Thank you for visiting nature.com. You are using a browser version with limited support for CSS. To obtain the best experience, we recommend you use a more up to date browser (or turn off compatibility mode in Internet Explorer). In the meantime, to ensure continued support, we are displaying the site without styles and JavaScript.
- View all journals
- My Account Login
- Explore content
- About the journal
- Publish with us
- Sign up for alerts
- Review Article
- Open access
- Published: 11 January 2023

The effectiveness of collaborative problem solving in promoting students’ critical thinking: A meta-analysis based on empirical literature
- Enwei Xu ORCID: orcid.org/0000-0001-6424-8169 1 ,
- Wei Wang 1 &
- Qingxia Wang 1
Humanities and Social Sciences Communications volume 10 , Article number: 16 ( 2023 ) Cite this article
15k Accesses
15 Citations
3 Altmetric
Metrics details
- Science, technology and society
Collaborative problem-solving has been widely embraced in the classroom instruction of critical thinking, which is regarded as the core of curriculum reform based on key competencies in the field of education as well as a key competence for learners in the 21st century. However, the effectiveness of collaborative problem-solving in promoting students’ critical thinking remains uncertain. This current research presents the major findings of a meta-analysis of 36 pieces of the literature revealed in worldwide educational periodicals during the 21st century to identify the effectiveness of collaborative problem-solving in promoting students’ critical thinking and to determine, based on evidence, whether and to what extent collaborative problem solving can result in a rise or decrease in critical thinking. The findings show that (1) collaborative problem solving is an effective teaching approach to foster students’ critical thinking, with a significant overall effect size (ES = 0.82, z = 12.78, P < 0.01, 95% CI [0.69, 0.95]); (2) in respect to the dimensions of critical thinking, collaborative problem solving can significantly and successfully enhance students’ attitudinal tendencies (ES = 1.17, z = 7.62, P < 0.01, 95% CI[0.87, 1.47]); nevertheless, it falls short in terms of improving students’ cognitive skills, having only an upper-middle impact (ES = 0.70, z = 11.55, P < 0.01, 95% CI[0.58, 0.82]); and (3) the teaching type (chi 2 = 7.20, P < 0.05), intervention duration (chi 2 = 12.18, P < 0.01), subject area (chi 2 = 13.36, P < 0.05), group size (chi 2 = 8.77, P < 0.05), and learning scaffold (chi 2 = 9.03, P < 0.01) all have an impact on critical thinking, and they can be viewed as important moderating factors that affect how critical thinking develops. On the basis of these results, recommendations are made for further study and instruction to better support students’ critical thinking in the context of collaborative problem-solving.
Similar content being viewed by others
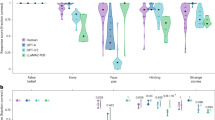
Testing theory of mind in large language models and humans
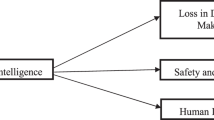
Impact of artificial intelligence on human loss in decision making, laziness and safety in education
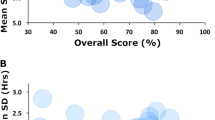
Sleep quality, duration, and consistency are associated with better academic performance in college students
Introduction.
Although critical thinking has a long history in research, the concept of critical thinking, which is regarded as an essential competence for learners in the 21st century, has recently attracted more attention from researchers and teaching practitioners (National Research Council, 2012 ). Critical thinking should be the core of curriculum reform based on key competencies in the field of education (Peng and Deng, 2017 ) because students with critical thinking can not only understand the meaning of knowledge but also effectively solve practical problems in real life even after knowledge is forgotten (Kek and Huijser, 2011 ). The definition of critical thinking is not universal (Ennis, 1989 ; Castle, 2009 ; Niu et al., 2013 ). In general, the definition of critical thinking is a self-aware and self-regulated thought process (Facione, 1990 ; Niu et al., 2013 ). It refers to the cognitive skills needed to interpret, analyze, synthesize, reason, and evaluate information as well as the attitudinal tendency to apply these abilities (Halpern, 2001 ). The view that critical thinking can be taught and learned through curriculum teaching has been widely supported by many researchers (e.g., Kuncel, 2011 ; Leng and Lu, 2020 ), leading to educators’ efforts to foster it among students. In the field of teaching practice, there are three types of courses for teaching critical thinking (Ennis, 1989 ). The first is an independent curriculum in which critical thinking is taught and cultivated without involving the knowledge of specific disciplines; the second is an integrated curriculum in which critical thinking is integrated into the teaching of other disciplines as a clear teaching goal; and the third is a mixed curriculum in which critical thinking is taught in parallel to the teaching of other disciplines for mixed teaching training. Furthermore, numerous measuring tools have been developed by researchers and educators to measure critical thinking in the context of teaching practice. These include standardized measurement tools, such as WGCTA, CCTST, CCTT, and CCTDI, which have been verified by repeated experiments and are considered effective and reliable by international scholars (Facione and Facione, 1992 ). In short, descriptions of critical thinking, including its two dimensions of attitudinal tendency and cognitive skills, different types of teaching courses, and standardized measurement tools provide a complex normative framework for understanding, teaching, and evaluating critical thinking.
Cultivating critical thinking in curriculum teaching can start with a problem, and one of the most popular critical thinking instructional approaches is problem-based learning (Liu et al., 2020 ). Duch et al. ( 2001 ) noted that problem-based learning in group collaboration is progressive active learning, which can improve students’ critical thinking and problem-solving skills. Collaborative problem-solving is the organic integration of collaborative learning and problem-based learning, which takes learners as the center of the learning process and uses problems with poor structure in real-world situations as the starting point for the learning process (Liang et al., 2017 ). Students learn the knowledge needed to solve problems in a collaborative group, reach a consensus on problems in the field, and form solutions through social cooperation methods, such as dialogue, interpretation, questioning, debate, negotiation, and reflection, thus promoting the development of learners’ domain knowledge and critical thinking (Cindy, 2004 ; Liang et al., 2017 ).
Collaborative problem-solving has been widely used in the teaching practice of critical thinking, and several studies have attempted to conduct a systematic review and meta-analysis of the empirical literature on critical thinking from various perspectives. However, little attention has been paid to the impact of collaborative problem-solving on critical thinking. Therefore, the best approach for developing and enhancing critical thinking throughout collaborative problem-solving is to examine how to implement critical thinking instruction; however, this issue is still unexplored, which means that many teachers are incapable of better instructing critical thinking (Leng and Lu, 2020 ; Niu et al., 2013 ). For example, Huber ( 2016 ) provided the meta-analysis findings of 71 publications on gaining critical thinking over various time frames in college with the aim of determining whether critical thinking was truly teachable. These authors found that learners significantly improve their critical thinking while in college and that critical thinking differs with factors such as teaching strategies, intervention duration, subject area, and teaching type. The usefulness of collaborative problem-solving in fostering students’ critical thinking, however, was not determined by this study, nor did it reveal whether there existed significant variations among the different elements. A meta-analysis of 31 pieces of educational literature was conducted by Liu et al. ( 2020 ) to assess the impact of problem-solving on college students’ critical thinking. These authors found that problem-solving could promote the development of critical thinking among college students and proposed establishing a reasonable group structure for problem-solving in a follow-up study to improve students’ critical thinking. Additionally, previous empirical studies have reached inconclusive and even contradictory conclusions about whether and to what extent collaborative problem-solving increases or decreases critical thinking levels. As an illustration, Yang et al. ( 2008 ) carried out an experiment on the integrated curriculum teaching of college students based on a web bulletin board with the goal of fostering participants’ critical thinking in the context of collaborative problem-solving. These authors’ research revealed that through sharing, debating, examining, and reflecting on various experiences and ideas, collaborative problem-solving can considerably enhance students’ critical thinking in real-life problem situations. In contrast, collaborative problem-solving had a positive impact on learners’ interaction and could improve learning interest and motivation but could not significantly improve students’ critical thinking when compared to traditional classroom teaching, according to research by Naber and Wyatt ( 2014 ) and Sendag and Odabasi ( 2009 ) on undergraduate and high school students, respectively.
The above studies show that there is inconsistency regarding the effectiveness of collaborative problem-solving in promoting students’ critical thinking. Therefore, it is essential to conduct a thorough and trustworthy review to detect and decide whether and to what degree collaborative problem-solving can result in a rise or decrease in critical thinking. Meta-analysis is a quantitative analysis approach that is utilized to examine quantitative data from various separate studies that are all focused on the same research topic. This approach characterizes the effectiveness of its impact by averaging the effect sizes of numerous qualitative studies in an effort to reduce the uncertainty brought on by independent research and produce more conclusive findings (Lipsey and Wilson, 2001 ).
This paper used a meta-analytic approach and carried out a meta-analysis to examine the effectiveness of collaborative problem-solving in promoting students’ critical thinking in order to make a contribution to both research and practice. The following research questions were addressed by this meta-analysis:
What is the overall effect size of collaborative problem-solving in promoting students’ critical thinking and its impact on the two dimensions of critical thinking (i.e., attitudinal tendency and cognitive skills)?
How are the disparities between the study conclusions impacted by various moderating variables if the impacts of various experimental designs in the included studies are heterogeneous?
This research followed the strict procedures (e.g., database searching, identification, screening, eligibility, merging, duplicate removal, and analysis of included studies) of Cooper’s ( 2010 ) proposed meta-analysis approach for examining quantitative data from various separate studies that are all focused on the same research topic. The relevant empirical research that appeared in worldwide educational periodicals within the 21st century was subjected to this meta-analysis using Rev-Man 5.4. The consistency of the data extracted separately by two researchers was tested using Cohen’s kappa coefficient, and a publication bias test and a heterogeneity test were run on the sample data to ascertain the quality of this meta-analysis.
Data sources and search strategies
There were three stages to the data collection process for this meta-analysis, as shown in Fig. 1 , which shows the number of articles included and eliminated during the selection process based on the statement and study eligibility criteria.
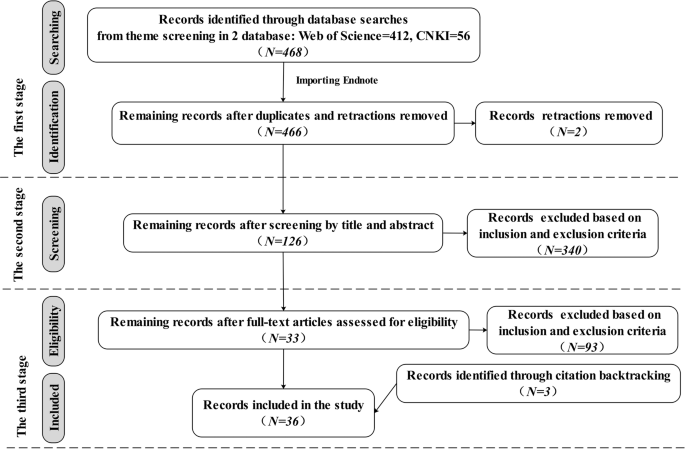
This flowchart shows the number of records identified, included and excluded in the article.
First, the databases used to systematically search for relevant articles were the journal papers of the Web of Science Core Collection and the Chinese Core source journal, as well as the Chinese Social Science Citation Index (CSSCI) source journal papers included in CNKI. These databases were selected because they are credible platforms that are sources of scholarly and peer-reviewed information with advanced search tools and contain literature relevant to the subject of our topic from reliable researchers and experts. The search string with the Boolean operator used in the Web of Science was “TS = (((“critical thinking” or “ct” and “pretest” or “posttest”) or (“critical thinking” or “ct” and “control group” or “quasi experiment” or “experiment”)) and (“collaboration” or “collaborative learning” or “CSCL”) and (“problem solving” or “problem-based learning” or “PBL”))”. The research area was “Education Educational Research”, and the search period was “January 1, 2000, to December 30, 2021”. A total of 412 papers were obtained. The search string with the Boolean operator used in the CNKI was “SU = (‘critical thinking’*‘collaboration’ + ‘critical thinking’*‘collaborative learning’ + ‘critical thinking’*‘CSCL’ + ‘critical thinking’*‘problem solving’ + ‘critical thinking’*‘problem-based learning’ + ‘critical thinking’*‘PBL’ + ‘critical thinking’*‘problem oriented’) AND FT = (‘experiment’ + ‘quasi experiment’ + ‘pretest’ + ‘posttest’ + ‘empirical study’)” (translated into Chinese when searching). A total of 56 studies were found throughout the search period of “January 2000 to December 2021”. From the databases, all duplicates and retractions were eliminated before exporting the references into Endnote, a program for managing bibliographic references. In all, 466 studies were found.
Second, the studies that matched the inclusion and exclusion criteria for the meta-analysis were chosen by two researchers after they had reviewed the abstracts and titles of the gathered articles, yielding a total of 126 studies.
Third, two researchers thoroughly reviewed each included article’s whole text in accordance with the inclusion and exclusion criteria. Meanwhile, a snowball search was performed using the references and citations of the included articles to ensure complete coverage of the articles. Ultimately, 36 articles were kept.
Two researchers worked together to carry out this entire process, and a consensus rate of almost 94.7% was reached after discussion and negotiation to clarify any emerging differences.
Eligibility criteria
Since not all the retrieved studies matched the criteria for this meta-analysis, eligibility criteria for both inclusion and exclusion were developed as follows:
The publication language of the included studies was limited to English and Chinese, and the full text could be obtained. Articles that did not meet the publication language and articles not published between 2000 and 2021 were excluded.
The research design of the included studies must be empirical and quantitative studies that can assess the effect of collaborative problem-solving on the development of critical thinking. Articles that could not identify the causal mechanisms by which collaborative problem-solving affects critical thinking, such as review articles and theoretical articles, were excluded.
The research method of the included studies must feature a randomized control experiment or a quasi-experiment, or a natural experiment, which have a higher degree of internal validity with strong experimental designs and can all plausibly provide evidence that critical thinking and collaborative problem-solving are causally related. Articles with non-experimental research methods, such as purely correlational or observational studies, were excluded.
The participants of the included studies were only students in school, including K-12 students and college students. Articles in which the participants were non-school students, such as social workers or adult learners, were excluded.
The research results of the included studies must mention definite signs that may be utilized to gauge critical thinking’s impact (e.g., sample size, mean value, or standard deviation). Articles that lacked specific measurement indicators for critical thinking and could not calculate the effect size were excluded.
Data coding design
In order to perform a meta-analysis, it is necessary to collect the most important information from the articles, codify that information’s properties, and convert descriptive data into quantitative data. Therefore, this study designed a data coding template (see Table 1 ). Ultimately, 16 coding fields were retained.
The designed data-coding template consisted of three pieces of information. Basic information about the papers was included in the descriptive information: the publishing year, author, serial number, and title of the paper.
The variable information for the experimental design had three variables: the independent variable (instruction method), the dependent variable (critical thinking), and the moderating variable (learning stage, teaching type, intervention duration, learning scaffold, group size, measuring tool, and subject area). Depending on the topic of this study, the intervention strategy, as the independent variable, was coded into collaborative and non-collaborative problem-solving. The dependent variable, critical thinking, was coded as a cognitive skill and an attitudinal tendency. And seven moderating variables were created by grouping and combining the experimental design variables discovered within the 36 studies (see Table 1 ), where learning stages were encoded as higher education, high school, middle school, and primary school or lower; teaching types were encoded as mixed courses, integrated courses, and independent courses; intervention durations were encoded as 0–1 weeks, 1–4 weeks, 4–12 weeks, and more than 12 weeks; group sizes were encoded as 2–3 persons, 4–6 persons, 7–10 persons, and more than 10 persons; learning scaffolds were encoded as teacher-supported learning scaffold, technique-supported learning scaffold, and resource-supported learning scaffold; measuring tools were encoded as standardized measurement tools (e.g., WGCTA, CCTT, CCTST, and CCTDI) and self-adapting measurement tools (e.g., modified or made by researchers); and subject areas were encoded according to the specific subjects used in the 36 included studies.
The data information contained three metrics for measuring critical thinking: sample size, average value, and standard deviation. It is vital to remember that studies with various experimental designs frequently adopt various formulas to determine the effect size. And this paper used Morris’ proposed standardized mean difference (SMD) calculation formula ( 2008 , p. 369; see Supplementary Table S3 ).
Procedure for extracting and coding data
According to the data coding template (see Table 1 ), the 36 papers’ information was retrieved by two researchers, who then entered them into Excel (see Supplementary Table S1 ). The results of each study were extracted separately in the data extraction procedure if an article contained numerous studies on critical thinking, or if a study assessed different critical thinking dimensions. For instance, Tiwari et al. ( 2010 ) used four time points, which were viewed as numerous different studies, to examine the outcomes of critical thinking, and Chen ( 2013 ) included the two outcome variables of attitudinal tendency and cognitive skills, which were regarded as two studies. After discussion and negotiation during data extraction, the two researchers’ consistency test coefficients were roughly 93.27%. Supplementary Table S2 details the key characteristics of the 36 included articles with 79 effect quantities, including descriptive information (e.g., the publishing year, author, serial number, and title of the paper), variable information (e.g., independent variables, dependent variables, and moderating variables), and data information (e.g., mean values, standard deviations, and sample size). Following that, testing for publication bias and heterogeneity was done on the sample data using the Rev-Man 5.4 software, and then the test results were used to conduct a meta-analysis.
Publication bias test
When the sample of studies included in a meta-analysis does not accurately reflect the general status of research on the relevant subject, publication bias is said to be exhibited in this research. The reliability and accuracy of the meta-analysis may be impacted by publication bias. Due to this, the meta-analysis needs to check the sample data for publication bias (Stewart et al., 2006 ). A popular method to check for publication bias is the funnel plot; and it is unlikely that there will be publishing bias when the data are equally dispersed on either side of the average effect size and targeted within the higher region. The data are equally dispersed within the higher portion of the efficient zone, consistent with the funnel plot connected with this analysis (see Fig. 2 ), indicating that publication bias is unlikely in this situation.
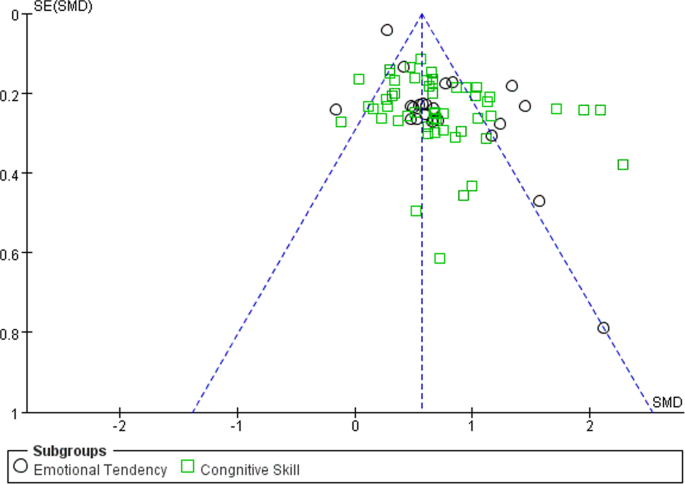
This funnel plot shows the result of publication bias of 79 effect quantities across 36 studies.
Heterogeneity test
To select the appropriate effect models for the meta-analysis, one might use the results of a heterogeneity test on the data effect sizes. In a meta-analysis, it is common practice to gauge the degree of data heterogeneity using the I 2 value, and I 2 ≥ 50% is typically understood to denote medium-high heterogeneity, which calls for the adoption of a random effect model; if not, a fixed effect model ought to be applied (Lipsey and Wilson, 2001 ). The findings of the heterogeneity test in this paper (see Table 2 ) revealed that I 2 was 86% and displayed significant heterogeneity ( P < 0.01). To ensure accuracy and reliability, the overall effect size ought to be calculated utilizing the random effect model.
The analysis of the overall effect size
This meta-analysis utilized a random effect model to examine 79 effect quantities from 36 studies after eliminating heterogeneity. In accordance with Cohen’s criterion (Cohen, 1992 ), it is abundantly clear from the analysis results, which are shown in the forest plot of the overall effect (see Fig. 3 ), that the cumulative impact size of cooperative problem-solving is 0.82, which is statistically significant ( z = 12.78, P < 0.01, 95% CI [0.69, 0.95]), and can encourage learners to practice critical thinking.
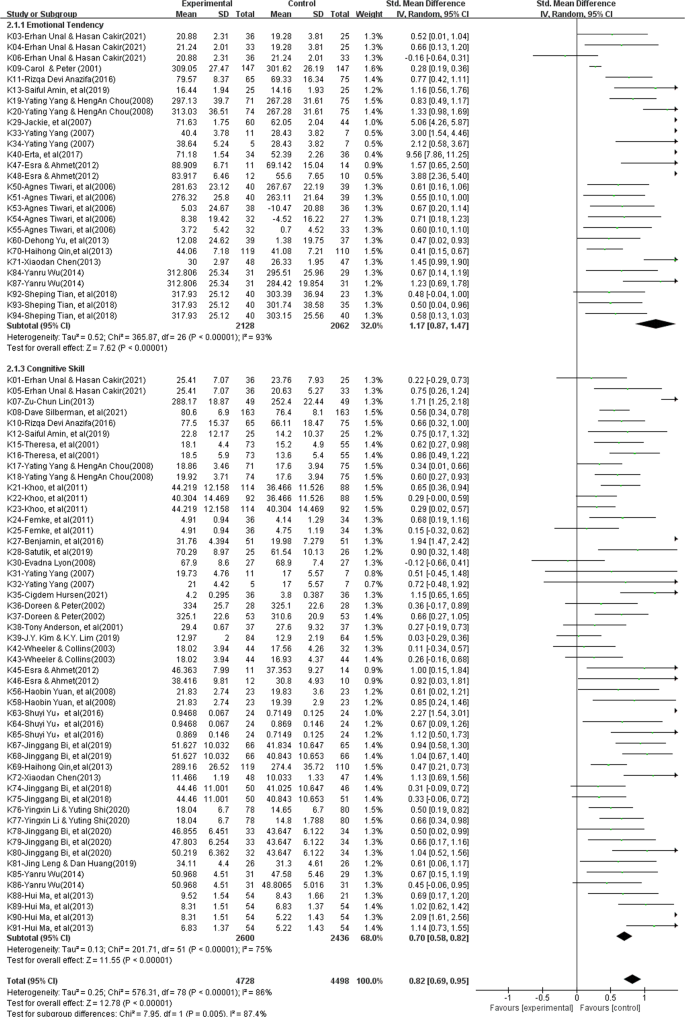
This forest plot shows the analysis result of the overall effect size across 36 studies.
In addition, this study examined two distinct dimensions of critical thinking to better understand the precise contributions that collaborative problem-solving makes to the growth of critical thinking. The findings (see Table 3 ) indicate that collaborative problem-solving improves cognitive skills (ES = 0.70) and attitudinal tendency (ES = 1.17), with significant intergroup differences (chi 2 = 7.95, P < 0.01). Although collaborative problem-solving improves both dimensions of critical thinking, it is essential to point out that the improvements in students’ attitudinal tendency are much more pronounced and have a significant comprehensive effect (ES = 1.17, z = 7.62, P < 0.01, 95% CI [0.87, 1.47]), whereas gains in learners’ cognitive skill are slightly improved and are just above average. (ES = 0.70, z = 11.55, P < 0.01, 95% CI [0.58, 0.82]).
The analysis of moderator effect size
The whole forest plot’s 79 effect quantities underwent a two-tailed test, which revealed significant heterogeneity ( I 2 = 86%, z = 12.78, P < 0.01), indicating differences between various effect sizes that may have been influenced by moderating factors other than sampling error. Therefore, exploring possible moderating factors that might produce considerable heterogeneity was done using subgroup analysis, such as the learning stage, learning scaffold, teaching type, group size, duration of the intervention, measuring tool, and the subject area included in the 36 experimental designs, in order to further explore the key factors that influence critical thinking. The findings (see Table 4 ) indicate that various moderating factors have advantageous effects on critical thinking. In this situation, the subject area (chi 2 = 13.36, P < 0.05), group size (chi 2 = 8.77, P < 0.05), intervention duration (chi 2 = 12.18, P < 0.01), learning scaffold (chi 2 = 9.03, P < 0.01), and teaching type (chi 2 = 7.20, P < 0.05) are all significant moderators that can be applied to support the cultivation of critical thinking. However, since the learning stage and the measuring tools did not significantly differ among intergroup (chi 2 = 3.15, P = 0.21 > 0.05, and chi 2 = 0.08, P = 0.78 > 0.05), we are unable to explain why these two factors are crucial in supporting the cultivation of critical thinking in the context of collaborative problem-solving. These are the precise outcomes, as follows:
Various learning stages influenced critical thinking positively, without significant intergroup differences (chi 2 = 3.15, P = 0.21 > 0.05). High school was first on the list of effect sizes (ES = 1.36, P < 0.01), then higher education (ES = 0.78, P < 0.01), and middle school (ES = 0.73, P < 0.01). These results show that, despite the learning stage’s beneficial influence on cultivating learners’ critical thinking, we are unable to explain why it is essential for cultivating critical thinking in the context of collaborative problem-solving.
Different teaching types had varying degrees of positive impact on critical thinking, with significant intergroup differences (chi 2 = 7.20, P < 0.05). The effect size was ranked as follows: mixed courses (ES = 1.34, P < 0.01), integrated courses (ES = 0.81, P < 0.01), and independent courses (ES = 0.27, P < 0.01). These results indicate that the most effective approach to cultivate critical thinking utilizing collaborative problem solving is through the teaching type of mixed courses.
Various intervention durations significantly improved critical thinking, and there were significant intergroup differences (chi 2 = 12.18, P < 0.01). The effect sizes related to this variable showed a tendency to increase with longer intervention durations. The improvement in critical thinking reached a significant level (ES = 0.85, P < 0.01) after more than 12 weeks of training. These findings indicate that the intervention duration and critical thinking’s impact are positively correlated, with a longer intervention duration having a greater effect.
Different learning scaffolds influenced critical thinking positively, with significant intergroup differences (chi 2 = 9.03, P < 0.01). The resource-supported learning scaffold (ES = 0.69, P < 0.01) acquired a medium-to-higher level of impact, the technique-supported learning scaffold (ES = 0.63, P < 0.01) also attained a medium-to-higher level of impact, and the teacher-supported learning scaffold (ES = 0.92, P < 0.01) displayed a high level of significant impact. These results show that the learning scaffold with teacher support has the greatest impact on cultivating critical thinking.
Various group sizes influenced critical thinking positively, and the intergroup differences were statistically significant (chi 2 = 8.77, P < 0.05). Critical thinking showed a general declining trend with increasing group size. The overall effect size of 2–3 people in this situation was the biggest (ES = 0.99, P < 0.01), and when the group size was greater than 7 people, the improvement in critical thinking was at the lower-middle level (ES < 0.5, P < 0.01). These results show that the impact on critical thinking is positively connected with group size, and as group size grows, so does the overall impact.
Various measuring tools influenced critical thinking positively, with significant intergroup differences (chi 2 = 0.08, P = 0.78 > 0.05). In this situation, the self-adapting measurement tools obtained an upper-medium level of effect (ES = 0.78), whereas the complete effect size of the standardized measurement tools was the largest, achieving a significant level of effect (ES = 0.84, P < 0.01). These results show that, despite the beneficial influence of the measuring tool on cultivating critical thinking, we are unable to explain why it is crucial in fostering the growth of critical thinking by utilizing the approach of collaborative problem-solving.
Different subject areas had a greater impact on critical thinking, and the intergroup differences were statistically significant (chi 2 = 13.36, P < 0.05). Mathematics had the greatest overall impact, achieving a significant level of effect (ES = 1.68, P < 0.01), followed by science (ES = 1.25, P < 0.01) and medical science (ES = 0.87, P < 0.01), both of which also achieved a significant level of effect. Programming technology was the least effective (ES = 0.39, P < 0.01), only having a medium-low degree of effect compared to education (ES = 0.72, P < 0.01) and other fields (such as language, art, and social sciences) (ES = 0.58, P < 0.01). These results suggest that scientific fields (e.g., mathematics, science) may be the most effective subject areas for cultivating critical thinking utilizing the approach of collaborative problem-solving.
The effectiveness of collaborative problem solving with regard to teaching critical thinking
According to this meta-analysis, using collaborative problem-solving as an intervention strategy in critical thinking teaching has a considerable amount of impact on cultivating learners’ critical thinking as a whole and has a favorable promotional effect on the two dimensions of critical thinking. According to certain studies, collaborative problem solving, the most frequently used critical thinking teaching strategy in curriculum instruction can considerably enhance students’ critical thinking (e.g., Liang et al., 2017 ; Liu et al., 2020 ; Cindy, 2004 ). This meta-analysis provides convergent data support for the above research views. Thus, the findings of this meta-analysis not only effectively address the first research query regarding the overall effect of cultivating critical thinking and its impact on the two dimensions of critical thinking (i.e., attitudinal tendency and cognitive skills) utilizing the approach of collaborative problem-solving, but also enhance our confidence in cultivating critical thinking by using collaborative problem-solving intervention approach in the context of classroom teaching.
Furthermore, the associated improvements in attitudinal tendency are much stronger, but the corresponding improvements in cognitive skill are only marginally better. According to certain studies, cognitive skill differs from the attitudinal tendency in classroom instruction; the cultivation and development of the former as a key ability is a process of gradual accumulation, while the latter as an attitude is affected by the context of the teaching situation (e.g., a novel and exciting teaching approach, challenging and rewarding tasks) (Halpern, 2001 ; Wei and Hong, 2022 ). Collaborative problem-solving as a teaching approach is exciting and interesting, as well as rewarding and challenging; because it takes the learners as the focus and examines problems with poor structure in real situations, and it can inspire students to fully realize their potential for problem-solving, which will significantly improve their attitudinal tendency toward solving problems (Liu et al., 2020 ). Similar to how collaborative problem-solving influences attitudinal tendency, attitudinal tendency impacts cognitive skill when attempting to solve a problem (Liu et al., 2020 ; Zhang et al., 2022 ), and stronger attitudinal tendencies are associated with improved learning achievement and cognitive ability in students (Sison, 2008 ; Zhang et al., 2022 ). It can be seen that the two specific dimensions of critical thinking as well as critical thinking as a whole are affected by collaborative problem-solving, and this study illuminates the nuanced links between cognitive skills and attitudinal tendencies with regard to these two dimensions of critical thinking. To fully develop students’ capacity for critical thinking, future empirical research should pay closer attention to cognitive skills.
The moderating effects of collaborative problem solving with regard to teaching critical thinking
In order to further explore the key factors that influence critical thinking, exploring possible moderating effects that might produce considerable heterogeneity was done using subgroup analysis. The findings show that the moderating factors, such as the teaching type, learning stage, group size, learning scaffold, duration of the intervention, measuring tool, and the subject area included in the 36 experimental designs, could all support the cultivation of collaborative problem-solving in critical thinking. Among them, the effect size differences between the learning stage and measuring tool are not significant, which does not explain why these two factors are crucial in supporting the cultivation of critical thinking utilizing the approach of collaborative problem-solving.
In terms of the learning stage, various learning stages influenced critical thinking positively without significant intergroup differences, indicating that we are unable to explain why it is crucial in fostering the growth of critical thinking.
Although high education accounts for 70.89% of all empirical studies performed by researchers, high school may be the appropriate learning stage to foster students’ critical thinking by utilizing the approach of collaborative problem-solving since it has the largest overall effect size. This phenomenon may be related to student’s cognitive development, which needs to be further studied in follow-up research.
With regard to teaching type, mixed course teaching may be the best teaching method to cultivate students’ critical thinking. Relevant studies have shown that in the actual teaching process if students are trained in thinking methods alone, the methods they learn are isolated and divorced from subject knowledge, which is not conducive to their transfer of thinking methods; therefore, if students’ thinking is trained only in subject teaching without systematic method training, it is challenging to apply to real-world circumstances (Ruggiero, 2012 ; Hu and Liu, 2015 ). Teaching critical thinking as mixed course teaching in parallel to other subject teachings can achieve the best effect on learners’ critical thinking, and explicit critical thinking instruction is more effective than less explicit critical thinking instruction (Bensley and Spero, 2014 ).
In terms of the intervention duration, with longer intervention times, the overall effect size shows an upward tendency. Thus, the intervention duration and critical thinking’s impact are positively correlated. Critical thinking, as a key competency for students in the 21st century, is difficult to get a meaningful improvement in a brief intervention duration. Instead, it could be developed over a lengthy period of time through consistent teaching and the progressive accumulation of knowledge (Halpern, 2001 ; Hu and Liu, 2015 ). Therefore, future empirical studies ought to take these restrictions into account throughout a longer period of critical thinking instruction.
With regard to group size, a group size of 2–3 persons has the highest effect size, and the comprehensive effect size decreases with increasing group size in general. This outcome is in line with some research findings; as an example, a group composed of two to four members is most appropriate for collaborative learning (Schellens and Valcke, 2006 ). However, the meta-analysis results also indicate that once the group size exceeds 7 people, small groups cannot produce better interaction and performance than large groups. This may be because the learning scaffolds of technique support, resource support, and teacher support improve the frequency and effectiveness of interaction among group members, and a collaborative group with more members may increase the diversity of views, which is helpful to cultivate critical thinking utilizing the approach of collaborative problem-solving.
With regard to the learning scaffold, the three different kinds of learning scaffolds can all enhance critical thinking. Among them, the teacher-supported learning scaffold has the largest overall effect size, demonstrating the interdependence of effective learning scaffolds and collaborative problem-solving. This outcome is in line with some research findings; as an example, a successful strategy is to encourage learners to collaborate, come up with solutions, and develop critical thinking skills by using learning scaffolds (Reiser, 2004 ; Xu et al., 2022 ); learning scaffolds can lower task complexity and unpleasant feelings while also enticing students to engage in learning activities (Wood et al., 2006 ); learning scaffolds are designed to assist students in using learning approaches more successfully to adapt the collaborative problem-solving process, and the teacher-supported learning scaffolds have the greatest influence on critical thinking in this process because they are more targeted, informative, and timely (Xu et al., 2022 ).
With respect to the measuring tool, despite the fact that standardized measurement tools (such as the WGCTA, CCTT, and CCTST) have been acknowledged as trustworthy and effective by worldwide experts, only 54.43% of the research included in this meta-analysis adopted them for assessment, and the results indicated no intergroup differences. These results suggest that not all teaching circumstances are appropriate for measuring critical thinking using standardized measurement tools. “The measuring tools for measuring thinking ability have limits in assessing learners in educational situations and should be adapted appropriately to accurately assess the changes in learners’ critical thinking.”, according to Simpson and Courtney ( 2002 , p. 91). As a result, in order to more fully and precisely gauge how learners’ critical thinking has evolved, we must properly modify standardized measuring tools based on collaborative problem-solving learning contexts.
With regard to the subject area, the comprehensive effect size of science departments (e.g., mathematics, science, medical science) is larger than that of language arts and social sciences. Some recent international education reforms have noted that critical thinking is a basic part of scientific literacy. Students with scientific literacy can prove the rationality of their judgment according to accurate evidence and reasonable standards when they face challenges or poorly structured problems (Kyndt et al., 2013 ), which makes critical thinking crucial for developing scientific understanding and applying this understanding to practical problem solving for problems related to science, technology, and society (Yore et al., 2007 ).
Suggestions for critical thinking teaching
Other than those stated in the discussion above, the following suggestions are offered for critical thinking instruction utilizing the approach of collaborative problem-solving.
First, teachers should put a special emphasis on the two core elements, which are collaboration and problem-solving, to design real problems based on collaborative situations. This meta-analysis provides evidence to support the view that collaborative problem-solving has a strong synergistic effect on promoting students’ critical thinking. Asking questions about real situations and allowing learners to take part in critical discussions on real problems during class instruction are key ways to teach critical thinking rather than simply reading speculative articles without practice (Mulnix, 2012 ). Furthermore, the improvement of students’ critical thinking is realized through cognitive conflict with other learners in the problem situation (Yang et al., 2008 ). Consequently, it is essential for teachers to put a special emphasis on the two core elements, which are collaboration and problem-solving, and design real problems and encourage students to discuss, negotiate, and argue based on collaborative problem-solving situations.
Second, teachers should design and implement mixed courses to cultivate learners’ critical thinking, utilizing the approach of collaborative problem-solving. Critical thinking can be taught through curriculum instruction (Kuncel, 2011 ; Leng and Lu, 2020 ), with the goal of cultivating learners’ critical thinking for flexible transfer and application in real problem-solving situations. This meta-analysis shows that mixed course teaching has a highly substantial impact on the cultivation and promotion of learners’ critical thinking. Therefore, teachers should design and implement mixed course teaching with real collaborative problem-solving situations in combination with the knowledge content of specific disciplines in conventional teaching, teach methods and strategies of critical thinking based on poorly structured problems to help students master critical thinking, and provide practical activities in which students can interact with each other to develop knowledge construction and critical thinking utilizing the approach of collaborative problem-solving.
Third, teachers should be more trained in critical thinking, particularly preservice teachers, and they also should be conscious of the ways in which teachers’ support for learning scaffolds can promote critical thinking. The learning scaffold supported by teachers had the greatest impact on learners’ critical thinking, in addition to being more directive, targeted, and timely (Wood et al., 2006 ). Critical thinking can only be effectively taught when teachers recognize the significance of critical thinking for students’ growth and use the proper approaches while designing instructional activities (Forawi, 2016 ). Therefore, with the intention of enabling teachers to create learning scaffolds to cultivate learners’ critical thinking utilizing the approach of collaborative problem solving, it is essential to concentrate on the teacher-supported learning scaffolds and enhance the instruction for teaching critical thinking to teachers, especially preservice teachers.
Implications and limitations
There are certain limitations in this meta-analysis, but future research can correct them. First, the search languages were restricted to English and Chinese, so it is possible that pertinent studies that were written in other languages were overlooked, resulting in an inadequate number of articles for review. Second, these data provided by the included studies are partially missing, such as whether teachers were trained in the theory and practice of critical thinking, the average age and gender of learners, and the differences in critical thinking among learners of various ages and genders. Third, as is typical for review articles, more studies were released while this meta-analysis was being done; therefore, it had a time limit. With the development of relevant research, future studies focusing on these issues are highly relevant and needed.
Conclusions
The subject of the magnitude of collaborative problem-solving’s impact on fostering students’ critical thinking, which received scant attention from other studies, was successfully addressed by this study. The question of the effectiveness of collaborative problem-solving in promoting students’ critical thinking was addressed in this study, which addressed a topic that had gotten little attention in earlier research. The following conclusions can be made:
Regarding the results obtained, collaborative problem solving is an effective teaching approach to foster learners’ critical thinking, with a significant overall effect size (ES = 0.82, z = 12.78, P < 0.01, 95% CI [0.69, 0.95]). With respect to the dimensions of critical thinking, collaborative problem-solving can significantly and effectively improve students’ attitudinal tendency, and the comprehensive effect is significant (ES = 1.17, z = 7.62, P < 0.01, 95% CI [0.87, 1.47]); nevertheless, it falls short in terms of improving students’ cognitive skills, having only an upper-middle impact (ES = 0.70, z = 11.55, P < 0.01, 95% CI [0.58, 0.82]).
As demonstrated by both the results and the discussion, there are varying degrees of beneficial effects on students’ critical thinking from all seven moderating factors, which were found across 36 studies. In this context, the teaching type (chi 2 = 7.20, P < 0.05), intervention duration (chi 2 = 12.18, P < 0.01), subject area (chi 2 = 13.36, P < 0.05), group size (chi 2 = 8.77, P < 0.05), and learning scaffold (chi 2 = 9.03, P < 0.01) all have a positive impact on critical thinking, and they can be viewed as important moderating factors that affect how critical thinking develops. Since the learning stage (chi 2 = 3.15, P = 0.21 > 0.05) and measuring tools (chi 2 = 0.08, P = 0.78 > 0.05) did not demonstrate any significant intergroup differences, we are unable to explain why these two factors are crucial in supporting the cultivation of critical thinking in the context of collaborative problem-solving.
Data availability
All data generated or analyzed during this study are included within the article and its supplementary information files, and the supplementary information files are available in the Dataverse repository: https://doi.org/10.7910/DVN/IPFJO6 .
Bensley DA, Spero RA (2014) Improving critical thinking skills and meta-cognitive monitoring through direct infusion. Think Skills Creat 12:55–68. https://doi.org/10.1016/j.tsc.2014.02.001
Article Google Scholar
Castle A (2009) Defining and assessing critical thinking skills for student radiographers. Radiography 15(1):70–76. https://doi.org/10.1016/j.radi.2007.10.007
Chen XD (2013) An empirical study on the influence of PBL teaching model on critical thinking ability of non-English majors. J PLA Foreign Lang College 36 (04):68–72
Google Scholar
Cohen A (1992) Antecedents of organizational commitment across occupational groups: a meta-analysis. J Organ Behav. https://doi.org/10.1002/job.4030130602
Cooper H (2010) Research synthesis and meta-analysis: a step-by-step approach, 4th edn. Sage, London, England
Cindy HS (2004) Problem-based learning: what and how do students learn? Educ Psychol Rev 51(1):31–39
Duch BJ, Gron SD, Allen DE (2001) The power of problem-based learning: a practical “how to” for teaching undergraduate courses in any discipline. Stylus Educ Sci 2:190–198
Ennis RH (1989) Critical thinking and subject specificity: clarification and needed research. Educ Res 18(3):4–10. https://doi.org/10.3102/0013189x018003004
Facione PA (1990) Critical thinking: a statement of expert consensus for purposes of educational assessment and instruction. Research findings and recommendations. Eric document reproduction service. https://eric.ed.gov/?id=ed315423
Facione PA, Facione NC (1992) The California Critical Thinking Dispositions Inventory (CCTDI) and the CCTDI test manual. California Academic Press, Millbrae, CA
Forawi SA (2016) Standard-based science education and critical thinking. Think Skills Creat 20:52–62. https://doi.org/10.1016/j.tsc.2016.02.005
Halpern DF (2001) Assessing the effectiveness of critical thinking instruction. J Gen Educ 50(4):270–286. https://doi.org/10.2307/27797889
Hu WP, Liu J (2015) Cultivation of pupils’ thinking ability: a five-year follow-up study. Psychol Behav Res 13(05):648–654. https://doi.org/10.3969/j.issn.1672-0628.2015.05.010
Huber K (2016) Does college teach critical thinking? A meta-analysis. Rev Educ Res 86(2):431–468. https://doi.org/10.3102/0034654315605917
Kek MYCA, Huijser H (2011) The power of problem-based learning in developing critical thinking skills: preparing students for tomorrow’s digital futures in today’s classrooms. High Educ Res Dev 30(3):329–341. https://doi.org/10.1080/07294360.2010.501074
Kuncel NR (2011) Measurement and meaning of critical thinking (Research report for the NRC 21st Century Skills Workshop). National Research Council, Washington, DC
Kyndt E, Raes E, Lismont B, Timmers F, Cascallar E, Dochy F (2013) A meta-analysis of the effects of face-to-face cooperative learning. Do recent studies falsify or verify earlier findings? Educ Res Rev 10(2):133–149. https://doi.org/10.1016/j.edurev.2013.02.002
Leng J, Lu XX (2020) Is critical thinking really teachable?—A meta-analysis based on 79 experimental or quasi experimental studies. Open Educ Res 26(06):110–118. https://doi.org/10.13966/j.cnki.kfjyyj.2020.06.011
Liang YZ, Zhu K, Zhao CL (2017) An empirical study on the depth of interaction promoted by collaborative problem solving learning activities. J E-educ Res 38(10):87–92. https://doi.org/10.13811/j.cnki.eer.2017.10.014
Lipsey M, Wilson D (2001) Practical meta-analysis. International Educational and Professional, London, pp. 92–160
Liu Z, Wu W, Jiang Q (2020) A study on the influence of problem based learning on college students’ critical thinking-based on a meta-analysis of 31 studies. Explor High Educ 03:43–49
Morris SB (2008) Estimating effect sizes from pretest-posttest-control group designs. Organ Res Methods 11(2):364–386. https://doi.org/10.1177/1094428106291059
Article ADS Google Scholar
Mulnix JW (2012) Thinking critically about critical thinking. Educ Philos Theory 44(5):464–479. https://doi.org/10.1111/j.1469-5812.2010.00673.x
Naber J, Wyatt TH (2014) The effect of reflective writing interventions on the critical thinking skills and dispositions of baccalaureate nursing students. Nurse Educ Today 34(1):67–72. https://doi.org/10.1016/j.nedt.2013.04.002
National Research Council (2012) Education for life and work: developing transferable knowledge and skills in the 21st century. The National Academies Press, Washington, DC
Niu L, Behar HLS, Garvan CW (2013) Do instructional interventions influence college students’ critical thinking skills? A meta-analysis. Educ Res Rev 9(12):114–128. https://doi.org/10.1016/j.edurev.2012.12.002
Peng ZM, Deng L (2017) Towards the core of education reform: cultivating critical thinking skills as the core of skills in the 21st century. Res Educ Dev 24:57–63. https://doi.org/10.14121/j.cnki.1008-3855.2017.24.011
Reiser BJ (2004) Scaffolding complex learning: the mechanisms of structuring and problematizing student work. J Learn Sci 13(3):273–304. https://doi.org/10.1207/s15327809jls1303_2
Ruggiero VR (2012) The art of thinking: a guide to critical and creative thought, 4th edn. Harper Collins College Publishers, New York
Schellens T, Valcke M (2006) Fostering knowledge construction in university students through asynchronous discussion groups. Comput Educ 46(4):349–370. https://doi.org/10.1016/j.compedu.2004.07.010
Sendag S, Odabasi HF (2009) Effects of an online problem based learning course on content knowledge acquisition and critical thinking skills. Comput Educ 53(1):132–141. https://doi.org/10.1016/j.compedu.2009.01.008
Sison R (2008) Investigating Pair Programming in a Software Engineering Course in an Asian Setting. 2008 15th Asia-Pacific Software Engineering Conference, pp. 325–331. https://doi.org/10.1109/APSEC.2008.61
Simpson E, Courtney M (2002) Critical thinking in nursing education: literature review. Mary Courtney 8(2):89–98
Stewart L, Tierney J, Burdett S (2006) Do systematic reviews based on individual patient data offer a means of circumventing biases associated with trial publications? Publication bias in meta-analysis. John Wiley and Sons Inc, New York, pp. 261–286
Tiwari A, Lai P, So M, Yuen K (2010) A comparison of the effects of problem-based learning and lecturing on the development of students’ critical thinking. Med Educ 40(6):547–554. https://doi.org/10.1111/j.1365-2929.2006.02481.x
Wood D, Bruner JS, Ross G (2006) The role of tutoring in problem solving. J Child Psychol Psychiatry 17(2):89–100. https://doi.org/10.1111/j.1469-7610.1976.tb00381.x
Wei T, Hong S (2022) The meaning and realization of teachable critical thinking. Educ Theory Practice 10:51–57
Xu EW, Wang W, Wang QX (2022) A meta-analysis of the effectiveness of programming teaching in promoting K-12 students’ computational thinking. Educ Inf Technol. https://doi.org/10.1007/s10639-022-11445-2
Yang YC, Newby T, Bill R (2008) Facilitating interactions through structured web-based bulletin boards: a quasi-experimental study on promoting learners’ critical thinking skills. Comput Educ 50(4):1572–1585. https://doi.org/10.1016/j.compedu.2007.04.006
Yore LD, Pimm D, Tuan HL (2007) The literacy component of mathematical and scientific literacy. Int J Sci Math Educ 5(4):559–589. https://doi.org/10.1007/s10763-007-9089-4
Zhang T, Zhang S, Gao QQ, Wang JH (2022) Research on the development of learners’ critical thinking in online peer review. Audio Visual Educ Res 6:53–60. https://doi.org/10.13811/j.cnki.eer.2022.06.08
Download references
Acknowledgements
This research was supported by the graduate scientific research and innovation project of Xinjiang Uygur Autonomous Region named “Research on in-depth learning of high school information technology courses for the cultivation of computing thinking” (No. XJ2022G190) and the independent innovation fund project for doctoral students of the College of Educational Science of Xinjiang Normal University named “Research on project-based teaching of high school information technology courses from the perspective of discipline core literacy” (No. XJNUJKYA2003).
Author information
Authors and affiliations.
College of Educational Science, Xinjiang Normal University, 830017, Urumqi, Xinjiang, China
Enwei Xu, Wei Wang & Qingxia Wang
You can also search for this author in PubMed Google Scholar
Corresponding authors
Correspondence to Enwei Xu or Wei Wang .
Ethics declarations
Competing interests.
The authors declare no competing interests.
Ethical approval
This article does not contain any studies with human participants performed by any of the authors.
Informed consent
Additional information.
Publisher’s note Springer Nature remains neutral with regard to jurisdictional claims in published maps and institutional affiliations.
Supplementary information
Supplementary tables, rights and permissions.
Open Access This article is licensed under a Creative Commons Attribution 4.0 International License, which permits use, sharing, adaptation, distribution and reproduction in any medium or format, as long as you give appropriate credit to the original author(s) and the source, provide a link to the Creative Commons license, and indicate if changes were made. The images or other third party material in this article are included in the article’s Creative Commons license, unless indicated otherwise in a credit line to the material. If material is not included in the article’s Creative Commons license and your intended use is not permitted by statutory regulation or exceeds the permitted use, you will need to obtain permission directly from the copyright holder. To view a copy of this license, visit http://creativecommons.org/licenses/by/4.0/ .
Reprints and permissions
About this article
Cite this article.
Xu, E., Wang, W. & Wang, Q. The effectiveness of collaborative problem solving in promoting students’ critical thinking: A meta-analysis based on empirical literature. Humanit Soc Sci Commun 10 , 16 (2023). https://doi.org/10.1057/s41599-023-01508-1
Download citation
Received : 07 August 2022
Accepted : 04 January 2023
Published : 11 January 2023
DOI : https://doi.org/10.1057/s41599-023-01508-1
Share this article
Anyone you share the following link with will be able to read this content:
Sorry, a shareable link is not currently available for this article.
Provided by the Springer Nature SharedIt content-sharing initiative
This article is cited by
Impacts of online collaborative learning on students’ intercultural communication apprehension and intercultural communicative competence.
- Hoa Thi Hoang Chau
- Hung Phu Bui
- Quynh Thi Huong Dinh
Education and Information Technologies (2024)
Exploring the effects of digital technology on deep learning: a meta-analysis
Sustainable electricity generation and farm-grid utilization from photovoltaic aquaculture: a bibliometric analysis.
- A. A. Amusa
- M. Alhassan
International Journal of Environmental Science and Technology (2024)
Quick links
- Explore articles by subject
- Guide to authors
- Editorial policies

- Reference Manager
- Simple TEXT file
People also looked at
Original research article, creative problem solving as overcoming a misunderstanding.
- Department of Psychology, University of Milano-Bicocca, Milan, Italy
Solving or attempting to solve problems is the typical and, hence, general function of thought. A theory of problem solving must first explain how the problem is constituted, and then how the solution happens, but also how it happens that it is not solved; it must explain the correct answer and with the same means the failure. The identification of the way in which the problem is formatted should help to understand how the solution of the problems happens, but even before that, the source of the difficulty. Sometimes the difficulty lies in the calculation, the number of operations to be performed, and the quantity of data to be processed and remembered. There are, however, other problems – the insight problems – in which the difficulty does not lie so much in the complexity of the calculations, but in one or more critical points that are susceptible to misinterpretation , incompatible with the solution. In our view, the way of thinking involved in insight problem solving is very close to the process involved in the understanding of an utterance, when a misunderstanding occurs. In this case, a more appropriate meaning has to be selected to resolve the misunderstanding (the “impasse”), the default interpretation (the “fixation”) has to be dropped in order to “restructure.” to grasp another meaning which appears more relevant to the context and the speaker’s intention (the “aim of the task”). In this article we support our view with experimental evidence, focusing on how a misunderstanding is formed. We have studied a paradigmatic insight problem, an apparent trivial arithmetical task, the Ties problem. We also reviewed other classical insight problems, reconsidering in particular one of the most intriguing one, which at first sight appears impossible to solve, the Study Window problem. By identifying the problem knots that alter the aim of the task, the reformulation technique has made it possible to eliminate misunderstanding, without changing the mathematical nature of the problem. With the experimental versions of the problems exposed we have obtained a significant increase in correct answers. Studying how an insight problem is formed, and not just how it is solved, may well become an important topic in education. We focus on undergraduate students’ strategies and their errors while solving problems, and the specific cognitive processes involved in misunderstanding, which are crucial to better exploit what could be beneficial to reach the solution and to teach how to improve the ability to solve problems.
Introduction
“A problem arises when a living creature has a goal but does not know how this goal is to be reached. Whenever one cannot go from the given situation to the desired situation simply by action, then there has to be recourse to thinking. (…) Such thinking has the task of devising some action which may mediate between the existing and the desired situations.” ( Duncker, 1945 , p. 1). We agree with Duncker’s general description of every situation we call a problem: the problem solving activity takes a central role in the general function of thought, if not even identifies with it.
So far, psychologists have been mainly interested in the solution and the solvers. But the formation of the problem remained in the shadows.
Let’s consider for example the two fundamental theoretical approaches to the study of problem solving. “What questions should a theory of problem solving answer? First, it should predict the performance of a problem solver handling specified tasks. It should explain how human problem solving takes place: what processes are used, and what mechanisms perform these processes.” ( Newell et al., 1958 , p. 151). In turn, authors of different orientations indicate as central in their research “How does the solution arise from the problem situation? In what ways is the solution of a problem attained?” ( Duncker, 1945 , p. 1) or that of what happens when you solve a problem, when you suddenly see the point ( Wertheimer, 1959 ). It is obvious, and it was inevitable, that the formation of the problem would remain in the shadows.
A theory of problem solving must first explain how the problem is constituted, and then how the solution happens, but also how it happens that it is not solved; it must explain the correct answer and with the same means the failure. The identification of the way in which the problem is constituted – the formation of the problem – and the awareness that this moment is decisive for everything that follows imply that failures are considered in a new way, the study of which should help to understand how the solution of the problems happens, but even before that, the source of the difficulty.
Sometimes the difficulty lies in the calculation, the number of operations to be performed, and the quantity of data to be processed and remembered. Take the well-known problems studied by Simon, Crypto-arithmetic task, for example, or the Cannibals and Missionaries problem ( Simon, 1979 ). The difficulty in these problems lies in the complexity of the calculation which characterizes them. But, the text and the request of the problem is univocally understood by the experimenter and by the participant in both the explicit ( said )and implicit ( implied ) parts. 1 As Simon says, “Subjects do not initially choose deliberately among problem representations, but almost always adopt the representation suggested by the verbal problem statement” ( Kaplan and Simon, 1990 , p. 376). The verbal problem statement determines a problem representation, implicit presuppositions of which are shared by both.
There are, however, other problems where the usual (generalized) interpretation of the text of the problem (and/or the associated figure) prevents and does not allow a solution to be found, so that we are soon faced with an impasse. We’ll call this kind of problems insight problems . “In these cases, where the complexity of the calculations does not play a relevant part in the difficulty of the problem, a misunderstanding would appear to be a more appropriate abstract model than the labyrinth” ( Mosconi, 2016 , p. 356). Insight problems do not arise from a fortuitous misunderstanding, but from a deliberate violation of Gricean conversational rules, since the implicit layer of the discourse (the implied ) is not shared both by experimenter and participant. Take for example the problem of how to remove a one-hundred dollar bill without causing a pyramid balanced atop the bill to topple: “A giant inverted steel pyramid is perfectly balanced on its point. Any movement of the pyramid will cause it to topple over. Underneath the pyramid is a $100 bill. How would you remove the bill without disturbing the pyramid?” ( Schooler et al., 1993 , p. 183). The solution is burn or tear the dollar bill but people assume that the 100 dollar bill must not be damaged, but contrary to his assumption, this is in fact the solution. Obviously this is not a trivial error of understanding between the two parties, but rather a misunderstanding due to social conventions, and dictated by conversational rules. It is the essential condition for the forming of the problem and the experimenter has played on the very fact that the condition was not explicitly stated (see also Bulbrook, 1932 ).
When insight problems are used in research, it could be said that the researcher sets a trap, more or less intentionally, inducing an interpretation that appears to be pertinent to the data and to the text; this interpretation is adopted more or less automatically because it has been validated by use but the default interpretation does not support understanding, and misunderstanding is inevitable; as a result, sooner or later we come up against an impasse. The theory of misunderstanding is supported by experimental evidence obtained by Mosconi in his research on insight problem solving ( Mosconi, 1990 ), and by Bagassi and Macchi on problem solving, decision making and probabilistic reasoning ( Bagassi and Macchi, 2006 , 2016 ; Macchi and Bagassi, 2012 , 2014 , 2015 , 2020 ; Macchi, 1995 , 2000 ; Mosconi and Macchi, 2001 ; Politzer and Macchi, 2000 ).
The implication of the focus on problem forming for education is remarkable: everything we say generates a communicative and therefore interpretative context, which is given by cultural and social assumptions, default interpretations, and attribution of intention to the speaker. Since the text of the problem is expressed in natural language, it is affected, it shares the characteristics of the language itself. Natural language is ambiguous in itself, differently from specialized languages (i.e., logical and statistical ones), which presuppose a univocal, unambiguous interpretation. The understanding of what a speaker means requires a disambiguation process centered on the intention attribution.
Restructuring as Reinterpreting
Traditionally, according to the Gestaltists, finding the solution to an insight problem is an example of “productive thought.” In addition to the reproductive activities of thought, there are processes which create, “produce” that which does not yet exist. It is characterized by a switch in direction which occurs together with the transformation of the problem or a change in our understanding of an essential relationship. The famous “aha!” experience of genuine insight accompanies this change in representation, or restructuring. As Wertheimer says: “… Solution becomes possible only when the central features of the problem are clearly recognized, and paths to a possible approach emerge. Irrelevant features must be stripped away, core features must become salient, and some representation must be developed that accurately reflects how various parts of the problem fit together; relevant relations among parts, and between parts and whole, must be understood, must make sense” ( Wertheimer, 1985 , p. 23).
The restructuring process circumscribed by the Gestaltists to the representation of the perceptual stimulus is actually a general feature of every human cognitive activity and, in particular, of communicative interaction, which allows the understanding, the attribution of meaning, thus extending to the solution of verbal insight problems. In this sense, restructuring becomes a process of reinterpretation.
We are able to get out of the impasse by neglecting the default interpretation and looking for another one that is more pertinent to the situation and which helps us grasp the meaning that matches both the context and the speaker’s intention; this requires continuous adjustments until all makes sense.
In our perspective, this interpretative function is a characteristic inherent to all reasoning processes and is an adaptive characteristic of the human cognitive system in general ( Levinson, 1995 , 2013 ; Macchi and Bagassi, 2019 ; Mercier and Sperber, 2011 ; Sperber and Wilson, 1986/1995 ; Tomasello, 2009 ). It guarantees cognitive economy when meanings and relations are familiar, permitting recognition in a “blink of an eye.” This same process becomes much more arduous when meanings and relations are unfamiliar, obliging us to face the novel. When this happens, we have to come to terms with the fact that the usual, default interpretation will not work, and this is a necessary condition for exploring other ways of interpreting the situation. A restless, conscious and unconscious search for other possible relations between the parts and the whole ensues until everything falls into place and nothing is left unexplained, with an interpretative heuristic-type process. Indeed, the solution restructuring – is a re -interpretation of the relationship between the data and the aim of the task, a search for the appropriate meaning carried out at a deeper level, not by automaticity. If this is true, then a disambiguant reformulation of the problem that eliminates the trap into which the subject has fallen, should produce restructuring and the way to the solution.
Insight Problem Solving as the Overcoming of a Misunderstanding: The Effect of Reformulation
In this article we support our view with experimental evidence, focusing on how a misunderstanding is formed, and how a pragmatic reformulation of the problem, more relevant to the aim of the task, allows the text of the problem to be interpreted in accordance with the solution.
We consider two paradigmatic insight problems, the intriguing Study Window problem, which at first sight appears impossible to solve, and an apparent trivial arithmetical task, the Ties problem ( Mosconi and D’Urso, 1974 ).
The Study Window problem
The study window measures 1 m in height and 1 m wide. The owner decides to enlarge it and calls in a workman. He instructs the man to double the area of the window without changing its shape and so that it still measures 1 m by 1 m. The workman carried out the commission. How did he do it?
This problem was investigated in a previous study ( Macchi and Bagassi, 2015 ). For all the participants the problem appeared impossible to solve, and nobody actually solved it. The explanation we gave for the difficulty was the following: “The information provided regarding the dimensions brings a square form to mind. The problem solver interprets the window to be a square 1 m high by 1 m wide, resting on one side. Furthermore, the problem states “without changing its shape,” intending geometric shape of the two windows (square, independently of the orientation of the window), while the problem solver interprets this as meaning the phenomenic shape of the two windows (two squares with the same orthogonal orientation)” ( Macchi and Bagassi, 2015 , p. 156). And this is where the difficulty of the problem lies, in the mental representation of the window and the concurrent interpretation of the text of the problem. Actually, spatial orientation is a decisive factor in the perception of forms. “Two identical shapes seen from different orientations take on a different phenomenic identity” ( Mach, 1914 ).
The solution is to be found in a square (geometric form) that “rests” on one of its angles, thus becoming a rhombus (phenomenic form). Now the dimensions given are those of the two diagonals of the represented rhombus (ABCD).
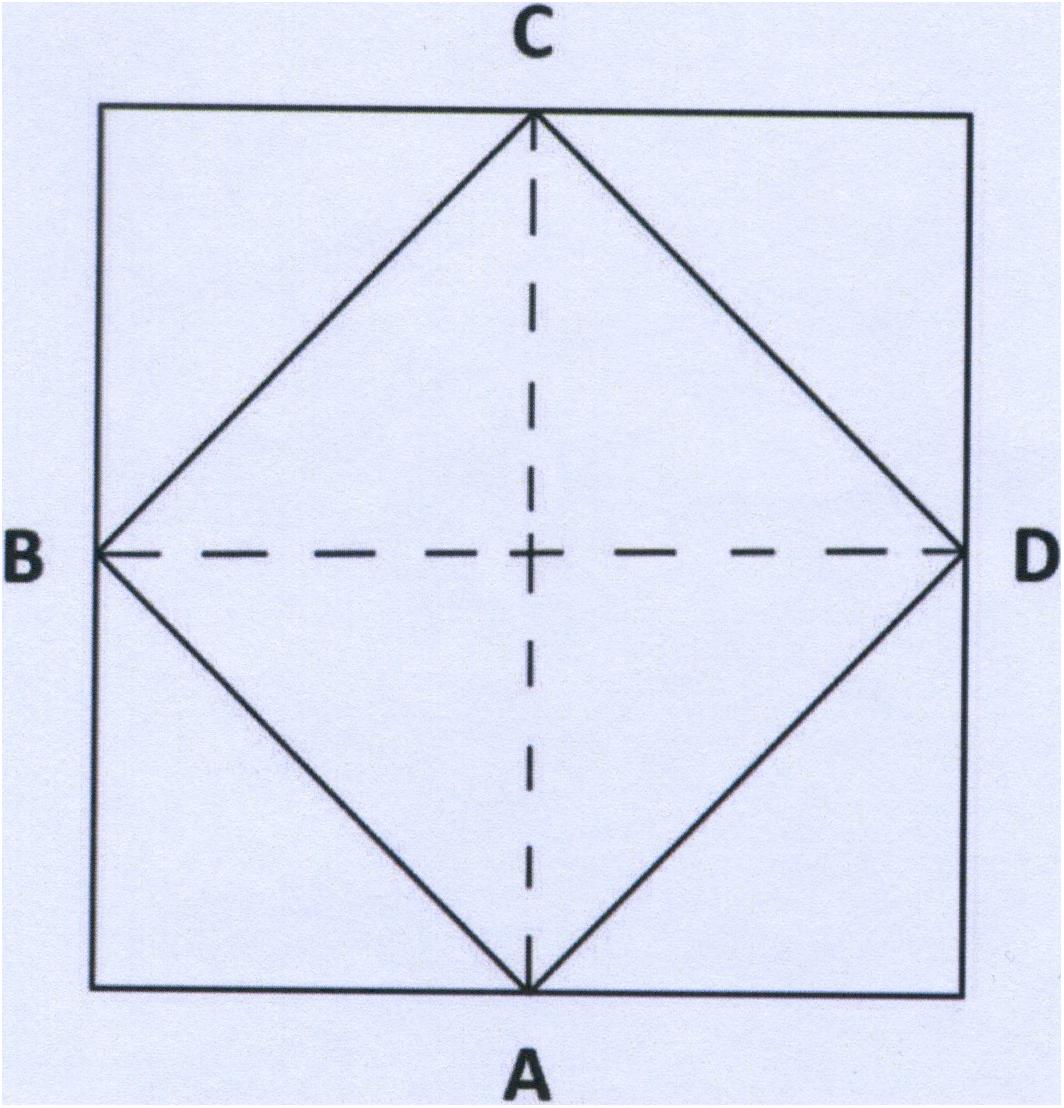
Figure 1. The study window problem solution.
The “inverted” version of the problem gave less trouble:
[…] The owner decides to make it smaller and calls in a workman. He instructs the man to halve the area of the window […].
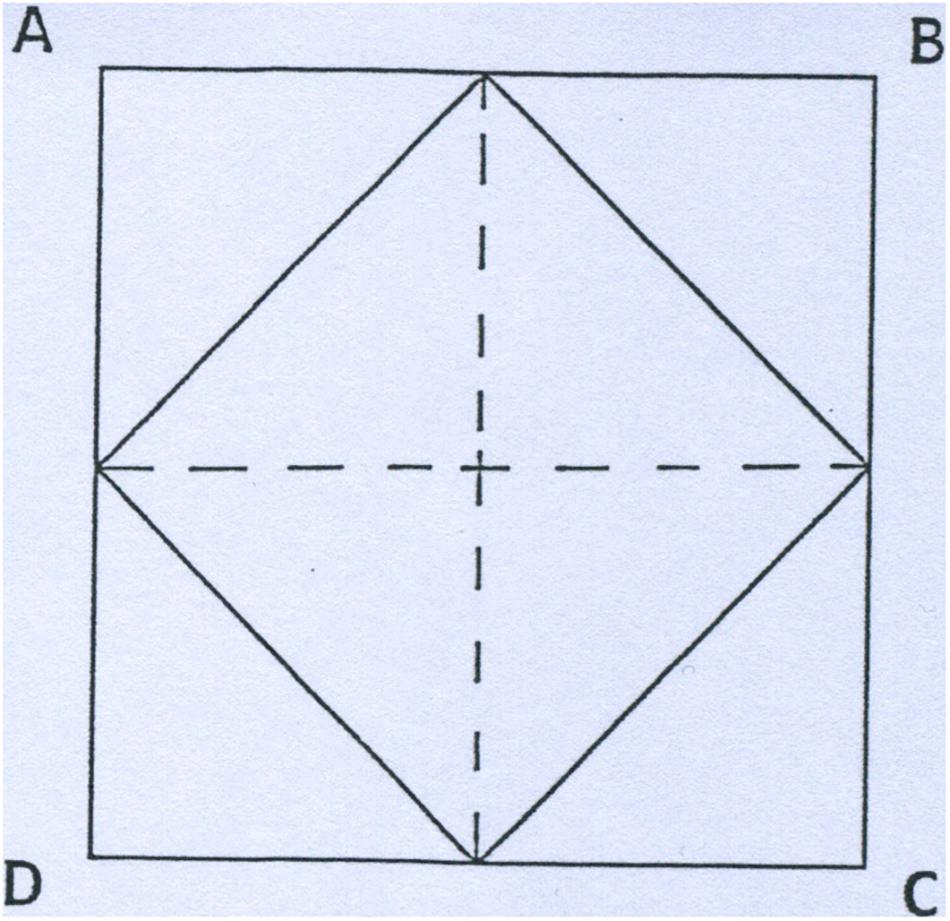
Figure 2. The inverted version.
With this version, 30% of the participants solved the problem ( n = 30). They started from the representation of the orthogonal square (ABCD) and looked for the solution within the square, trying to respect the required height and width of the window, and inevitably changing the orientation of the internal square. This time the height and width are the diagonals, rather than the side (base and height) of the square.
Eventually, in another version (the “orientation” version) it was explicit that orientation was not a mandatory attribute of the shape, and this time 66% of the participants found the solution immediately ( n = 30). This confirms the hypothesis that an inappropriate representation of the relation between the orthogonal orientation of the square and its geometric shape is the origin of the misunderstanding .
The “orientation” version:
A study window measures 1 m in height and 1 m wide. The owner decides to make it smaller and calls in a workman. He instructs the man to halve the area of the window: the workman can change the orientation of the window, but not its shape and in such a way that it still measures one meter by one meter. The workman carries out the commission. How did he do it?
While with the Study window problem the subjects who do not arrive at the solution, and who are the totality, know they are wrong, with the problem we are now going to examine, the Ties problem, those who are wrong do not realize it at all and the solution they propose is experienced as the correct solution.
The Ties Problem ( Mosconi and D’Urso, 1974 )
Peter and John have the same number of ties.
Peter gives John five of his ties.
How many ties does John have now more than Peter?
We believe that the seemingly trivial problem is actually the result of the simultaneous activation and mutual interference of complex cognitive processes that prevent its solution.
The problem has been submitted to 50 undergraduate students of the Humanities Faculty of the University of Milano-Bicocca. The participants were tested individually and were randomly assigned to three groups: control version ( n = 50), experimental version 2 ( n = 20), and experimental version 3 ( n = 23). All groups were tested in Italian. Each participant was randomly assigned to one of the conditions and received a form containing only one version of the two assigned problems. There was no time limit. They were invited to think aloud and their spontaneous justifications were recorded and then transcribed.
The correct answer is obviously “ten,” but it must not be so obvious if it is given by only one third of the subjects (32%), while the remaining two thirds give the wrong answer “five,” which is so dominant.
If we consider the text of the problem from the point of view of the information explicitly transmitted ( said ), we have that it only theoretically provides the necessary information to reach the solution and precisely that: (a) the number of ties initially owned by P. and J. is equal, (b) P. gives J. five of his ties. However, the subjects are wrong. What emerges, however, from the spontaneous justifications given by the subjects who give the wrong answer is that they see only the increase of J. and not the consequent loss of P. by five ties. We report two typical justifications: “P. gives five of his to J., J. has five more ties than P., the five P. gave him” and also “They started from the same number of ties, so if P. gives J. five ties, J. should have five more than P.”
Slightly different from the previous ones is the following recurrent answer, in which the participants also consider the decrease of P. as well as the increase of J.: “I see five ties at stake, which are the ones that move,” or also “There are these five ties that go from one to the other, so one has five ties less and the other has five more,” reaching however the conclusion similar to the previous one that “J. has five ties more, because the other gave them to him.” 2
Almost always the participants who answer “five” use a numerical example to justify the answer given or to find a solution to the problem, after some unsuccessful attempts. It is paradoxical how many of these participants accept that the problem has two solutions, one “five ties” obtained by reasoning without considering a concrete number of initial ties, owned by P. and J., the other “ten ties” obtained by using a numerical example. So, for example, we read in the protocol of a participant who, after having answered “five more ties,” using a numerical example, finds “ten” of difference between the ties of P. and those of J.: “Well! I think the “five” is still more and more exact; for me this one has five more, period and that’s it.” “Making the concrete example: “ten” – he chases another subject on an abstract level. I would be more inclined to another formula, to five.”
About half of the subjects who give the answer “five,” in fact, at first refuse to answer because “we don’t know the initial number and therefore we can’t know how many ties J. has more than P.,” or at the most they answer: “J. has five ties more, P. five less, more we can’t know, because a data is missing.”
Even before this difficulty, so to speak, operational, the text of the problem is difficult because in it the quantity relative to the decrease of P. remains implicit (−5). The resulting misunderstanding is that if the quantity transferred is five ties, the resulting difference is only five ties: if the ties that P. gives to J. are five, how can J. have 10 ties more than P.?
So the difficulty of the problem lies in the discrepancy between the quantity transferred and the bidirectional effect that this quantity determines with its displacement. Resolving implies a restructuring of the sentence: “Peter gives John five of his ties (and therefore he loses five).” And this is precisely the reasoning carried out by those subjects who give the right answer “ten.”
We have therefore formulated a new version in which a pair of verbs should make explicit the loss of P.:
Peter loses five of his ties and John takes them.
However, the results obtained with this version, submitted to 20 other subjects, substantially confirm the results obtained with the original version: the correct answers are 17% (3/20) and the wrong ones 75% (15/20). From a Chi-square test (χ 2 = 2,088 p = 0.148) it results no significant difference between the two versions.
If we go to read the spontaneous justifications, we find that the subjects who give the answer “five” motivate it in a similar way to the subjects of the original version. So, for example: “P. loses five, J. gets them, so J. has five ties more than P.”
The decrease of P. is still not perceived, and the discrepancy between the lost amount of ties and the double effect that this quantity determines with its displacement persists.
Therefore, a new version has been realized in which the amount of ties lost by P. has nothing to do with J’s acquisition of five ties, the two amounts of ties are different and then they are perceived as decoupled, so as to neutralize the perceptual-conceptual factor underlying it.
Peter loses five of his ties and John buys five new ones.
It was submitted to 23 participants. Of them, 17 (74%) gave the answer “ten” and only 3 (13%) the answer “five.” There was a significant difference (χ 2 = 16,104 p = 0.000) between the results obtained using the present experimental version and the results from the control version. The participants who give the correct solution “ten” mostly motivate their answer as follows: “P. loses five and therefore J. has also those five that P. lost; he buys another five, there are ten,” declaring that he “added to the five that P. had lost the five that J. had bought.” The effectiveness of the experimental manipulation adopted is confirmed. 3
The satisfactory results obtained with this version cannot be attributed to the use of two different verbs, which proved to be ineffective (see version 2), but to the splitting, and consequent differentiation (J. has in addition five new ties), of the two quantities.
This time, the increase of J. and the decrease of P. are grasped as simultaneous and distinct and their combined effect is not identified with one or the other, but is equal to the sum of +5 and −5 in absolute terms.
The hypothesis regarding the effect of reformulation has also been confirmed in classical insight problems such as the Square and the Parallelogram ( Wertheimer, 1925 ), the Pigs in a Pen ( Schooler et al., 1993 ), the Bat & Ball ( Frederick, 2005 ) in recent studies ( Macchi and Bagassi, 2012 , 2015 ) which showed a dramatic increase in the number of solutions.
In their original version these problems are true brain teasers, and the majority of participants in these studies needed them to be reformulated in order to reach the solution. In Appendix B we present in detail the results obtained (see Table 1 ). Below we report, for each problem, the text of the original version in comparison with the reformulated experimental version.
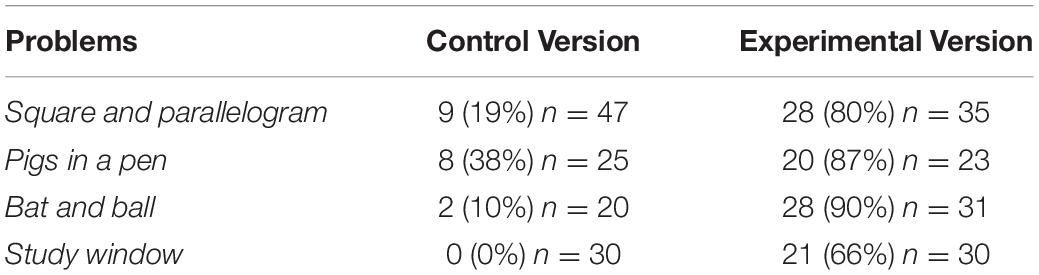
Table 1. Percentages of correct solutions with reformulated experimental versions.
Square and Parallelogram Problem ( Wertheimer, 1925 )
Given that AB = a and AG = b, find the sum of the areas of square ABCD and parallelogram EBGD ( Figures 3 , 4 ).
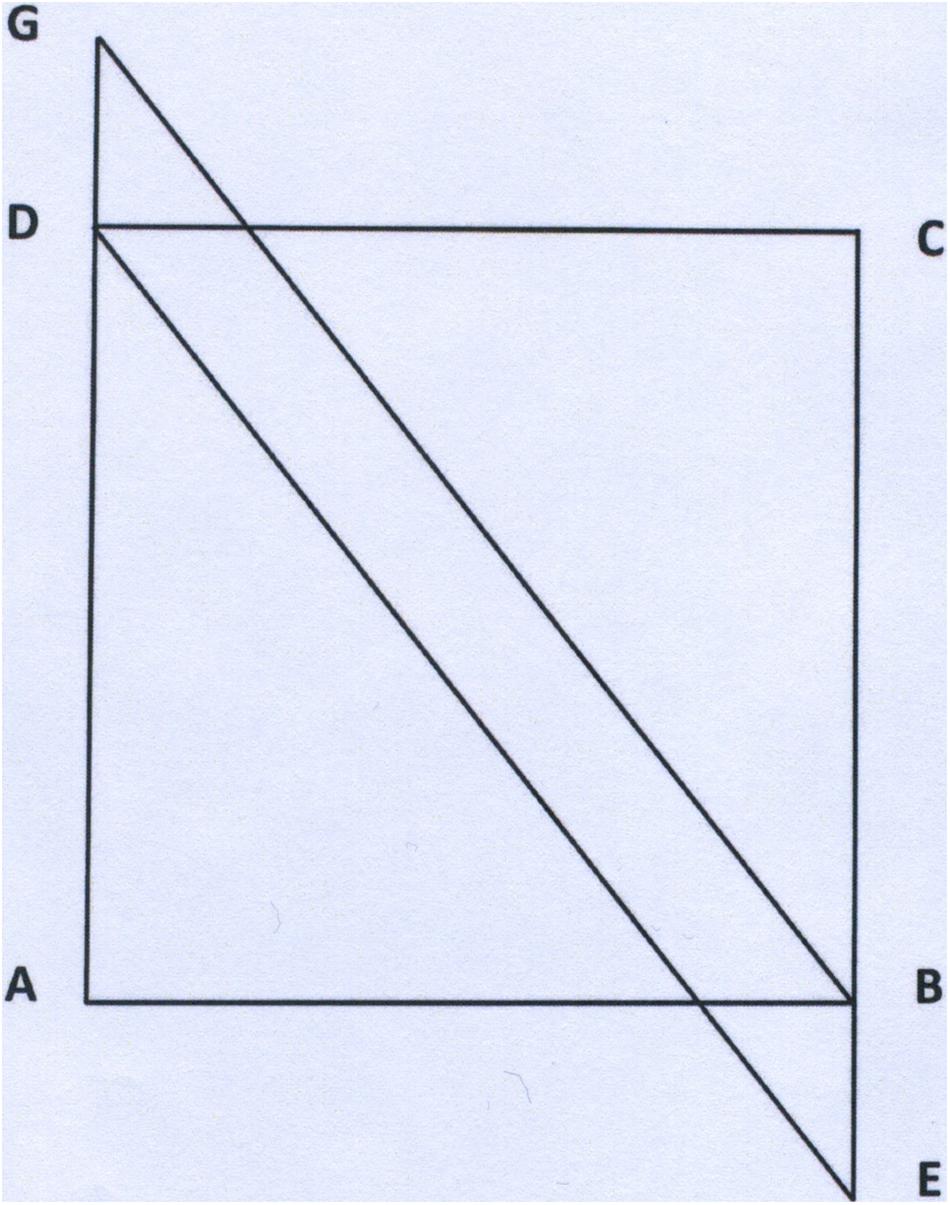
Figure 3. The square and parallelogram problem.
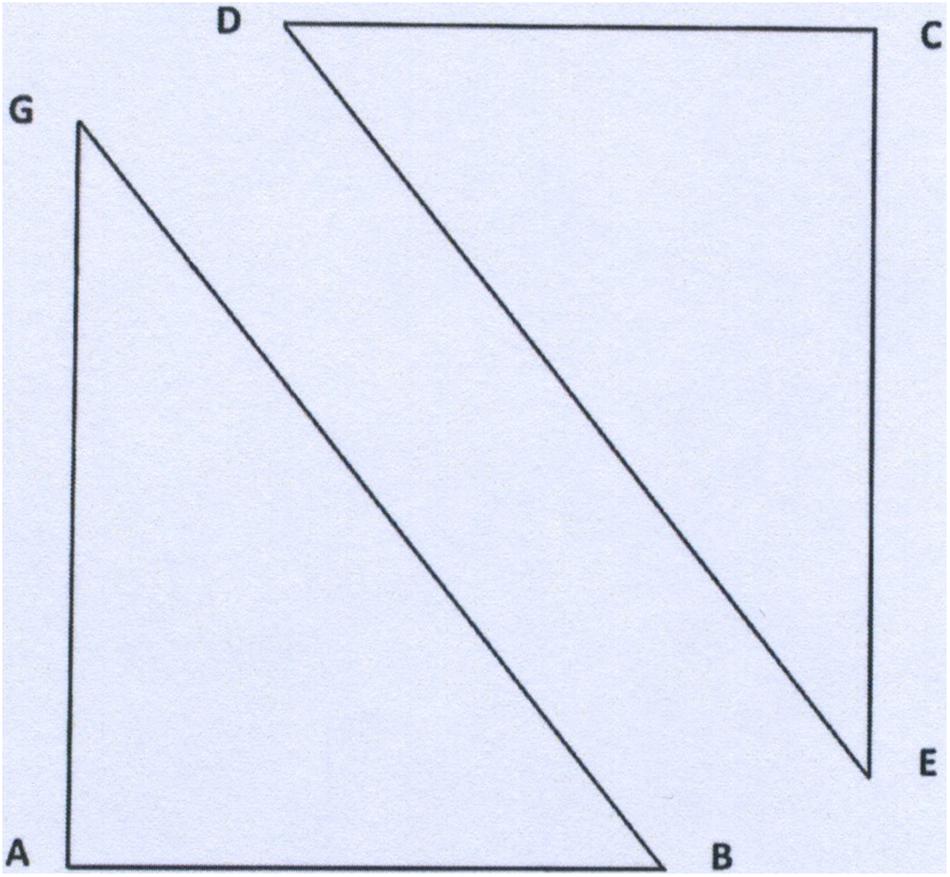
Figure 4. Solution.
Experimental Version
Given that AB = a and AG = b , find the sum of the areas of the two partially overlapping figures .
Pigs in a Pen Problem ( Schooler et al., 1993 )
Nine pigs are kept in a square pen . Build two more square enclosures that would put each pig in a pen by itself ( Figures 5 , 6 ).
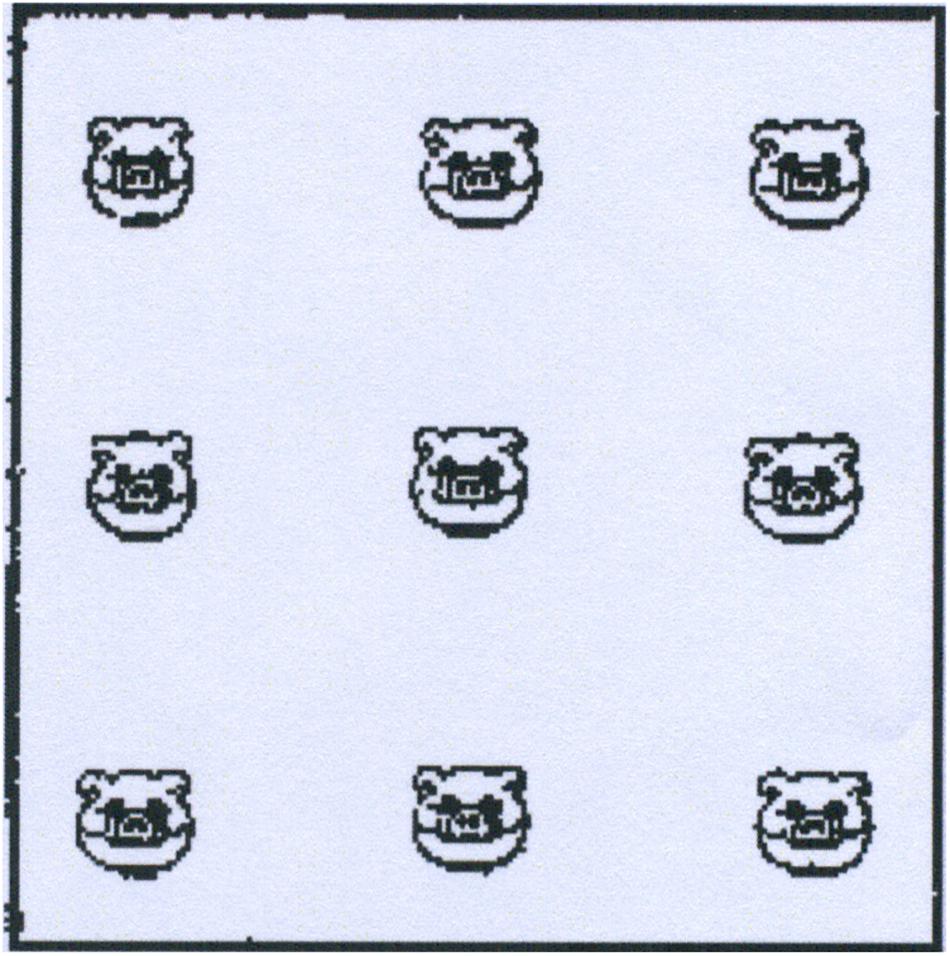
Figure 5. The pigs in a pen problem.
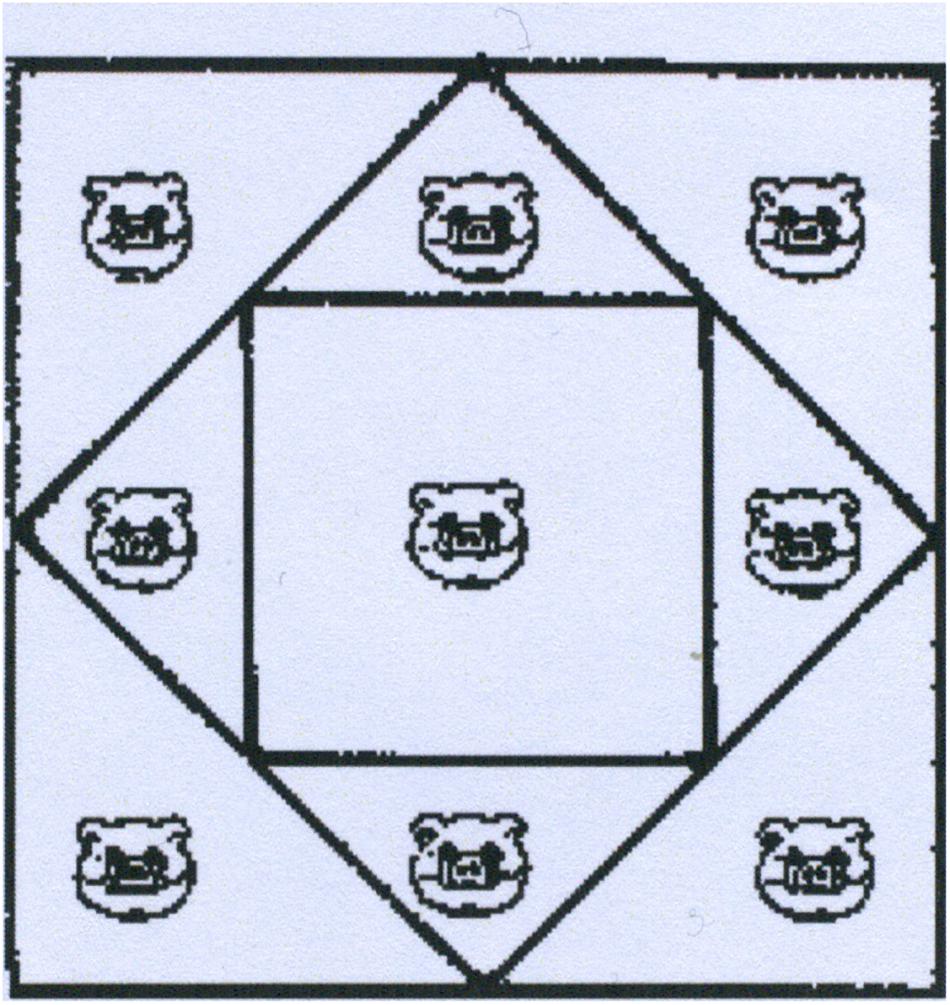
Figure 6. Solution.
Nine pigs are kept in a square pen. Build two more squares that would put each pig in a by itself .
Bat and Ball Problem ( Frederick, 2005 )
A bat and a ball cost $1.10 in total. The bat costs $ 1.00 more than the ball. How much does the ball cost? ___cents.
A bat and a ball cost $1.10 in total. The bat costs $ 1.00 more than the ball. Find the cost of the bat and of the ball .
Once the problem knots that alter the aim of the task have been identified, the reformulation technique can be a valid didactic tool, as it allows to reveal the misunderstanding and to eliminate it without changing the mathematical nature of the problem. The training to creativity would consist in this sense in training to have interpretative keys different from the usual, when the difficulty cannot be addressed through computational techniques.
Closing Thoughts
By identifying the misunderstanding in problem solving, the reformulation technique has made it possible to eliminate the problem knots, without changing the mathematical nature of the problem. With the experimental reformulated versions of paradigmatic problems, both apparent trivial tasks or brain teasers have obtained a significant increase in correct answers.
Studying how an insight problem is formed, and not just how it is solved, may well become an important topic in education. We focus on undergraduate students’ strategies and their errors while solving problems, and the specific cognitive processes involved in misunderstanding, which are crucial to better exploit what could be beneficial to reach the solution and to teach how to improve the ability to solve problems.
Without violating the need for the necessary rigor of a demonstration, for example, it is possible to organize the problem-demonstration discourse according to a different criterion, precisely by favoring the psychological needs of the subject to whom the explanation discourse is addressed, taking care to organize the explanation with regard to the way his mind works, to what can favor its comprehension and facilitate its memory.
On the other hand, one of the criteria traditionally followed by mathematicians in constructing, for example, demonstrations, or at least in explaining them, is to never make any statement that is not supported by the elements provided above. In essence, in the course of the demonstration nothing is anticipated, and indeed it happens frequently that the propositions directly relevant and relevant to the development of the reasoning (for example, the steps of a geometric demonstration) are preceded by digressions intended to introduce and deal with the elements that legitimize them. As a consequence of such an expositive formalism, the recipient of the speech (the student) often finds himself in the situation of being led to the final conclusion a bit like a blind man who, even though he knows the goal, does not see the way, but can only control step by step the road he is walking along and with difficulty becomes aware of the itinerary.
The text of every problem, if formulated in natural language, has a psychorhetoric dimension, in the sense that in every speech, that is in the production and reception of every speech, there are aspects related to the way the mind works – and therefore psychological and rhetorical – that are decisive for comprehensibility, expressive adequacy and communicative effectiveness. It is precisely to these aspects that we refer to when we talk about the psychorhetoric dimension. Rhetoric, from the point of view of the broadcaster, has studied discourse in relation to the recipient, and therefore to its acceptability, comprehensibility and effectiveness, so that we can say that rhetoric has studied discourse “psychologically.”
Adopting this perspective, the commonplace that the rhetorical dimension only concerns the common discourse, i.e., the discourse that concerns debatable issues, and not the scientific discourse (logical-mathematical-demonstrative), which would be exempt from it, is falling away. The matter dealt with, the truth of what is actually said, is not sufficient to guarantee comprehension.
Data Availability Statement
The datasets generated for this study are available on request to the corresponding author.
Ethics Statement
Ethical review and approval was not required for the study on human participants in accordance with the local legislation and institutional requirements. Written informed consent for participation was not required for this study in accordance with the national legislation and the institutional requirements.
Author Contributions
LM and MB devised the project, developed the theory, carried out the experiment and wrote the manuscript. Both authors contributed to the article and approved the submitted version.
Conflict of Interest
The authors declare that the research was conducted in the absence of any commercial or financial relationships that could be construed as a potential conflict of interest.
- ^ The theoretical framework assumed here is Paul Grice’s theory of communication (1975) based on the existence in communication of the explicit layer ( said ) and of the implicit ( implied ), so that the recognition of the communicative intention of the speaker by the interlocutor is crucial for comprehension.
- ^ A participant who after having given the solution “five” corrects himself in “ten” explains the first answer as follows: “it is more immediate, in my opinion, to see the real five ties that are moved, because they are five things that are moved; then as a more immediate answer is ‘five,’ because it is something more real, less mathematical.”
- ^ The factor indicated is certainly the main responsible for the answer “five,” but not the only one (see the Appendix for a pragmatic analysis of the text).
- ^ Versions and results of the problems exposed are already published in Macchi e Bagassi 2012, 2014, 2015.
Bagassi, M., and Macchi, L. (2006). Pragmatic approach to decision making under uncertainty: the case of the disjunction effect. Think. Reason. 12, 329–350. doi: 10.1080/13546780500375663
CrossRef Full Text | Google Scholar
Bagassi, M., and Macchi, L. (2016). “The interpretative function and the emergence of unconscious analytic thought,” in Cognitive Unconscious and Human Rationality , eds L. Macchi, M. Bagassi, and R. Viale (Cambridge, MA: MIT Press), 43–76.
Google Scholar
Bulbrook, M. E. (1932). An experimental inquiry into the existence and nature of “insight”. Am. J. Psycho. 44, 409–453. doi: 10.2307/1415348
Duncker, K. (1945). “On problem solving,” in Psychological Monographs , Vol. 58, (Berlin: Springer), I–IX;1–113. Original in German, Psychologie des produktiven Denkens. doi: 10.1037/h0093599
Frederick, S. (2005). Cognitive reflection and decision making. J. Econ. Perspect. 19, 25–42. doi: 10.1257/089533005775196732
Kahneman, D. (2003). A perspective on judgment and choice: mapping bounded rationality. Am. Psychol. 58, 697–720. doi: 10.1037/0003-066x.58.9.697
PubMed Abstract | CrossRef Full Text | Google Scholar
Kaplan, C. A., and Simon, H. A. (1990). In search of insight. Cogn. Psychol. 22, 374–419. doi: 10.1016/0010-0285(90)90008-R
Levinson, S. C. (1995). “Interactional biases in human thinking,” in Social Intelligence and Interaction , ed. E. N. Goody (Cambridge: Cambridge University Press), 221–261. doi: 10.1017/cbo9780511621710.014
Levinson, S. C. (2013). “Cross-cultural universals and communication structures,” in Language, Music, and the Brain: A Mysterious Relationship , ed. M. A. Arbib (Cambridge, MA: MIT Press), 67–80. doi: 10.7551/mitpress/9780262018104.003.0003
Macchi, L. (1995). Pragmatic aspects of the base-rate fallacy. Q. J. Exp. Psychol. 4, 188–207. doi: 10.1080/14640749508401384
Macchi, L. (2000). Partitive formulation of information in probabilistic reasoning: beyond heuristics and frequency format explanations. Organ. Behav. Hum. 82, 217–236. doi: 10.1006/obhd.2000.2895
Macchi, L., and Bagassi, M. (2012). Intuitive and analytical processes in insight problem solving: a psycho-rhetorical approach to the study of reasoning. Mind Soc. 11, 53–67. doi: 10.1007/s11299-012-0103-3
Macchi, L., and Bagassi, M. (2014). The interpretative heuristic in insight problem solving. Mind Soc. 13, 97–108. doi: 10.1007/s11299-014-0139-7
Macchi, L., and Bagassi, M. (2015). When analytic thought is challenged by a misunderstanding. Think. Reas. 21, 147–164. doi: 10.4324/9781315144061-9
Macchi, L., and Bagassi, M. (2019). The argumentative and the interpretative functions of thought: two adaptive characteristics of the human cognitive system. Teorema 38, 87–96.
Macchi, L., and Bagassi, M. (2020). “Bounded rationality and problem solving: the interpretative function of thought,” in Handbook of Bounded Rationality , ed. Riccardo Viale (London: Routledge).
Mach, E. (1914). The Analysis of Sensations. Chicago IL: Open Court.
Mercier, H., and Sperber, D. (2011). Why do human reason? Arguments for an argumentative theory. Behav. Brain Sci. 34, 57–74. doi: 10.1017/s0140525x10000968
Mosconi, G. (1990). Discorso e Pensiero. Bologna: Il Mulino.
Mosconi, G. (2016). “Closing thoughts,” in Cognive Unconscious and Human rationality , eds L. Macchi, M. Bagassi, and R. Viale (Cambridge, MA: MIT Press), 355–363. Original in Italian Discorso e pensiero.
Mosconi, G., and D’Urso, V. (1974). Il Farsi e il Disfarsi del Problema. Firenze: Giunti-Barbera.
Mosconi, G., and Macchi, L. (2001). The role of pragmatic rules in the conjunction fallacy. Mind Soc. 3, 31–57. doi: 10.1007/bf02512074
Newell, A., Shaw, J. C., and Simon, H. A. (1958). Elements of a theory of human problem solving. Psychol. Rev. 65, 151–166. doi: 10.1037/h0048495
Politzer, G., and Macchi, L. (2000). Reasoning and pragmatics. Mind Soc. 1, 73–93. doi: 10.1007/BF02512230
Schooler, J. W., Ohlsson, S., and Brooks, K. (1993). Thoughts beyond words: when language overshadows insight. J. Exp. Psychol. 122, 166–183. doi: 10.1037/0096-3445.122.2.166
Simon, H. A. (1979). Information processing models of cognition. Annu. Rev. Psychol. 30, 363–396. doi: 10.1146/annurev.ps.30.020179.002051
Sperber, D., and Wilson, D. (1986/1995). Relevance: Communication and cognition. Oxford: Blackwell.
Tomasello, M. (2009). Why We Cooperate. Cambridge, Mass: MIT Press. doi: 10.7551/mitpress/8470.001.0001
Wertheimer, M. (1925). Drei Abhandlungen zur Gestalttheorie. Erlangen: Verlag der Philosophischen Akademie.
Wertheimer, M. (1959). Productive Thinking. New York, NY: Harper & Row.
Wertheimer, M. (1985). A gestalt perspective on computer simulations of cognitive processes. Comput. Hum. Behav. 1, 19–33. doi: 10.1016/0747-5632(85)90004-4
Pragmatic analysis of the problematic loci of the Ties problem, which emerged from the spontaneous verbalizations of the participants:
- “the same number of ties”
This expression is understood as a neutral information, a kind of base or sliding plane on which the transfer of the five ties takes place and, in fact, these subjects motivate their answer “five” with: “there is this transfer of five ties from P. to J. ….”
- “5 more, 5 less”
We frequently resort to similar expressions in situations where, if I have five units more than another, the other has five less than me and the difference between us is five.
Consider, for example, the case of the years: say that J. is five years older than P. means to say that P. is five years younger than J. and that the difference in years between the two is five, not ten.
In comparisons, we evaluate the difference with something used as a term of reference, for example the age of P., which serves as a basis, the benchmark, precisely.
- “he gives”
The verb “to give” conveys the concept of the growth of the recipient, not the decrease of the giver, therefore, contributes to the crystallization of the “same number,” preventing to grasp the decrease of P.
Appendix B 4
Given that AB = a and AG = b, find the sum of the areas of square ABCD and parallelogram EBGD .
Typically, problem solvers find the problem difficult and fail to see that a is also the altitude of parallelogram EBGD. They tend to calculate its area with onerous and futile methods, while the solution can be reached with a smart method, consisting of restructuring the entire given shape into two partially overlapping triangles ABG and ECD. The sum of their areas is 2 x a b /2 = a b . Moreover, by shifting one of the triangles so that DE coincides with GB, the answer is “ a b ,” which is the area of the resultant rectangle. Referring to a square and a parallelogram fixes a favored interpretation of the perceptive stimuli, according to those principles of perceptive organization thoroughly studied by the Gestalt Theory. It firmly sets the calculation of the area on the sum of the two specific shapes dealt with in the text, while, the problem actually requires calculation of the area of the shape, however organized, as the sum of two triangles rectangles, or the area of only one rectangle, as well as the sum of square and parallelogram. Hence, the process of restructuring is quite difficult.
To test our hypotheses we formulated an experimental version:
In this formulation of the problem, the text does not impose constraints on the interpretation/organization of the figure, and the spontaneous, default interpretation is no longer fixed. Instead of asking for “the areas of square and parallelogram,” the problem asks for the areas of “the two partially overlapping figures.” We predicted that the experimental version would allow the subjects to see and consider the two triangles also.
Actually, we found that 80% of the participants (28 out of 35) gave a correct answer, and most of them (21 out of 28) gave the smart “two triangles” solution. In the control version, on the other hand, only 19% (9 out of 47) gave the correct response, and of these only two gave the “two triangles” solution.
The findings were replicated in the “Pigs in a pen” problem:
Nine pigs are kept in a square pen . Build two more square enclosures that would put each pig in a pen by itself.
The difficulty of this problem lies in the interpretation of the request, nine pigs each individually enclosed in a square pen, having only two more square enclosures. This interpretation is supported by the favored, orthogonal reference scheme, with which we represent the square. This privileged organization, according to our hypothesis, is fixed by the text which transmits the implicature that the pens in which the piglets are individually isolated must be square in shape too. The function of enclosure wrongfully implies the concept of a square. The task, on the contrary, only requires to pen each pig.
Once again, we created an experimental version by reformulating the problem, eliminating the word “enclosure” and the phrase “in a pen.” The implicit inference that the pen is necessarily square is not drawn.
The experimental version yielded 87% correct answers (20 out of 23), while the control version yielded only 38% correct answers (8 out of 25).
The formulation of the experimental versions was more relevant to the aim of the task, and allowed the perceptual stimuli to be interpreted in accordance with the solution.
The relevance of text and the re-interpretation of perceptual stimuli, goal oriented to the aim of the task, were worked out in unison in an interrelated interpretative “game.”
We further investigated the interpretative activity of thinking, by studying the “Bat and ball” problem, which is part of the CRT. Correct performance is usually considered to be evidence of reflective cognitive ability (correlated with high IQ scores), versus intuitive, erroneous answers to the problem ( Frederick, 2005 ).
Bat and Ball problem
A bat and a ball cost $1.10 in total. The bat costs $ 1.00 more than the ball. How much does the ball cost?___cents
Of course the answer which immediately comes to mind is 10 cents, which is incorrect as, in this case, the difference between $ 1.00 and 10 cents is only 90 cents, not $1.00 as the problem stipulates. The correct response is 5 cents.
Number physiognomics and the plausibility of the cost are traditionally considered responsible for this kind of error ( Frederick, 2005 ; Kahneman, 2003 ).
These factors aside, we argue that if the rhetoric structure of the text is analyzed, the question as formulated concerns only the ball, implying that the cost of the bat is already known. The question gives the key to the interpretation of what has been said in each problem and, generally speaking, in every discourse. Given data, therefore, is interpreted in the light of the question. Hence, “The bat costs $ 1.00 more than” becomes “The bat costs $ 1.00,” by leaving out “more than.”
According to our hypothesis, independently of the different cognitive styles, erroneous responses could be the effect of the rhetorical structure of the text, where the question is not adequate to the aim of the task. Consequently, we predicted that if the question were to be reformulated to become more relevant, the subjects would find it easier to grasp the correct response. In the light of our perspective, the cognitive abilities involved in the correct response were also reinterpreted. Consequently, we reformulated the text as follows in order to eliminate this misleading inference:
This time we predicted an increase in the number of correct answers. The difference in the percentages of correct solutions was significant: in the experimental version 90% of the participants gave a correct answer (28 out of 31), and only 10% (2 out of 20) answered correctly in the control condition.
The simple reformulation of the question, which expresses the real aim of the task (to find the cost of both items), does not favor the “short circuit” of considering the cost of the bat as already known (“$1,” by leaving out part of the phrase “more than”).
It still remains to be verified if those subjects who gave the correct response in the control version have a higher level of cognitive reflexive ability compared to the “intuitive” respondents. This has been the general interpretation given in the literature to the difference in performance.
We think it is a matter of a particular kind of reflexive ability, due to which the task is interpreted in the light of the context and not abstracting from it. The difficulty which the problem implicates does not so much involve a high level of abstract reasoning ability as high levels of pragmatic competence, which disambiguates the text. So much so that, intervening only on the pragmatic level, keeping numbers physiognomics and maintaining the plausible costs identical, the problem becomes a trivial arithmetical task.
Keywords : creative problem solving, insight, misunderstanding, pragmatics, language and thought
Citation: Bagassi M and Macchi L (2020) Creative Problem Solving as Overcoming a Misunderstanding. Front. Educ. 5:538202. doi: 10.3389/feduc.2020.538202
Received: 26 February 2020; Accepted: 29 October 2020; Published: 03 December 2020.
Reviewed by:
Copyright © 2020 Bagassi and Macchi. This is an open-access article distributed under the terms of the Creative Commons Attribution License (CC BY) . The use, distribution or reproduction in other forums is permitted, provided the original author(s) and the copyright owner(s) are credited and that the original publication in this journal is cited, in accordance with accepted academic practice. No use, distribution or reproduction is permitted which does not comply with these terms.
*Correspondence: Laura Macchi, [email protected]
This article is part of the Research Topic
Psychology and Mathematics Education

An official website of the United States government
The .gov means it’s official. Federal government websites often end in .gov or .mil. Before sharing sensitive information, make sure you’re on a federal government site.
The site is secure. The https:// ensures that you are connecting to the official website and that any information you provide is encrypted and transmitted securely.
- Publications
- Account settings
Preview improvements coming to the PMC website in October 2024. Learn More or Try it out now .
- Advanced Search
- Journal List

Analysing Complex Problem-Solving Strategies from a Cognitive Perspective: The Role of Thinking Skills
1 MTA-SZTE Digital Learning Technologies Research Group, Center for Learning and Instruction, University of Szeged, 6722 Szeged, Hungary
Gyöngyvér Molnár
2 MTA-SZTE Digital Learning Technologies Research Group, Institute of Education, University of Szeged, 6722 Szeged, Hungary; uh.degezs-u.yspde@ranlomyg
Associated Data
The data used to support the findings cannot be shared at this time as it also forms part of an ongoing study.
Complex problem solving (CPS) is considered to be one of the most important skills for successful learning. In an effort to explore the nature of CPS, this study aims to investigate the role of inductive reasoning (IR) and combinatorial reasoning (CR) in the problem-solving process of students using statistically distinguishable exploration strategies in the CPS environment. The sample was drawn from a group of university students (N = 1343). The tests were delivered via the eDia online assessment platform. Latent class analyses were employed to seek students whose problem-solving strategies showed similar patterns. Four qualitatively different class profiles were identified: (1) 84.3% of the students were proficient strategy users, (2) 6.2% were rapid learners, (3) 3.1% were non-persistent explorers, and (4) 6.5% were non-performing explorers. Better exploration strategy users showed greater development in thinking skills, and the roles of IR and CR in the CPS process were varied for each type of strategy user. To sum up, the analysis identified students’ problem-solving behaviours in respect of exploration strategy in the CPS environment and detected a number of remarkable differences in terms of the use of thinking skills between students with different exploration strategies.
1. Introduction
Problem solving is part and parcel of our daily activities, for instance, in determining what to wear in the morning, how to use our new electronic devices, how to reach a restaurant by public transport, how to arrange our schedule to achieve the greatest work efficiency and how to communicate with people in a foreign country. In most cases, it is essential to solve the problems that recur in our study, work and daily lives. These situations require problem solving. Generally, problem solving is the thinking that occurs if we want “to overcome barriers between a given state and a desired goal state by means of behavioural and/or cognitive, multistep activities” ( Frensch and Funke 1995, p. 18 ). It has also been considered as one of the most important skills for successful learning in the 21st century. This study focuses on one specific kind of problem solving, complex problem solving (CPS). (Numerous other terms are also used ( Funke et al. 2018 ), such as interactive problem solving ( Greiff et al. 2013 ; Wu and Molnár 2018 ), and creative problem solving ( OECD 2010 ), etc.).
CPS is a transversal skill ( Greiff et al. 2014 ), operating several mental activities and thinking skills (see Molnár et al. 2013 ). In order to explore the nature of CPS, some studies have focused on detecting its component skills ( Wu and Molnár 2018 ), whereas others have analysed students’ behaviour during the problem-solving process ( Greiff et al. 2018 ; Wu and Molnár 2021 ). This study aims to link these two fields by investigating the role of thinking skills in learning by examining students’ use of statistically distinguishable exploration strategies in the CPS environment.
1.1. Complex Problem Solving: Definition, Assessment and Relations to Intelligence
According to a widely accepted definition proposed by Buchner ( 1995 ), CPS is “the successful interaction with task environments that are dynamic (i.e., change as a function of users’ intervention and/or as a function of time) and in which some, if not all, of the environment’s regularities can only be revealed by successful exploration and integration of the information gained in that process” ( Buchner 1995, p. 14 ). A CPS process is split into two phases, knowledge acquisition and knowledge application. In the knowledge acquisition (KAC) phase of CPS, the problem solver understands the problem itself and stores the acquired information ( Funke 2001 ; Novick and Bassok 2005 ). In the knowledge application (KAP) phase, the problem solver applies the acquired knowledge to bring about the transition from a given state to a goal state ( Novick and Bassok 2005 ).
Problem solving, especially CPS, has frequently been compared or linked to intelligence in previous studies (e.g., Beckmann and Guthke 1995 ; Stadler et al. 2015 ; Wenke et al. 2005 ). Lotz et al. ( 2017 ) observed that “intelligence and [CPS] are two strongly overlapping constructs” (p. 98). There are many similarities and commonalities that can be detected between CPS and intelligence. For instance, CPS and intelligence share some of the same key features, such as the integration of information ( Stadler et al. 2015 ). Furthermore, Wenke et al. ( 2005 ) stated that “the ability to solve problems has featured prominently in virtually every definition of human intelligence” (p. 9); meanwhile, from the opposite perspective, intelligence has also been considered as one of the most important predictors of the ability to solve problems ( Wenke et al. 2005 ). Moreover, the relation between CPS and intelligence has also been discussed from an empirical perspective. A meta-analysis conducted by Stadler et al. ( 2015 ) selected 47 empirical studies (total sample size N = 13,740) which focused on the correlation between CPS and intelligence. The results of their analysis confirmed that a correlation between CPS and intelligence exists with a moderate effect size of M(g) = 0.43.
Due to the strong link between CPS and intelligence, assessments of these two domains have been connected and have overlapped to a certain extent. For instance, Beckmann and Guthke ( 1995 ) observed that some of the intelligence tests “capture something akin to an individual’s general ability to solve problems (e.g., Sternberg 1982 )” (p. 184). Nowadays, some widely used CPS assessment methods are related to intelligence but still constitute a distinct construct ( Schweizer et al. 2013 ), such as the MicroDYN approach ( Greiff and Funke 2009 ; Greiff et al. 2012 ; Schweizer et al. 2013 ). This approach uses the minimal complex system to simulate simplistic, artificial but still complex problems following certain construction rules ( Greiff and Funke 2009 ; Greiff et al. 2012 ).
The MicroDYN approach has been widely employed to measure problem solving in a well-defined problem context (i.e., “problems have a clear set of means for reaching a precisely described goal state”, Dörner and Funke 2017, p. 1 ). To complete a task based on the MicroDYN approach, the problem solver engages in dynamic interaction with the task to acquire relevant knowledge. It is not possible to create this kind of test environment with the traditional paper-and-pencil-based method. Therefore, it is currently only possible to conduct a MicroDYN-based CPS assessment within the computer-based assessment framework. In the context of computer-based assessment, the problem-solvers’ operations were recorded and logged by the assessment platform. Thus, except for regular achievement-focused result data, logfile data are also available for analysis. This provides the option of exploring and monitoring problem solvers’ behaviour and thinking processes, specifically, their exploration strategies, during the problem-solving process (see, e.g., Chen et al. 2019 ; Greiff et al. 2015a ; Molnár and Csapó 2018 ; Molnár et al. 2022 ; Wu and Molnár 2021 ).
Problem solving, in the context of an ill-defined problem (i.e., “problems have no clear problem definition, their goal state is not defined clearly, and the means of moving towards the (diffusely described) goal state are not clear”, Dörner and Funke 2017, p. 1), involved a different cognitive process than that in the context of a well-defined problem ( Funke 2010 ; Schraw et al. 1995 ), and it cannot be measured with the MicroDYN approach. The nature of ill-defined problem solving has been explored and discussed in numerous studies (e.g., Dörner and Funke 2017 ; Hołda et al. 2020 ; Schraw et al. 1995 ; Welter et al. 2017 ). This will not be discussed here as this study focuses on well-defined problem solving.
1.2. Inductive and Combinatorial Reasoning as Component Skills of Complex Problem Solving
Frensch and Funke ( 1995 ) constructed a theoretical framework that summarizes the basic components of CPS and the interrelations among the components. The framework contains three separate components: problem solver, task and environment. The impact of the problem solver is mainly relevant to three main categories, which are memory contents, dynamic information processing and non-cognitive variables. Some thinking skills have been reported to play an important role in dynamic information processing. We can thus describe them as component skills of CPS. Inductive reasoning (IR) and combinatorial reasoning (CR) are the two thinking skills that have been most frequently discussed as component skills of CPS.
IR is the reasoning skill that has been covered most commonly in the literature. Currently, there is no universally accepted definition. Molnár et al. ( 2013 ) described it as the cognitive process of acquiring general regularities by generalizing single and specific observations and experiences, whereas Klauer ( 1990 ) defined it as the discovery of regularities that relies upon the detection of similarities and/or dissimilarities as concerns attributes of or relations to or between objects. Sandberg and McCullough ( 2010 ) provided a general conclusion of the definitions of IR: it is the process of moving from the specific to the general.
Csapó ( 1997 ) pointed out that IR is a basic component of thinking and that it forms a central aspect of intellectual functioning. Some studies have also discussed the role of IR in a problem-solving environment. For instance, Mayer ( 1998 ) stated that IR will be applied in information processing during the process of solving general problems. Gilhooly ( 1982 ) also pointed out that IR plays a key role in some activities in the problem-solving process, such as hypothesis generation and hypothesis testing. Moreover, the influence of IR on both KAC and KAP has been analysed and demonstrated in previous studies ( Molnár et al. 2013 ).
Empirical studies have also provided evidence that IR and CPS are related. Based on the results of a large-scale assessment (N = 2769), Molnár et al. ( 2013 ) showed that IR significantly correlated with 9–17-year-old students’ domain-general problem-solving achievement (r = 0.44–0.52). Greiff et al. ( 2015b ) conducted a large-scale assessment project (N = 2021) in Finland to explore the links between fluid reasoning skills and domain-general CPS. The study measured fluid reasoning as a two-dimensional model which consisted of deductive reasoning and scientific reasoning and included inductive thinking processes ( Greiff et al. 2015b ). The results drawing on structural equation modelling indicated that fluid reasoning which was partly based on IR had significant and strong predictive effects on both KAC (β = 0.51) and KAP (β = 0.55), the two phases of problem solving. Such studies have suggested that IR is one of the component skills of CPS.
According to Adey and Csapó ’s ( 2012 ) definition, CR is the process of creating complex constructions out of a set of given elements that satisfy the conditions explicitly given in or inferred from the situation. In this process, some cognitive operations, such as combinations, arrangements, permutations, notations and formulae, will be employed ( English 2005 ). CR is one of the basic components of formal thinking ( Batanero et al. 1997 ). The relationship between CR and CPS has frequently been discussed. English ( 2005 ) demonstrated that CR has an essential meaning in several types of problem situations, such as problems requiring the systematic testing of alternative solutions. Moreover, Newell ( 1993 ) pointed out that CR is applied in some key activities of problem-solving information processing, such as strategy generation and application. Its functions include, but are not limited to, helping problem solvers to discover relationships between certain elements and concepts, promoting their fluency of thinking when they are considering different strategies ( Csapó 1999 ) and identifying all possible alternatives ( OECD 2014 ). Moreover, Wu and Molnár ’s ( 2018 ) empirical study drew on a sample (N = 187) of 11–13-year-old primary school students in China. Their study built a structural equation model between CPS, IR and CR, and the result indicated that CR showed a strong and statistically significant predictive power for CPS (β = 0.55). Thus, the results of the empirical study also support the argument that CR is one of the component skills of CPS.
1.3. Behaviours and Strategies in a Complex Problem-Solving Environment
Wüstenberg et al. ( 2012 ) stated that the creation and implementation of strategic exploration are core actions of the problem-solving task. Exploring and generating effective information are key to successfully solving a problem. Wittmann and Hattrup ( 2004 ) illustrated that “riskier strategies [create] a learning environment with greater opportunities to discover and master the rules and boundaries [of a problem]” (p. 406). Thus, when gathering information about a complex problem, there may be differences between exploration strategies in terms of efficacy. The MicroDYN scenarios, a simplification and simulation of the real-world problem-solving context, will also be influenced by the adoption and implementation of exploration strategies.
The effectiveness of the isolated variation strategy (or “Vary-One-Thing-At-A-Time” strategy—VOTAT; Vollmeyer et al. 1996 ) in a CPS environment has been hotly debated ( Chen et al. 2019 ; Greiff et al. 2018 ; Molnár and Csapó 2018 ; Molnár et al. 2022 ; Wu and Molnár 2021 ; Wüstenberg et al. 2014 ). To use the VOTAT strategy, a problem solver “systematically varies only one input variable, whereas the others remain unchanged. This way, the effect of the variable that has just been changed can be observed directly by monitoring the changes in the output variables” ( Molnár and Csapó 2018, p. 2 ). Understanding and using VOTAT effectively is the foundation for developing more complex strategies for coordinating multiple variables and the basis for some phases of scientific thinking (i.e., inquiry, analysis, inference and argument; Kuhn 2010 ; Kuhn et al. 1995 ).
Some previous studies have indicated that students who are able to apply VOTAT are more likely to achieve higher performance in a CPS assessment ( Greiff et al. 2018 ), especially if the problem is a well-defined minimal complex system (such as MicroDYN) ( Fischer et al. 2012 ; Molnár and Csapó 2018 ; Wu and Molnár 2021 ). For instance, Molnár and Csapó ( 2018 ) conducted an empirical study to explore how students’ exploration strategies influence their performance in an interactive problem-solving environment. They measured a group (N = 4371) of 3rd- to 12th-grade (aged 9–18) Hungarian students’ problem-solving achievement and modelled students’ exploration strategies. This result confirmed that students’ exploration strategies influence their problem-solving performance. For example, conscious VOTAT strategy users proved to be the best problem-solvers. Furthermore, other empirical studies (e.g., Molnár et al. 2022 ; Wu and Molnár 2021 ) achieved similar results, thus confirming the importance of VOTAT in a MicroDYN-based CPS environment.
Lotz et al. ( 2017 ) illustrated that effective use of VOTAT is associated with higher levels of intelligence. Their study also pointed out that intelligence has the potential to facilitate successful exploration behaviour. Reasoning skills are an important component of general intelligence. Based on Lotz et al. ’s ( 2017 ) statements, the roles IR and CR play in the CPS process might vary due to students’ different strategy usage patterns. However, there is still a lack of empirical studies in this regard.
2. Research Aims and Questions
Numerous studies have explored the nature of CPS, some of them discussing and analysing it from behavioural or cognitive perspectives. However, there have barely been any that have merged these two perspectives. From the cognitive perspective, this study explores the role of thinking skills (including IR and CR) in the cognition process of CPS. From the behavioural perspective, the study focuses on students’ behaviour (i.e., their exploration strategy) in the CPS assessment process. More specifically, the research aims to fill this gap and examine students’ use of statistically distinguishable exploration strategies in CPS environments and to detect the connection between the level of students’ thinking skills and their behaviour strategies in the CPS environment. The following research questions were thus formed.
- (RQ1) What exploration strategy profiles characterise the various problem-solvers at the university level?
- (RQ2) Can developmental differences in CPS, IR and CR be detected among students with different exploration strategy profiles?
- (RQ3) What are the similarities and differences in the roles IR and CR play in the CPS process as well as in the two phases of CPS (i.e., KAC and KAP) among students with different exploration strategy profiles?
3.1. Participants and Procedure
The sample was drawn from one of the largest universities in Hungary. Participation was voluntary, but students were able to earn one course credit for taking part in the assessment. The participants were students who had just started their studies there (N = 1671). 43.4% of the first-year students took part in the assessment. 50.9% of the participants were female, and 49.1% were male. We filtered the sample and excluded those who had more than 80% missing data on any of the tests. After the data were cleaned, data from 1343 students were available for analysis. The test was designed and delivered via the eDia online assessment system ( Csapó and Molnár 2019 ). The assessment was held in the university ICT room and divided into two sessions. The first session involved the CPS test, whereas the second session entailed the IR and CR tests. Each session lasted 45 min. The language of the tests was Hungarian, the mother tongue of the students.
3.2. Instruments
3.2.1. complex problem solving (cps).
The CPS assessment instrument adopted the MicroDYN approach. It contains a total of twelve scenarios, and each scenario consisted of two items (one item in the KAC phase and one item in the KAP phase in each problem scenario). Twelve KAC items and twelve KAP items were therefore delivered on the CPS test for a total of twenty-four items. Each scenario has a fictional cover story. For instance, students found a sick cat in front of their house, and they were expected to feed the cat with two different kinds of cat food to help it recover.
Each item contains up to three input and three output variables. The relations between the input and output variables were formulated with linear structural equations ( Funke 2001 ). Figure 1 shows a MicroDYN sample structure containing three input variables (A, B and C), three output variables (X, Y and Z) and a number of possible relations between the variables. The complexity of the item was defined by the number of input and output variables, and the number of relations between the variables. The test began with the item with the lowest complexity. The complexity of each item gradually increased as the test progressed.

A typical MicroDYN structure with three input variables and three output variables ( Greiff and Funke 2009 ).
The interface of each item displays the value of each variable in both numerical and figural forms (See Figure 2 ). Each of the input variables has a controller, which makes it possible to vary and set the value between +2 (+ +) and −2 (− −). To operate the system, students need to click the “+” or “−” button or use the slider directly to select the value they want to be added to or subtracted from the current value of the input variable. After clicking the “Apply” button in the interface, the input variables will add or subtract the selected value, and the output variables will show the corresponding changes. The history of the values for the input and output variables within the same problem scenario is displayed on screen. If students want to withdraw all the changes and set all the variables to their original status, they can click the “Reset” button.

Screenshot of the MicroDYN item Cat—first phase (knowledge acquisition). (The items were administered in Hungarian.)
In the first phase of the problem-solving process, the KAC phase, students are asked to interact with the system by changing the value of the input variables and observing and analysing the corresponding changes in the output variables. They are then expected to determine the relationship between the input and output variables and draw it in the form of (an) arrow(s) on the concept map at the bottom of the interface. To avoid item dependence in the second phase of the problem-solving process, the students are provided with a concept map during the KAP phase (see Figure 3 ), which shows the correct connections between the input and output variables. The students are expected to interact with the system by manipulating the input variables to make the output variables reach the given target values in four steps or less. That is, they cannot click on the “Apply” button more than four times. The first phase had a 180 s time limit, whereas the second had a 90 s time limit.

Screenshot of the MicroDYN item Cat—second phase (knowledge application). (The items were administered in Hungarian).
3.2.2. Inductive Reasoning (IR)
The IR instrument (see Figure 4 ) was originally designed and developed in Hungary ( Csapó 1997 ). In the last 25 years, the instrument has been further developed and scaled for a wide age range ( Molnár and Csapó 2011 ). In addition, figural items have been added, and the assessment method has evolved from paper-and-pencil to computer-based ( Pásztor 2016 ). Currently, the instrument is widely employed in a number of countries (see, e.g., Mousa and Molnár 2020 ; Pásztor et al. 2018 ; Wu et al. 2022 ; Wu and Molnár 2018 ). In the present study, four types of items were included after test adaptation: figural series, figural analogies, number analogies and number series. Students were expected to ascertain the correct relationship between the given figures and numbers and select a suitable figure or number as their answer. Students used the drag-and-drop operation to provide their answers. In total, 49 inductive reasoning items were delivered to the participating students.

Sample items for the IR test. (The items were administered in Hungarian.).
3.2.3. Combinatorial Reasoning (CR)
The CR instrument (see Figure 5 ) was originally designed by Csapó ( 1988 ). The instrument was first developed in paper-and-pencil format and then modified for computer use ( Pásztor and Csapó 2014 ). Each item contained figural or verbal elements and a clear requirement for combing through the elements. Students were asked to list every single combination based on a given rule they could find. For the figural items, students provided their answers using the drag-and-drop operation; for the verbal items, they were asked to type their answers in a text box provided on screen. The test consisted of eight combinatorial reasoning items in total.

Sample item for the CR test. (The items were administered in Hungarian).
3.3. Scoring
Students’ performance was automatically scored via the eDia platform. Items on the CPS and IR tests were scored dichotomously. In the first phase (KAC) of the CPS test, if a student drew all the correct relations on the concept map provided on screen within the given timeframe, his/her performance was assigned a score of 1 or otherwise a score of 0. In the second phase (KAP) of the CPS test, if the student successfully reached the given target values of the output variables by manipulating the level of the input variables within no more than four steps and the given timeframe, then his/her performance earned a score of 1 or otherwise a score of 0. On the IR test items, if a student selected the correct figure or number as his/her answer, then he or she received a score of 1; otherwise, the score was 0.
Students’ performance on the CR test items was scored according to a special J index, which was developed by Csapó ( 1988 ). The J index ranges from 0 to 1, where 1 means that the student provided all the correct combinations without any redundant combinations on the task. The formula for computing the J index is the following:
x stands for the number of correct combinations in the student’s answer,
T stands for the number of all possible correct combinations, and
y stands for the number of redundant combinations in the student’s answer.
Furthermore, according to Csapó ’s ( 1988 ) design, if y is higher than T, then the J index will be counted as 0.
3.4. Coding and Labelling the Logfile Data
Beyond concrete answer data, students’ interaction and manipulation behaviour were also logged in the assessment system. This made it possible to analyse students’ exploration behaviour in the first phase of the CPS process (KAC phase). Toward this aim, we adopted a labelling system developed by Molnár and Csapó ( 2018 ) to transfer the raw logfile data to structured data files for analysis. Based on the system, each trial (i.e., the sum of manipulations within the same problem scenario which was applied and tested by clicking the “Apply” button) was modelled as a single data entity. The sum of these trials within the same problem was defined as a strategy. In our study, we only consider the trials which were able to provide useful and new information for the problem-solvers, whereas the redundant or operations trials were excluded.
In this study, we analysed students’ trials to determine the extent to which they used the VOTAT strategy: fully, partially or not at all. This strategy is the most successful exploration strategy for such problems; it is the easiest to interpret and provides direct information about the given variable without any mediation effects ( Fischer et al. 2012 ; Greiff et al. 2018 ; Molnár and Csapó 2018 ; Wüstenberg et al. 2014 ; Wu and Molnár 2021 ). Based on the definition of VOTAT noted in Section 1.3 , we checked students’ trials to ascertain if they systematically varied one input variable while keeping the others unchanged, or applied a different, less successful strategy. We considered the following three types of trials:
- “Only one single input variable was manipulated, whose relationship to the output variables was unknown (we considered a relationship unknown if its effect cannot be known from previous settings), while the other variables were set at a neutral value like zero […]
- One single input variable was changed, whose relationship to the output variables was unknown. The others were not at zero, but at a setting used earlier. […]
- One single input variable was changed, whose relationship to the output variables was unknown, and the others were not at zero; however, the effect of the other input variable(s) was known from earlier settings. Even so, this combination was not attempted earlier” ( Molnár and Csapó 2018, p. 8 )
We used the numbers 0, 1 and 2 to distinguish the level of students’ use of the most effective exploration strategy (i.e., VOTAT). If a student applied one or more of the above trials for every input variable within the same scenario, we considered that they had used the full VOTAT strategy and labelled this behaviour 2. If a student had only employed VOTAT on some but not all of the input variables, we concluded that they had used a partial VOTAT strategy for that problem scenario and labelled it 1. If a student had used none of the trials noted above in their problem exploration, then we determined that they had not used VOTAT at all and thus gave them a label of 0.
3.5. Data Analysis Plan
We used LCA (latent class analysis) to explore students’ exploration strategy profiles. LCA is a latent variable modelling approach that can be used to identify unmeasured (latent) classes of samples with similarly observed variables. LCA has been widely used in analysing logfile data for CPS assessment and in exploring students’ behaviour patterns (see, e.g., Gnaldi et al. 2020 ; Greiff et al. 2018 ; Molnár et al. 2022 ; Molnár and Csapó 2018 ; Mustafić et al. 2019 ; Wu and Molnár 2021 ). The scores for the use of VOTAT in the KAC phase (0, 1, 2; see Section 3.4 ) were used for the LCA analysis. We used Mplus ( Muthén and Muthén 2010 ) to run the LCA analysis. Several indices were used to measure the model fit: AIC (Akaike information criterion), BIC (Bayesian information criterion) and aBIC (adjusted Bayesian information criterion). With these three indicators, lower values indicate a better model fit. Entropy (ranging from 0 to 1, with values close to 1 indicating high certainty in the classification). The Lo–Mendell–Rubin adjusted likelihood ratio was used to compare the model containing n latent classes with the model containing n − 1 latent classes, and the p value was the indicator for whether a significant difference could be detected ( Lo et al. 2001 ). The results of the Lo–Mendell–Rubin adjusted likelihood ratio analysis were used to decide the correct number of latent classes in LCA models.
ANOVA was used to analyse the performance differences for CPS, IR and CR across the students from the different class profiles. The analysis was run using SPSS. A path analysis (PA) was employed in the structural equation modelling (SEM) framework to investigate the roles of CR and IR in CPS and the similarities and differences across the students from the different exploration strategy profiles. The PA models were carried out with Mplus. The Tucker–Lewis index (TLI), the comparative fit index (CFI) and the root-mean-square error of approximation (RMSEA) were used as indicators for the model fit. A TLI and CFI larger than 0.90 paired with a RMSEA less than 0.08 are commonly considered as an acceptable model fit ( van de Schoot et al. 2012 ).
4.1. Descriptive Results
All three tests showed good reliability (Cronbach’s α: CPS: 0.89; IR: 0.87; CR: 0.79). Furthermore, the two sub-dimensions of the CPS test, KAC and KAP, also showed satisfactory reliability (Cronbach’s α: KAC: 0.86; KAP: 0.78). The tests thus proved to be reliable. The means and standard deviations of students’ performance (in percentage) on each test are provided in Table 1 .
The means and standard deviations of students’ performance on each test.
4.2. Four Qualitatively Different Exploration Strategy Profiles Can Be Distinguished in CPS
Based on the labelled logfile data for CPS, we applied latent class analyses to identify the behaviour patterns of the students in the exploration phase of the problem-solving process. The model fits for the LCA analysis are listed in Table 2 . Compared with the 2 or 3 latent class models, the 4 latent class model has a lower AIC, BIC and aBIC, and the likelihood ratio statistical test (the Lo–Mendell–Rubin adjusted likelihood ratio test) confirmed it has a significantly better model fit. The 5 and 6 latent class models did not show a better model fit than the 4 latent class model. Therefore, based on the results, four qualitatively different exploration strategy profiles can be distinguished, which covered 96% of the students.
Fit indices for latent class analyses.
The patterns for the four qualitatively different exploration strategy profiles are shown in Figure 6 . In total, 84.3% of the students were proficient exploration strategy users, who were able to use VOTAT in each problem scenario independent of its difficulty level (represented by the red line in Figure 5 ). In total, 6.2% of the students were rapid learners. They were not able to apply VOTAT at the beginning of the test on the easiest problems but managed to learn quickly, and, after a rapid learning curve by the end of the test, they reached the level of proficient exploration strategy users, even though the problems became much more complex (represented by the blue line). In total, 3.1% of the students proved to be non-persistent explorers, and they employed VOTAT on the easiest problems but did not transfer this knowledge to the more complex problems. Finally, they were no longer able to apply VOTAT when the complexity of the problems increased (represented by the green line). In total, 6.5% of the students were non-performing explorers; they barely used any VOTAT strategy during the whole test (represented by the pink line) independent of problem complexity.

Four qualitatively different exploration strategy profiles.
4.3. Better Exploration Strategy Users Showed Better Performance in Reasoning Skills
Students with different exploration strategy profiles showed different kinds of performance in each reasoning skill under investigation. Results (see Table 3 ) showed that more proficient strategy users tended to have higher achievement in all the domains assessed as well as in the two sub-dimensions in CPS (i.e., KAC and KAP; ANOVA: CPS: F(3, 1339) = 187.28, p < 0.001; KAC: F(3, 1339) = 237.15, p < 0.001; KAP: F(3, 1339) = 74.91, p < 0.001; IR: F(3, 1339) = 48.10, p < 0.001; CR: F(3, 1339) = 28.72, p < 0.001); specifically, students identified as “proficient exploration strategy users” achieved the highest level on the reasoning skills tests independent of the domains. On average, they were followed by rapid learners, non-persistent explorers and, finally, non-performing explorers. Tukey’s post hoc tests revealed more details on the performance differences of students with different exploration profiles in each of the domains being measured. Proficient strategy users proved to be significantly more skilled in each of the reasoning domains. They were followed by rapid learners, who outperformed non-persistent explorers and non-performing explorers in CPS. In the domains of IR and CR, there were no achievement differences between rapid learners and non-persistent explorers, who significantly outperformed non-performing strategy explorers.
Students’ performance on each test—grouped according to the different exploration strategy profiles.
4.4. The Roles of IR and CR in CPS and Its Processes Were Different for Each Type of Exploration Strategy User
Path analysis was used to explore the predictive power of IR and CR for CPS and its processes, knowledge acquisition and knowledge application, for each group of students with different exploration strategy profiles. That is, four path analysis models were built to indicate the predictive power of IR and CR for CPS (see Figure 7 ), and another four path analyses models were developed to monitor the predictive power of IR and CR for the two empirically distinguishable phases of CPS (i.e., KAC and KAP) (see Figure 8 ). All eight models had good model fits, the fit indices TLI and CFI were above 0.90, and RMSEA was less than 0.08.

Path analysis models (with CPS, IR and CR) for each type of strategy user; * significant at 0.05 ( p < 0.05); ** significant at 0.01 ( p < 0.01); N.S.: no significant effect can be found.

Path analysis models (with KAC, KAP, IR and CR) for each type of strategy user; * significant at 0.05 ( p < 0.05); ** significant at 0.01 ( p < 0.01); N.S.: no significant effect can be found.
Students’ level of IR significantly predicted their level of CPS in all four path analysis models independent of their exploration strategy profile ( Figure 7 ; proficient strategy users: β = 0.432, p < 0.01; rapid learners: β = 0.350, p < 0.01; non-persistent explorers: β = 0.309, p < 0.05; and non-performing explorers: β = 0.386, p < 0.01). This was not the case for CR, which only proved to have predictive power for CPS among proficient strategy users (β = 0.104, p < 0.01). IR and CR were significantly correlated in all four models.
After examining the roles of IR and CR in the CPS process, we went further to explore the roles of these two reasoning skills in the distinguishable phases of CPS. The path analysis models ( Figure 8 ) showed that the predictive power of IR and CR for KAC and KAP was varied in each group. Levels of IR and CR among non-persistent explorers and non-performing explorers failed to predict their achievement in the KAC phase of the CPS process. Moreover, rapid learners’ level of IR significantly predicted their achievement in the KAC phase (β = 0.327, p < 0.01), but their level of CR did not have the same predictive power. Furthermore, the proficient strategy users’ levels of both reasoning skills had significant predictive power for KAC (IR: β = 0.363, p < 0.01; CR: β = 0.132, p < 0.01). In addition, in the KAP phase of the CPS problems, IR played a significant role for all types of strategy users, although with different power (proficient strategy users: β = 0.408, p < 0.01; rapid learners: β = 0.339, p < 0.01; non-persistent explorers: β = 0.361, p < 0.01; and non-performing explorers: β = 0.447, p < 0.01); by contrast, CR did not have significant predictive power for the KAP phase in any of the models.
5. Discussion
The study aims to investigate the role of IR and CR in CPS and its phases among students using statistically distinguishable exploration strategies in different CPS environments. We examined 1343 Hungarian university students and assessed their CPS, IR and CR skills. Both achievement data and logfile data were used in the analysis. The traditional achievement indicators formed the foundation for analysing the students’ CPS, CR and IR performance, whereas process data extracted from logfile data were used to explore students’ exploration behaviour in various CPS environments.
Four qualitatively different exploration strategy profiles were distinguished: proficient strategy users, rapid learners, non-persistent explorers and non-performing explorers (RQ1). The four profiles were consistent with the result of another study conducted at university level (see Molnár et al. 2022 ), and the frequencies of these four profiles in these two studies were very similar. The two studies therefore corroborate and validate each other’s results. The majority of the participants were identified as proficient strategy users. More than 80% of the university students were able to employ effective exploration strategies in various CPS environments. Of the remaining students, some performed poorly in exploration strategy use in the early part of the test (rapid learners), some in the last part (non-persistent explorers) and some throughout the test (non-performing explorers). However, students with these three exploration strategy profiles only constituted small portions of the total sample (with proportions ranging from 3.1% to 6.5%). The university students therefore exhibited generally good performance in terms of exploration strategy use in a CPS environment, especially compared with previous results among younger students (e.g., primary school students, see Greiff et al. 2018 ; Wu and Molnár 2021 ; primary to secondary students, see Molnár and Csapó 2018 ).
The results have indicated that better exploration strategy users achieved higher CPS performance and had better development levels of IR and CR (RQ2). First, the results have confirmed the importance of VOTAT in a CPS environment. This finding is consistent with previous studies (e.g., Greiff et al. 2015a ; Molnár and Csapó 2018 ; Mustafić et al. 2019 ; Wu and Molnár 2021 ). Second, the results have confirmed that effective use of VOTAT is strongly tied to the level of IR and CR development. Reasoning forms an important component of human intelligence, and the level of development in reasoning was an indicator of the level of intelligence ( Klauer et al. 2002 ; Sternberg and Kaufman 2011 ). Therefore, this finding has supplemented empirical evidence for the argument that effective use of VOTAT is associated with levels of intelligence to a certain extent.
The roles of IR and CR proved to be varied for each type of exploration strategy user (RQ3). For instance, the level of CPS among the best exploration strategy users (i.e., the proficient strategy users) was predicted by both the levels of IR and CR, but this was not the case for students with other profiles. In addition, the results have indicated that IR played important roles in both the KAC and KAP phases for the students with relatively good exploration strategy profiles (i.e., proficient strategy users and rapid learners) but only in the KAP phase for the rest of the students (non-persistent explorers and non-performing explorers); moreover, the predictive power of CR can only be detected in the KAC phase of the proficient strategy users. To sum up, the results suggest a general trend of IR and CR playing more important roles in the CPS process among better exploration strategy users.
Combining the answers to RQ2 and RQ3, we can gain further insights into students’ exploration strategy use in a CPS environment. Our results have confirmed that the use of VOTAT is associated with the level of IR and CR development and that the importance of IR and CR increases with proficiency in exploration strategy use. Based on these findings, we can make a reasonable argument that IR and CR are essential skills for using VOTAT and that underdeveloped IR and CR will prevent students from using effective strategies in a CPS environment. Therefore, if we want to encourage students to become better exploration strategy users, it is important to first enhance their IR and CR skills. Previous studies have suggested that establishing explicit training in using effective strategies in a CPS environment is important for students’ CPS development ( Molnár et al. 2022 ). Our findings have identified the importance of IR and CR in exploration strategy use, which has important implications for designing training programmes.
The results have also provided a basis for further studies. Future studies have been suggested to further link the behavioural and cognitive perspectives in CPS research. For instance, IR and CR were considered as component skills of CPS (see Section 1.2 ). The results of the study have indicated the possibility of not only discussing the roles of IR and CR in the cognitive process of CPS, but also exploration behaviour in a CPS environment. The results have thus provided a new perspective for exploring the component skills of CPS.
6. Limitations
There are some limitations in the study. All the tests were low stake; therefore, students might not be sufficiently motivated to do their best. This feature might have produced the missing values detected in the sample. In addition, some students’ exploration behaviour shown in this study might theoretically be below their true level. However, considering that data cleaning was adopted in this study (see Section 3.1 ), we believe this phenomenon will not have a remarkable influence on the results. Moreover, the CPS test in this study was based on the MicroDYN approach, which is a well-established and widely used artificial model with a limited number of variables and relations. However, it does not have the power to cover all kinds of complex and dynamic problems in real life. For instance, the MicroDYN approach cannot measure ill-defined problem solving. Thus, this study can only demonstrate the influence of IR and CR on problem solving in well-defined MicroDYN-simulated problems. Furthermore, VOTAT is helpful with minimally complex problems under well-defined laboratory conditions, but it may not be that helpful with real-world, ill-defined complex problems ( Dörner and Funke 2017 ; Funke 2021 ). Therefore, the generalizability of the findings is limited.
7. Conclusions
In general, the results have shed new light on students’ problem-solving behaviours in respect of exploration strategy in a CPS environment and explored differences in terms of the use of thinking skills between students with different exploration strategies. Most studies discuss students’ problem-solving strategies from a behavioural perspective. By contrast, this paper discusses them from both behavioural and cognitive perspectives, thus expanding our understanding in this area. As for educational implications, the study contributes to designing and revising training methods for CPS by identifying the importance of IR and CR in exploration behaviour in a CPS environment. To sum up, the study has investigated the nature of CPS from a fresh angle and provided a sound basis for future studies.
Funding Statement
This study has been conducted with support provided by the National Research, Development and Innovation Fund of Hungary, financed under the OTKA K135727 funding scheme and supported by the Research Programme for Public Education Development, Hungarian Academy of Sciences (KOZOKT2021-16).
Author Contributions
Conceptualization, H.W. and G.M.; methodology, H.W. and G.M.; formal analysis, H.W.; writing—original draft preparation, H.W.; writing—review and editing, G.M.; project administration, G.M.; funding acquisition, G.M. All authors have read and agreed to the published version of the manuscript.
Institutional Review Board Statement
Ethical approval was not required for this study in accordance with the national and institutional guidelines. The assessments which provided data for this study were integrated parts of the educational processes of the participating university. The participation was voluntary.
Informed Consent Statement
All of the students in the assessment turned 18, that is, it was not required or possible to request and obtain written informed parental consent from the participants.
Data Availability Statement
Conflicts of interest.
Authors declare no conflict of interest.
Publisher’s Note: MDPI stays neutral with regard to jurisdictional claims in published maps and institutional affiliations.
- Adey Philip, Csapó Benő. Developing and Assessing Scientific Reasoning. In: Csapó Benő, Szabó Gábor., editors. Framework for Diagnostic Assessment of Science. Nemzeti Tankönyvkiadó; Budapest: 2012. pp. 17–53. [ Google Scholar ]
- Batanero Carmen, Navarro-Pelayo Virginia, Godino Juan D. Effect of the implicit combinatorial model on combinatorial reasoning in secondary school pupils. Educational Studies in Mathematics. 1997; 32 :181–99. doi: 10.1023/A:1002954428327. [ CrossRef ] [ Google Scholar ]
- Beckmann Jens F., Guthke Jürgen. Complex problem solving, intelligence, and learning ability. In: Frensch Peter A., Funke Joachim., editors. Complex Problem Solving: The European Perspective. Erlbaum; Hillsdale: 1995. pp. 177–200. [ Google Scholar ]
- Buchner Axel. Basic topics and approaches to the study of complex problem solving. In: Frensch Peter A., Funke Joachim., editors. Complex Problem Solving: The European Perspective. Erlbaum; Hillsdale: 1995. pp. 27–63. [ Google Scholar ]
- Chen Yunxiao, Li Xiaoou, Liu Jincheng, Ying Zhiliang. Statistical analysis of complex problem-solving process data: An event history analysis approach. Frontiers in Psychology. 2019; 10 :486. doi: 10.3389/fpsyg.2019.00486. [ PMC free article ] [ PubMed ] [ CrossRef ] [ Google Scholar ]
- Csapó Benő. A kombinatív képesség struktúrája és fejlődése. Akadémiai Kiadó; Budapest: 1988. [ Google Scholar ]
- Csapó Benő. The development of inductive reasoning: Cross-sectional assessments in an educational context. International Journal of Behavioral Development. 1997; 20 :609–26. doi: 10.1080/016502597385081. [ CrossRef ] [ Google Scholar ]
- Csapó Benő. Teaching and Learning Thinking Skills. Swets & Zeitlinger; Lisse: 1999. Improving thinking through the content of teaching; pp. 37–62. [ Google Scholar ]
- Csapó Benő, Molnár Gyöngyvér. Online diagnostic assessment in support of personalized teaching and learning: The eDia System. Frontiers in Psychology. 2019; 10 :1522. doi: 10.3389/fpsyg.2019.01522. [ PMC free article ] [ PubMed ] [ CrossRef ] [ Google Scholar ]
- Dörner Dietrich, Funke Joachim. Complex problem solving: What it is and what it is not. Frontiers in Psychology. 2017; 8 :1153. doi: 10.3389/fpsyg.2017.01153. [ PMC free article ] [ PubMed ] [ CrossRef ] [ Google Scholar ]
- English Lyn D. Combinatorics and the development of children’s combinatorial reasoning. In: Jones Graham A., editor. Exploring Probability in School: Challenges for Teaching and Learning. Springer; New York: 2005. pp. 121–41. [ Google Scholar ]
- Fischer Andreas, Greiff Samuel, Funke Joachim. The process of solving complex problems. Journal of Problem Solving. 2012; 4 :19–42. doi: 10.7771/1932-6246.1118. [ CrossRef ] [ Google Scholar ]
- Frensch Peter A., Funke Joachim. Complex Problem Solving: The European Perspective. Psychology Press; New York: 1995. [ Google Scholar ]
- Funke Joachim. Dynamic systems as tools for analysing human judgement. Thinking and Reasoning. 2001; 7 :69–89. doi: 10.1080/13546780042000046. [ CrossRef ] [ Google Scholar ]
- Funke Joachim. Complex problem solving: A case for complex cognition? Cognitive Processing. 2010; 11 :133–42. doi: 10.1007/s10339-009-0345-0. [ PubMed ] [ CrossRef ] [ Google Scholar ]
- Funke Joachim. It Requires More Than Intelligence to Solve Consequential World Problems. Journal of Intelligence. 2021; 9 :38. doi: 10.3390/jintelligence9030038. [ PMC free article ] [ PubMed ] [ CrossRef ] [ Google Scholar ]
- Funke Joachim, Fischer Andreas, Holt Daniel V. Competencies for complexity: Problem solving in the twenty-first century. In: Care Esther, Griffin Patrick, Wilson Mark., editors. Assessment and Teaching of 21st Century Skills. Springer; Dordrecht: 2018. pp. 41–53. [ Google Scholar ]
- Gilhooly Kenneth J. Thinking: Directed, Undirected and Creative. Academic Press; London: 1982. [ Google Scholar ]
- Gnaldi Michela, Bacci Silvia, Kunze Thiemo, Greiff Samuel. Students’ complex problem solving profiles. Psychometrika. 2020; 85 :469–501. doi: 10.1007/s11336-020-09709-2. [ PubMed ] [ CrossRef ] [ Google Scholar ]
- Greiff Samuel, Funke Joachim. Measuring complex problem solving-the MicroDYN approach. In: Scheuermann Friedrich, Björnsson Julius., editors. The Transition to Computer-Based Assessment. Office for Official Publications of the European Communities; Luxembourg: 2009. pp. 157–63. [ Google Scholar ]
- Greiff Samuel, Holt Daniel V., Funke Joachim. Perspectives on problem solving in educational assessment: Analytical, interactive, and collaborative problem solving. Journal of Problem Solving. 2013; 5 :71–91. doi: 10.7771/1932-6246.1153. [ CrossRef ] [ Google Scholar ]
- Greiff Samuel, Molnár Gyöngyvér, Martina Romain, Zimmermann Johannes, Csapó Benő. Students’ exploration strategies in computer-simulated complex problem environments: A latent class approach. Computers & Education. 2018; 126 :248–63. [ Google Scholar ]
- Greiff Samuel, Wüstenberg Sascha, Avvisati Francesco. Computer-generated log-file analyses as a window into students’ minds? A showcase study based on the PISA 2012 assessment of problem solving. Computers & Education. 2015a; 91 :92–105. [ Google Scholar ]
- Greiff Samuel, Wüstenberg Sascha, Funke Joachim. Dynamic problem solving: A new measurement perspective. Applied Psychological Measurement. 2012; 36 :189–213. doi: 10.1177/0146621612439620. [ CrossRef ] [ Google Scholar ]
- Greiff Samuel, Wüstenberg Sascha, Csapó Benő, Demetriou Andreas, Hautamäki Jarkko, Graesser Arthur C., Martin Romain. Domain-general problem solving skills and education in the 21st century. Educational Research Review. 2014; 13 :74–83. doi: 10.1016/j.edurev.2014.10.002. [ CrossRef ] [ Google Scholar ]
- Greiff Samuel, Wüstenberg Sascha, Goetz Thomas, Vainikainen Mari-Pauliina, Hautamäki Jarkko, Bornstein Marc H. A longitudinal study of higher-order thinking skills: Working memory and fluid reasoning in childhood enhance complex problem solving in adolescence. Frontiers in Psychology. 2015b; 6 :1060. doi: 10.3389/fpsyg.2015.01060. [ PMC free article ] [ PubMed ] [ CrossRef ] [ Google Scholar ]
- Hołda Małgorzata, Głodek Anna, Dankiewicz-Berger Malwina, Skrzypińska Dagna, Szmigielska Barbara. Ill-defined problem solving does not benefit from daytime napping. Frontiers in Psychology. 2020; 11 :559. doi: 10.3389/fpsyg.2020.00559. [ PMC free article ] [ PubMed ] [ CrossRef ] [ Google Scholar ]
- Klauer Karl Josef. Paradigmatic teaching of inductive thinking. Learning and Instruction. 1990; 2 :23–45. [ Google Scholar ]
- Klauer Karl Josef, Willmes Klaus, Phye Gary D. Inducing inductive reasoning: Does it transfer to fluid intelligence? Contemporary Educational Psychology. 2002; 27 :1–25. doi: 10.1006/ceps.2001.1079. [ CrossRef ] [ Google Scholar ]
- Kuhn Deanna. What is scientific thinking and how does it develop? In: Goswami Usha., editor. The Wiley-Blackwell Handbook of Childhood Cognitive Development. Wiley-Blackwell; Oxford: 2010. pp. 371–93. [ Google Scholar ]
- Kuhn Deanna, Garcia-Mila Merce, Zohar Anat, Andersen Christopher, Sheldon H. White, Klahr David, Carver Sharon M. Strategies of knowledge acquisition. Monographs of the Society for Research in Child Development. 1995; 60 :1–157. doi: 10.2307/1166059. [ CrossRef ] [ Google Scholar ]
- Lo Yungtai, Mendell Nancy R., Rubin Donald B. Testing the number of components in a normal mixture. Biometrika. 2001; 88 :767–78. doi: 10.1093/biomet/88.3.767. [ CrossRef ] [ Google Scholar ]
- Lotz Christin, Scherer Ronny, Greiff Samuel, Sparfeldt Jörn R. Intelligence in action—Effective strategic behaviors while solving complex problems. Intelligence. 2017; 64 :98–112. doi: 10.1016/j.intell.2017.08.002. [ CrossRef ] [ Google Scholar ]
- Mayer Richard E. Cognitive, metacognitive, and motivational aspects of problem solving. Instructional Science. 1998; 26 :49–63. doi: 10.1023/A:1003088013286. [ CrossRef ] [ Google Scholar ]
- Molnár Gyöngyvér, Csapó Benő. Az 1–11 évfolyamot átfogó induktív gondolkodás kompetenciaskála készítése a valószínűségi tesztelmélet alkalmazásával. Magyar Pedagógia. 2011; 111 :127–40. [ Google Scholar ]
- Molnár Gyöngyvér, Csapó Benő. The efficacy and development of students’ problem-solving strategies during compulsory schooling: Logfile analyses. Frontiers in Psychology. 2018; 9 :302. doi: 10.3389/fpsyg.2018.00302. [ PMC free article ] [ PubMed ] [ CrossRef ] [ Google Scholar ]
- Molnár Gyöngyvér, Alrababah Saleh Ahmad, Greiff Samuel. How we explore, interpret, and solve complex problems: A cross-national study of problem-solving processes. Heliyon. 2022; 8 :e08775. doi: 10.1016/j.heliyon.2022.e08775. [ PMC free article ] [ PubMed ] [ CrossRef ] [ Google Scholar ]
- Molnár Gyöngyvér, Greiff Samuel, Csapó Benő. Inductive reasoning, domain specific and complex problem solving: Relations and development. Thinking Skills and Creativity. 2013; 9 :35–45. doi: 10.1016/j.tsc.2013.03.002. [ CrossRef ] [ Google Scholar ]
- Mousa Mojahed, Molnár Gyöngyvér. Computer-based training in math improves inductive reasoning of 9- to 11-year-old children. Thinking Skills and Creativity. 2020; 37 :100687. doi: 10.1016/j.tsc.2020.100687. [ CrossRef ] [ Google Scholar ]
- Mustafić Maida, Yu Jing, Stadler Matthias, Vainikainen Mari-Pauliina, Bornstein Marc H., Putnick Diane L., Greiff Samuel. Complex problem solving: Profiles and developmental paths revealed via latent transition analysis. Developmental Psychology. 2019; 55 :2090–101. doi: 10.1037/dev0000764. [ PMC free article ] [ PubMed ] [ CrossRef ] [ Google Scholar ]
- Muthén Linda K., Muthén Bengt O. Mplus User’s Guide. Muthén & Muthén; Los Angeles: 2010. [ Google Scholar ]
- Newell Allen. Reasoning, Problem Solving, and Decision Processes: The Problem Space as a Fundamental Category. MIT Press; Boston: 1993. [ Google Scholar ]
- Novick Laura R., Bassok Miriam. Problem solving. In: Holyoak Keith James, Morrison Robert G., editors. The Cambridge Handbook of Thinking and Reasoning. Cambridge University Press; New York: 2005. pp. 321–49. [ Google Scholar ]
- OECD . PISA 2012 Field Trial Problem Solving Framework. OECD Publishing; Paris: 2010. [ Google Scholar ]
- OECD . Results: Creative Problem Solving—Students’ Skills in Tackling Real-Life Problems (Volume V) OECD Publishing; Paris: 2014. [ Google Scholar ]
- Pásztor Attila. Ph.D. thesis. Doctoral School of Education, University of Szeged; Szeged, Hungary: 2016. Technology-Based Assessment and Development of Inductive Reasoning. [ Google Scholar ]
- Pásztor Attila, Csapó Benő. Improving Combinatorial Reasoning through Inquiry-Based Science Learning; Paper presented at the Science and Mathematics Education Conference; Dublin, Ireland. June 24–25; 2014. [ Google Scholar ]
- Pásztor Attila, Kupiainen Sirkku, Hotulainen Risto, Molnár Gyöngyvér, Csapó Benő. Comparing Finnish and Hungarian Fourth Grade Students’ Inductive Reasoning Skills; Paper presented at the EARLI SIG 1 Conference; Helsinki, Finland. August 29–31; 2018. [ Google Scholar ]
- Sandberg Elisabeth Hollister, McCullough Mary Beth. The development of reasoning skills. In: Sandberg Elisabeth Hollister, Spritz Becky L., editors. A Clinician’s Guide to Normal Cognitive Development in Childhood. Routledge; New York: 2010. pp. 179–89. [ Google Scholar ]
- Schraw Gregory, Dunkle Michael E., Bendixen Lisa D. Cognitive processes in well-defined and ill-defined problem solving. Applied Cognitive Psychology. 1995; 9 :523–38. doi: 10.1002/acp.2350090605. [ CrossRef ] [ Google Scholar ]
- Schweizer Fabian, Wüstenberg Sascha, Greiff Samuel. Validity of the MicroDYN approach: Complex problem solving predicts school grades beyond working memory capacity. Learning and Individual Differences. 2013; 24 :42–52. doi: 10.1016/j.lindif.2012.12.011. [ CrossRef ] [ Google Scholar ]
- Stadler Matthias, Becker Nicolas, Gödker Markus, Leutner Detlev, Greiff Samuel. Complex problem solving and intelligence: A meta-analysis. Intelligence. 2015; 53 :92–101. doi: 10.1016/j.intell.2015.09.005. [ CrossRef ] [ Google Scholar ]
- Sternberg Robert J. Handbook of Human Intelligence. Cambridge University Press; New York: 1982. [ Google Scholar ]
- Sternberg Robert J., Kaufman Scott Barry. The Cambridge Handbook of Intelligence. Cambridge University Press; New York: 2011. [ Google Scholar ]
- van de Schoot Rens, Lugtig Peter, Hox Joop. A checklist for testing measurement invariance. European Journal of Developmental Psychology. 2012; 9 :486–92. doi: 10.1080/17405629.2012.686740. [ CrossRef ] [ Google Scholar ]
- Vollmeyer Regina, Burns Bruce D., Holyoak Keith J. The impact of goal specificity on strategy use and the acquisition of problem structure. Cognitive Science. 1996; 20 :75–100. doi: 10.1207/s15516709cog2001_3. [ CrossRef ] [ Google Scholar ]
- Welter Marisete Maria, Jaarsveld Saskia, Lachmann Thomas. Problem space matters: The development of creativity and intelligence in primary school children. Creativity Research Journal. 2017; 29 :125–32. doi: 10.1080/10400419.2017.1302769. [ CrossRef ] [ Google Scholar ]
- Wenke Dorit, Frensch Peter A., Funke Joachim. Complex Problem Solving and intelligence: Empirical relation and causal direction. In: Sternberg Robert J., Pretz Jean E., editors. Cognition and Intelligence: Identifying the Mechanisms of the Mind. Cambridge University Press; New York: 2005. pp. 160–87. [ Google Scholar ]
- Wittmann Werner W., Hattrup Keith. The relationship between performance in dynamic systems and intelligence. Systems Research and Behavioral Science. 2004; 21 :393–409. doi: 10.1002/sres.653. [ CrossRef ] [ Google Scholar ]
- Wu Hao, Molnár Gyöngyvér. Interactive problem solving: Assessment and relations to combinatorial and inductive reasoning. Journal of Psychological and Educational Research. 2018; 26 :90–105. [ Google Scholar ]
- Wu Hao, Molnár Gyöngyvér. Logfile analyses of successful and unsuccessful strategy use in complex problem-solving: A cross-national comparison study. European Journal of Psychology of Education. 2021; 36 :1009–32. doi: 10.1007/s10212-020-00516-y. [ CrossRef ] [ Google Scholar ]
- Wu Hao, Saleh Andi Rahmat, Molnár Gyöngyvér. Inductive and combinatorial reasoning in international educational context: Assessment, measurement invariance, and latent mean differences. Asia Pacific Education Review. 2022; 23 :297–310. doi: 10.1007/s12564-022-09750-z. [ CrossRef ] [ Google Scholar ]
- Wüstenberg Sascha, Greiff Samuel, Funke Joachim. Complex problem solving—More than reasoning? Intelligence. 2012; 40 :1–14. doi: 10.1016/j.intell.2011.11.003. [ CrossRef ] [ Google Scholar ]
- Wüstenberg Sascha, Greiff Samuel, Molnár Gyöngyvér, Funke Joachim. Cross-national gender differences in complex problem solving and their determinants. Learning and Individual Differences. 2014; 29 :18–29. doi: 10.1016/j.lindif.2013.10.006. [ CrossRef ] [ Google Scholar ]

Empirical research on problem solving and problem posing: a look at the state of the art
- Survey Paper
- Published: 01 July 2021
- Volume 53 , pages 723–735, ( 2021 )
Cite this article
- Peter Liljedahl ORCID: orcid.org/0000-0003-3149-2282 1 &
- Jinfa Cai ORCID: orcid.org/0000-0002-0501-3826 2
4502 Accesses
39 Citations
Explore all metrics
Problem solving and problem posing have long been of interest to the mathematics education community. In this survey paper we first look at some of the seminal moments in the history of research on the important topics. We then use this history to position the state-of-the-art research being done in both problem solving and problem posing, before introducing the presented state-of-the-art developments in problem solving and problem posing. We then use this work as a backdrop against which to introduce the 16 empirical papers that make up this special issue. Together these 16 papers add nuance to what is already known about problem solving and problem posing; this nuance is the result of attending to very specific contexts and purposes in which these activities are embedded. We end the paper by discussing the future directions these fields can take.
This is a preview of subscription content, log in via an institution to check access.
Access this article
Price includes VAT (Russian Federation)
Instant access to the full article PDF.
Rent this article via DeepDyve
Institutional subscriptions
Similar content being viewed by others
Recent Advances in Research on Problem Solving and Problem Posing
Problem-Posing Research in Mathematics Education: Some Answered and Unanswered Questions
Four Mathematical Miniatures on Problem Posing
It is with great sadness that, as we write this historical survey on the emergence of problem solving and problem posing in mathematics education, we learn that Marion Walter passed away at the age of 92. Her contributions to mathematics education in general and problem posing in particular are significant, valuable, and much appreciated.
Amado, N., Carreira, S., & Nobre, S. (2019). The spreadsheet affordances in solving complex word problems. In P. Liljedahl & M. Santos-Trigo (Eds.), Mathematical problem solving: Current themes, trends, and research. Springer.
Google Scholar
Barron, B. (2003). When smart groups fail. Journal of the Learning Sciences, 12 (3), 307–359.
Article Google Scholar
Bartolini Bussi, M. G., & Funghi, S. (2019). Lesson study in primary pre-service teachers’ education: Influences on beliefs about lesson planning and conduction. In M. Graven, H. Venkat, A. Essien, & P. Vale (Eds.), Proceedings of the 43rd conference of the international group for the psychology of mathematics education (Vol. 2, pp. 81–88). PME.
Berk, D., & Cai, J. (2019). Mathematics teacher beliefs. In M. A. Peters (Ed.), Encyclopedia of teacher education. Singapore : Springer. https://doi.org/10.1007/978-981-13-1179-6_236-1
Chapter Google Scholar
Borba, M. C., & Villarreal, M. E. (2006). Humans-with-media and the reorganization of mathematical thinking: Information and communication technologies, modeling, visuali-zation and experimentation . Springer.
Brown, S. I., & Walter, M. I. (1983). The art of problem posing . Lawrence Erlbaum Associates.
Butts, T. (1980). Posing problems properly. In S. Krulik & R. E. Reys (Eds.), Problem solving in school mathematics (pp. 23–33). National Council of Teachers of Mathematics.
Cai, J. (1998). An investigation of U.S. and Chinese students’ mathematical problem posing and problem solving. Mathematics Education Research Journal, 10 (1), 37–50.
Cai, J. (2003a). What research tells us about teaching mathematics through problem solving. In F. Lester (Ed.), Research and issues in teaching mathematics through problem solving. National Council of Teachers of Mathematics.
Cai, J. (2003b). Singaporean students’ mathematical thinking in problem solving and problem posing: An exploratory study. International Journal of Mathematical Education in Science and Technology, 34 (5), 719–737.
Cai, J. (2010). Helping students becoming successful problem solvers. In D. V. Lambdin & F. K. Lester (Eds.), Teaching and learning mathematics: Translating research to the elementary classroom (pp. 9–14). NCTM.
Cai, J., & Hwang, S. (2020). Learning to teach mathematics through problem posing: Theoretical considerations, methodology, and directions for future research. International Journal of Educational Research, 102 , 101391.
Cai, J., & Hwang, S. (2021). Teachers as redesigners of curriculum to teach mathematics through problem posing: Conceptualization and initial findings of a problem-posing project. ZDM . https://doi.org/10.1007/s11858-021-01252-3
Cai, J., Hwang, S., Jiang, C., & Silber, S. (2015). Problem posing research in mathematics: Some answered and unanswered questions. In F. M. Singer, N. Ellerton, & J. Cai (Eds.), Mathematical problem posing: From research to effective practice (pp. 3–34). Springer.
Cai, J., Hwang, S., Melville, M. & Robson, V. (in press). Theories for teaching and teaching for theories: Artifacts as tangible entities for storing and improving professional knowledge for teaching. In A. Praetorius & C. Y. Charalambous (Eds.), Theorizing teaching: Bringing together expert perspectives to move the field forward . Springer.
Cai, J., & Jiang, C. (2017). An analysis of problem-posing tasks in Chinese and U.S. elementary mathematics textbooks. International Journal of Science and Mathematics Education, 15 , 1521–1540.
Cai, J., & Leikin, R. (2020). Affect in mathematical problem posing: Conceptualization, advances, and future directions for research. Educational Studies in Mathematics, 105 , 287–301.
Cai, J., & Mamlok-Naaman, R. (2020). Posing researchable questions in mathematics and science education: Purposefully questioning the questions for investigation. International Journal of Science and Mathematics Education, 18 , 1–7.
Carotenuto, G., Di Martin, P., & Lemmi, M. (2021). Students’ suspension of sense making in problem solving . ZDM Mathematics Education: DOI. https://doi.org/10.1007/s11858-020-01215-0 this special issue.
Book Google Scholar
Carreira, S., & Jacinto, H. (2019). A model of mathematical problem solving with technology: The case of Marco solving-and-expressing two geometry problems. In P. Liljedahl & M. Santos-Trigo (Eds.), Mathematical problem solving (pp. 41–62). Springer.
Chapman, O. (2016). An exemplary mathematics teacher’s way of holding problem-solving knowledge for teaching. In C. Csíkos, A. Rausch, & J. Szitányi (Eds.), Proceedings of the 40th conference of the international group for the psychology of mathematics education (Vol. 2, pp. 139–146). PME.
Chu, S., Quek, F., Saenz, M., Bhangaonkar, S., & Okundaye, O. (2015). Enabling instrumental interaction through electronics making: Effects on children’s storytelling. In H. Schoenauog, L. Bruni, S. Louchart, & S. Baceviciute (Eds.), Interactive storytelling. ICIDS 2015. Lecture notes in computer science (Vol. 9445, pp. 329–337). Springer.
Cirillo, M., & Hummer, J. (2021). Competencies and behaviors observed when students solve geometry proof problems: An interview study with Smartpen Technology . ZDM Mathematics Education: DOI. https://doi.org/10.1007/s11858-021-01221-w this special issue.
Clark, K., James, A., & Montelle, C. (2014). “We definitely wouldn’t be able to solve it all by ourselves, but together…”: Group synergy in tertiary students’ problem-solving practices. Research in Mathematics Education, 16 , 306–323.
Cobb, P. (2000). Conducting teaching experiments in collaboration with teachers. In A. Kelly & R. Lesh (Eds.), Handbook of research design in mathematics and science education (pp. 307–333). Lawrence Erlbaum.
Dahl, H., Klemp, T., & Nilssen, V. (2018). Collaborative talk in mathematics—Contrasting examples from third graders Education 3–13. International Journal of Primary, Elementary and Early Years Education, 46 (5), 599–611.
Dewey, J. (1910). How we think . D C Heath.
Di Mascio, R., Kalyuga, S., & Sweller, J. (2018). The effect of wording and placement of task instructions on problem-solving creativity. Journal of Creative Behavior, 52 (4), 335–353.
Einstein, A., & Infeld, L. (1938). The evolution of physics: The growth of ideas from early concepts to relativity and quanta . Cambridge University Press.
Elgrably, H., & Leikin, R. (2021). Creativity as a function of problem-solving expertise: Posing new problems through investigations. ZDM . https://doi.org/10.1007/s11858-021-01228-3 this special issue.
Ellerton, N. F. (1986). Children’s made-up mathematics problems—A new perspective on talented mathematicians. Educational Studies in Mathematics, 17 , 261–271.
English, L. (1998). Reasoning by analogy in solving comparison problems. Mathematical Cognition, 4 (2), 125–146.
English, L., & Sriraman, B. (2010). Problem solving for the 21st century. In L. English & B. Sriraman (Eds.), Theories of mathematics education (pp. 263–290). Springer.
Felmer, P., Kilpatrick, J., & Pehkonen, E. (2016). Posing and solving mathematical problems: Advances and new perspectives . Springer.
Felmer, P., Liljedahl, P., & Koichu, B. (2019). Problem solving in mathematics instruction and teacher professional development . Springer.
Forgasz, H. J., Vale, C., & Ursini, S. (2010). Technology for mathematics education: Equity, access, and agency. In C. Hoyles & J.-B. Lagrange (Eds.), Mathematics education and tech-nology: Rethinking the terrain (pp. 385–404). Springer.
Frensch, P. A., & Funke, J. (Eds.). (1995). Complex problem solving: The European perspective . Lawrence Erlbaum Associates.
Getzels, J. W. (1979). Problem finding: A theoretical note. Cognitive Science, 3 , 167–172.
Goldin, G., & McClintock, C. (Eds.). (1979). Task variables in mathematical problem solving . Lawrence Erlbaum.
Greefrath, G., Hertleif, C., & Siller, H. S. (2018). Mathematical modeling with digital tools—A quantitative study on mathematising with dynamic geometry software. ZDM, 50 (12), 233–244.
Greefrath, G., & Siller, H. S. (2017). Modeling and simulation with the help of digital tools. In G. Stillman, W. Blum, & G. Kaiser (Eds.), Mathematical modeling and applications (pp. 529–539). Springer.
Gros, B. (2016). The dialogue between emerging pedagogies and emerging technologies. In B. Gros (Ed.), The future of ubiquitous learning: Learning design for emerging pedagogies (pp. 3–23). Springer.
Guilford, J. P. (1950). Creativity. The American Psychologist, 5 (9), 444–454.
Guo, Y., Yan, J., & Men, T. (2021). Chinese junior high school students’ mathematical problem-posing performance. ZDM . https://doi.org/10.1007/s11858-021-01240-7 this special issue.
Hartmann, L.-M., Krawitz, J., & Schukajlow, S. (2021). Create your own problem! When given descriptions of real-world situations, do students pose and solve modeling problems? . ZDM . https://doi.org/10.1007/s11858-021-01224-7 this special issue.
Hilbert, D. (1901). 1902 Mathematical problems. Bulletin of American Mathematical Society, 8 , 437–479.
Hughes, J., Gadanidis, G., & Yiu, C. (2017). Digital making in elementary mathematics education. Digital Experiences in Mathematics Education, 3 (2), 139–153.
Jacinto, H., & Carreira, S. (2017). Mathematical problem solving with technology: The techno-mathematical fluency of a student-with-GeoGebra. International Journal of Science and Mathematics Education, 15 , 1115–1136.
Jacinto, H., Carreira, S., & Mariotti, M. A. (2016). Mathematical problem solving with tech-nology beyond the classroom: The use of unconventional tools and methods. In C. Csíkos, A. Rausch, & J. Szitányi (Eds.), Proceedings of the 40th conference of the international group for the psychology of mathematics education (Vol. 3, pp. 27–34). PME.
Jia, S., & Yao, Y. (2021). 70 years of problem-posing in Chinese primary mathematics textbooks. ZDM . https://doi.org/10.1007/s11858-021-01284-9 this special issue.
Kilpatrick, J. (1969). Problem solving and creative behavior in mathematics. In J. W. Wilson & R. Carey (Eds.), Reviews of recent research in mathematics education Studies in Mathematics Series (Vol. 19, pp. 153–187). School Mathematics Study Group.
Kilpatrick, J. (1978). Variables and methodologies in research on problem solving. In Hatfield & D. A. Bradbard (Eds.), Mathematical problem solving: From a research (pp. 7–20). ERIC: Columbus.
Kilpatrick, J. (1985). A retrospective account of the past twenty-five years of research on teaching mathematical problem solving. In E. A. Silver (Ed.), Teaching and learning mathematical problem solving: Multiple research perspectives (pp. 1–16). Erlbaum.
Kilpatrick, J. (1987). Problem formulating: Where do good problems come from? In A. H. Schoenfeld (Ed.), Cognitive science and mathematics education (pp. 123–147). Lawrence Erlbaum Associates.
Kilpatrick, J. (1992). A history of research in mathematics education. In D. A. Grouws (Ed.), Handbook of research on mathematics teaching and learning (pp. 3–38). Macmillan.
Koichu, B. (2015). Towards a confluence framework of problem solving in educational contexts. In K. Krainer & N. Vondrová (Eds.), Proceedings of the Ninth Conference of the European Society for Research in Mathematics Education (pp. 2668–2674). Charles University.
Koichu, B. (2018). Mathematical problem solving in choice-affluent environments. In A. Kuzniak & H. Forgasz (Eds.), Invited lectures from the 13th international congress on mathematical education (pp. 307–324). Springer.
Koichu, B. (2019). A discursively oriented conceptualization of mathematical problem solving. In P. Felmer, P. Liljedahl, & B. Koichu (Eds.), Problem solving in mathematics instruction and teacher professional development (pp. 43–66). Springer.
Koichu, B., & Keller, N. (2017). Implementation enterprise through the lens of a theory of diffusion of innovations: A case of online problem-solving forums. The 10th Conference of the European Society for Research in Mathematics Education . Institute of Education, Dublin City University, Ireland. Available at https://keynote.conference-services.net/resources/444/5118/pdf/CERME10_0562.pdf .
Koichu, B., Parasha, R., & Tabach, M. (2021). Who-is-right tasks as a means for supporting collective looking-back practices. ZDM . https://doi.org/10.1007/s11858-021-01264-z this special issue.
Kyndt, E., Raes, E., Lismont, B., Timmers, F., Cascallar, E., & Dochy, F. (2013). A meta-analysis of the effects of face-to-face cooperative learning. Do recent studies falsify or verify earlier findings? Educational Research Review, 10 , 133–149.
Langer-Osuna, J. M. (2016). The social construction of authority among peers and its implications for collaborative mathematics problem solving. Mathematical Thinking and Learning, 18 (2), 107–124.
Lesh, R. (1985). Conceptual analysis of problem-solving performance. In E. A. Silver (Ed.), Teaching and learning mathematical problem solving: Multiple research perspectives (pp. 309–329). Erlbaum.
Lesh, R., & Doerr, H. (Eds.). (2003). Beyond problem solving: Models and modeling perspectives on mathematical problem solving, learning, and teaching . Erlbaum.
Lesh, R., & Zawojewski, J. S. (2007). Problem solving and modeling. In F. K. Lester (Ed.), The second handbook of research on mathematics teaching and learning (pp. 763–804). Information Age Publishing.
Lester, F. (1980). Research on mathematical problem solving. In R. J. Shumway (Ed.), Research in mathematics education (pp. 286–323). Reston: National Council of Teachers of Mathematics.
Lester, F. (1994). Musings about mathematical problem solving: 1970–1994. Journal of Research in Mathematics Education, 25 , 660–675.
Lester, F., & Cai, J. (2016). Can mathematical problem solving be taught? Preliminary answers from 30 years of research. In P. Felmer, J. Kilpatrick, & E. Pehkonen (Eds.), Posing and solving mathematical problems: Advances and new perspectives (pp. 117–136). Springer.
Lester, F., Garofalor, J., & Kroll, D. (1989). Self-confidence, interest, beliefs, and metacognition: Key influences on problem-solving behavior. In D. B. McLeod & V. M. Adams (Eds.), Affect and mathematical problem solving: A new perspective (pp. 75–88). Springer.
Liljedahl, P. (2020). Building thinking classrooms in mathematics (Grades K–12): 14 teaching practices for enhancing learning . Corwin Press.
Liljedahl, P., & Andrà, C. (2014). Students’ gazes: New insights into student interactions. In C. Bernack-Schüler, R. Erens, T. Leuders, & A. Eichler (Eds.), Views and beliefs in mathematics education (pp. 213–226). Springer.
Liljedahl, P., & Santos-Trigo, M. (Eds.). (2019). Mathematical problem solving: Current themes, trends, and research . Springer.
Liljedahl, P., Santos-Trigo, M., Malaspina Jurado, U., & Bruder, R. (Eds.). (2016). Problem solving in mathematics education . Springer.
Masingila, J., Olanoff, D., & Kimani, P. (2018). Mathematical knowledge for teaching teachers: Knowledge used and developed by mathematics teacher educators in learning to teach via problem solving. Journal of Mathematics Teacher Education, 21 , 429–450.
Mason, J., Burton, L., & Stacey, K. (1982). Thinking mathematically . Harlow: Pearson Prentice Hall.
Mellone, M., Pacelli, T., & Liljedahl, P. (2021). Cultural transposition of a thinking classroom: To conceive possible unthoughts in mathematical problem solving activity. ZDM . https://doi.org/10.1007/s11858-021-01256-z
Mellone, M., Ramploud, A., Di Paola, B., & Martignone, F. (2018). Cultural transposition: Italian didactic experiences inspired by Chinese and Russian perspectives on whole number arithmetic. ZDM, 51 (1), 199–212.
National Council of Teachers of Mathematics (NCTM). (1991). Professional standard for teaching mathematics . Author.
National Council of Teachers of Mathematics. (1989). Principles and standards for school mathematics . Author.
Ng, O., & Chan, T. (2019). Learning as making: Using 3D computer-aided design to enhance the learning of shapes and space in STEM-integrated ways. British Journal of Educational Technology, 50 (1), 294–308.
Ng, O. L., & Cui, Z. (2021). Examining primary students’ mathematical problem-solving in a programming context: Towards computationally enhanced mathematics education. ZDM . https://doi.org/10.1007/s11858-020-01200-7 this special issue.
Ng, O., & Ferrara, F. (2020). Towards a materialist vision of ‘learning as making’: The case of 3D printing pens in school mathematics. International Journal of Science and Mathematics Education, 18 , 925–944.
Papert, S. (1980). Mindstorms: Children, computers, and powerful ideas . Basic Books.
Pijls, M., Dekker, R., & Van Hout-Wolters, B. (2007). Reconstruction of a collaborative mathematical learning process. Educational Studies in Mathematics, 65 (3), 309–329.
Pólya, G. (1945). How to solve it . Princeton University Press.
Pruner, M., & Liljedahl, P. (2021). Collaborative problem solving in a choice-affluent environment. ZDM . https://doi.org/10.1007/s11858-021-01232-7
Rasmussen, C., Yackel, E., & King, K. (2003). Social and sociomathematical norms in mathematics classrooms. In F. Lester (Ed.), Teaching mathematics through problem solving: Grade (pp. 6–12). Author.
Rodríguez-Martínez, J., González-Calero, J., & Sáez-López, J. (2019). Computational thinking and mathematics using Scratch: An experiment with sixth-grade students. Interactive Learning Environments, 28 (3), 316–327.
Roschelle, J. (1992). Learning by collaborating: Convergent conceptual change. The Journal of the Learning Sciences, 2 (3), 235–276.
Rott, B. (2020). Teachers’ behaviors, epistemological beliefs, and their interplay in lessons on the topic of problem solving. International Journal of Science and Mathematics Education, 18 , 903–924.
Rott, B., Specht, B., & Knipping, C. (2021). A descriptive phase model of problem-solving processes. ZDM . https://doi.org/10.1007/s11858-021-01244-3
Russo, J., & Hopkins, S. (2019). Teachers’ perceptions of students when observing lessons involving challenging tasks. International Journal of Science and Mathematics Education, 17 , 759–779.
Ryve, A. (2006). Making explicit the analysis of students’ mathematical discourses—Revisiting a newly developed methodological framework. Educational Studies in Mathematics, 62 (1–3), 191–209.
Saadati, F., & Felmer, P. (2021). Assessing impact of a teacher professional development program on student problem-solving performance. ZDM . https://doi.org/10.1007/s11858-020-01214-1 this special issue.
Salminen-Saari, J., Moreno-Esteva, E., Haataja, E., Toivanen, M., Laine, A., & Hannula, M. (2021). Phases of collaborative mathematical problem solving and joint attention: A case study utilizing mobile gaze-tracking. ZDM . https://doi.org/10.1007/s11858-021-01280-z this special issue.
Santos-Trigo, M. (2019). Mathematical problem solving and the use of digital technologies. In P. Liljedahl & M. Santos-Trigo (Eds.), Mathematical problem solving (pp. 63–89). Springer.
Santos-Trigo, M., & Moreno-Armella, L., et al. (2016). The use of digital technologies to frame and foster learners’ problem-solving experiences. In P. Felmer (Ed.), Posing and solving mathematical problems, Research in Mathematics Education (pp. 189–207). Springer.
Schoenfeld, A. (1982). Some thoughts on problem-solving research and mathematics education. In F. Lester & J. Garofalo (Eds.), Mathematical problem solving: Issues in research (pp. 27–37). Franklin Institute Press.
Schoenfeld, A. (1985). Mathematical problem solving . Academic Press.
Schoenfeld, A. (1987). What’s all the fuss about metacognition? In A. H. Schoenfeld (Ed.), Cognitive science and mathematics education (pp. 189–215). Lawrence Erlbaum Associates.
Schoenfeld, A. (1992). Learning to think mathematically: Problem solving, metacognition, and sense making in mathematics. In D. A. Grouws (Ed.), Handbook of research on mathematics teaching and learning (pp. 334–370). Macmillan.
Sekiguchi, Y. (2021). Activity systems analysis of classroom teaching and learning of mathematics: A case study of Japanese secondary schools. Educational Studies in Mathematics . ( Published online: 05 June 2021 )
Silber, S., & Cai, J. (2021). Exploring underprepared undergraduate students’ mathematical problem posing. ZDM . https://doi.org/10.1007/s11858-021-01272-z this special issue.
Silver, E. (Ed.). (1985). Teaching and learning mathematical problem solving: Multiple research perspectives . Erlbaum.
Silver, E. (1988). Teaching and assessing mathematical problem solving: Towards a research agenda. In R. Charles & E. Silver (Eds.), The teaching and assessing of mathematical problem solving (pp. 273–282). The Council.
Silver, E. A. (1990). Contribution of research to practice: Applying findings, methods, and perspectives. In T. Cooney & C. R. Hirsch (Eds.), Teaching and learning mathematics in the 1990s (pp. 1–11). The Council.
Silver, E. A. (1994). On mathematical problem posing. For the Learning of Mathematics, 14 , 19–28.
Silver, E. A. (2013). Problem-posing research in mathematics education: Looking back, looking around, and looking ahead. Educational Studies in Mathematics, 83 , 157–162. https://doi.org/10.1007/s10649-013-9477-3
Silver, E. A., & Cai, J. (1996). An analysis of arithmetic problem posing by middle school students. Journal for Research in Mathematics Education, 27 , 521–539.
Silver, E., Mamona-Downs, J., Leung, S., & Kenney, P. (1996). Posing mathematical problems: An exploratory study. Journal for Research in Mathematics Education, 27 (3), 293–309. https://doi.org/10.2307/749366
Singer, F. M., Ellerton, N., & Cai, J. (2013). Problem-posing research in mathematics education: New questions and directions. Educational Studies in Mathematics, 83 , 1–7. https://doi.org/10.1007/s10649-013-9478-2
Singer, F. M., Ellerton, N. F., & Cai, J. (2015). Mathematical problem posing: From research to effective practice . New York: Springer.
Son, J. W., & Lee, M. Y. (2021). Exploring the relationship between preservice teachers’ conceptions of problem solving and their problem-solving performances. International Journal of Science and Mathematics Education, 19 , 129–150.
Stanic, G., & Kilpatrick, J. (1989). Historical perspectives on problem solving in the mathematics curriculum. In R. I. Charles & E. A. Silver (Eds.), The teaching and assessing of mathematical problem solving (pp. 1–22). Reston: National Council of Teachers of Mathematics.
Törner, G., Schoenfeld, A., & Reiss, K. (2007). Problem solving around the world: Summing up the state of the art. ZDM, 39 (5–6), 353.
van den Brink, J. F. (1987). Children as arithmetic book authors. For the Learning of Mathematics, 7 , 44–48.
Verschaffel, L., Schukajlow, S., Star, J., & Van Dooren, W. (2020). Word problems in mathematics education: A survey. ZDM, 52 , 1–16.
Voigt, J. (1994). Negotiation of mathematical meaning and learning mathematics. Educational Studies in Mathematics, 26 , 275–298.
Vörös, Z., Kehl, D., & Rouet, J. F. (2021). Task characteristics as source of difficulty and moderators of the effect of time-on-task in digital problem-solving. Journal of Educational Computing Research, 58 (8), 1494–1514.
Weber, K., & Leikin, R. (2016). Problem solving and problem posing. In A. Gutierez, G. Leder, & P. Boero (Eds.), 2nd Handbook on the psychology of mathematics education (pp. 353–382). Sense Publishers.
Wilson, J., Fernandez, M., & Hadaway, N. (1993). Mathematical problem solving. In P. S. Wilson (Ed.), Research ideas for the classroom: High school mathematics (pp. 57–77). MacMillan.
Yao, Y., Hwang, S., & Cai, J. (2021). Preservice teachers’ mathematical understanding exhibited in problem posing and problem solving. ZDM . https://doi.org/10.1007/s11858-021-01277-8 this special issue.
Yimmer, A., & Ellerton, N. F. (2010). A five-phase model for mathematical problem solving: Identifying synergies in pre-service-teachers’ metacognitive and cognitive actions. ZDM, 42 , 245–261.
Zhang, H., & Cai, J. (2021). Teaching mathematics through problem posing: Insights from an analysis of teaching cases. ZDM . https://doi.org/10.1007/s11858-021-01260-3 this special issue.
Download references
Author information
Authors and affiliations.
Simon Fraser University, Burnaby, BC, Canada
Peter Liljedahl
University of Delaware, Newark, DE, USA
You can also search for this author in PubMed Google Scholar
Corresponding author
Correspondence to Peter Liljedahl .
Additional information
Publisher's note.
Springer Nature remains neutral with regard to jurisdictional claims in published maps and institutional affiliations.
Rights and permissions
Reprints and permissions
About this article
Liljedahl, P., Cai, J. Empirical research on problem solving and problem posing: a look at the state of the art. ZDM Mathematics Education 53 , 723–735 (2021). https://doi.org/10.1007/s11858-021-01291-w
Download citation
Accepted : 24 June 2021
Published : 01 July 2021
Issue Date : August 2021
DOI : https://doi.org/10.1007/s11858-021-01291-w
Share this article
Anyone you share the following link with will be able to read this content:
Sorry, a shareable link is not currently available for this article.
Provided by the Springer Nature SharedIt content-sharing initiative
- Find a journal
- Publish with us
- Track your research
- Open access
- Published: 05 February 2018
The role of problem solving ability on innovative behavior and opportunity recognition in university students
- Ji Young Kim 1 ,
- Dae Soo Choi 1 ,
- Chang-Soo Sung 1 &
- Joo Y. Park 2
Journal of Open Innovation: Technology, Market, and Complexity volume 4 , Article number: 4 ( 2018 ) Cite this article
25k Accesses
32 Citations
1 Altmetric
Metrics details
Universities engage in entrepreneurship education to increase social value creation, through students’ new opportunities recognition. However, there are not enough of empirical researches on whether the current entrepreneurship education can be differentiated from other curriculum to improve the opportunity recognition process. This study argues that it is very important for cognitive abilities to be manifested as behavior when students in university are new opportunities recognition. For this purpose, the relationship between problem solving ability, innovation behavior, and opportunity perception was verified empirically. This study was conducted on 203 students who took entrepreneurship education courses at Korean universities. The results of this study showed that problem solving ability positively influenced innovation behavior and opportunity perception. Innovation behavior was identified as a key parameter that partially mediated the relationship between problem solving ability and innovation behavior. The implication of this study is to prove the relationship between individual ‘s problem - solving ability considering the characteristics of education in Korea and the opportunity through innovative behavior and various learning strategies to help entrepreneurship education to design better courses for the future It has important implications for strategic pedagogy that can enhance behavioral elements in development.
It is the new opportunity recognition that all firms focus on for a new economic paradigm (Ancona and Caldwell, 1992 ). Recognizing high opportunities can significantly improve profit, growth, and / or competitive positioning. And this new opportunity leads to innovation. From a conceptual point of view, research is continuing on the question of ‘what is opportunity’ and ‘where is opportunity’ (Gartner and Carter, 2003 ; Venkataraman & Sarasvathy, 2001 ). Research on the discovery and realization of new opportunities is a very important research area that suggests how to discover and utilize creative opportunities that create new value and profit for pre-service workers, and is the ultimate goal of entrepreneurship education. (Kim et al., 2016 ). Particularly, there is a lot of debate about the relationship between opportunity perception and personal characteristics. Despite many arguments, however, research on individual characteristics and opportunity perceptions is still insufficient, and a unified opinion has not been created due to differences between cognitive and behavioral theories (Ko & Butler, 2003 ). In particular, there is much controversy over the relationship between opportunity recognition and personal traits, and research has been continuing to demonstrate that organizational learning in organizations can influence opportunity recognition (Shane & Venkataraman, 2000 ). In particular, learning enhances cognitive ability, which is an opportunity that leads to opportunity recognition through the manifestation of behavior (Lumpkin and Dess, 2004 ). Many studies have also demonstrated the difference in behavior that successful entrepreneurs see as contributing to their ability to recognize opportunities and create innovative business ideas (Dyer et al., 2008 ; Kim et al., 2017 ). For example, Alvarez and Barney ( 2005 ) argue for mountain climbing and mountain building to understand the implications of entrepreneurial behavior in relation to these theories. In other words, a new opportunity for entrepreneurs is not a passive case that is generally found and climbed by climbers such as mountains, but rather by the actions of entrepreneurs, creating competition for the market, creating another market, Is the same. Therefore, in order for a person’s cognitive ability to recognize a new opportunity, it must focus on manifesting an action that can realize an innovative idea. In this regard, Kanter ( 1988 ) proved the relationship between new opportunity recognition and those with innovative tendencies and regarded this new opportunity recognition as innovation activity through organizational education. Scott and Bruce ( 1994 ) have integrated a number of research flows into innovation pioneers to develop and test individual innovative behavioral models. In particular, they argued that individual problem-solving styles are very important to induce innovative behavior. Although there are a number of studies on problem solving ability, innovation behavior, and new opportunities, most of the opportunistic researches have been conducted in organizational units of companies. Is still insufficient. Furthermore, unified opinions were not created due to differences between cognitive theory and behavioral theory (Ko & Butler, 2003 ). It is also true that the effects of entrepreneurship education in university have not been studied empirically because they are mainly focused on promoting cognitive ability and applied to various kinds of teaching methods.
This study argues that it is very important for cognitive abilities to be manifested as behavior that. “Through” courses, In other words, it is very important to induce students to act through ‘learning through process’ learning through behavioral learning by providing students with some (virtual or real) business to start doing some of the actions of the entrepreneur. When students in university are new opportunity recognition. Especially, entrepreneurship education, which ultimately focuses on whether it is a new opportunity, is very important to induce behavior through behavior learning beyond the cognitive ability as the general education curriculum. Particularly, innovative behaviors that create and realize innovative ideas are very important for new opportunity recognition (Paine & Organ, 2000 ).In order to achieve this, various kinds of teaching methods are being pursued in the university, but studies on the effectiveness of behavioral learning have not been studied yet. In this study, we are based on team-based learning among various teaching methods for behavior learning that leads to innovative behaviors. Team learning instructional activity sequence designed by Michaelsen and Sweet ( 2008 ), the most well known team-based learning in entrepreneurship education as in class-primarily group work and outside class-primarily individual work. In this way, we demonstrate empirically the relationship between individual problem solving ability and opportunity through innovative behavior, and develop a variety of learning strategies that help entrepreneurship education to design better courses for the future. I would like to point out some implications for strategic pedagogy to increase the element.
The paper proceeds as follows: Initially we present the theory of innovative behavior with individual problem-solving ability, innovative behavior and opportunity recognition. We develop hypotheses to confirm its basic predictions in the student context. Finally, we link the findings with the wider social effect of entrepreneurship literature and highlight the theoretical contributions and practical implications.
Theoretical background
‘opportunity recognition’ as entrepreneurship education unit of analysis.
A commonly focused analysis in entrepreneurship research over the last 30 years has been the ‘opportunity’, most simply defined as any situation in which new products or services can be development of production (Casson, 1982 ; Shane & Venkataraman, 2000 ; Venkataraman, 1997 ). The definition of opportunity recognition is defined in many ways, but opportunity is defined as a perceived means of generating economic value (ie, profit) that has not been exploited previously and is not currently exploited by others. If opportunity is defined in this way, opportunity recognition can be defined as a cognitive process (or process) that concludes that an individual has identified an opportunity (Baron and Ensley, 2006 ). Kirzner ( 1997 ) pointed out that the distribution of information in society affects the discovery of entrepreneurial opportunities and that only a few individuals can identify and recognize specific opportunities in the market. The process of finding opportunities also depends on the individual’s ability and discovery (Stevenson & Gumpert, 1985 ). For example, people may miss opportunities due to a lack of cognitive ability to change external environments (Stevenson & Gumpert, 1985 ). Only those who recognize and value the existence of opportunity can benefit from new opportunities (Ardichvili et al., 2003a , b ; Shane & Venkataraman, 2000 ). Opportunity recognition is an early step in transforming value into a business concept that creates value and generates revenue and distinguishes it from the aggressive stages of detailed assessment and development of recognized opportunities and potential economic value. The focus of the new venture business is also an innovative opportunity to create new opportunities rather than merely expanding or repeating existing business models (Gaglio & Katz, 2001 ). As a result, universities need to make use of a variety of initiatives to educate students to recognize innovative opportunities. Therefore, entrepreneurship education aimed at a new opportunity recognition should be able to provide learning opportunities based on various theories of favorable conditions for new business creation and the types of traits required for new ventures (Garavan & O’Cinne’ide, 1994 ).
Based on these considerations, we also define opportunity recognition as the formation of beliefs that can be translated into actions in order to understand the signals of change (new information on new conditions) and respond to these changes.
Problem-solving ability and innovative behavior of education for students
Problem-solving abilities have been proven to be one of the key factors for success in organizations and personal careers (Anderson & Anderson 1995 ). Through decades of research data, organizations and schools have studied factors that affect improvement. Problem-solving abilities are defined in a number of prior studies, and problem-solving abilities in a volatile and sophisticated knowledge- and technology-based industry are an important ability to drive innovation and sustainable growth and development in the industry. Table 1 show the concept of problem solving ability defined in previous research.
There have been a number of previous studies, emphasis has been placed on the importance and meaning of rational problem-solving processes in order to improve problem-solving abilities, and research has focused on individual problem solving styles (Woodman et al., 1993 ; Scott & Bruce, 1994 ). According to the personal innovation behavior model of Scott and Bruce ( 1994 ), climate has shown individual innovative behavior as a result of individuals signaling the organization’s expectations of behavior and the potential consequences of action. Innovative organizations are, last but not least, equipment, facilities and time, including the direction of creativity and innovative change (Kanter, 1983 ; Siegel & Kaemmerer, 1978 ) Proper supply of such resources is important to innovation (Amabile, 1988 ; Van de Ven & Angle, 1989 ; Dubickis & Gaile-Sarkane, 2017 ). Based on a study of Koestler’s ( 1964 ) creative thinking, Jabri conceptualized a problem-solving style consisting of two independent thinking styles. He uses a structured problem-solving styles that is based on associative thinking, follows a set of rules, resolves reasonably logically, and uses an intuitive problem-solving ability that focuses on problem-solving, not tied to existing rules with multiple ideas. Intuitive problem solving styles tend to process information from different paradigms simultaneously. It is therefore more likely to create new problem solutions as possible (Isaksen, 1987 ; Kirton, 1976 ). However, style assessment is not desirable because the style of problem solving affects style differently depending on the individual problem-solving situations (Scott & Bruce, 1994 ). We are proposing a role for the University to encourage innovative behavior based on the individuality of our students in order to recognize new opportunities through education about Scott and Bruce’s innovative behavioral models and diverse entrepreneurship education approaches. And involvement of resources, such as entrepreneurship awareness programs, ultimately leads to the identification of individual characteristics and innovation. In addition, current Korean entrepreneurship education is mainly focused on cognitive learning to improve problem solving ability, and one aspect of cognitive learning plays an important role in learning process of new venture firms. This study has a more direct focus on behavior learning such as team-based learning.
Hypothesis development
Problem-solving ability and innovative behavior.
Problem solving is to discover knowledge and skills that reach the target country by interfering with a set of processes and goals where the solution is unknown, unfamiliar, or reaching a new state of goal (Jonassen, 2004 ; Inkinen, 2015 ). There are various approaches to solve this problem. To solve problems and improve problem solving with a successful solution experience, you should adopt the method that best suits your problem solution. You need to select the appropriate inputs for the solution elements and a flexible process structure. Problem solving ability has been recognized as a key element of innovative behavior in responding to rapid changes with the ability to find various alternatives and predict outcomes from these alternatives to maximize positive results, minimize negative consequences, and select solutions to problems (Barron & Harrington, 1981 ; Jabri, 1991 ; Kirton, 1976 ). We pose the following hypotheses:
Hypothesis 1: Individual problem-solving ability has an effect on the innovative behavior of students.
Innovative behavior and opportunity recognition
Innovation involves introducing ideas from outside the organization, through creative processes, and linking these ideas to products or processes. Many scholars studying innovation recognize that designing ideas is only one step in the innovation process (Kanter, 1988 ). Innovation is changing at the organizational or individual level. Kanter, Scott and Bruce defined personal innovation. In other words, an innovation act starts with recognition of a problem, adoption of a new idea, or creation of a solution, and an individual with an innovative tendency wants to create a realistically realizable group with the sympathy of such an idea. Innovative individuals create prototypes for innovations that enable ideas to be realized specifically with goods or services and become productive use and social day merchandising. According to previous studies, opportunity perception can be seen as an individual’s corporate strategy that focuses on the perception and exploitation of individuals about potential business ideas and opportunities and finds resources to create innovative outcomes (Manev et al., 2005 ). New Venture Ideas (NVI) are imaginary combinations of product/service offerings; potential markets or users, and means of bringing these offerings into existence (Davidsson, 2015 ). From the viewpoint of a potential entrepreneur like a university student, entrepreneurship starts with an idea. This process continues with a range of practices including attractiveness and feasibility of an idea, gathering information to minimize value-related uncertainty and possibility and perhaps the main idea’s conformity ratio in terms of newly discovered needs (Hayton & Cholakova, 2012 ). Earlier we proposed that the program as a whole increases the students’ innovative behavior and that innovative performance is the new venture ideas. Since it is logical to assume that the relationship between innovative behavior and opportunity recognition. We pose the following hypotheses:
Hypothesis 2: Innovative behavior will be a more potent inducer of opportunity recognition.
Problem-solving ability and opportunity recognition
Among the many factors influencing opportunity perception, the problems that arise in the fourth industry, the knowledge-based industry of the twenty-first century, are unpredictable and unstructured; they cannot be solved with existing solutions and require creative problem-solving skills. In order to determine how to solve problem situations that are different from the current situation and have unknown results, problems are solved through the process of adjusting previous experience, knowledge, and intuition (Charles & Lester, 1982 ). Experience, knowledge, and intuition are applied simultaneously to a single problem, not individually or collectively, and the intellectual and creative results that can be quickly and effectively solved in problem solving are seen as problem solving abilities (Ardichvili et al., 2003a , b ). Empirical studies of problem-solving abilities and opportunity perceptions have provided strong evidence that there is a positive relationship between theoretical integrative processes and corporate opportunity recognition (Ucbasaran et al., 2009 ). Therefore, we hypothesized that:
Hypothesis 3: Problem solving ability has an effect on the opportunity recognition.
The respondents for this study were randomly selected from three universities in Korea. Most of the respondents in this study were Korean university students who experienced team-based learning during behavioral learning through entrepreneurship education. Since then, we have been guided by two main criteria when choosing these universities. First, students who take entrepreneurship courses are critical to their innovation behavior. This led us to realize that innovative behavior is an important factor in an individual’s survival and growth. The second is that the parallel process of theoretical and behavioral learning is highly satisfied. A pilot study was conducted to verify the reliability and validity of the research measurements with 28 students at a university. The results of the pilot study showed high clarity and reliability (Cronbach ‘s alphas were all above 0.70) of the research measurements. The sample of the pilot study was not incorporated in the present study.
This study was conducted in a four - year undergraduate course (various majors) that took entrepreneurship courses in Korea university programs. Students in this course have a mix of students who have previously experienced entrepreneurship and those who have not. During the course, students were taught the theoretical lessons for 8 weeks and the team for the 8 weeks. The questionnaire was administered during the last week of the course.
The data were analyzed from 203 participants, out of a total of 209, of which 7 were not appropriate. Of the 203 participants, 27% were female and 73% were male and the grade distribution was 3% for freshmen, 12% for grade 2, 26% for grade 2, and 59% for grade 2. The main distribution is 26% in social science, 16% in business and economics, 39% in engineering, 11% in music and athletics and 7% in others (see Table 2 ).
Measurement
The structure of the model was measured by questionnaires (problem-solving ability, innovation behavior and opportunity recognition questionnaire) consisting of the scale taken from questionnaires verified in previous studies. Tool selection was performed on two criteria. First, the selected tool should measure the same structure (ie, the original measured structure had to be conceptually identical to the way the structure was defined in this study model). Secondly, the psychometric qualities of the instrument for the student had to be high.
Assessment of the factors was carried out through principal component analyses (varimax rotation with eigenvalues of 1.0 or above) of the scales connected to the same level of the model to confirm the uniqueness of the scales with respect to each other. This was supplemented by the computation of the internal consistency reliability of the scales (Cronbach’s α). These analyses were executed using the individual participants’ responses (Nunnally & Bernstein, 1994 ).
Problem- solving ability was measured on a 7-point Likert-scale (1 = ‘completely disagree’; 7 = ‘completely agree’). Jabri ( 1991 ) used a measurement tool to measure individual problem solving ability.
Innovative behavior was measured on a 7-point Likert-scale (1 = ‘completely disagree’; 7 = ‘completely agree’). In order to measure innovation behavior, we modified the questionnaire items to fit the intention of this study among the questionnaire items used by Scott and Bruce ( 1994 ) and Kim and Rho ( 2010 ).
Opportunity recognition was measured on a 7-point Likert-scale (1 = ‘completely disagree’; 7 = ‘completely agree’). In order to measure opportunity recognition, we modified the questionnaire items to fit the intention of this study among the questionnaire items used by Kim and Rho ( 2010 ).
Methods of analysis
The first two parts of the analysis were primarily based on (multiple) regression analyses. The last part of the analysis was informed through the path analyses. The adequacy of the models was assessed by AMOS 18(Arbuckle & Wothke, 2003 ). Models were all tested with standardized coefficients obtained from the Principal Component Analysis. To ascertain the model fit, we analyzed the comparative fit index (CFI), the normed fit index (NFI), the Root Mean Square Err of Approximation (RMSEA), the standardized root mean square residual (SRMR) and the chi-square test statistic.
Reliability and validity are essential psychometrics to be reported. The first step to evaluate those aspects was to use the Cronbach’s alpha and the composite reliability to test reliability of the proposed scales. The usual threshold level is 0.7 for newly developed measures (Fornell and Larcker, 1981 ). Values range from 0.69 to 0.79 in the case of Cronbach’s alpha, and from 0.85 to 0.92 in the case of composite reliability (see Table 3 ). Therefore, these scales may be considered as reliable. Next, we estimated the research model, displayed in Fig. 1 , using structural equation modeling (SEM) and AMOS 18 (Arbuckle & Wothke, 2003 ). Our analysis revealed an adequate measurement model with high factor loadings for all the items on the expected factors and communalities of each item exceeding 0.50. We discuss three fit indices that are generally considered as important (Hu & Bentler, 1998 ). First, the CFI-value represents the overall difference between observed and predicted correlations. A value of 0.04 which is situated well below the cut-off value of 0.08, suggests that the hypothesized model resembles the actual correlations. Secondly, Bentler’s CFI (comparative fit index) greater than 0.90 and 0.95 which is above the cut-off of 0.90 (Schumacker & Lomax, 1996 ). Thirdly, NFI greater greater than 0.90 and 0.95 which is above the cut-off of 0.90 (Schumacker & Lomax, 1996 ). Fourthly, the standardized root mean square residual (SRMR) value of 0.0392 which is situated well below the cut-off value of 0.05(Hu & Bentler, 1998 ), and the chi-square value of 3581.622 which is situated well below the cut-off value of 0.0005. Finally, the RMSEA (root mean square error of approximation) equals 0.04 with a 90% confidence interval between 0.03 and 0.05.
Analysis of mediation effect
The value and confidence interval are situated over but below the cut-off value of 0.1 which suggests not a great but a good fit. Factor analysis was verified by factor analysis using principal component analysis and only factors with an eigenvalue of 1 or more by orthogonal rotation method were selected. Factor loading was considered to be significant at 0.5 or more (Hair et al., 2006a , b ). As a result of the analysis, cumulative explanation for 72.4% of the total variance. Confirmatory factor analysis thus supported the differentiation of the three components Also we tested the confirmatory validity of the construct by testing whether the structural linkage of each square is greater than the mean variance extraction (AVE) of each structure. The AVE ranged from 0.52 to 0.53, reaching the recommended level of .50 for both Fornell and Larcker ( 1981 ). Therefore, all constructs showed sufficient convergent validity (see Table 3 ).
As shown in Table 4 , the AVE value of each variable has a higher value than that of other factors. Therefore, the discriminant validity of the proposed model can be judged as appropriate.
Means, standard deviations, and correlations among the study variables are shown in Table 5 .
The mean scores for the conceptual model were as follows for problem-solving ability (MD. 5.20, SD.1.08), innovative behavior (MD.5.20, SD.1.03), and opportunity recognition (MD. 5.14, SD. 1.06) conditions. The means of problem-solving ability, innovative behavior, and opportunity recognition were high. Furthermore, those variables correlated positively with each other.
Figure 1 showed that all paths and their significance levels are presented in Table 6 . The path between the latent variables problem-solving ability and innovative behavior was significant (p, 0.001), consistent with Hypotheses 1. In addition, there was innovative behavior and opportunity recognition (p, 0.01), this result provide empirical support for Hypothesis 2.
H3 proposed that Problem-solving ability is positively related to opportunity recognition. The results of the correlation analysis: The coefficient of problem solving and opportunity perception weakened from .717 to .444, but it is still partly mediated because it is still significant (C. R = 7.604 ***). This supports H3 (see Table 6 ).
In order to verify the significance of the indirect effect, the bootstrapping must be performed in AMOS, and the actual significance test should be identified using two-tailed significance. As a result, the significance of indirect effect is 0.04 ( p < 0.05), which is statistically significant (see Table 7 ).
Discussion and conclusion
We have tried to demonstrate the effects of behavior and its significance by differentiating from the general curriculum emphasizing cognitive effects as a model of problem solving ability emerging as innovative behavior through opportunity of university entrepreneurship education.. This supports the premise that entrepreneurship education can improve opportunities or processes through behavioral learning. The results of this study support the role of entrepreneurship education in creating opportunities for innovative behavior and problem solving abilities. Entrepreneurship education should provide different types of learning for new opportunities and focus on what is manifested in behavior.
In addition, based on previous research, we propose whether the following contents are well followed and whether it is effective. First, the emergence of innovative behavior in problem-solving abilities increases as the cognitive diversity of students with diverse majors and diverse backgrounds increases. Second, the more entrepreneurial learning experiences, the greater the chance of new opportunities. Third, it is necessary to investigate students’ problem solving style and problem-solving ability first, and then a teaching strategy based on this combination of systematic and effective theory and practice is needed. Of course, as demonstrated by many studies, it may be easier to enhance the effectiveness of opportunity recognition through cognitive learning. This is because it emphasizes the achievement of knowledge and understanding with acquiring skills and competence. This process, however, is not enough for entrepreneurship education. However, we do not support full team-based behavioral learning in the class designed by Michaelsen and Sweet ( 2008 ). As with the results of this study, problem solving ability is positively related to opportunity perception directly. As previously demonstrated in previous studies, problem solving ability can be enhanced by cognitive learning (Anderson et al., 2001 ; Charles & Lester, 1982 ).
Therefore, it has been demonstrated that it is more efficient to balance a certain level of cognitive learning and behavior learning in consideration of the level of students in a course. Also this study satisfies the need for empirical research by Lumpkin and Lichtenstein ( 2005 ) and Robinson et al. ( 2016 ) and others. This will help to improve understanding of how entrepreneurship training is linked to various learning models and their effectiveness and to design better courses for the future. Finally, this study sought to provide an awareness of entrepreneurship education as the best curriculum for solutions that evolved into innovative behaviors that create new values and ultimately represent new opportunities. This study shows that it can positively influence the social effect of creating new value, that is, not only the cognitive effect of general pedagogy, but also the innovation behavior. By providing this awareness, we have laid the groundwork for empirical research on entrepreneurship education in order to create more opportunities for prospective students in education through education and to expand their capabilities.
Limitation and future research
Indeed, the concepts presented here and the limitations of this study have important implications that can fruitfully be addressed in future research. First, we selected a sample of college students taking entrepreneurship training. However, since it is not the whole of Korean university students, it is difficult to extend the research results to all college students in Korea. Second, there is no precedent research on the role of innovation behavior as intermedia in college students. Therefore, we were forced to proceed as an exploratory study.
The ability to recognize opportunities can provide significant benefits that can remain firm and competitive in an ever-changing environment. Future research should therefore expand these insights and try to empirically test more ways in which entrepreneurship pedagogy teaches how learning methods can be integrated into venture creation and growth processes to help new process opportunities. By providing this study, we will help entrepreneurship education in the university to create more opportunities and expand the capacity of prospective members.
Alvarez, S. A., & Barney, J. B. (2005). How do entrepreneurs organize firms under conditions of uncertainty? Journal of Management, 31 (5), 776–793.
Article Google Scholar
Amabile, T. M. (1988). A model of creativity and innovation in organizations. Research in Organizational Behavior, 10 (1), 123–167.
Google Scholar
Ancona, D. G., & Caldwell, D. F. (1992). Demography and design: Predictors of new product team performance. Organization Science, 3 (3), 321–341.
Anderson, P. M., & Anderson, P. M. (1995). Analysis of faulted power systems (Vol. 445). New York: IEEE press.
Anderson, L. W., Krathwohl, D. R., Airasian, P., Cruikshank, K., Mayer, R., Pintrich, P., & Wittrock, M. (2001). A taxonomy for learning, teaching and assessing: A revision of Bloom’s taxonomy . New York: Longman Publishing.
Arbuckle, J. L., & Wothke, W. (2003). AMOS 5 user’s guide . Chicago: Smallwaters.
Ardichvili, A., Cardozo, R., & Ray, S. (2003a). A theory of entrepreneurial opportunity identification and development. Journal of Business Venturing, 18 (1), 105–123.
Ardichvili, A., Cardozo, R., & Ray, S. (2003b). A theory of entrepreneurial opportunity identification and development. Journal of Business Venturing, 18 (1), 105–123.
Baron, R. A., & Ensley, M. D. (2006). Opportunity recognition as the detection of meaningful patterns: Evidence from comparisons of novice and experienced entrepreneurs. Management Science, 52 (9), 1331–1344.
Barron, F., & Harrington, D. M. (1981). Creativity, intelligence, and personality. Annual review of psychology, 32 (1), 439–476.
Casson, M. (1982). The entrepreneur: An economic theory . Lanham: Rowman & Littlefield.
Charles, R., & Lester, F. (1982). Teaching problem solving: What, why & how . Palo Alto: Dale Seymour Publications.
Davidsson, P. (2015). Entrepreneurial opportunities and the entrepreneurship nexus: A re-conceptualization. Journal of Business Venturing, 30 (5), 674–695.
Dubickis, M., & Gaile-Sarkane, E. (2017). Transfer of know-how based on learning outcomes for development of open innovation. Journal of Open Innovation : Technology, market, and complexity , 3 (1), 4.
Dyer, J. H., Gregersen, H. B., & Christensen, C. (2008). Entrepreneur behaviors, opportunity recognition, and the origins of innovative ventures. Strategic Entrepreneurship Journal, 2 (4), 317–338.
D'zurilla, T. J., & Nezu, A. M. (1990). Development and preliminary evaluation of the social problem-solving inventory. Psychological Assessment: A Journal of Consulting and Clinical Psychology, 2 (2), 156.
Fornell, C., & Larcker, D. F. (1981). Evaluating structural equation models with unobservable variables and measurement error. Journal of Marketing Research , 39–50.
Gaglio, C. M., & Katz, J. A. (2001). The psychological basis of opportunity identification: Entrepreneurial alertness. Small Business Economics, 16 (2), 95–111.
Garavan, T. N., & O’Cinneide, B. (1994). Entrepreneurship education and training programmes: A review and evaluation-part 1. Journal of European Industrial Training, 18 (8), 3–12.
Gartner, W. B., & Carter, N. M. (2003). Entrepreneurial behavior and firm organizing processes. In Handbook of entrepreneurship research (pp. 195–221). New Mexico: Springer US.
Hair, E., Halle, T., Terry-Humen, E., Lavelle, B., & Calkins, J. (2006a). Children's school readiness in the ECLS-K: Predictions to academic, health, and social outcomes in first grade. Early Childhood Research Quarterly, 21 (4), 431–454.
Hair, J. F., Black, W. C., Babin, B. J., Anderson, R. E., & Tatham, R. L. (2006b). Multivariate Data Analysis (6th ed.). Upper Saddle River: Pearson Education, Inc..
Hayton, J. C., & Cholakova, M. (2012). The role of affect in the creation and intentional pursuit of entrepreneurial ideas. Entrepreneurship Theory and Practice, 36 (1), 41–68.
Hu, L. T., & Bentler, P. M. (1998). Fit indices in covariance structure modeling: Sensitivity to underparameterized model misspecification. Psychological Methods, 3 (4), 424.
Inkinen, T. (2015). Reflections on the innovative city: Examining three innovative locations in a knowledge bases framework. Journal of Open Innovation : Technolodgy, market. Complexity, 1 (1), 8.
Isaksen, S. G. (1987). Frontiers of creativity research: Beyond the basics. Bearly Ltd.
Jabri, M. M. (1991). The development of conceptually independent subscales in the measurement of modes of problem solving. Educational and Psychological Measurement, 51 (4), 975–983.
Jonassen, D. H. (2004). Learning to solve problems: An instructional design guide (Vol. 6). Hoboken: John Wiley & Sons.
Kanter, R. M. (1983). The change masters: Binnovation and entrepreneturship in the American corporation. Touchstone Book.
Kanter, R. M. (1988). Three tiers for innovation research. Communication Research, 15 (5), 509–523.
Kim, H. C., Song, C. H., & An, B. R. (2016). A study on effects of personal characteristics on start-up opportunity and entrepreneurial intention of start-up. Korean Management Consulting review, 16 (3), 75–87.
Kim, S. A., Ryoo, H. Y., & Ahn, H. J. (2017). Student customized creative education model based on open innovation. Journal of Open Innovation : Technology, Market, and Complexity , 3 (1), 6.
Kim, T. H., & Roh, J. H. (2010). A Study of the Impact of Public Service Motivation on Innovative Behavior of Organizational Members. Korean Journal of Public Administration, 48(3).
Kirton, M. (1976). Adaptors and innovators: A description and measure. Journal of Applied Psychology, 61 (5), 622.
Kirzner, I. M. (1997). Entrepreneurial discovery and the competitive market process: An Austrian approach. Journal of Economic Literature, 35 (1), 60–85.
Ko, S., & Butler, J. E. (2003). Alertness, bisociative thinking ability, and discovery of entrepreneurial opportunities in Asian hi-tech firms.
Koestler, A. (1964). The act of creation: A study of the conscious and unconscious processes of humor, scientific discovery and art.
Lumpkin, G. T., & Dess, G. G. (2004). E-Business Strategies and Internet Business Models: How the Internet Adds Value. Organizational Dynamics, 33 (2), 161–173.
Lumpkin, G. T., & Lichtenstein, B. B. (2005). The role of organizational learning in the opportunity-recognition process. Entrepreneurship Theory and Practice, 29 (4), 451–472.
Manev, I. M., Gyoshev, B. S., & Manolova, T. S. (2005). The role of human and social capital and entrepreneurial orientation for small business performance in a transitional economy. International Journal of Entrepreneurship and Innovation Management, 5 (3–4), 298–318.
Michaelsen, L. K., & Sweet, M. (2008). The essential elements of team-based learning. New directions for teaching and learning, 2008 (116), 7–27.
Nunnally, J. C., & Bernstein, I. H. (1994). Validity. Psychometric theory, 99–132.
Paine, J. B., & Organ, D. W. (2000). The cultural matrix of organizational citizenship behavior: Some preliminary conceptual and empirical observations. Human Resource Management Review, 10 (1), 45–59.
Robinson, S., Neergaard, H., Tanggaard, L., & Krueger, N. F. (2016). New horizons in entrepreneurship education: from teacher-led to student-centered learning. Education+ Training, 58(7/8), 661–683.
Schumacker, R. E., & Lomax, R. G. (1996). A beginner's guide to structural equation modeling . Mahwah: Laurence Erlbaum Google Scholar.
Scott, S. G., & Bruce, R. A. (1994). Determinants of innovative behavior: A path model of individual innovation in the workplace. Academy of Management Journal, 37 (3), 580–607.
Shane, S. A. (2003). A general theory of entrepreneurship: The individual-opportunity nexus . Cheltenham: Edward Elgar Publishing.
Book Google Scholar
Shane, S., & Venkataraman, S. (2000). The promise of entrepreneurship as a field of research. Academy of Management Review, 25 (1), 217–226.
Siegel, S. M., & Kaemmerer, W. F. (1978). Measuring the perceived support for innovation in organizations. Journal of Applied Psychology, 63 (5), 553–562.
Spivack, G., Platt, J. J., & Shure, M. B. (1976). The problem-solving approach to adjustment . San Francisco: Jossey-Bass.
Stevenson, H., & Gumpert, D. (1985). The heart of entrepreneurship.
Stevenson, H. H. & J. C. Jarillo (1990). 'A paradigm of entrepreneurship: Entrepreneurial management', Strategic Management Journal, 11, pp. 17–27.
Ucbasaran, D., Westhead, P., & Wright, M. (2009). The extent and nature of opportunity identification by experienced entrepreneurs. Journal of Business Venturing, 24 (2), 99–115.
Van de Ven, A. H., & Angle, H. L. (1989). Suggestions for managing the innovation journey (No. 9). Strategic Management Research Center, University of Minnesota.
Venkataraman, S. (1997). The distinctive domain of entrepreneurship research. Advances in entrepreneurship, firm emergence and growth, 3 (1), 119–138.
Venkataraman, S., & Sarasvathy, S. D. (2001). Strategy and entrepreneurship: Outlines of an untold story.
Warner, M. (2002). Publics and counterpublics. Public Culture, 14 (1), 49–90.
Woodman, R. W., Sawyer, J. E., & Griffin, R. W. (1993). Toward a theory of organizational creativity. Academy of Management Review, 18 (2), 293–321.
Download references
Author information
Authors and affiliations.
Dept. of Technology Entrepreneurship (Graduate School), Dongguk University, 904 Chungmurogwn, Toegye-ro 36Gil, Jung-gu, Seoul, 100-272, South Korea
Ji Young Kim, Dae Soo Choi & Chang-Soo Sung
Yonsei School of Business, Yonsei University, 50 Yonsei-ro, Seodaemun-gu, Seoul, 120-749, South Korea
Joo Y. Park
You can also search for this author in PubMed Google Scholar
Corresponding author
Correspondence to Joo Y. Park .
Ethics declarations
Publisher’s note.
Springer Nature remains neutral with regard to jurisdictional claims in published maps and institutional affiliations.
Rights and permissions
Open Access This article is distributed under the terms of the Creative Commons Attribution 4.0 International License ( http://creativecommons.org/licenses/by/4.0/ ), which permits unrestricted use, distribution, and reproduction in any medium, provided you give appropriate credit to the original author(s) and the source, provide a link to the Creative Commons license, and indicate if changes were made.
Reprints and permissions
About this article
Cite this article.
Kim, J.Y., Choi, D.S., Sung, CS. et al. The role of problem solving ability on innovative behavior and opportunity recognition in university students. J. open innov. 4 , 4 (2018). https://doi.org/10.1186/s40852-018-0085-4
Download citation
Received : 12 September 2017
Accepted : 22 January 2018
Published : 05 February 2018
DOI : https://doi.org/10.1186/s40852-018-0085-4
Share this article
Anyone you share the following link with will be able to read this content:
Sorry, a shareable link is not currently available for this article.
Provided by the Springer Nature SharedIt content-sharing initiative
- Problem-solving ability
- Innovative behavior
- Opportunity recognition
- Entrepreneurship education
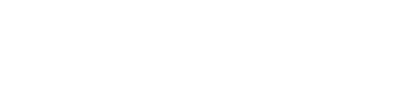
Along with Stanford news and stories, show me:
- Student information
- Faculty/Staff information
We want to provide announcements, events, leadership messages and resources that are relevant to you. Your selection is stored in a browser cookie which you can remove at any time using “Clear all personalization” below.
For everyone whose relationship with mathematics is distant or broken, Jo Boaler , a professor at Stanford Graduate School of Education (GSE), has ideas for repairing it. She particularly wants young people to feel comfortable with numbers from the start – to approach the subject with playfulness and curiosity, not anxiety or dread.
“Most people have only ever experienced what I call narrow mathematics – a set of procedures they need to follow, at speed,” Boaler says. “Mathematics should be flexible, conceptual, a place where we play with ideas and make connections. If we open it up and invite more creativity, more diverse thinking, we can completely transform the experience.”
Boaler, the Nomellini and Olivier Professor of Education at the GSE, is the co-founder and faculty director of Youcubed , a Stanford research center that provides resources for math learning that has reached more than 230 million students in over 140 countries. In 2013 Boaler, a former high school math teacher, produced How to Learn Math , the first massive open online course (MOOC) on mathematics education. She leads workshops and leadership summits for teachers and administrators, and her online courses have been taken by over a million users.
In her new book, Math-ish: Finding Creativity, Diversity, and Meaning in Mathematics , Boaler argues for a broad, inclusive approach to math education, offering strategies and activities for learners at any age. We spoke with her about why creativity is an important part of mathematics, the impact of representing numbers visually and physically, and how what she calls “ishing” a math problem can help students make better sense of the answer.
What do you mean by “math-ish” thinking?
It’s a way of thinking about numbers in the real world, which are usually imprecise estimates. If someone asks how old you are, how warm it is outside, how long it takes to drive to the airport – these are generally answered with what I call “ish” numbers, and that’s very different from the way we use and learn numbers in school.
In the book I share an example of a multiple-choice question from a nationwide exam where students are asked to estimate the sum of two fractions: 12/13 + 7/8. They’re given four choices for the closest answer: 1, 2, 19, or 21. Each of the fractions in the question is very close to 1, so the answer would be 2 – but the most common answer 13-year-olds gave was 19. The second most common was 21.
I’m not surprised, because when students learn fractions, they often don’t learn to think conceptually or to consider the relationship between the numerator or denominator. They learn rules about creating common denominators and adding or subtracting the numerators, without making sense of the fraction as a whole. But stepping back and judging whether a calculation is reasonable might be the most valuable mathematical skill a person can develop.
But don’t you also risk sending the message that mathematical precision isn’t important?
I’m not saying precision isn’t important. What I’m suggesting is that we ask students to estimate before they calculate, so when they come up with a precise answer, they’ll have a real sense for whether it makes sense. This also helps students learn how to move between big-picture and focused thinking, which are two different but equally important modes of reasoning.
Some people ask me, “Isn’t ‘ishing’ just estimating?” It is, but when we ask students to estimate, they often groan, thinking it’s yet another mathematical method. But when we ask them to “ish” a number, they're more willing to offer their thinking.
Ishing helps students develop a sense for numbers and shapes. It can help soften the sharp edges in mathematics, making it easier for kids to jump in and engage. It can buffer students against the dangers of perfectionism, which we know can be a damaging mindset. I think we all need a little more ish in our lives.
You also argue that mathematics should be taught in more visual ways. What do you mean by that?
For most people, mathematics is an almost entirely symbolic, numerical experience. Any visuals are usually sterile images in a textbook, showing bisecting angles, or circles divided into slices. But the way we function in life is by developing models of things in our minds. Take a stapler: Knowing what it looks like, what it feels and sounds like, how to interact with it, how it changes things – all of that contributes to our understanding of how it works.
There’s an activity we do with middle-school students where we show them an image of a 4 x 4 x 4 cm cube made up of smaller 1 cm cubes, like a Rubik’s Cube. The larger cube is dipped into a can of blue paint, and we ask the students, if they could take apart the little cubes, how many sides would be painted blue? Sometimes we give the students sugar cubes and have them physically build a larger 4 x 4 x 4 cube. This is an activity that leads into algebraic thinking.
Some years back we were interviewing students a year after they’d done that activity in our summer camp and asked what had stayed with them. One student said, “I’m in geometry class now, and I still remember that sugar cube, what it looked like and felt like.” His class had been asked to estimate the volume of their shoes, and he said he’d imagined his shoes filled with 1 cm sugar cubes in order to solve that question. He had built a mental model of a cube.
When we learn about cubes, most of us don’t get to see and manipulate them. When we learn about square roots, we don’t take squares and look at their diagonals. We just manipulate numbers.
I wonder if people consider the physical representations more appropriate for younger kids.
That’s the thing – elementary school teachers are amazing at giving kids those experiences, but it dies out in middle school, and by high school it’s all symbolic. There’s a myth that there’s a hierarchy of sophistication where you start out with visual and physical representations and then build up to the symbolic. But so much of high-level mathematical work now is visual. Here in Silicon Valley, if you look at Tesla engineers, they're drawing, they're sketching, they're building models, and nobody says that's elementary mathematics.
There’s an example in the book where you’ve asked students how they would calculate 38 x 5 in their heads, and they come up with several different ways of arriving at the same answer. The creativity is fascinating, but wouldn’t it be easier to teach students one standard method?
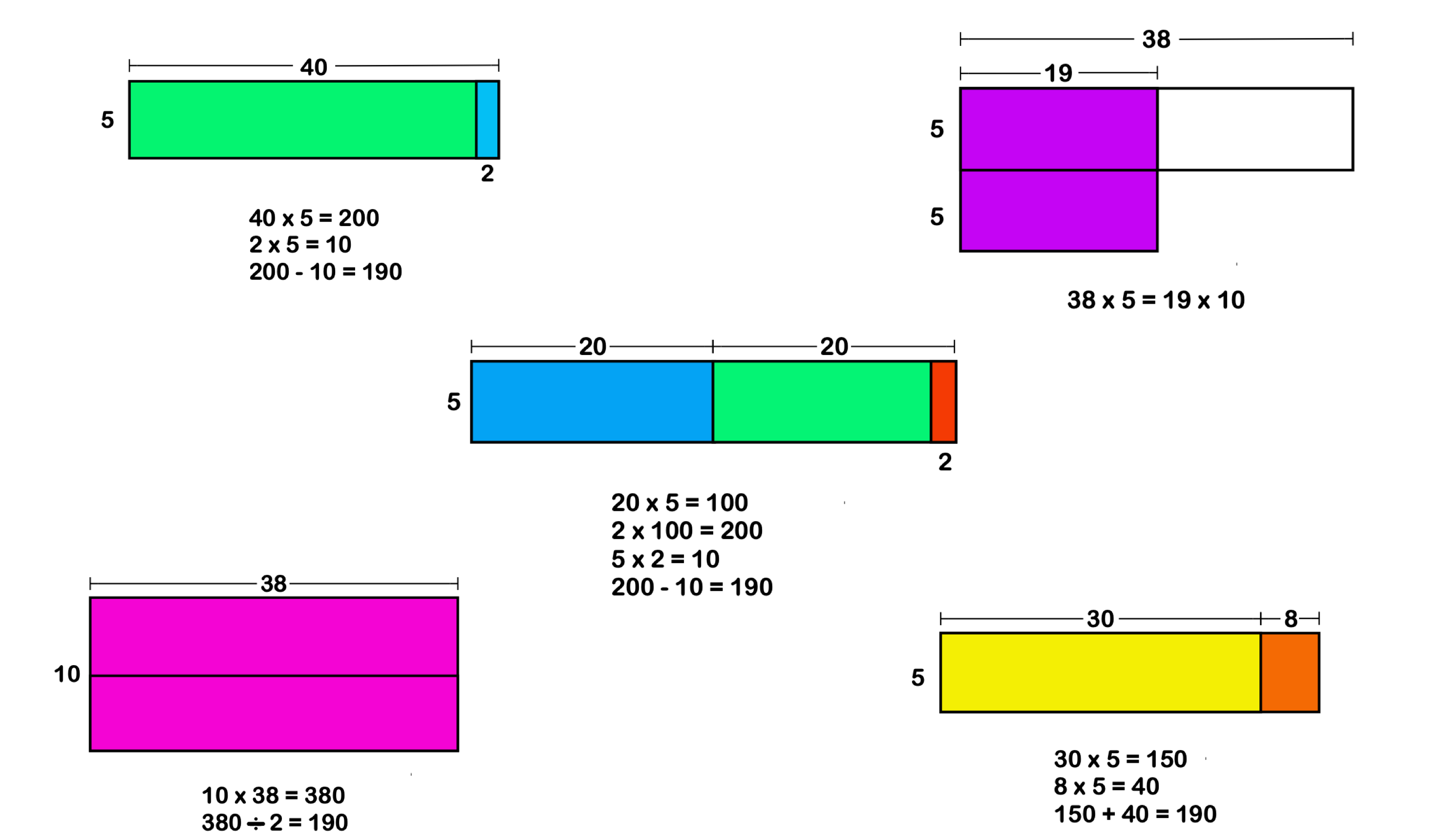
A depiction of various ways to calculate 38 x 5, numerically and visually. | Courtesy Jo Boaler
That narrow, rigid version of mathematics where there’s only one right approach is what most students experience, and it’s a big part of why people have such math trauma. It keeps them from realizing the full range and power of mathematics. When you only have students blindly memorizing math facts, they’re not developing number sense. They don’t learn how to use numbers flexibly in different situations. It also makes students who think differently believe there’s something wrong with them.
When we open mathematics to acknowledge the different ways a concept or problem can be viewed, we also open the subject to many more students. Mathematical diversity, to me, is a concept that includes both the value of diversity in people and the diverse ways we can see and learn mathematics. When we bring those forms of diversity together, it’s powerful. If we want to value different ways of thinking and problem-solving in the world, we need to embrace mathematical diversity.
- PRO Courses Guides New Tech Help Pro Expert Videos About wikiHow Pro Upgrade Sign In
- EDIT Edit this Article
- EXPLORE Tech Help Pro About Us Random Article Quizzes Request a New Article Community Dashboard This Or That Game Popular Categories Arts and Entertainment Artwork Books Movies Computers and Electronics Computers Phone Skills Technology Hacks Health Men's Health Mental Health Women's Health Relationships Dating Love Relationship Issues Hobbies and Crafts Crafts Drawing Games Education & Communication Communication Skills Personal Development Studying Personal Care and Style Fashion Hair Care Personal Hygiene Youth Personal Care School Stuff Dating All Categories Arts and Entertainment Finance and Business Home and Garden Relationship Quizzes Cars & Other Vehicles Food and Entertaining Personal Care and Style Sports and Fitness Computers and Electronics Health Pets and Animals Travel Education & Communication Hobbies and Crafts Philosophy and Religion Work World Family Life Holidays and Traditions Relationships Youth
- Browse Articles
- Learn Something New
- Quizzes Hot
- This Or That Game
- Train Your Brain
- Explore More
- Support wikiHow
- About wikiHow
- Log in / Sign up
- Education and Communications
- College University and Postgraduate
- Academic Writing
How to Write a Problem Solution Paper
Last Updated: July 22, 2023 Fact Checked
This article was co-authored by Stephanie Wong Ken, MFA . Stephanie Wong Ken is a writer based in Canada. Stephanie's writing has appeared in Joyland, Catapult, Pithead Chapel, Cosmonaut's Avenue, and other publications. She holds an MFA in Fiction and Creative Writing from Portland State University. This article has been fact-checked, ensuring the accuracy of any cited facts and confirming the authority of its sources. This article has been viewed 147,396 times.
A problem solution paper focuses on a particular problem or set of problems. As the essay writer, you will then need to come up with a solution or several solutions to the stated problem. Problem solution papers are common on exams, as they allow you to explore an issue and use critical thinking to respond with a solution. To write a problem solution paper, start by outlining the paper. Then, follow the structure of a problem solution paper and polish the paper so it is at its best when you turn it in.
Starting the Paper

- For example, you may have a main situation like, “obesity and poor fitness,” or “trigger warnings on college campuses.”
- If you get to choose the situation, make a list of groups you belong to, such as “school,” “family,” “race,” “culture”,” or “local community.” Then, identify a situation or issue you have encountered as a member of one of these groups.

- In the situation component, you will paraphrase the prompt of the paper in your own words.
- In the problem component, you will state the problem or problems and explain what they are in your own words.
- In the solution component, you will state your solution or solutions to the problem. You will also explain how it will address the problem.
- In the evaluation component, you will list the main ideas in the paper and offer a prediction or recommendation based on your solution to the problem.
- There will only be one situation presented to you in the prompt for the paper. You can then have multiple problems and multiple solutions that link back to the situation.

- Introduction section, where you discuss the situation
- Transition sentence or paragraph
- Conclusion section, where you discuss the evaluation

- Problem 1 and Solution to Problem 1
- Problem 2 and Solution to Problem 2
- Problem 3 and Solution to Problem 3
Writing the Paper

- For example, if the situation in the paper prompt is “obesity and poor fitness,” you may focus on specific aspects of the situation in the introduction. You may look at how the consumption of unhealthy food and the overuse of cars plays into obesity and poor fitness in society.

- If you cannot find a lot of outside material on the problem, you can collect your own data for the paper. Do this by making a survey that you give to people who are affected by the problem. You can also interview people associated with the problem, or with possible solutions.
- For example, if you were researching the problem “trigger warnings on college campuses,” you may interview college representatives at your university or college. You may also talk to students on campus.
- Most problem solution papers written for exams do not require you to cite any outside sources. You may need to cite your sources if you are writing the problem solution paper for a class.

- For example, if you were writing about the situation “obesity and poor fitness,” you may have the following thesis statement: “Obesity and poor fitness can lead to a decrease in life expectancy, and it is essential that individuals and governments work together to tackle this issue by improving their citizen's diet and fitness.”

- For example, you may come up with a solution that addresses a lack of resources by adding support, money, or more staff. Or you may come up with a solution that addresses the problem by changing an existing practice or habit.

- For example, if one of your solutions to the problem of obesity and poor fitness is to encourage people to cook at home, you may list a few specific ways people can do this. You may suggest that national eating healthy at home campaign is created, offering recipes online that take less than 30 minutes to prepare at home.

- For example, you may end up a call to action like, “With rising levels of obesity in our country, it is essential that we take action now to address this serious issue.”
Polishing the Paper

- You can create a reverse outline using your paper as a guide, where you go through each section and confirm it follows the outline you started with.

- You can also show the paper to a peer, friend, or family member and get them to proofread it for you.

- If you are writing the problem solution paper for a class assignment, you may still have a set word count. Check that your paper falls within this word count.
- ↑ https://www.jccc.edu/student-resources/academic-resource-center/writing-center/files/problem-solution-paper.pdf
- ↑ http://www.eapfoundation.com/writing/essays/problemsolution/
- ↑ https://grammar.yourdictionary.com/grammar/writing/how-to-write-a-problem-solution-essay.html
- ↑ https://www.shsu.edu/centers/academic-success-center/writing/handouts/modes/essays/9.-.Problem.Solution.Essay.pdf
- ↑ https://clt.library.jwu.edu/c.php?g=1028305&p=7459493
- ↑ https://clt.library.jwu.edu/c.php?g=1028305&p=7459493#s-lg-box-wrapper-27749528
Community Q&A

You Might Also Like

About This Article

A problem solution paper focuses on a particular issue and should include one or more solutions to it. You’ll need to begin the paper by stating the situation in your own words. For example, the situation could be “obesity and core fitness.” Include a thesis statement at the end of your introduction, which could be something like, “Obesity can lead to decreased life expectancy, making it imperative for governments and people to tackle this issue by improving diet and fitness.” The following sections should deal with identifying all of the problems arising from the situation and proposing solutions to them. Try to give examples to explain each solution. For instance, if you say the growth of obesity can be stopped by improving people’s diets, you could propose a national healthy eating campaign. Finally, you should conclude by evaluating the whole paper and making recommendations about how to implement your solutions. For tips from our Writing co-author on how to plan an outline for your problem solving paper, read on! Did this summary help you? Yes No
- Send fan mail to authors
Reader Success Stories

Hongjuan Yang
May 18, 2018
Did this article help you?

Liset Chevarria
Feb 5, 2020

Bertrand Bhikarry
Mar 18, 2020

Cressida Melchik
Sep 25, 2021

Featured Articles

Trending Articles

Watch Articles

- Terms of Use
- Privacy Policy
- Do Not Sell or Share My Info
- Not Selling Info
wikiHow Tech Help Pro:
Level up your tech skills and stay ahead of the curve

- SUGGESTED TOPICS
- The Magazine
- Newsletters
- Managing Yourself
- Managing Teams
- Work-life Balance
- The Big Idea
- Data & Visuals
- Reading Lists
- Case Selections
- HBR Learning
- Topic Feeds
- Account Settings
- Email Preferences
Share Podcast

A Better Framework for Solving Tough Problems
Start with trust and end with speed.
- Apple Podcasts
When it comes to solving complicated problems, the default for many organizational leaders is to take their time to work through the issues at hand. Unfortunately, that often leads to patchwork solutions or problems not truly getting resolved.
But Anne Morriss offers a different framework. In this episode, she outlines a five-step process for solving any problem and explains why starting with trust and ending with speed is so important for effective change leadership. As she says, “Let’s get into dialogue with the people who are also impacted by the problem before we start running down the path of solving it.”
Morriss is an entrepreneur and leadership coach. She’s also the coauthor of the book, Move Fast and Fix Things: The Trusted Leader’s Guide to Solving Hard Problems .
Key episode topics include: strategy, decision making and problem solving, strategy execution, managing people, collaboration and teams, trustworthiness, organizational culture, change leadership, problem solving, leadership.
HBR On Strategy curates the best case studies and conversations with the world’s top business and management experts, to help you unlock new ways of doing business. New episodes every week.
- Listen to the full HBR IdeaCast episode: How to Solve Tough Problems Better and Faster (2023)
- Find more episodes of HBR IdeaCast
- Discover 100 years of Harvard Business Review articles, case studies, podcasts, and more at HBR.org .
HANNAH BATES: Welcome to HBR On Strategy , case studies and conversations with the world’s top business and management experts, hand-selected to help you unlock new ways of doing business.
When it comes to solving complicated problems, many leaders only focus on the most apparent issues. Unfortunately that often leads to patchwork or partial solutions. But Anne Morriss offers a different framework that aims to truly tackle big problems by first leaning into trust and then focusing on speed.
Morriss is an entrepreneur and leadership coach. She’s also the co-author of the book, Move Fast and Fix Things: The Trusted Leader’s Guide to Solving Hard Problems . In this episode, she outlines a five-step process for solving any problem. Some, she says, can be solved in a week, while others take much longer. She also explains why starting with trust and ending with speed is so important for effective change leadership.
This episode originally aired on HBR IdeaCast in October 2023. Here it is.
CURT NICKISCH: Welcome to the HBR IdeaCast from Harvard Business Review. I’m Curt Nickisch.
Problems can be intimidating. Sure, some problems are fun to dig into. You roll up your sleeves, you just take care of them; but others, well, they’re complicated. Sometimes it’s hard to wrap your brain around a problem, much less fix it.
And that’s especially true for leaders in organizations where problems are often layered and complex. They sometimes demand technical, financial, or interpersonal knowledge to fix. And whether it’s avoidance on the leaders’ part or just the perception that a problem is systemic or even intractable, problems find a way to endure, to keep going, to keep being a problem that everyone tries to work around or just puts up with.
But today’s guest says that just compounds it and makes the problem harder to fix. Instead, she says, speed and momentum are key to overcoming a problem.
Anne Morriss is an entrepreneur, leadership coach and founder of the Leadership Consortium and with Harvard Business School Professor Francis Frei, she wrote the new book, Move Fast and Fix Things: The Trusted Leaders Guide to Solving Hard Problems . Anne, welcome back to the show.
ANNE MORRISS: Curt, thank you so much for having me.
CURT NICKISCH: So, to generate momentum at an organization, you say that you really need speed and trust. We’ll get into those essential ingredients some more, but why are those two essential?
ANNE MORRISS: Yeah. Well, the essential pattern that we observed was that the most effective change leaders out there were building trust and speed, and it didn’t seem to be a well-known observation. We all know the phrase, “Move fast and break things,” but the people who were really getting it right were moving fast and fixing things, and that was really our jumping off point. So when we dug into the pattern, what we observed was they were building trust first and then speed. This foundation of trust was what allowed them to fix more things and break fewer.
CURT NICKISCH: Trust sounds like a slow thing, right? If you talk about building trust, that is something that takes interactions, it takes communication, it takes experiences. Does that run counter to the speed idea?
ANNE MORRISS: Yeah. Well, this issue of trust is something we’ve been looking at for over a decade. One of the headlines in our research is it’s actually something we’re building and rebuilding and breaking all the time. And so instead of being this precious, almost farbege egg, it’s this thing that is constantly in motion and this thing that we can really impact when we’re deliberate about our choices and have some self-awareness around where it’s breaking down and how it’s breaking down.
CURT NICKISCH: You said break trust in there, which is intriguing, right? That you may have to break trust to build trust. Can you explain that a little?
ANNE MORRISS: Yeah, well, I’ll clarify. It’s not that you have to break it in order to build it. It’s just that we all do it some of the time. Most of us are trusted most of the time. Most of your listeners I imagine are trusted most of the time, but all of us have a pattern where we break trust or where we don’t build as much as could be possible.
CURT NICKISCH: I want to talk about speed, this other essential ingredient that’s so intriguing, right? Because you think about solving hard problems as something that just takes a lot of time and thinking and coordination and planning and designing. Explain what you mean by it? And also, just how we maybe approach problems wrong by taking them on too slowly?
ANNE MORRISS: Well, Curt, no one has ever said to us, “I wish I had taken longer and done less.” We hear the opposite all the time, by the way. So what we really set out to do was to create a playbook that anyone can use to take less time to do more of the things that are going to make your teams and organizations stronger.
And the way we set up the book is okay, it’s really a five step process. Speed is the last step. It’s the payoff for the hard work you’re going to do to figure out your problem, build or rebuild trust, expand the team in thoughtful and strategic ways, and then tell a real and compelling story about the change you’re leading.
Only then do you get to go fast, but that’s an essential part of the process, and we find that either people under emphasize it or speed has gotten a bad name in this world of moving fast and breaking things. And part of our mission for sure was to rehabilitate speed’s reputation because it is an essential part of the change leader’s equation. It can be the difference between good intentions and getting anything done at all.
CURT NICKISCH: You know, the fact that nobody ever tells you, “I wish we had done less and taken more time.” I think we all feel that, right? Sometimes we do something and then realize, “Oh, that wasn’t that hard and why did it take me so long to do it? And I wish I’d done this a long time ago.” Is it ever possible to solve a problem too quickly?
ANNE MORRISS: Absolutely. And we see that all the time too. What we push people to do in those scenarios is really take a look at the underlying issue because in most cases, the solution is not to take your foot off the accelerator per se and slow down. The solution is to get into the underlying problem. So if it’s burnout or a strategic disconnect between what you’re building and the marketplace you’re serving, what we find is the anxiety that people attach to speed or the frustration people attach to speed is often misplaced.
CURT NICKISCH: What is a good timeline to think about solving a problem then? Because if we by default take too long or else jump ahead and we don’t fix it right, what’s a good target time to have in your mind for how long solving a problem should take?
ANNE MORRISS: Yeah. Well, we’re playful in the book and talking about the idea that many problems can be solved in a week. We set the book up five chapters. They’re titled Monday, Tuesday, Wednesday, Thursday, Friday, and we’re definitely having fun with that. And yet, if you count the hours in a week, there are a lot of them. Many of our problems, if you were to spend a focused 40 hours of effort on a problem, you’re going to get pretty far.
But our main message is, listen, of course it’s going to depend on the nature of the problem, and you’re going to take weeks and maybe even some cases months to get to the other side. What we don’t want you to do is take years, which tends to be our default timeline for solving hard problems.
CURT NICKISCH: So you say to start with identifying the problem that’s holding you back, seems kind of obvious. But where do companies go right and wrong with this first step of just identifying the problem that’s holding you back?
ANNE MORRISS: And our goal is that all of these are going to feel obvious in retrospect. The problem is we skip over a lot of these steps and this is why we wanted to underline them. So this one is really rooted in our observation and I think the pattern of our species that we tend to be overconfident in the quality of our thoughts, particularly when it comes to diagnosing problems.
And so we want to invite you to start in a very humble and curious place, which tends not to be our default mode when we’re showing up for work. We convince ourselves that we’re being paid for our judgment. That’s exactly what gets reinforced everywhere. And so we tend to counterintuitively, given what we just talked about, we tend to move too quickly through the diagnostic phase.
CURT NICKISCH: “I know what to do, that’s why you hired me.”
ANNE MORRISS: Exactly. “I know what to do. That’s why you hired me. I’ve seen this before. I have a plan. Follow me.” We get rewarded for the expression of confidence and clarity. And so what we’re inviting people to do here is actually pause and really lean into what are the root causes of the problem you’re seeing? What are some alternative explanations? Let’s get into dialogue with the people who are also impacted by the problem before we start running down the path of solving it.
CURT NICKISCH: So what do you recommend for this step, for getting to the root of the problem? What are questions you should ask? What’s the right thought process? What do you do on Monday of the week?
ANNE MORRISS: In our experience of doing this work, people tend to undervalue the power of conversation, particularly with other people in the organization. So we will often advocate putting together a team of problem solvers, make it a temporary team, really pull in people who have a particular perspective on the problem and create the space, make it as psychologically safe as you can for people to really, as Chris Argyris so beautifully articulated, discuss the undiscussable.
And so the conditions for that are going to look different in every organization depending on the problem, but if you can get a space where smart people who have direct experience of a problem are in a room and talking honestly with each other, you can make an extraordinary amount of progress, certainly in a day.
CURT NICKISCH: Yeah, that gets back to the trust piece.
ANNE MORRISS: Definitely.
CURT NICKISCH: How do you like to start that meeting, or how do you like to talk about it? I’m just curious what somebody on that team might hear in that meeting, just to get the sense that it’s psychologically safe, you can discuss the undiscussable and you’re also focusing on the identification part. What’s key to communicate there?
ANNE MORRISS: Yeah. Well, we sometimes encourage people to do a little bit of data gathering before those conversations. So the power of a quick anonymous survey around whatever problem you’re solving, but also be really thoughtful about the questions you’re going to ask in the moment. So a little bit of preparation can go a long way and a little bit of thoughtfulness about the power dynamic. So who’s going to walk in there with license to speak and who’s going to hold back? So being thoughtful about the agenda, about the questions you’re asking about the room, about the facilitation, and then courage is a very infectious emotion.
So if you can early on create the conditions for people to show up bravely in that conversation, then the chance that you’re going to get good information and that you’re going to walk out of that room with new insight in the problem that you didn’t have when you walked in is extraordinarily high.
CURT NICKISCH: Now, in those discussions, you may have people who have different perspectives on what the problem really is. They also bear different costs of addressing the problem or solving it. You talked about the power dynamic, but there’s also an unfairness dynamic of who’s going to actually have to do the work to take care of it, and I wonder how you create a culture in that meeting where it’s the most productive?
ANNE MORRISS: For sure, the burden of work is not going to be equitably distributed around the room. But I would say, Curt, the dynamic that we see most often is that people are deeply relieved that hard problems are being addressed. So it really can create, and more often than not in our experience, it does create this beautiful flywheel of action, creativity, optimism. Often when problems haven’t been addressed, there is a fair amount of anxiety in the organization, frustration, stagnation. And so credible movement towards action and progress is often the best antidote. So even if the plan isn’t super clear yet, if it’s credible, given who’s in the room and their decision rights and mandate, if there’s real momentum coming out of that to make progress, then that tends to be deeply energizing to people.
CURT NICKISCH: I wonder if there’s an organization that you’ve worked with that you could talk about how this rolled out and how this took shape?
ANNE MORRISS: When we started working with Uber, that was wrestling with some very public issues of culture and trust with a range of stakeholders internally, the organization, also external, that work really started with a campaign of listening and really trying to understand where trust was breaking down from the perspective of these stakeholders?
So whether it was female employees or regulators or riders who had safety concerns getting into the car with a stranger. This work, it starts with an honest internal dialogue, but often the problem has threads that go external. And so bringing that same commitment to curiosity and humility and dialogue to anyone who’s impacted by the problem is the fastest way to surface what’s really going on.
CURT NICKISCH: There’s a step in this process that you lay out and that’s communicating powerfully as a leader. So we’ve heard about listening and trust building, but now you’re talking about powerful communication. How do you do this and why is it maybe this step in the process rather than the first thing you do or the last thing you do?
ANNE MORRISS: So in our process, again, it’s the days of the week. On Monday you figured out the problem. Tuesday you really got into the sandbox in figuring out what a good enough plan is for building trust. Wednesday, step three, you made it better. You created an even better plan, bringing in new perspectives. Thursday, this fourth step is the day we’re saying you got to go get buy-in. You got to bring other people along. And again, this is a step where we see people often underinvest in the power and payoff of really executing it well.
CURT NICKISCH: How does that go wrong?
ANNE MORRISS: Yeah, people don’t know the why. Human behavior and the change in human behavior really depends on a strong why. It’s not just a selfish, “What’s in it for me?” Although that’s helpful, but where are we going? I may be invested in a status quo and I need to understand, okay, if you’re going to ask me to change, if you’re going to invite me into this uncomfortable place of doing things differently, why am I here? Help me understand it and articulate the way forward and language that not only I can understand, but also that’s going to be motivating to me.
CURT NICKISCH: And who on my team was part of this process and all that kind of stuff?
ANNE MORRISS: Oh, yeah. I may have some really important questions that may be in the way of my buy-in and commitment to this plan. So certainly creating a space where those questions can be addressed is essential. But what we found is that there is an architecture of a great change story, and it starts with honoring the past, honoring the starting place. Sometimes we’re so excited about the change and animated about the change that what has happened before or what is even happening in the present tense is low on our list of priorities.
Or we want to label it bad, because that’s the way we’ve thought about the change, but really pausing and honoring what came before you and all the reasonable decisions that led up to it, I think can be really helpful to getting people emotionally where you want them to be willing to be guided by you. Going back to Uber, when Dara Khosrowshahi came in.
CURT NICKISCH: This is the new CEO.
ANNE MORRISS: The new CEO.
CURT NICKISCH: Replaced Travis Kalanick, the founder and first CEO, yeah.
ANNE MORRISS: Yeah, and had his first all-hands meeting. One of his key messages, and this is a quote, was that he was going to retain the edge that had made Uber, “A force of nature.” And in that meeting, the crowd went wild because this is also a company that had been beaten up publicly for months and months and months, and it was a really powerful choice. And his predecessor, Travis was in the room, and he also honored Travis’ incredible work and investment in bringing the company to the place where it was.
And I would use words like grace to also describe those choices, but there’s also an incredible strategic value to naming the starting place for everybody in the room because in most cases, most people in that room played a role in getting to that starting place, and you’re acknowledging that.
CURT NICKISCH: You can call it grace. Somebody else might call it diplomatic or strategic. But yeah, I guess like it or not, it’s helpful to call out and honor the complexity of the way things have been done and also the change that’s happening.
ANNE MORRISS: Yeah, and the value. Sometimes honoring the past is also owning what didn’t work or what wasn’t working for stakeholders or segments of the employee team, and we see that around culture change. Sometimes you’ve got to acknowledge that it was not an equitable environment, but whatever the worker, everyone in that room is bringing that pass with them. So again, making it discussable and using it as the jumping off place is where we advise people to start.
Then you’ve earned the right to talk about the change mandate, which we suggest using clear and compelling language about the why. “This is what happened, this is where we are, this is the good and the bad of it, and here’s the case for change.”
And then the last part, which is to describe a rigorous and optimistic way forward. It’s a simple past, present, future arc, which will be familiar to human beings. We love stories as human beings. It’s among the most powerful currency we have to make sense of the world.
CURT NICKISCH: Yeah. Chronological is a pretty powerful order.
ANNE MORRISS: Right. But again, the change leaders we see really get it right, are investing an incredible amount of time into the storytelling part of their job. Ursula Burns, the Head of Xerox is famous for the months and years she spent on the road just telling the story of Xerox’s change, its pivot into services to everyone who would listen, and that was a huge part of her success.
CURT NICKISCH: So Friday or your fifth step, you end with empowering teams and removing roadblocks. That seems obvious, but it’s critical. Can you dig into that a little bit?
ANNE MORRISS: Yeah. Friday is the fun day. Friday’s the release of energy into the system. Again, you’ve now earned the right to go fast. You have a plan, you’re pretty confident it’s going to work. You’ve told the story of change the organization, and now you get to sprint. So this is about really executing with urgency, and it’s about a lot of the tactics of speed is where we focus in the book. So the tactics of empowerment, making tough strategic trade-offs so that your priorities are clear and clearly communicated, creating mechanisms to fast-track progress. At Etsy, CEO Josh Silverman, he labeled these projects ambulances. It’s an unfortunate metaphor, but it’s super memorable. These are the products that get to speed out in front of the other ones because the stakes are high and the clock is sticking.
CURT NICKISCH: You pull over and let it go by.
ANNE MORRISS: Yeah, exactly. And so we have to agree as an organization on how to do something like that. And so we see lots of great examples both in young organizations and big complex biotech companies with lots of regulatory guardrails have still found ways to do this gracefully.
And I think we end with this idea of conflict debt, which is a term we really love. Leanne Davey, who’s a team scholar and researcher, and anyone in a tech company will recognize the idea of tech debt, which is this weight the organization drags around until they resolve it. Conflict debt is a beautiful metaphor because it is this weight that we drag around and slows us down until we decide to clean it up and fix it. The organizations that are really getting speed right have figured out either formally or informally, how to create an environment where conflict and disagreements can be gracefully resolved.
CURT NICKISCH: Well, let’s talk about this speed more, right? Because I think this is one of those places that maybe people go wrong or take too long, and then you lose the awareness of the problem, you lose that urgency. And then that also just makes it less effective, right? It’s not just about getting the problem solved as quickly as possible. It’s also just speed in some ways helps solve the problem.
ANNE MORRISS: Oh, yeah. It really is the difference between imagining the change you want to lead and really being able to bring it to life. Speed is the thing that unlocks your ability to lead change. It needs a foundation, and that’s what Monday through Thursday is all about, steps one through four, but the finish line is executing with urgency, and it’s that urgency that releases the system’s energy, that communicates your priorities, that creates the conditions for your team to make progress.
CURT NICKISCH: Moving fast is something that entrepreneurs and tech companies certainly understand, but there’s also this awareness that with big companies, the bigger the organization, the harder it is to turn the aircraft carrier around, right? Is speed relative when you get at those levels, or do you think this is something that any company should be able to apply equally?
ANNE MORRISS: We think this applies to any company. The culture really lives at the level of team. So we believe you can make a tremendous amount of progress even within your circle of control as a team leader. I want to bring some humility to this and careful of words like universal, but we do think there’s some universal truths here around the value of speed, and then some of the byproducts like keeping fantastic people. Your best people want to solve problems, they want to execute, they want to make progress and speed, and the ability to do that is going to be a variable in their own equation of whether they stay or they go somewhere else where they can have an impact.
CURT NICKISCH: Right. They want to accomplish something before they go or before they retire or finish something out. And if you’re able to just bring more things on the horizon and have it not feel like it’s going to be another two years to do something meaningful.
ANNE MORRISS: People – I mean, they want to make stuff happen and they want to be around the energy and the vitality of making things happen, which again, is also a super infectious phenomenon. One of the most important jobs of a leader, we believe, is to set the metabolic pace of their teams and organizations. And so what we really dig into on Friday is, well, what does that look like to speed something up? What are the tactics of that?
CURT NICKISCH: I wonder if that universal truth, that a body in motion stays in motion applies to organizations, right? If an organization in motion stays in motion, there is something to that.
ANNE MORRISS: Absolutely.
CURT NICKISCH: Do you have a favorite client story to share, just where you saw speed just become a bit of a flywheel or just a positive reinforcement loop for more positive change at the organization?
ANNE MORRISS: Yeah. We work with a fair number of organizations that are on fire. We do a fair amount of firefighting, but we also less dramatically do a lot of fire prevention. So we’re brought into organizations that are working well and want to get better, looking out on the horizon. That work is super gratifying, and there is always a component of, well, how do we speed this up?
What I love about that work is there’s often already a high foundation of trust, and so it’s, well, how do we maintain that foundation but move this flywheel, as you said, even faster? And it’s really energizing because often there’s a lot of pent-up energy that… There’s a lot of loyalty to the organization, but often it’s also frustration and pent-up energy. And so when that gets released, when good people get the opportunity to sprint for the first time in a little while, it’s incredibly energizing, not just for us, but for the whole organization.
CURT NICKISCH: Anne, this is great. I think finding a way to solve problems better but also faster is going to be really helpful. So thanks for coming on the show to talk about it.
ANNE MORRISS: Oh, Curt, it was such a pleasure. This is my favorite conversation. I’m delighted to have it anytime.
HANNAH BATES: That was entrepreneur, leadership coach, and author Anne Morriss – in conversation with Curt Nickisch on HBR IdeaCast.
We’ll be back next Wednesday with another hand-picked conversation about business strategy from Harvard Business Review. If you found this episode helpful, share it with your friends and colleagues, and follow our show on Apple Podcasts, Spotify, or wherever you get your podcasts. While you’re there, be sure to leave us a review.
When you’re ready for more podcasts, articles, case studies, books, and videos with the world’s top business and management experts, you’ll find it all at HBR.org.
This episode was produced by Mary Dooe, Anne Saini, and me, Hannah Bates. Ian Fox is our editor. Special thanks to Rob Eckhardt, Maureen Hoch, Erica Truxler, Ramsey Khabbaz, Nicole Smith, Anne Bartholomew, and you – our listener. See you next week.
- Subscribe On:
Latest in this series
This article is about strategy.
- Decision making and problem solving
- Strategy execution
- Leadership and managing people
- Collaboration and teams
- Trustworthiness
- Organizational culture
Partner Center
- USC Libraries
- Research Guides
Organizing Your Social Sciences Research Paper
- The Research Problem/Question
- Purpose of Guide
- Design Flaws to Avoid
- Independent and Dependent Variables
- Glossary of Research Terms
- Reading Research Effectively
- Narrowing a Topic Idea
- Broadening a Topic Idea
- Extending the Timeliness of a Topic Idea
- Academic Writing Style
- Applying Critical Thinking
- Choosing a Title
- Making an Outline
- Paragraph Development
- Research Process Video Series
- Executive Summary
- The C.A.R.S. Model
- Background Information
- Theoretical Framework
- Citation Tracking
- Content Alert Services
- Evaluating Sources
- Primary Sources
- Secondary Sources
- Tiertiary Sources
- Scholarly vs. Popular Publications
- Qualitative Methods
- Quantitative Methods
- Insiderness
- Using Non-Textual Elements
- Limitations of the Study
- Common Grammar Mistakes
- Writing Concisely
- Avoiding Plagiarism
- Footnotes or Endnotes?
- Further Readings
- Generative AI and Writing
- USC Libraries Tutorials and Other Guides
- Bibliography
A research problem is a definite or clear expression [statement] about an area of concern, a condition to be improved upon, a difficulty to be eliminated, or a troubling question that exists in scholarly literature, in theory, or within existing practice that points to a need for meaningful understanding and deliberate investigation. A research problem does not state how to do something, offer a vague or broad proposition, or present a value question. In the social and behavioral sciences, studies are most often framed around examining a problem that needs to be understood and resolved in order to improve society and the human condition.
Bryman, Alan. “The Research Question in Social Research: What is its Role?” International Journal of Social Research Methodology 10 (2007): 5-20; Guba, Egon G., and Yvonna S. Lincoln. “Competing Paradigms in Qualitative Research.” In Handbook of Qualitative Research . Norman K. Denzin and Yvonna S. Lincoln, editors. (Thousand Oaks, CA: Sage, 1994), pp. 105-117; Pardede, Parlindungan. “Identifying and Formulating the Research Problem." Research in ELT: Module 4 (October 2018): 1-13; Li, Yanmei, and Sumei Zhang. "Identifying the Research Problem." In Applied Research Methods in Urban and Regional Planning . (Cham, Switzerland: Springer International Publishing, 2022), pp. 13-21.
Importance of...
The purpose of a problem statement is to:
- Introduce the reader to the importance of the topic being studied . The reader is oriented to the significance of the study.
- Anchors the research questions, hypotheses, or assumptions to follow . It offers a concise statement about the purpose of your paper.
- Place the topic into a particular context that defines the parameters of what is to be investigated.
- Provide the framework for reporting the results and indicates what is probably necessary to conduct the study and explain how the findings will present this information.
In the social sciences, the research problem establishes the means by which you must answer the "So What?" question. This declarative question refers to a research problem surviving the relevancy test [the quality of a measurement procedure that provides repeatability and accuracy]. Note that answering the "So What?" question requires a commitment on your part to not only show that you have reviewed the literature, but that you have thoroughly considered the significance of the research problem and its implications applied to creating new knowledge and understanding or informing practice.
To survive the "So What" question, problem statements should possess the following attributes:
- Clarity and precision [a well-written statement does not make sweeping generalizations and irresponsible pronouncements; it also does include unspecific determinates like "very" or "giant"],
- Demonstrate a researchable topic or issue [i.e., feasibility of conducting the study is based upon access to information that can be effectively acquired, gathered, interpreted, synthesized, and understood],
- Identification of what would be studied, while avoiding the use of value-laden words and terms,
- Identification of an overarching question or small set of questions accompanied by key factors or variables,
- Identification of key concepts and terms,
- Articulation of the study's conceptual boundaries or parameters or limitations,
- Some generalizability in regards to applicability and bringing results into general use,
- Conveyance of the study's importance, benefits, and justification [i.e., regardless of the type of research, it is important to demonstrate that the research is not trivial],
- Does not have unnecessary jargon or overly complex sentence constructions; and,
- Conveyance of more than the mere gathering of descriptive data providing only a snapshot of the issue or phenomenon under investigation.
Bryman, Alan. “The Research Question in Social Research: What is its Role?” International Journal of Social Research Methodology 10 (2007): 5-20; Brown, Perry J., Allen Dyer, and Ross S. Whaley. "Recreation Research—So What?" Journal of Leisure Research 5 (1973): 16-24; Castellanos, Susie. Critical Writing and Thinking. The Writing Center. Dean of the College. Brown University; Ellis, Timothy J. and Yair Levy Nova. "Framework of Problem-Based Research: A Guide for Novice Researchers on the Development of a Research-Worthy Problem." Informing Science: the International Journal of an Emerging Transdiscipline 11 (2008); Thesis and Purpose Statements. The Writer’s Handbook. Writing Center. University of Wisconsin, Madison; Thesis Statements. The Writing Center. University of North Carolina; Tips and Examples for Writing Thesis Statements. The Writing Lab and The OWL. Purdue University; Selwyn, Neil. "‘So What?’…A Question that Every Journal Article Needs to Answer." Learning, Media, and Technology 39 (2014): 1-5; Shoket, Mohd. "Research Problem: Identification and Formulation." International Journal of Research 1 (May 2014): 512-518.
Structure and Writing Style
I. Types and Content
There are four general conceptualizations of a research problem in the social sciences:
- Casuist Research Problem -- this type of problem relates to the determination of right and wrong in questions of conduct or conscience by analyzing moral dilemmas through the application of general rules and the careful distinction of special cases.
- Difference Research Problem -- typically asks the question, “Is there a difference between two or more groups or treatments?” This type of problem statement is used when the researcher compares or contrasts two or more phenomena. This a common approach to defining a problem in the clinical social sciences or behavioral sciences.
- Descriptive Research Problem -- typically asks the question, "what is...?" with the underlying purpose to describe the significance of a situation, state, or existence of a specific phenomenon. This problem is often associated with revealing hidden or understudied issues.
- Relational Research Problem -- suggests a relationship of some sort between two or more variables to be investigated. The underlying purpose is to investigate specific qualities or characteristics that may be connected in some way.
A problem statement in the social sciences should contain :
- A lead-in that helps ensure the reader will maintain interest over the study,
- A declaration of originality [e.g., mentioning a knowledge void or a lack of clarity about a topic that will be revealed in the literature review of prior research],
- An indication of the central focus of the study [establishing the boundaries of analysis], and
- An explanation of the study's significance or the benefits to be derived from investigating the research problem.
NOTE : A statement describing the research problem of your paper should not be viewed as a thesis statement that you may be familiar with from high school. Given the content listed above, a description of the research problem is usually a short paragraph in length.
II. Sources of Problems for Investigation
The identification of a problem to study can be challenging, not because there's a lack of issues that could be investigated, but due to the challenge of formulating an academically relevant and researchable problem which is unique and does not simply duplicate the work of others. To facilitate how you might select a problem from which to build a research study, consider these sources of inspiration:
Deductions from Theory This relates to deductions made from social philosophy or generalizations embodied in life and in society that the researcher is familiar with. These deductions from human behavior are then placed within an empirical frame of reference through research. From a theory, the researcher can formulate a research problem or hypothesis stating the expected findings in certain empirical situations. The research asks the question: “What relationship between variables will be observed if theory aptly summarizes the state of affairs?” One can then design and carry out a systematic investigation to assess whether empirical data confirm or reject the hypothesis, and hence, the theory.
Interdisciplinary Perspectives Identifying a problem that forms the basis for a research study can come from academic movements and scholarship originating in disciplines outside of your primary area of study. This can be an intellectually stimulating exercise. A review of pertinent literature should include examining research from related disciplines that can reveal new avenues of exploration and analysis. An interdisciplinary approach to selecting a research problem offers an opportunity to construct a more comprehensive understanding of a very complex issue that any single discipline may be able to provide.
Interviewing Practitioners The identification of research problems about particular topics can arise from formal interviews or informal discussions with practitioners who provide insight into new directions for future research and how to make research findings more relevant to practice. Discussions with experts in the field, such as, teachers, social workers, health care providers, lawyers, business leaders, etc., offers the chance to identify practical, “real world” problems that may be understudied or ignored within academic circles. This approach also provides some practical knowledge which may help in the process of designing and conducting your study.
Personal Experience Don't undervalue your everyday experiences or encounters as worthwhile problems for investigation. Think critically about your own experiences and/or frustrations with an issue facing society or related to your community, your neighborhood, your family, or your personal life. This can be derived, for example, from deliberate observations of certain relationships for which there is no clear explanation or witnessing an event that appears harmful to a person or group or that is out of the ordinary.
Relevant Literature The selection of a research problem can be derived from a thorough review of pertinent research associated with your overall area of interest. This may reveal where gaps exist in understanding a topic or where an issue has been understudied. Research may be conducted to: 1) fill such gaps in knowledge; 2) evaluate if the methodologies employed in prior studies can be adapted to solve other problems; or, 3) determine if a similar study could be conducted in a different subject area or applied in a different context or to different study sample [i.e., different setting or different group of people]. Also, authors frequently conclude their studies by noting implications for further research; read the conclusion of pertinent studies because statements about further research can be a valuable source for identifying new problems to investigate. The fact that a researcher has identified a topic worthy of further exploration validates the fact it is worth pursuing.
III. What Makes a Good Research Statement?
A good problem statement begins by introducing the broad area in which your research is centered, gradually leading the reader to the more specific issues you are investigating. The statement need not be lengthy, but a good research problem should incorporate the following features:
1. Compelling Topic The problem chosen should be one that motivates you to address it but simple curiosity is not a good enough reason to pursue a research study because this does not indicate significance. The problem that you choose to explore must be important to you, but it must also be viewed as important by your readers and to a the larger academic and/or social community that could be impacted by the results of your study. 2. Supports Multiple Perspectives The problem must be phrased in a way that avoids dichotomies and instead supports the generation and exploration of multiple perspectives. A general rule of thumb in the social sciences is that a good research problem is one that would generate a variety of viewpoints from a composite audience made up of reasonable people. 3. Researchability This isn't a real word but it represents an important aspect of creating a good research statement. It seems a bit obvious, but you don't want to find yourself in the midst of investigating a complex research project and realize that you don't have enough prior research to draw from for your analysis. There's nothing inherently wrong with original research, but you must choose research problems that can be supported, in some way, by the resources available to you. If you are not sure if something is researchable, don't assume that it isn't if you don't find information right away--seek help from a librarian !
NOTE: Do not confuse a research problem with a research topic. A topic is something to read and obtain information about, whereas a problem is something to be solved or framed as a question raised for inquiry, consideration, or solution, or explained as a source of perplexity, distress, or vexation. In short, a research topic is something to be understood; a research problem is something that needs to be investigated.
IV. Asking Analytical Questions about the Research Problem
Research problems in the social and behavioral sciences are often analyzed around critical questions that must be investigated. These questions can be explicitly listed in the introduction [i.e., "This study addresses three research questions about women's psychological recovery from domestic abuse in multi-generational home settings..."], or, the questions are implied in the text as specific areas of study related to the research problem. Explicitly listing your research questions at the end of your introduction can help in designing a clear roadmap of what you plan to address in your study, whereas, implicitly integrating them into the text of the introduction allows you to create a more compelling narrative around the key issues under investigation. Either approach is appropriate.
The number of questions you attempt to address should be based on the complexity of the problem you are investigating and what areas of inquiry you find most critical to study. Practical considerations, such as, the length of the paper you are writing or the availability of resources to analyze the issue can also factor in how many questions to ask. In general, however, there should be no more than four research questions underpinning a single research problem.
Given this, well-developed analytical questions can focus on any of the following:
- Highlights a genuine dilemma, area of ambiguity, or point of confusion about a topic open to interpretation by your readers;
- Yields an answer that is unexpected and not obvious rather than inevitable and self-evident;
- Provokes meaningful thought or discussion;
- Raises the visibility of the key ideas or concepts that may be understudied or hidden;
- Suggests the need for complex analysis or argument rather than a basic description or summary; and,
- Offers a specific path of inquiry that avoids eliciting generalizations about the problem.
NOTE: Questions of how and why concerning a research problem often require more analysis than questions about who, what, where, and when. You should still ask yourself these latter questions, however. Thinking introspectively about the who, what, where, and when of a research problem can help ensure that you have thoroughly considered all aspects of the problem under investigation and helps define the scope of the study in relation to the problem.
V. Mistakes to Avoid
Beware of circular reasoning! Do not state the research problem as simply the absence of the thing you are suggesting. For example, if you propose the following, "The problem in this community is that there is no hospital," this only leads to a research problem where:
- The need is for a hospital
- The objective is to create a hospital
- The method is to plan for building a hospital, and
- The evaluation is to measure if there is a hospital or not.
This is an example of a research problem that fails the "So What?" test . In this example, the problem does not reveal the relevance of why you are investigating the fact there is no hospital in the community [e.g., perhaps there's a hospital in the community ten miles away]; it does not elucidate the significance of why one should study the fact there is no hospital in the community [e.g., that hospital in the community ten miles away has no emergency room]; the research problem does not offer an intellectual pathway towards adding new knowledge or clarifying prior knowledge [e.g., the county in which there is no hospital already conducted a study about the need for a hospital, but it was conducted ten years ago]; and, the problem does not offer meaningful outcomes that lead to recommendations that can be generalized for other situations or that could suggest areas for further research [e.g., the challenges of building a new hospital serves as a case study for other communities].
Alvesson, Mats and Jörgen Sandberg. “Generating Research Questions Through Problematization.” Academy of Management Review 36 (April 2011): 247-271 ; Choosing and Refining Topics. Writing@CSU. Colorado State University; D'Souza, Victor S. "Use of Induction and Deduction in Research in Social Sciences: An Illustration." Journal of the Indian Law Institute 24 (1982): 655-661; Ellis, Timothy J. and Yair Levy Nova. "Framework of Problem-Based Research: A Guide for Novice Researchers on the Development of a Research-Worthy Problem." Informing Science: the International Journal of an Emerging Transdiscipline 11 (2008); How to Write a Research Question. The Writing Center. George Mason University; Invention: Developing a Thesis Statement. The Reading/Writing Center. Hunter College; Problem Statements PowerPoint Presentation. The Writing Lab and The OWL. Purdue University; Procter, Margaret. Using Thesis Statements. University College Writing Centre. University of Toronto; Shoket, Mohd. "Research Problem: Identification and Formulation." International Journal of Research 1 (May 2014): 512-518; Trochim, William M.K. Problem Formulation. Research Methods Knowledge Base. 2006; Thesis and Purpose Statements. The Writer’s Handbook. Writing Center. University of Wisconsin, Madison; Thesis Statements. The Writing Center. University of North Carolina; Tips and Examples for Writing Thesis Statements. The Writing Lab and The OWL. Purdue University; Pardede, Parlindungan. “Identifying and Formulating the Research Problem." Research in ELT: Module 4 (October 2018): 1-13; Walk, Kerry. Asking an Analytical Question. [Class handout or worksheet]. Princeton University; White, Patrick. Developing Research Questions: A Guide for Social Scientists . New York: Palgrave McMillan, 2009; Li, Yanmei, and Sumei Zhang. "Identifying the Research Problem." In Applied Research Methods in Urban and Regional Planning . (Cham, Switzerland: Springer International Publishing, 2022), pp. 13-21.
- << Previous: Background Information
- Next: Theoretical Framework >>
- Last Updated: May 22, 2024 12:03 PM
- URL: https://libguides.usc.edu/writingguide
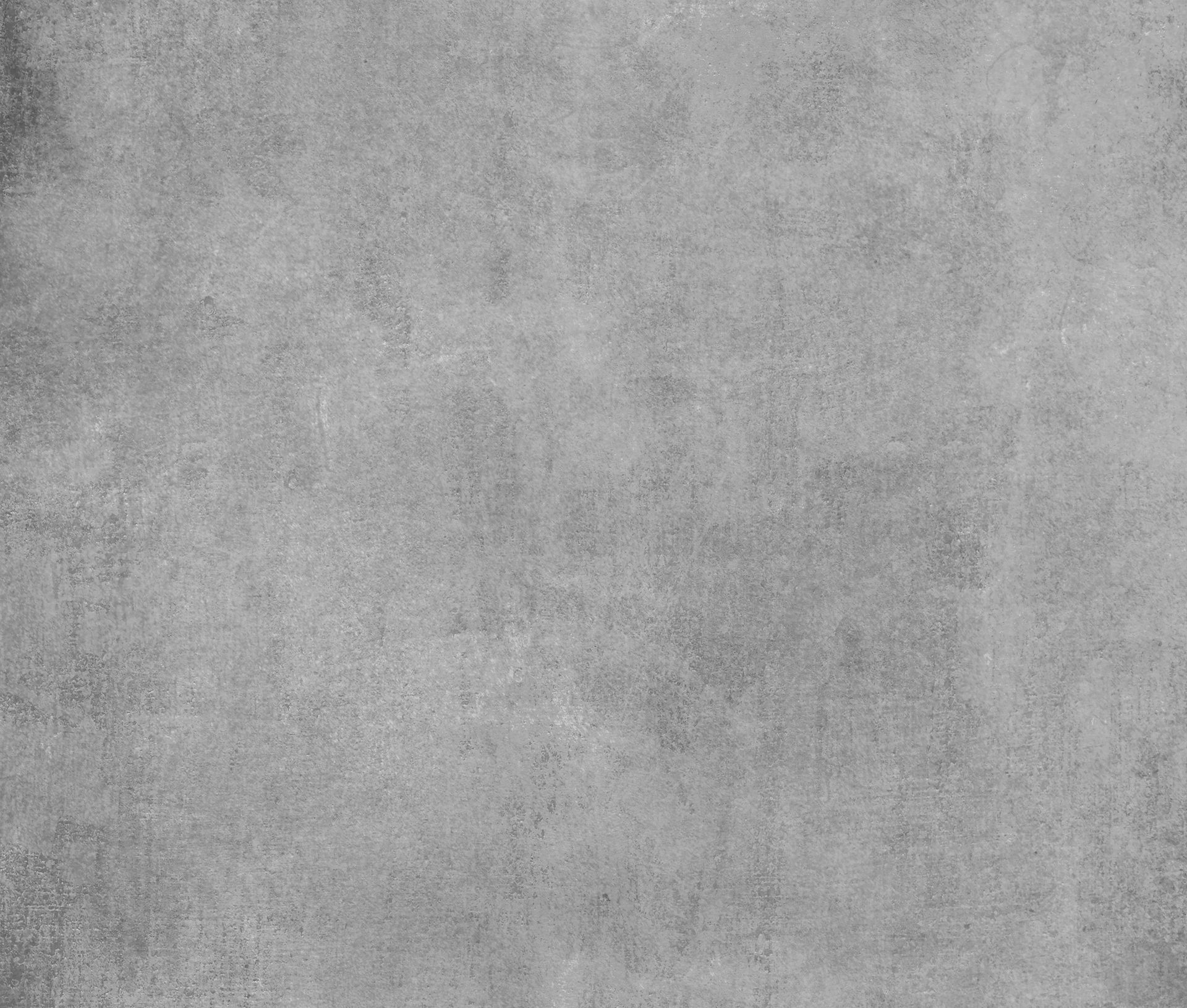
- Spencer Greenberg
- 14 min read
Problem-Solving Techniques That Work For All Types of Challenges
Essay by Spencer Greenberg, Clearer Thinking founder
A lot of people don’t realize that there are general purpose problem solving techniques that cut across domains. They can help you deal with thorny challenges in work, your personal life, startups, or even if you’re trying to prove a new theorem in math.
Below are the 26 general purpose problem solving techniques that I like best, along with a one-word name I picked for each, and hypothetical examples to illustrate what sort of strategy I’m referring to.
Consider opening up this list whenever you’re stuck solving a challenging problem. It’s likely that one or more of these techniques can help!

1. Clarifying
Try to define the problem you are facing as precisely as you can, maybe by writing down a detailed description of exactly what the problem is and what constraints exist for a solution, or by describing it in detail to another person. This may lead to you realizing the problem is not quite what you had thought, or that it has a more obvious solution than you thought.
Life Example
“I thought that I needed to find a new job, but when I thought really carefully about what I don’t like about my current job, I realized that I could likely fix those things by talking to my boss or even, potentially, just by thinking about them differently.”
Startup Example
“we thought we had a problem with users not wanting to sign up for the product, but when we carefully investigated what the problem really was, we discovered it was actually more of a problem of users wanting the product but then growing frustrated because of bad interface design.”
2. Subdividing
Break the problem down into smaller problems in such a way that if you solve each of the small problems, you will have solved the entire problem. Once a problem is subdivided it can also sometimes be parallelized (e.g., by involving different people to work on the different components).
“My goal is to get company Z to become a partner with my company, and that seems hard, so let me break that goal into the steps of (a) listing the ways that company Z would benefit from becoming a partner with us, (b) finding an employee at company Z who would be responsive to hearing about these benefits, and (c) tracking down someone who can introduce me to that employee.”
Math Example
“I want to prove that a certain property applies to all functions of a specific type, so I start by (a) showing that every function of that type can be written as a sum of a more specific type of function, then I show that (b) the property applies to each function of the more specific type, and finally I show that (c) if the property applies to each function in a set of functions then it applies to arbitrary sums of those functions as well.”
3. Simplifying
Think of the simplest variation of the problem that you expect you can solve that shares important features in common with your problem, and see if solving this simpler problem gives you ideas for how to solve the more difficult version.
“I don’t know how to hire a CTO, but I do know how to hire a software engineer because I’ve done it many times, and good CTOs will often themselves be good software engineers, so how can I tweak my software engineer hiring to make it appropriate for hiring a CTO?”
“I don’t know how to calculate this integral as it is, but if I remove one of the free parameters, I actually do know how to calculate it, and maybe doing that calculation will give me insight into the solution of the more complex integral.”
4. Crowd-sourcing
Use suggestions from multiple people to gain insight into how to solve the problem, for instance by posting on Facebook or Twitter requesting people’s help, or by posting to a Q&A site like Quora, or by sending emails to 10 people you know explaining the problem and requesting assistance.
Business Example
“Do you have experience outsourcing manufacturing to China? If so, I’d appreciate hearing your thoughts about how to approach choosing a vendor.”
Health Example
“I have trouble getting myself to stick to doing exercise daily. If you also used to have trouble getting yourself to exercise but don’t anymore, I’d love to know what worked to make it easier for you.”
5. Splintering
If the problem you are trying to solve has special cases that a solution to the general problem would also apply to, consider just one or two of these special cases as examples and solve the problem just for those cases first. Then see if a solution to one of those special cases helps you solve the problem in general.
“I want to figure out how to improve employee retention in general, let me examine how I could have improved retention in the case of the last three people that quit.”
“I want to figure out how to convince a large number of people to become customers, let me first figure out how to convince just Bill and John to become customers since they seem like the sort of customer I want to attract, and see what general lessons I learn from doing that.”
Read the books or textbooks that seem most related to the topic, and see whether they provide a solution to the problem, or teach you enough related information that you can now solve it yourself.
Economics Example
“Economists probably have already figured out reasonable ways to estimate demand elasticity, let’s see what an econometrics textbook says rather than trying to invent a technique from scratch.”
Mental Health Example
“I’ve been feeling depressed for a long time, maybe I should read some well-liked books about depression.”
7. Searching
Think of a similar problem that you think practitioners, bloggers or academics might have already solved and search online (e.g., via google, Q&A sites, or google scholar academic paper search) to see if anyone has done a write-up about how they solved it.
Advertising Example
“I’m having trouble figuring out the right advertising keywords to bid on for my specific product, I bet someone has a blog post describing how to approach choosing keywords for other related products.”
Machine Learning Example
“I can’t get this neural network to train properly in my specific case, I wonder if someone has written a tutorial about how to apply neural networks to related problems.”
8. Unconstraining
List all the constraints of the problem, then temporarily ignore one or more of the constraints that make the problem especially hard, and try to solve it without those constraints. If you can, then see if you can modify that unconstrained solution until it becomes a solution for the fully constrained problem.
“I need to hire someone who can do work at the intersection of machine learning and cryptography, let me drop the constraint of having cryptography experience and recruit machine learning people, then pick from among them a person that seems both generally capable and well positioned to learn the necessary cryptography.”
Computer Science Example
“I need to implement a certain algorithm, and it needs to be efficient, but that seems very difficult, so let me first figure out how to implement an inefficient version of the algorithm (i.e., drop the efficiency constraint), then at the end I will try to figure out how to optimize that algorithm for efficiency.”
9. Distracting
Fill your mind with everything you know about the problem, including facts, constraints, challenges, considerations, etc. and then stop thinking about the problem, and go and do a relaxing activity that requires little focus, such as walking, swimming, cooking, napping or taking a bath to see if new ideas or potential solutions pop into your mind unexpectedly as your subconscious continues to work on the problem without your attention.
“For three days, I’ve been trying to solve this problem at work, but the solution only came to me when I was strolling in the woods and not even thinking about it.”
Example from mathematician Henri Poincaré
“The incidents of the travel made me forget my mathematical work. Having reached Coutances, we entered an omnibus to go someplace or other. At the moment when I put my foot on the step, the idea came to me, without anything in my former thoughts seeming to have paved the way for it, that the transformations I had used to define the Fuchsian functions were identical with those of non-Euclidean geometry.”
10. Reexamining
Write down all the assumptions you’ve been making about the problem or about what a solution should I look like (yes – make an actual list). Then start challenging them one by one to see if they are actually needed or whether some may be unnecessary or mistaken.
Psychology Example
“We were assuming in our lab experiments that when people get angry they have some underlying reason behind it, but there may be some anger that is better modeled as a chemical fluctuation that is only loosely related to what happens in the lab, such as when people are quick to anger because they are hungry.”
“I need to construct a function that has this strange property, and so far I’ve assumed that the function must be smooth, but if it doesn’t actually need to be then perhaps I can construct just such a function out of simple linear pieces that are glued together.”
11. Reframing
Try to see the problem differently. For instance, by flipping the default, analyzing the inverse of the problem instead, thinking about how you would achieve the opposite of what you want, or shifting to an opposing perspective.
If we were building this company over again completely from scratch, what would we do differently in the design of our product, and can we pivot the product in that direction right now?”
“Should move to New York to take a job that pays $20,000 more per year? Well, if I already lived in New York, the decision to stay there rather than taking a $20,000 pay cut to move here would be an easy one. So maybe I’m overly focused on the current default of not being in New York and the short term unpleasantness of relocating.”
Marketing Example
“If I were one of our typical potential customers, what would I do to try to find a product like ours?”
12. Brainstorming
Set a timer for at least 5 minutes, and generate as many plausible solutions or ideas that you can without worrying about quality at all. Evaluate the ideas only at the end after the timer goes off.
“I’m going to set a timer for 5 minutes and come up with at least three new ways I could go about looking for a co-founder.”
“I’m going to set a timer for 20 minutes and come up with at least five possible explanations for why I’ve been feeling so anxious lately.”
13. Experting
Find an expert (or someone highly knowledgeable) in the topic area and ask their opinion about the best way to solve the problem.
“Why do you think most attempts at creating digital medical records failed, and what would someone have to do differently to have a reasonable chance at success?”
“What sort of optimization algorithm would be most efficient for minimizing the objective functions of this type?”
14. Eggheading
Ask the smartest person you know how they would solve the problem. Be sure to send an email in advance, describing the details so that this person has time to deeply consider the problem before you discuss it.
“Given the information I sent you about our competitors and the interviews we’ve done with potential customers, in which direction would you pivot our product if you were me (and why)?”
Research Example
“Given the information I sent you about our goals and the fact that our previous research attempts have gotten nowhere, how would you approach researching this topic to find the answer we need?”
15. Guessing
Start with a guess for what the solution could be, now check if it actually works and if not, start tweaking that guess to see if you can morph it into something that could work.
“I don’t know what price to use for the product we’re selling, so let me start with an initial guess and then begin trying to sell the thing, and tweak the price down if it seems to be a sticking point for customers, and tweak the price up if the customers don’t seem to pay much attention to the price.”
“My off the cuff intuition says that this differential equation might have a solution of the form x^a * e^(b x)for some a or b, let me plug it into the equation to see if indeed it satisfies the equation for any choice of a and b, and if not, let me see if I can tweak it to make something similar work.”
“I don’t know what the most effective diet for me would be, so I’ll just use my intuition to ban from my diet some foods that seem both unhealthy and addictive, and see if that helps.”
16. Comparing
Think of similar domains you already understand or similar problems you have already solved in the past, and see whether your knowledge of those domains or solutions to those similar problems may work as a complete or partial solution here.
“I don’t know how to find someone to fix things in my apartment, but I have found a good house cleaner before by asking a few friends who they use, so maybe I can simply use the same approach for finding a person to fix things.”
“This equation I’m trying to simplify reminds me of work I’m familiar with related to Kullback-Leibler divergence, I wonder if results from information theory could be applied in this case.”
17. Outsourcing
Consider whether you can hire someone to solve this problem, instead of figuring out how to solve it yourself.
“I don’t really understand how to get media attention for my company, so let me hire a public relations firm and let them handle the process.”
“I have no fashion sense, but I’d like to look better. Maybe I should hire someone fashionable who works in apparel to go shopping with me and help me choose what I should wear.”
18. Experimenting
Rapidly develop possible solutions and test them out (in sequence, or in parallel) by applying cheap and fast experiments. Discard those that don’t work, or iterate on them to improve them based on what you learn from the experiments.
“We don’t know if people will like a product like the one we have in mind, but we can put together a functioning prototype quickly, show five people that seem like they could be potential users, and iterate or create an entirely new design based on how they respond.”
“I don’t know if cutting out sugar will help improve my energy levels, but I can try it for two weeks and see if I notice any differences.”
19. Generalizing
Consider the more general case of the specific problem you are trying to solve, and then work on solving the general version instead. Paradoxically, it is sometimes easier to make progress on the general case rather than a specific one because it increases your focus on the structure of the problem rather than unimportant details.
“I want to figure out how to get this particular key employee more motivated to do good work, let me construct a model of what makes employees motivated to do good work in general, then I’ll apply it to this case.”
“I want to solve this specific differential equation, but it’s clearly a special case of a more general class of differential equations, let me study the general class and see what I can learn about them first and then apply what I learn to the specific case.”
20. Approximating
Consider whether a partial or approximate solution would be acceptable and, if so, aim for that instead of a full or exact solution.
“Our goal is to figure out which truck to send out for which delivery, which theoretically depends on many factors such as current location, traffic conditions, truck capacity, fuel efficiency, how many hours the driver has been on duty, the number of people manning each truck, the hourly rate we pay each driver, etc. etc. Maybe if we focus on just the three variables that we think are most important, we can find a good enough solution.”
“Finding a solution to this equation seems difficult, but if I approximate one of the terms linearly it becomes much easier, and maybe for the range of values we’re interested in, that’s close enough to an exact solution!”
21. Annihilating
Try to prove that the problem you are attempting to solve is actually impossible. If you succeed, you may save yourself a lot of time working on something impossible. Furthermore, in attempting to prove that the problem is impossible, you may gain insight into what makes it actually possible to solve, or if it turns out to truly be impossible, figure out how you could tweak the problem to make it solvable.
“I’m struggling to find a design for a theoretical voting system that has properties X, Y, and Z, let me see if I can instead prove that no such voting system with these three properties could possibly exist.”
“My goal has been to prove that this property always applies to this class of functions, let me see if I can generate a counterexample to prove that this goal is actually impossible.”
Physics Example
“I was trying to design a physical system with certain properties, but I now realize that if such a system could be realized, then it would allow for perpetual motion, and therefore it is impossible to build the sort of system I had in mind.”
22. Modeling
Try to build an explicit model of the situation, including what elements there are and how they related to each other. For instance, try drawing a diagram or flow chart that encapsulates your understanding of all the important information that relates to the problem.
“I’ve noticed that there are certain situations that cause me to freak out that would not bother other people. So what are the common elements when this happens, and how do they seem to relate to each other and to the way I end up feeling? Let me see if I can draw a diagram of this on paper.”
“What are all the different groups (e.g., providers, payers, patients) involved in the healthcare system, and if we diagram how they interact with each other, will that give us ideas for how we can sell our healthcare product?”
23. Brute forcing
One-by-one, consider every possible solution to the problem until you’ve found a good one or exhausted them all.
Startup example
“We’re not sure the order that these four parts of the user registration process should go in, so let’s make a list of all 24 possible orderings, and examine them one by one to see which makes the most sense.”
“It’s not clear how to pick which of these machine learning methods to use on this problem, but since we have lots of data, we can just try each of the algorithms and see which makes the most accurate predictions on data we’ve held to the side for testing.”
24. Refocusing
Forget about trying to solve the problem, and instead consider why you are trying to solve it. Then consider if there is a different problem you can work on that is aimed at producing the same sort of value in a different way.
Startup Example 1
“Maybe instead of trying increasingly hard to figure out how to get this type of consumer to buy, we need to switch our focus to the problem of how to sell to businesses, since what we actually care about is selling it, not selling it to one particular group.”
Startup Example 2
“I’ve been banging my head against the wall trying to implement this extremely complex feature, but there are lots of features that users would find just as valuable that are much easier to implement, maybe I should focus on those instead.”
25. Sidestepping
Consider whether you really want to spend more time trying to solve this problem and whether you can avoid the problem by instead working on totally different problems that you also care about.
“We’ve tried selling our solution to replace Excel for 12 months without much success, maybe we should go back to the drawing board and consider designing a totally new product. Our assumptions about customer needs seem to simply have been wrong.”
“I’ve spent six months on this math problem with little progress, but there are two other math problems I’m equally excited about, so maybe I should spend some time investigating whether one of those may be more tractable.”
26. Aggregating
Consider whether multiple problems you’re now experiencing might, in fact, be caused by the same source of difficulty, rather than being independent problems.
“I seem to be having conflict with a few different friends right now – could it be that I’m doing something without realizing it that is increasing my chance of conflict with all of them?”
“Three employees have quit in the last month. Perhaps the primary problem isn’t really about convincing this one important employee to stay, which is how I was framing it, but rather, about identifying why people keep leaving more generally.”
Recent Posts
We're launching a scientific test of astrology, and anyone can participate!
Remembering Daniel Kahneman: 7 theories that can help you understand how you think
Test how well you understand human psychology with our new quiz

IMAGES
VIDEO
COMMENTS
Abstract. The paper aims to introduce the conceptual framework of problem solving through values. The framework consists of problem analysis, selection of value (s) as a background for the solution, the search for alternative ways of the solution, and the rationale for the solution. This framework reveals when, how, and why is important to ...
Collaborative problem-solving has been widely embraced in the classroom instruction of critical thinking, which is regarded as the core of curriculum reform based on key competencies in the field ...
Problem-based learning (PBL) has been widely adopted in diverse fields and educational contexts to promote critical thinking and problem-solving in authentic learning situations. Its close affiliation with workplace collaboration and interdisciplinary learning contributed to its spread beyond the traditional realm of clinical education 1 to ...
Problem-solving ability is an essential part of daily life. Thus, curiosity and a thirst for knowledge should be cultivated in students to help them develop problem solving and independent thinking skills. Along with positive attitudes and an active disposition, these abilities are needed to solve problems throughout the lifespan and develop ...
Solving or attempting to solve problems is the typical and, hence, general function of thought. A theory of problem solving must first explain how the problem is constituted, and then how the solution happens, but also how it happens that it is not solved; it must explain the correct answer and with the same means the failure. The identification of the way in which the problem is formatted ...
Problem solving (PS), a component of critical thinking (Chaisri et al., 2019; Kuo et al., 2020), is a form of human intelligence that uses a structural phase to find an unknown or developing answer (Jones-Harris & Chamblee, 2017; Polya, 1981); PS organizes thoughts and processes to find a solution.Problem solving is a human skill that is required to deal with the complexity of problems (Durak ...
Research into technological problem solving has shown it to exist in a range of forms and draw upon different processes and knowledge types. This paper adds to this understanding by identifying procedural and epistemic differences in relation to task performance for pupils solving a well-defined technological problem. The study is theoretically grounded in a transformative epistemology of ...
Complex problem solving (CPS) is considered to be one of the most important skills for successful learning. In an effort to explore the nature of CPS, this study aims to investigate the role of inductive reasoning (IR) and combinatorial reasoning (CR) in the problem-solving process of students using statistically distinguishable exploration strategies in the CPS environment.
Problem solving and problem posing have long been of interest to the mathematics education community. In this survey paper we first look at some of the seminal moments in the history of research on the important topics. We then use this history to position the state-of-the-art research being done in both problem solving and problem posing, before introducing the presented state-of-the-art ...
Problem solving is perhaps the key characteristic that makes us human. Given the kinds of problems that we face in a competitive economy and society, the new generation of learners is ever more required to have problem-solving abilities. By drawing from the literature on technological pedagogical content knowledge, design thinking, general and ...
First, learners themselves can contribute to better learning outcomes through their motivation, goal orientation, learnings efforts, and self-efficacy. Second, the design of the learning environment can facilitate learning and problem-solving processes by adapting important conditions such as difficulty, language, or learning aids.
same, if the errors or mistakes they make at each stages are the same, DM and P S processes. are the same. Problem-solving is often considered to be based upon application of an algorithm, while ...
problem-solving difficulties, by sex, by phase and th e students' problem-solving difficulties by academic program by phase. In this section of the paper, it should
The main areas that are taken into account in this research paper are, meaning and significance of problem solving skills, problem solving processes, and measures to bring about improvements in ...
Defining collaborative problem solving. Collaborative problem solving refers to "problem-solving activities that involve interactions among a group of individuals" (O'Neil et al., Citation 2003, p. 4; Zhang, Citation 1998, p. 1).In a more detailed definition, "CPS in educational setting is a process in which two or more collaborative parties interact with each other to share and ...
Research in Problem-Solving: Improving the Progression from Novice to Expert Roxi Finney Abstract: This paper presents a review of research in problem solving. The first section includes various research papers across multiple disciplines which call for the need to improve the problem solving skills of students and the need to improve the ...
Problem-solving ability is a mental process that is the conducting part of the larger problem process that includes problem finding, shaping, and reaching towards a final goal. A problem is any situation where you have an opportunity to make a difference to make things better
Out 46 of 303 children 174 influencing during mathematical physics problems (57.4%) the teachers children were perceived that the lack of ability in remembering books or reflections (37.6%), materials on physics problem classes factor skills and (55.1%), in Poor on on physics inexperience necessary problem-solving comprehensive Lack problem of ...
There have been a number of previous studies, emphasis has been placed on the importance and meaning of rational problem-solving processes in order to improve problem-solving abilities, and research has focused on individual problem solving styles (Woodman et al., 1993; Scott & Bruce, 1994).According to the personal innovation behavior model of Scott and Bruce (), climate has shown individual ...
72 university beginning physics students were constrained to analyze mechanics problems according to a hierarchical scheme that integrated concepts, principles, and procedures. After 5 1-hr sessions, students increased their reliance on the use of principles in categorizing problems according to similarity of solution and in writing qualitative explanations of physical situations. In contrast ...
The case for 'math-ish' thinking. In a new book, Jo Boaler argues for a more flexible, creative approach to math. "Stepping back and judging whether a calculation is reasonable might be the ...
1. Confirm the paper follows a clear structure or outline. Review the paper and confirm it covers the four components of a problem solution paper. Make sure it addresses the problem and the solution in detail. Check that your thesis statement appears in the introduction and in the conclusion sections of the paper.
Start with trust and end with speed. May 22, 2024. When it comes to solving complicated problems, the default for many organizational leaders is to take their time to work through the issues at hand.
How to Define a Research Problem | Ideas & Examples. Published on November 2, 2022 by Shona McCombes and Tegan George. Revised on May 31, 2023. A research problem is a specific issue or gap in existing knowledge that you aim to address in your research. You may choose to look for practical problems aimed at contributing to change, or theoretical problems aimed at expanding knowledge.
After you have chosen your topic, you can find instructions about how to develop your ideas, find a unique solution to the problem, and organize your essay in "How to Write Your Problem Solution Paper." 4 Parts of Problem Solution. Describe a problem vividly. Propose a solution. Argue that the solution is practical, feasible, cost-effective ...
A research problem is a definite or clear expression [statement] about an area of concern, a condition to be improved upon, a difficulty to be eliminated, or a troubling question that exists in scholarly literature, in theory, or within existing practice that points to a need for meaningful understanding and deliberate investigation.
Consider opening up this list whenever you're stuck solving a challenging problem. It's likely that one or more of these techniques can help! 1. Clarifying. Try to define the problem you are facing as precisely as you can, maybe by writing down a detailed description of exactly what the problem is and what constraints exist for a solution ...
4 Embrace Failure. In research, failure is not the opposite of success; it's a stepping stone towards it. Embrace each setback as an opportunity to learn and refine your approach. Analyzing why an ...
Problem solving is defined as the area of. cognitive psychology which deals with the processe s. involved in solving problems. Fig. 1: Deviation is Problem. Problem solving depends on individual ...