyon. 2023;9(6):e17089.
Dere, A.A., Knowledge and Attitude of Medical Students and Doctors towards Artificial Intelligence: A study of University of Ilorin. 2023.
Yüzbaşıoğlu E. Attitudes and perceptions of dental students towards artificial intelligence. J Dent Educ. 2021;85(1):60–8.
Keser G, PEKİNER F.M.N. Attitudes, perceptions and knowledge regarding the future of artificial intelligence in oral radiology among a group of dental students in Turkey: a survey. Clinical and Experimental Health Sciences. 2021;11(4):637–41.
Barreiro-Ares A, et al. Impact of the rise of artificial intelligence in radiology: what do students think? Int J Environ Res Public Health. 2023;20(2):1589.
Khanagar S, et al. Knowledge, attitudes, and perceptions of dental students towards artificial intelligence in Riyadh. Saudi Arabia Med Sci. 2021;25(114):1857–67.
Google Scholar
Kansal R, et al. Differences in knowledge and perspectives on the usage of artificial intelligence among doctors and medical students of a developing country: a cross-sectional study. Cureus. 2022;14(1):e21434.
Asmatahasin M, et al. Attitude and perception of dental students towards artificial intelligence. Indian Journal of Basic and Applied Medical Research. 2021;10(3):305–14.
Thulasi MS, et al. Knowledge attitude and practices of dental students and dental practitioners towards artificial intelligence. International Journal of Intelligent Systems and Applications in Engineering. 2022;10(1s):248–53.
Hassan Mekawy S, Ali Mohamed Ismail S, Zayed Mohamed M. Digital Health Literacy (DHL) Levels Among Nursing Baccalaureate Students And Their Perception And Attitudes Toward The Application Of Artificial Intelligence (AI) In Nursing. Egyptian Journal of Health Care. 2020;11(1):1266–77.
Karan-Romero M, Salazar-Gamarra RE, Leon-Rios XA. Evaluation of Attitudes and Perceptions in Students about the Use of Artificial Intelligence in Dentistry. Dentistry Journal. 2023;11(5):125.
Jha N, et al. Undergraduate medical Students’ and Interns’ knowledge and perception of artificial intelligence in medicine. Adv Med Educ Pract. 2022;(13):927–37.
Buabbas, A.J., et al. Investigating Students’ Perceptions towards Artificial Intelligence in Medical Education. in Healthcare. 2023. MDPI.
Swed S, et al. Knowledge, attitude, and practice of artificial intelligence among doctors and medical students in Syria: a cross-sectional online survey. Frontiers in artificial intelligence. 2022;5:1011524.
Ejaz H, et al. Artificial intelligence and medical education: A global mixed-methods study of medical students’ perspectives. Digital Health. 2022;8:20552076221089100.
Banerjee M, et al. The impact of artificial intelligence on clinical education: perceptions of postgraduate trainee doctors in London (UK) and recommendations for trainers. BMC Med Educ. 2021;21(1):429.
Pupic N, et al. An evidence-based approach to artificial intelligence education for medical students: A systematic review. PLOS Digit Health. 2023;2(11): e0000255.
Singh N, et al. Attitude, perception and barriers of dental professionals towards artificial intelligence. J Oral Biol Craniofac Res. 2023;13(5):584–8.
Mousavi Baigi SF, et al. Attitudes, knowledge, and skills towards artificial intelligence among healthcare students: A systematic review. Health Sci Rep. 2023;6(3): e1138.
AlZaabi A, AlMaskari S, AalAbdulsalam A. Are physicians and medical students ready for artificial intelligence applications in healthcare? DIGITAL HEALTH. 2023;9:20552076231152170.
Chen, M., et al., Acceptance of clinical artificial intelligence among physicians and medical students: A systematic review with cross-sectional survey. Frontiers in Medicine, 2022. 9.
Gillissen A, Kochanek T, Zupanic M, Ehlers E. "Medical students’ perceptions towards digitization and artificial intelligence: a mixed-methods study." Healthcare. 2022;10(4):723.
Karan-Romero M, Salazar-Gamarra RE,Leon-Rios XA. Evaluation of attitudes and perceptions in students about the use of artificial intelligence in dentistry. Dent J. 2023;11(5):125.
Sheela J. Attitude of Nursing Students towards Artificial Intelligence. Int J Sci Healthc Res. 2022;7(2):344-347.
Jussupow E, Spohrer K, Heinzl A. Identity Threats as a Reason for Resistance to Artificial Intelligence: Survey Study With Medical Students and Professionals. JMIR Form Res. 2022;6(3): e28750.
Prakash S, et al. Ethical Conundrums in the Application of Artificial Intelligence (AI) in Healthcare-A Scoping Review of Reviews. J Pers Med. 2022;12(11):1914.
Busch F, et al. International pharmacy students' perceptions towards artificial intelligence in medicine-A multinational, multicentre cross-sectional study. Br J Clin Pharmacol. 2023;90(3):649–61.
Liu DS, et al. Perceptions of US Medical Students on Artificial Intelligence in Medicine: Mixed Methods Survey Study. JMIR Med Educ. 2022;8(4): e38325.
McLennan S, et al. German medical students´ views regarding artificial intelligence in medicine: A cross-sectional survey. PLOS Digit Health. 2022;1(10): e0000114.
Download references
Acknowledgements
We appreciate all the authors of included studies.
Author information
Hamidreza Amiri, Samira Peiravi and Seyedeh sara rezazadeh shojaee contributed equally to this work.
Authors and Affiliations
Student Research Committee, Arak University of Medical Sciences, Arak, Iran
Hamidreza Amiri
Department of Emergency Medicine, Faculty of Medicine, Mashhad University of Medical Sciences, Mashhad, Iran
Samira Peiravi
Department of Nursing, Faculty of Nursing and Midwifery, Mashhad Medical Sciences, Islamic Azad University, Mashhad, Iran
Seyedeh sara rezazadeh shojaee
Student Research Committee, School of Nursing and Midwifery, Shahid Sadoughi University of Medical Sciences, Yazd, Iran
Motahareh Rouhparvarzamin
Student Research Committee, Faculty of Nursing and Midwifery, Mashhad University of Medical Sciences, Mashhad, Iran
Mohammad Naser Nateghi
Students Research Committee, School of Medicine, Isfahan University of Medical Sciences, Isfahan, Iran
Mohammad Hossein Etemadi
Medical Informatics Research Center, Institute for Futures Studies in Health, Kerman University of Medical Sciences, Kerman, Iran
Mahdie ShojaeiBaghini
Dentistry Student, Dental Branch, Islamic Azad University, Tehran, Iran
Farhan Musaie
Master of Health Science, Faculty of Health Sciences, Universiti Kebangsaan Malaysia (UKM), Kuala Lumpur, Malaysia
Mohammad Hossein Anvari
Student Research Committee, School of Medicine, Shahid Beheshti University of Medical Sciences, SBUMS, Arabi Ave, Daneshjoo Blvd, Velenjak, Tehran, 19839-63113, Iran
Mahsa Asadi Anar
You can also search for this author in PubMed Google Scholar
Contributions
Study design and conception: M.AA; Search & study selection: H.A, S.P; Data extraction: SS.RS, M.P; Quality assessment: MN.N, MH.E; Statistical analysis and interpretation: M.S, F.M; Drafting the manuscript: H.A, S.P, SS.RS, M.P, MN.N, MH.E, M.S, F.M, MH.A; Critical revision: M.AA. All authors were approved the submitted version.
Corresponding author
Correspondence to Mahsa Asadi Anar .
Ethics declarations
Ethics approval and consent to participate.
Not applicable.
Consent for publication
Competing interests.
The authors declare no competing interests.
Additional information
Publisher’s note.
Springer Nature remains neutral with regard to jurisdictional claims in published maps and institutional affiliations.
Supplementary Information
Supplementary materials 1., rights and permissions.
Open Access This article is licensed under a Creative Commons Attribution 4.0 International License, which permits use, sharing, adaptation, distribution and reproduction in any medium or format, as long as you give appropriate credit to the original author(s) and the source, provide a link to the Creative Commons licence, and indicate if changes were made. The images or other third party material in this article are included in the article's Creative Commons licence, unless indicated otherwise in a credit line to the material. If material is not included in the article's Creative Commons licence and your intended use is not permitted by statutory regulation or exceeds the permitted use, you will need to obtain permission directly from the copyright holder. To view a copy of this licence, visit http://creativecommons.org/licenses/by/4.0/ . The Creative Commons Public Domain Dedication waiver ( http://creativecommons.org/publicdomain/zero/1.0/ ) applies to the data made available in this article, unless otherwise stated in a credit line to the data.
Reprints and permissions
About this article
Cite this article.
Amiri, H., Peiravi, S., rezazadeh shojaee, S. et al. Medical, dental, and nursing students’ attitudes and knowledge towards artificial intelligence: a systematic review and meta-analysis. BMC Med Educ 24 , 412 (2024). https://doi.org/10.1186/s12909-024-05406-1
Download citation
Received : 13 February 2024
Accepted : 09 April 2024
Published : 15 April 2024
DOI : https://doi.org/10.1186/s12909-024-05406-1
Share this article
Anyone you share the following link with will be able to read this content:
Sorry, a shareable link is not currently available for this article.
Provided by the Springer Nature SharedIt content-sharing initiative
- Medical students
- Dental students
- Nursing students
- Meta-analysis
- Systematic review
BMC Medical Education
ISSN: 1472-6920
- Submission enquiries: [email protected]
- General enquiries: [email protected]
Embargoed Country
Due to U.S. export compliance requirements, Spunk has blocked your access to Splunk web properties. If you believe that the action was made in error, please send an email to [email protected] with your name, complete address, your physical location at the time of seeking access, email, and phone number. Splunk will research the issue and respond.
Numbers, Facts and Trends Shaping Your World
Read our research on:
Full Topic List
Regions & Countries
- Publications
- Our Methods
- Short Reads
- Tools & Resources
Read Our Research On:
Changing Partisan Coalitions in a Politically Divided Nation
2. partisanship by race, ethnicity and education, table of contents.
- What this report tells us – and what it doesn’t
- Partisans and partisan leaners in the U.S. electorate
- Party identification and ideology
- Education and partisanship
- Education, race and partisanship
- Partisanship by race and gender
- Partisanship across educational and gender groups by race and ethnicity
- Gender and partisanship
- Parents are more Republican than voters without children
- Partisanship among men and women within age groups
- Race, age and partisanship
- The partisanship of generational cohorts
- Religion, race and ethnicity, and partisanship
- Party identification among atheists, agnostics and ‘nothing in particular’
- Partisanship and religious service attendance
- Partisanship by income groups
- The relationship between income and partisanship differs by education
- Union members remain more Democratic than Republican
- Homeowners are more Republican than renters
- Partisanship of military veterans
- Demographic differences in partisanship by community type
- Race and ethnicity
- Age and the U.S. electorate
- Education by race and ethnicity
- Religious affiliation
- Ideological composition of voters
- Acknowledgments
- Overview of survey methodologies
- The 2023 American Trends Panel profile survey methodology
- Measuring party identification across survey modes
- Adjusting telephone survey trends
- Appendix B: Religious category definitions
- Appendix C: Age cohort definitions
As has long been the case, White voters are much more likely than those in other racial and ethnic groups to associate with the Republican Party. Hispanic and Asian voters tilt more Democratic. Black voters remain overwhelmingly Democratic.
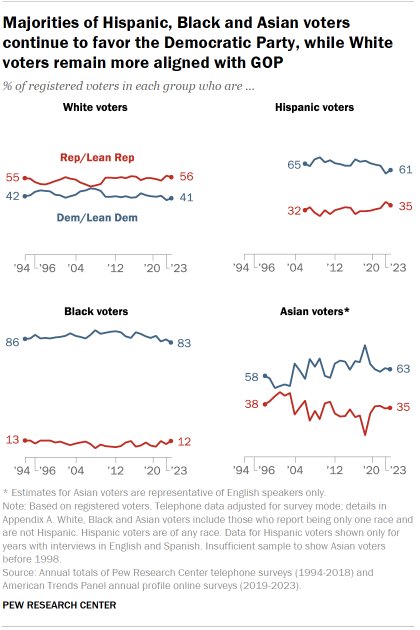
However, there have been some shifts toward the GOP in most groups in recent years.
The Republican Party now holds a 15 percentage point advantage among White voters: 56% of non-Hispanic White voters identify with or lean toward the Republican Party, while 41% align with the Democratic Party.
- This double-digit lead for the GOP among White voters has held for more than a decade. The last time White voters were about equally split between the two parties was in 2008.
About six-in-ten Hispanic voters (61%) are Democrats or lean to the Democratic Party, while 35% are Republicans or Republican leaners.
- The Democratic Party’s edge among Hispanic voters over the last two years is somewhat narrower than it was in years prior.
Black voters continue to overwhelmingly associate with the Democratic Party, although the extent of the Democratic advantage among this group has fallen off over the last few years.
- Currently, 83% of Black voters are Democrats or lean Democratic, while 12% align with the GOP.
- As recently as 2020, the share associating with the Democratic Party was 5 percentage points higher. That somewhat larger edge in party affiliation had been in place for much of the last two decades.
About six-in-ten Asian voters (63%) align with the Democratic Party, while 36% are oriented toward the GOP.
- The balance of partisan association among Asian voters has changed little over the last few years.
The relationship between education and partisanship has shifted significantly since the early years of the 21st century.
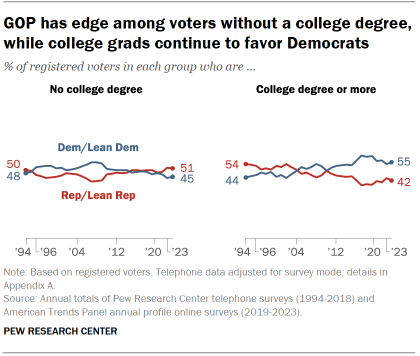
- The Republican Party now holds a 6 percentage point advantage over the Democratic Party (51% to 45%) among voters who do not have a bachelor’s degree. Voters who do not have a four-year degree make up a 60% majority of all registered voters.
- By comparison, the Democratic Party has a 13-point advantage (55% vs. 42%) among those with a bachelor’s degree or more formal education.
This pattern is relatively recent. In fact, until about two decades ago the Republican Party fared better among college graduates and worse among those without a college degree.
In the last years of George W. Bush’s presidency and the first year of Barack Obama’s, Democrats had a double-digit advantage in affiliation over Republicans among voters without a college degree. For example, in 2007, 56% of voters without a degree were Democrats or leaned Democratic, while 42% were Republicans or GOP leaners. This group was narrowly divided between the two parties for most of the next 15 years, but in the last few years it has tilted more Republican.
College graduates moved in the opposite direction, becoming more Democratic over this same period.
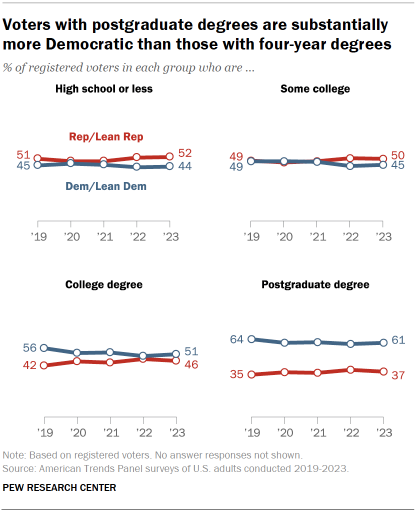
- Since 2017, the gap in partisanship between college graduates and those without a degree has been wider than at any previous point in Pew Research Center surveys dating back to the 1990s.
Voters with postgraduate degrees are even more Democratic than those with bachelor’s degrees. About six-in-ten registered voters who have a postgraduate degree (61%) identify with or lean toward the Democratic Party, while 37% associate with the Republican Party. Voters with a bachelor’s degree but no graduate degree are more closely divided: 51% Democratic, 46% Republican.
Voters with a high school degree or less education and those who have attended some college but do not have a bachelor’s degree both tilt Republican by similar margins.
White voters are far more polarized along educational lines than are Hispanic and Black voters.
White voters by education
By nearly two-to-one (63% vs. 33%), White voters without a bachelor’s degree associate with the Republican Party.
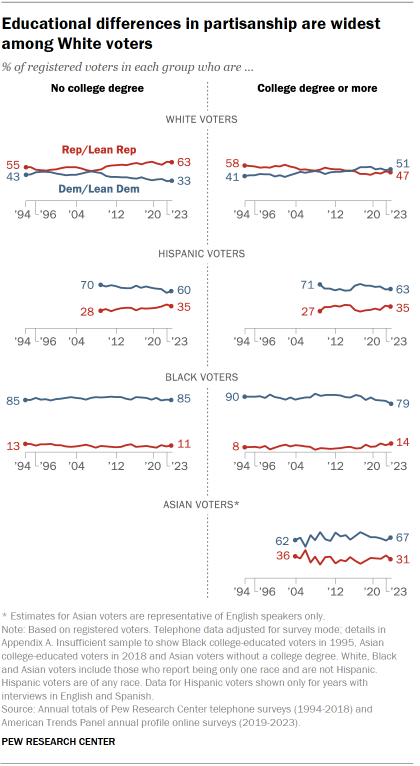
The GOP’s advantage among this group has remained relatively steady over the last several years but reflects a major shift since 2009. This group is now substantially more Republican-oriented than at any prior point in the last three decades.
Today, White voters with a bachelor’s degree are closely divided between associating with the Democratic Party (51%) and the Republican Party (47%). Prior to 2005, this group had a clear Republican orientation.
Hispanic voters by education
In contrast, there are no meaningful differences in the partisan leanings of Hispanic voters with and without bachelor’s degrees. Democrats hold a clear advantage in affiliation among both groups of Hispanic voters, although the share of Hispanics (both those with and without bachelor’s degrees) who align with the Democratic Party has edged lower in recent years.
Black voters by education
Black voters both with (79%) and without college degrees (85%) remain overwhelmingly Democratic in their partisan affinity.
Black college graduates are somewhat less closely aligned with the Democratic Party now than they were for most of the prior three decades (for most of this period, 85% or more of Black college graduate voters affiliated with the Democratic Party).
Asian voters by education
Two-thirds of Asian voters with a college degree align with the Democratic Party; 31% associate with the Republican Party. The partisan balance among Asian voters with a college degree has remained largely the same over our last two decades of surveys. (Asian American voters without a college degree are a smaller group, and sample sizes do not allow for reporting trends among this group.)
Visit the chapter on partisanship by gender, sexual orientation and marital and parental status for discussion of overall trends among men and women.
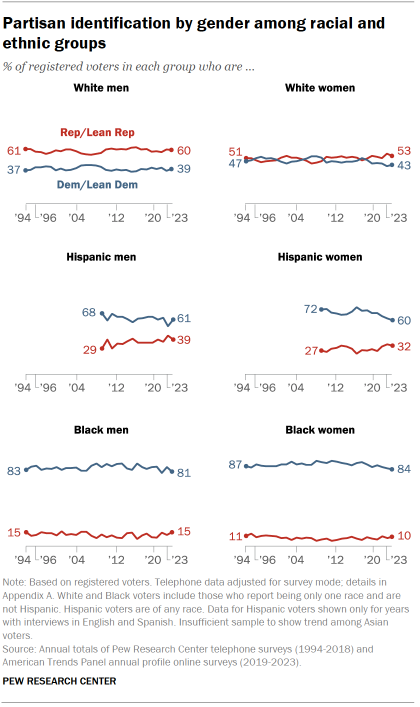
Six-in-ten White men who are registered voters identify as Republicans or lean Republican, as do 53% of White women voters.
The balance of partisanship among White women voters has tilted toward the GOP in recent years, but it was more divided in 2017 and 2018.
Among Hispanic voters, about six-in-ten men (61%) and women (60%) associate with the Democrats. Hispanic women voters have become somewhat less Democratic in recent years (down from 74% in 2016).
About eight-in-ten Black voters – both women (84%) and men (81%) – are Democrats or Democratic leaners.
About six-in-ten men (61%) and women (64%) among Asian voters identify as Democrats or lean toward the Democratic Party. (There is insufficient sample to show longer-term trends among Asian voters by gender.)
Among White voters, there are wide differences in partisanship by gender, by educational attainment – and by the combination of these.
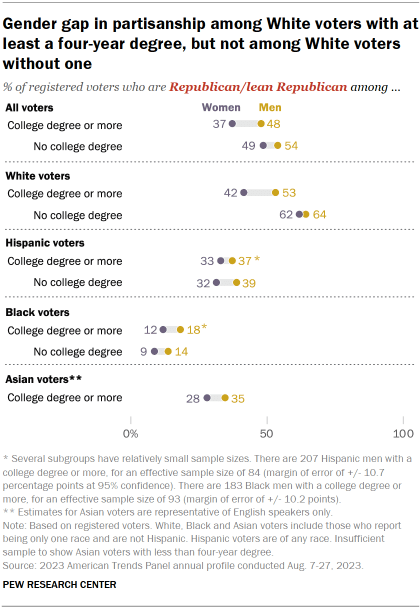
- Among White voters without a college degree, 64% of men and 62% of women say they identify as or lean toward the Republican Party (about a third of each associate with the Democrats).
- White men with a college degree also tilt Republican among voters, though to a lesser extent (53% are Republicans or lean Republican, 45% are Democrats or lean Democratic).
- In contrast, White women with a college degree are more Democratic than Republican by 15 percentage points (42% Republican or Republican leaning, 57% Democrat or lean Democrat).
Among Black and Hispanic voters, there are only modest differences in partisanship across the combination of gender and education. In both groups, there are no significant differences between men with and without college degrees, or between their women counterparts. (Because Asian American voters without a college degree are a small group, sample sizes do not allow comparing college and non-college Asian men and women.)
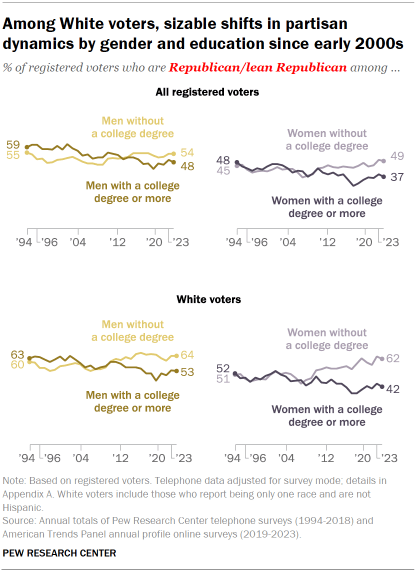
This dynamic has changed over time, as college-educated White men and women have grown more Democratic and those with less formal education have grown more Republican.
As recently as 15 years ago, there were sizable gender gaps in partisanship among both college and non-college White voters. In both cases, men were substantially more likely than women to associate with the Republican Party.
But, at that time, there was not a substantial difference in the partisanship of college and non-college White voters – for either women or men.
Today, there is no gender gap in partisanship among non-college White voters, while there is a gender gap among college graduate White voters. The difference in partisanship between White women voters who have a college degree and those who do not, in particular, is quite large.
By contrast, there is little variation in the partisanship of Black and Hispanic voters by these characteristics, and the relationship has varied less over time.
Sign up for The Briefing
Weekly updates on the world of news & information
- Education & Politics
- Election 2024
- Gender & Politics
- Political Parties
- Race, Ethnicity & Politics
- Religion & Politics
- Rural, Urban and Suburban Communities
- Voter Demographics
What’s It Like To Be a Teacher in America Today?
Republican gains in 2022 midterms driven mostly by turnout advantage, more americans disapprove than approve of colleges considering race, ethnicity in admissions decisions, partisan divides over k-12 education in 8 charts, school district mission statements highlight a partisan divide over diversity, equity and inclusion in k-12 education, most popular, report materials.
- Party Identification Detailed Tables, 1994-2023
1615 L St. NW, Suite 800 Washington, DC 20036 USA (+1) 202-419-4300 | Main (+1) 202-857-8562 | Fax (+1) 202-419-4372 | Media Inquiries
Research Topics
- Age & Generations
- Coronavirus (COVID-19)
- Economy & Work
- Family & Relationships
- Gender & LGBTQ
- Immigration & Migration
- International Affairs
- Internet & Technology
- Methodological Research
- News Habits & Media
- Non-U.S. Governments
- Other Topics
- Politics & Policy
- Race & Ethnicity
- Email Newsletters
ABOUT PEW RESEARCH CENTER Pew Research Center is a nonpartisan fact tank that informs the public about the issues, attitudes and trends shaping the world. It conducts public opinion polling, demographic research, media content analysis and other empirical social science research. Pew Research Center does not take policy positions. It is a subsidiary of The Pew Charitable Trusts .
Copyright 2024 Pew Research Center
Terms & Conditions
Privacy Policy
Cookie Settings
Reprints, Permissions & Use Policy
- Skip to main content
- Skip to FDA Search
- Skip to in this section menu
- Skip to footer links

The .gov means it’s official. Federal government websites often end in .gov or .mil. Before sharing sensitive information, make sure you're on a federal government site.
The site is secure. The https:// ensures that you are connecting to the official website and that any information you provide is encrypted and transmitted securely.
U.S. Food and Drug Administration
- Search
- Menu
- Science & Research
- About Science & Research at FDA
- The FDA Science Forum
Real-World Data Analysis of Adverse Events Attributable to Large Joint Arthroplasty Implants
2023 FDA Science Forum
Background:
With various adverse events reportedly associated with metal implants, their clinical manifestations and biological underpinnings remain unclear. We employed a comprehensive analysis using real-world data (RWD) from electronic health records (EHR) to explore arthroplasty implant-related adverse outcomes with respect to device/patient characteristics.
This research aims to: 1) outline the scope, frequency, and underlying nature of clinically relevant adverse outcomes potentially attributable to arthroplasty implants; 2) explore pre-implantation risk factors and post-implantation complications likely associated with arthroplasty implant reactivity; and 3) develop device-oriented RWD analysis/visualization algorithms.
This research focused on large joint arthroplasty,utilized an EHR dataset of ~27,000 patients who had an arthroplasty encounter (2016 - 2019) that was collated by Loopback Analytics LLC for FDA. Cohorts with hip, knee, or shoulder arthroplasty were established using standardized ICD-10 codes. Comorbidity analysis with respect to the implantation time was performed in subjects with Revision and known arthroplasty-related Adverse Outcomes (AO+Rev) versus those without these outcomes (Control). Inter-cohort differences were assessed using chi-square test with odds ratios, relative risk ratios, and multivariate regression. Time-to-event analysis using Kaplan-Meier approach, log-rank test, and Cox proportional hazards regression were applied to evaluate the inter-cohort differences in pre-selected conditions representing potential implant-related immune/inflammatory responses. LASSO regression modelling was conducted as an unsupervised assessment of diagnoses that may predict AO+Rev. The co-occurrence and correlations between diagnoses pairs were assessed and visualized by network analysis; comorbidity score was introduced to quantify the correlations pertaining to diagnoses that may represent arthroplasty implant reactivity. Hierarchical clustering and correlation heatmaps were applied to visualize the intergroup differences in AO+Rev vs. Control comorbidity patterns and relationships between diagnoses of interest.
Compared to Controls, the AO+Rev cohort showed distinct likelihoods of different diagnoses that potentially represent arthroplasty-related underlying patient conditions (pre-implantation) or underrecognized complications (post-implantation), including some allergic and immune/inflammatory conditions. Different RWD analysis/visualization approaches with the respective results will be illustrated.
Conclusion:
The developed RWD algorithm can be applied for providing insights into the risk factors and complications pertaining to various arthroplasty implants, thereby optimizing leading to a more predictive evaluation of implant safety in the real-world setting.
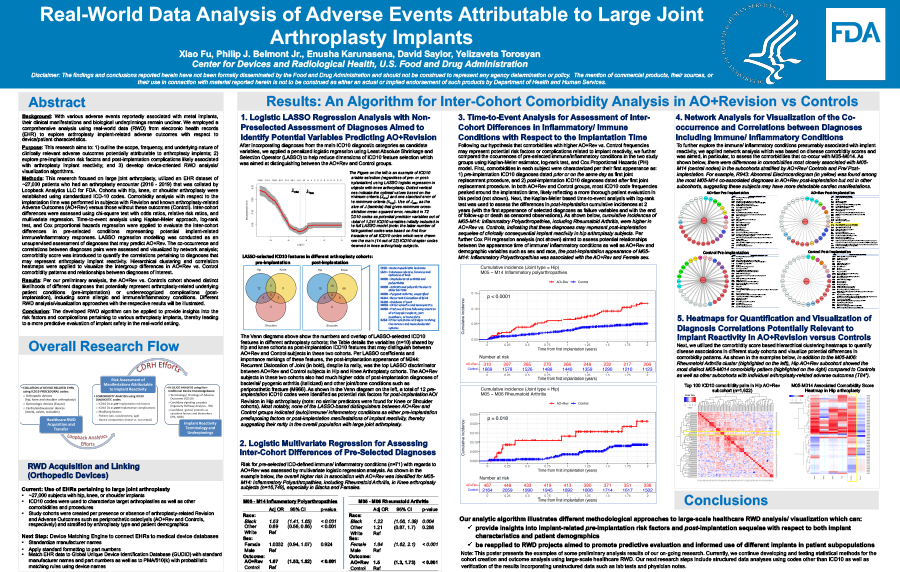
Download the Poster (PDF; 2.93 MB)

IMAGES
VIDEO
COMMENTS
What implications did this rise of data science as a transdisciplinary methodological toolkit have for the field of education?One means of illustrating the salience of data science in education research is to study its emergence in the Education Resources Information Center's (ERIC) publication corpus. 1 In the corpus, the growth of data science in education can be identified by the adoption ...
Peel, Karen L. (2020) "A Beginner's Guide to Applied Educational Research using Thematic Analysis," Practical Assessment, Research, and Evaluation: Vol. 25, Article 2. This Article is brought to you for free and open access by ScholarWorks@UMass Amherst. It has been accepted for inclusion in Practical Assessment, Research, and Evaluation by an ...
Whether the goal is to identify and describe trends and variation in populations, create new measures of key phenomena, or describe samples in studies aimed at identifying causal effects, description plays a critical role in the scientific process in general and education research in particular. Descriptive analysis identifies patterns in data ...
Data collection methods in educational research are used to gather information that is then analyzed and interpreted. As such, data collection is a very important step in conducting research and can influence results significantly. Once the research question and sources of data are identified, appropriate methods of data collection are determined.
Thematic analysis (TA) is a commonly used qualitative data analysis approach in psychology (Braun & Clarke, 2006), health care (Braun & Clarke, 2014), sport and exercise (Braun et al., 2017), and many other fields (Boyatzis, 1998).However, a lack of description about the process and details of analysis often leads the TA report readers to wonder how exactly qualitative information is ...
Dirk's research focuses on the intersection of cognitive psychology, educational technology, data analytics, and organisational learning. His research outcomes include numerous co-authored books, book series, book chapters, journal articles, and international conference papers, as well as successful grant funding in Australia, Germany, and USA.
In this full review paper, the recent emerging trends in Educational Data Science have been reviewed and explored to address the recent topics and contributions in the era of Smart Education. This includes a set of rigorously reviewed world-class manuscripts addressing and detailing state-of-the-art, frameworks and techniques research projects in the area of Data Science applied to Education ...
As a consequence, the general and systematic use of advanced methodologies for data analysis is certainly the 'new frontier' for educational research today. This has been a constant guideline while preparing this issue, and the main criterion for selecting contributing papers. A number of papers applying quantitative methodologies have been ...
Teaching Analytics (TA) is a new theoretical approach, which combines teaching expertise, visual analytics and design-based research to support teacher's diagnostic pedagogical ability to use data and evidence to improve the quality of teaching. TA is now gaining prominence because it offers enormous opportunities to the teachers. It also identifies optimal ways in which teaching performance ...
Both communities share a common interest in data-intensive approaches to educational research, ... • Educational Data Science ... solving educational problems (Romero & Ventura, 2017). Data science is a concept to unify statistics, data analysis, machine learning and their related methods. This survey is an updated and improved version of the ...
1. Describe the application of the following statistical areas to educational interventions: study designs, variable types, exploratory data analysis, confirmatory (inferential) data analysis, and basic interpretation of results. 2. Use a four-step approach to choosing a statistical test for educational cases. Go to:
In addition, the analysis found that the most significant country in the area of education big data and learning analytics research was the United States, with the highest number of publications.
The data generated in educational contexts is often large, complex, and heterogeneous, making it difficult to understand—even with advanced data analysis capabilities. For instance, students enrolled in Massive Online Open Courses (MOOCs) generate large, heterogeneous datasets comprising clickstream data, contributions to discussion forums ...
Data analysis is an important step in the research process as it influences the quality and standard of reporting research findings. Based on a review of the content of 255 doctorate theses, the use of computer applications for data analysis in educational research was assessed.
Discourse or narrative analysis; Grouded theory; Phenomenological analysis or interpretative phenomenological analysis (IPA) Further reading and resources. As a starting point for most of these, we would recommend the relevant chapter from Part 5 of Cohen, Manion and Morrison (2018), Research Methods in Education. You may also find the ...
The education-research community is in the middle of two advances that are changing the way we can analyze and understand educational processes. First, more than ever in the past, we have access to broad, rich, educationally-relevant text data from sources such as online discussion forums, transcribed
1.2 Why a book on data analysis in R for educational research. There are at least three reasons why data analysis in educational research is hard: Educational researchers have unique methods: an emphasis on multi-level models, networks, and measurement are just some examples. Educational researchers face unique challenges: coming from myriad ...
Investigation of Higher Education Data Needs After a decade of supporting data and analysis in PK-12 education, the leadership of the Strategic ... The Harvard Center for Education Policy Research launched SDP in 2008 to meet a need for analytical capacity in state and local K-12 agencies. Reform-minded school superintendents
By developing fast and efficient algorithms and data-driven models for real-time data processing, machine learning can produce accurate results and analysis [7]. In the education sector, the data ...
The National Center for Analysis of Longitudinal Data in Education Research (CALDER) is a joint project of AIR and scholars at Duke University, Northwestern University, Stanford University, the University of Missouri, the University of Texas at Dallas, and the University of Washington.. The Center's research scientists are leaders in the field of economics and other social sciences as they ...
Aim/Purpose: This study aimed to evaluate the extant research on data science education (DSE) to identify the existing gaps, opportunities, and challenges, and make recommendations for current and future DSE. Background: There has been an increase in the number of data science programs especially because of the increased appreciation of data as a multidisciplinary strategic resource.
Background Nowadays, Artificial intelligence (AI) is one of the most popular topics that can be integrated into healthcare activities. Currently, AI is used in specialized fields such as radiology, pathology, and ophthalmology. Despite the advantages of AI, the fear of human labor being replaced by this technology makes some students reluctant to choose specific fields. This meta-analysis aims ...
research on education big data and learning analytics. Figure 5 is a map that visualizes the most significant countries in this regard in terms of TP, according to the Scopus database. Table 3 ...
July is both Disability Pride Month and the anniversary of the Americans with Disabilities Act. To mark these occasions, Pew Research Center used federal education data from the National Center for Education Statistics to learn more about students who receive special education services in U.S. public schools.. In this analysis, students with disabilities include those ages 3 to 21 who are ...
For many researchers unfamiliar with qualitative research, determining how to conduct qualitative analyses is often quite challenging. Part of this challenge is due to the seemingly limitless approaches that a qualitative researcher might leverage, as well as simply learning to think like a qualitative researcher when analyzing data. From framework analysis (Ritchie & Spencer, 1994) to content ...
Data analysis is crucial in today's businesses and organizations. With the increasing amount of data being created at 328.77 million terabytes of data per day, and them being readily available to most businesses, having efficient tools that can help analyze and interpret this data effectively is essential.. In this article, we will discuss the top 9 best data analysis tools currently used in ...
For this analysis, we used annual totals of data from Pew Research Center telephone surveys (1994-2018) and online surveys (2019-2023) among registered voters. All telephone survey data was adjusted to account for differences in how people respond to surveys on the telephone compared with online surveys (refer to Appendix A for details).
About Pew Research Center Pew Research Center is a nonpartisan fact tank that informs the public about the issues, attitudes and trends shaping the world. It conducts public opinion polling, demographic research, media content analysis and other empirical social science research. Pew Research Center does not take policy positions.
The research reported here was commissioned by the U.S. Air Force (USAF) and conducted within the Resource Management Program of RAND Project AIR FORCE. This report is part of the RAND research report series. RAND reports present research findings and objective analysis that address the challenges facing the public and private sectors.
This research focused on large joint arthroplasty,utilized an EHR dataset of ~27,000 patients who had an arthroplasty encounter (2016 - 2019) that was collated by Loopback Analytics LLC for FDA.