Have a language expert improve your writing
Run a free plagiarism check in 10 minutes, generate accurate citations for free.
- Knowledge Base
Methodology
- How to Write a Literature Review | Guide, Examples, & Templates

How to Write a Literature Review | Guide, Examples, & Templates
Published on January 2, 2023 by Shona McCombes . Revised on September 11, 2023.
What is a literature review? A literature review is a survey of scholarly sources on a specific topic. It provides an overview of current knowledge, allowing you to identify relevant theories, methods, and gaps in the existing research that you can later apply to your paper, thesis, or dissertation topic .
There are five key steps to writing a literature review:
- Search for relevant literature
- Evaluate sources
- Identify themes, debates, and gaps
- Outline the structure
- Write your literature review
A good literature review doesn’t just summarize sources—it analyzes, synthesizes , and critically evaluates to give a clear picture of the state of knowledge on the subject.
Instantly correct all language mistakes in your text
Upload your document to correct all your mistakes in minutes
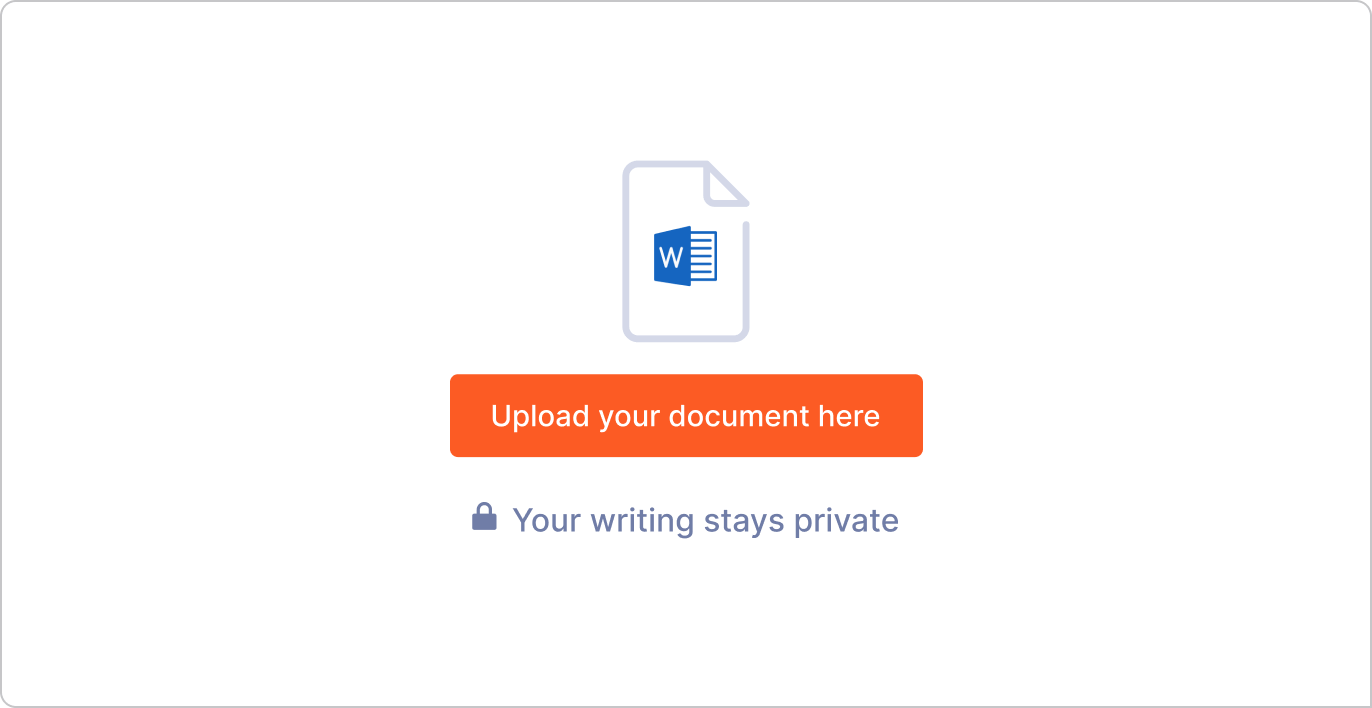
Table of contents
What is the purpose of a literature review, examples of literature reviews, step 1 – search for relevant literature, step 2 – evaluate and select sources, step 3 – identify themes, debates, and gaps, step 4 – outline your literature review’s structure, step 5 – write your literature review, free lecture slides, other interesting articles, frequently asked questions, introduction.
- Quick Run-through
- Step 1 & 2
When you write a thesis , dissertation , or research paper , you will likely have to conduct a literature review to situate your research within existing knowledge. The literature review gives you a chance to:
- Demonstrate your familiarity with the topic and its scholarly context
- Develop a theoretical framework and methodology for your research
- Position your work in relation to other researchers and theorists
- Show how your research addresses a gap or contributes to a debate
- Evaluate the current state of research and demonstrate your knowledge of the scholarly debates around your topic.
Writing literature reviews is a particularly important skill if you want to apply for graduate school or pursue a career in research. We’ve written a step-by-step guide that you can follow below.
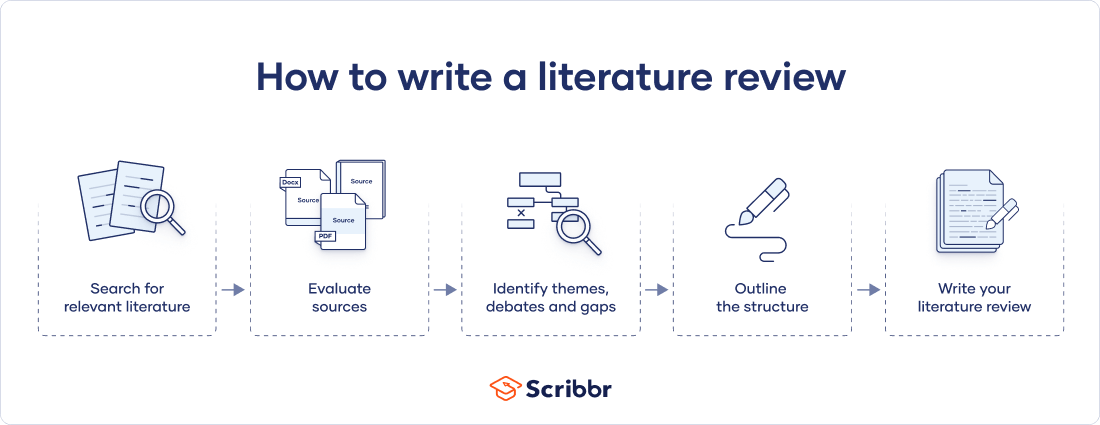
Here's why students love Scribbr's proofreading services
Discover proofreading & editing
Writing literature reviews can be quite challenging! A good starting point could be to look at some examples, depending on what kind of literature review you’d like to write.
- Example literature review #1: “Why Do People Migrate? A Review of the Theoretical Literature” ( Theoretical literature review about the development of economic migration theory from the 1950s to today.)
- Example literature review #2: “Literature review as a research methodology: An overview and guidelines” ( Methodological literature review about interdisciplinary knowledge acquisition and production.)
- Example literature review #3: “The Use of Technology in English Language Learning: A Literature Review” ( Thematic literature review about the effects of technology on language acquisition.)
- Example literature review #4: “Learners’ Listening Comprehension Difficulties in English Language Learning: A Literature Review” ( Chronological literature review about how the concept of listening skills has changed over time.)
You can also check out our templates with literature review examples and sample outlines at the links below.
Download Word doc Download Google doc
Before you begin searching for literature, you need a clearly defined topic .
If you are writing the literature review section of a dissertation or research paper, you will search for literature related to your research problem and questions .
Make a list of keywords
Start by creating a list of keywords related to your research question. Include each of the key concepts or variables you’re interested in, and list any synonyms and related terms. You can add to this list as you discover new keywords in the process of your literature search.
- Social media, Facebook, Instagram, Twitter, Snapchat, TikTok
- Body image, self-perception, self-esteem, mental health
- Generation Z, teenagers, adolescents, youth
Search for relevant sources
Use your keywords to begin searching for sources. Some useful databases to search for journals and articles include:
- Your university’s library catalogue
- Google Scholar
- Project Muse (humanities and social sciences)
- Medline (life sciences and biomedicine)
- EconLit (economics)
- Inspec (physics, engineering and computer science)
You can also use boolean operators to help narrow down your search.
Make sure to read the abstract to find out whether an article is relevant to your question. When you find a useful book or article, you can check the bibliography to find other relevant sources.
You likely won’t be able to read absolutely everything that has been written on your topic, so it will be necessary to evaluate which sources are most relevant to your research question.
For each publication, ask yourself:
- What question or problem is the author addressing?
- What are the key concepts and how are they defined?
- What are the key theories, models, and methods?
- Does the research use established frameworks or take an innovative approach?
- What are the results and conclusions of the study?
- How does the publication relate to other literature in the field? Does it confirm, add to, or challenge established knowledge?
- What are the strengths and weaknesses of the research?
Make sure the sources you use are credible , and make sure you read any landmark studies and major theories in your field of research.
You can use our template to summarize and evaluate sources you’re thinking about using. Click on either button below to download.
Take notes and cite your sources
As you read, you should also begin the writing process. Take notes that you can later incorporate into the text of your literature review.
It is important to keep track of your sources with citations to avoid plagiarism . It can be helpful to make an annotated bibliography , where you compile full citation information and write a paragraph of summary and analysis for each source. This helps you remember what you read and saves time later in the process.
To begin organizing your literature review’s argument and structure, be sure you understand the connections and relationships between the sources you’ve read. Based on your reading and notes, you can look for:
- Trends and patterns (in theory, method or results): do certain approaches become more or less popular over time?
- Themes: what questions or concepts recur across the literature?
- Debates, conflicts and contradictions: where do sources disagree?
- Pivotal publications: are there any influential theories or studies that changed the direction of the field?
- Gaps: what is missing from the literature? Are there weaknesses that need to be addressed?
This step will help you work out the structure of your literature review and (if applicable) show how your own research will contribute to existing knowledge.
- Most research has focused on young women.
- There is an increasing interest in the visual aspects of social media.
- But there is still a lack of robust research on highly visual platforms like Instagram and Snapchat—this is a gap that you could address in your own research.
There are various approaches to organizing the body of a literature review. Depending on the length of your literature review, you can combine several of these strategies (for example, your overall structure might be thematic, but each theme is discussed chronologically).
Chronological
The simplest approach is to trace the development of the topic over time. However, if you choose this strategy, be careful to avoid simply listing and summarizing sources in order.
Try to analyze patterns, turning points and key debates that have shaped the direction of the field. Give your interpretation of how and why certain developments occurred.
If you have found some recurring central themes, you can organize your literature review into subsections that address different aspects of the topic.
For example, if you are reviewing literature about inequalities in migrant health outcomes, key themes might include healthcare policy, language barriers, cultural attitudes, legal status, and economic access.
Methodological
If you draw your sources from different disciplines or fields that use a variety of research methods , you might want to compare the results and conclusions that emerge from different approaches. For example:
- Look at what results have emerged in qualitative versus quantitative research
- Discuss how the topic has been approached by empirical versus theoretical scholarship
- Divide the literature into sociological, historical, and cultural sources
Theoretical
A literature review is often the foundation for a theoretical framework . You can use it to discuss various theories, models, and definitions of key concepts.
You might argue for the relevance of a specific theoretical approach, or combine various theoretical concepts to create a framework for your research.
Like any other academic text , your literature review should have an introduction , a main body, and a conclusion . What you include in each depends on the objective of your literature review.
The introduction should clearly establish the focus and purpose of the literature review.
Depending on the length of your literature review, you might want to divide the body into subsections. You can use a subheading for each theme, time period, or methodological approach.
As you write, you can follow these tips:
- Summarize and synthesize: give an overview of the main points of each source and combine them into a coherent whole
- Analyze and interpret: don’t just paraphrase other researchers — add your own interpretations where possible, discussing the significance of findings in relation to the literature as a whole
- Critically evaluate: mention the strengths and weaknesses of your sources
- Write in well-structured paragraphs: use transition words and topic sentences to draw connections, comparisons and contrasts
In the conclusion, you should summarize the key findings you have taken from the literature and emphasize their significance.
When you’ve finished writing and revising your literature review, don’t forget to proofread thoroughly before submitting. Not a language expert? Check out Scribbr’s professional proofreading services !
This article has been adapted into lecture slides that you can use to teach your students about writing a literature review.
Scribbr slides are free to use, customize, and distribute for educational purposes.
Open Google Slides Download PowerPoint
If you want to know more about the research process , methodology , research bias , or statistics , make sure to check out some of our other articles with explanations and examples.
- Sampling methods
- Simple random sampling
- Stratified sampling
- Cluster sampling
- Likert scales
- Reproducibility
Statistics
- Null hypothesis
- Statistical power
- Probability distribution
- Effect size
- Poisson distribution
Research bias
- Optimism bias
- Cognitive bias
- Implicit bias
- Hawthorne effect
- Anchoring bias
- Explicit bias
A literature review is a survey of scholarly sources (such as books, journal articles, and theses) related to a specific topic or research question .
It is often written as part of a thesis, dissertation , or research paper , in order to situate your work in relation to existing knowledge.
There are several reasons to conduct a literature review at the beginning of a research project:
- To familiarize yourself with the current state of knowledge on your topic
- To ensure that you’re not just repeating what others have already done
- To identify gaps in knowledge and unresolved problems that your research can address
- To develop your theoretical framework and methodology
- To provide an overview of the key findings and debates on the topic
Writing the literature review shows your reader how your work relates to existing research and what new insights it will contribute.
The literature review usually comes near the beginning of your thesis or dissertation . After the introduction , it grounds your research in a scholarly field and leads directly to your theoretical framework or methodology .
A literature review is a survey of credible sources on a topic, often used in dissertations , theses, and research papers . Literature reviews give an overview of knowledge on a subject, helping you identify relevant theories and methods, as well as gaps in existing research. Literature reviews are set up similarly to other academic texts , with an introduction , a main body, and a conclusion .
An annotated bibliography is a list of source references that has a short description (called an annotation ) for each of the sources. It is often assigned as part of the research process for a paper .
Cite this Scribbr article
If you want to cite this source, you can copy and paste the citation or click the “Cite this Scribbr article” button to automatically add the citation to our free Citation Generator.
McCombes, S. (2023, September 11). How to Write a Literature Review | Guide, Examples, & Templates. Scribbr. Retrieved July 8, 2024, from https://www.scribbr.com/dissertation/literature-review/
Is this article helpful?
Shona McCombes
Other students also liked, what is a theoretical framework | guide to organizing, what is a research methodology | steps & tips, how to write a research proposal | examples & templates, get unlimited documents corrected.
✔ Free APA citation check included ✔ Unlimited document corrections ✔ Specialized in correcting academic texts
Purdue Online Writing Lab Purdue OWL® College of Liberal Arts
Writing a Literature Review
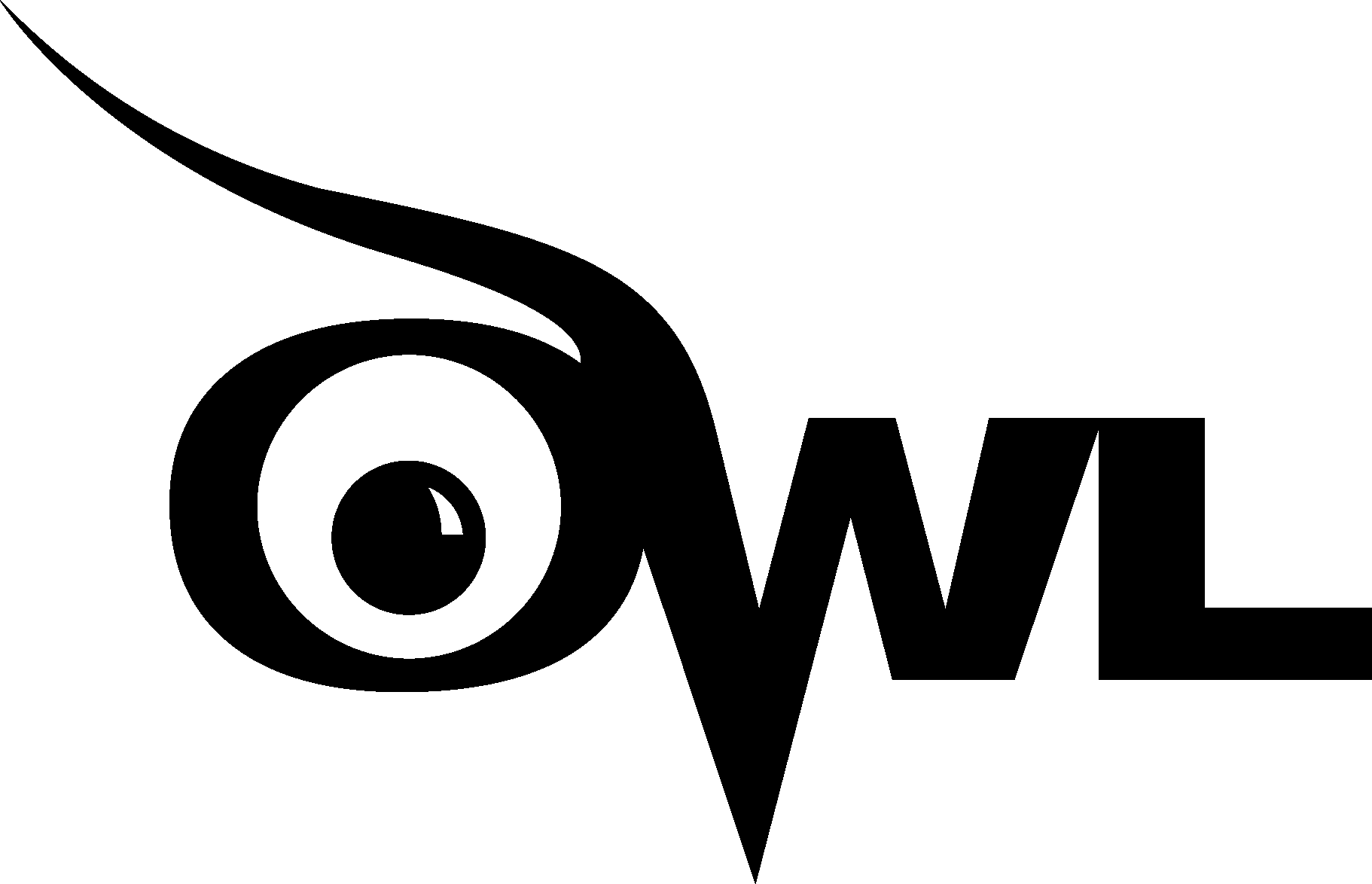
Welcome to the Purdue OWL
This page is brought to you by the OWL at Purdue University. When printing this page, you must include the entire legal notice.
Copyright ©1995-2018 by The Writing Lab & The OWL at Purdue and Purdue University. All rights reserved. This material may not be published, reproduced, broadcast, rewritten, or redistributed without permission. Use of this site constitutes acceptance of our terms and conditions of fair use.
A literature review is a document or section of a document that collects key sources on a topic and discusses those sources in conversation with each other (also called synthesis ). The lit review is an important genre in many disciplines, not just literature (i.e., the study of works of literature such as novels and plays). When we say “literature review” or refer to “the literature,” we are talking about the research ( scholarship ) in a given field. You will often see the terms “the research,” “the scholarship,” and “the literature” used mostly interchangeably.
Where, when, and why would I write a lit review?
There are a number of different situations where you might write a literature review, each with slightly different expectations; different disciplines, too, have field-specific expectations for what a literature review is and does. For instance, in the humanities, authors might include more overt argumentation and interpretation of source material in their literature reviews, whereas in the sciences, authors are more likely to report study designs and results in their literature reviews; these differences reflect these disciplines’ purposes and conventions in scholarship. You should always look at examples from your own discipline and talk to professors or mentors in your field to be sure you understand your discipline’s conventions, for literature reviews as well as for any other genre.
A literature review can be a part of a research paper or scholarly article, usually falling after the introduction and before the research methods sections. In these cases, the lit review just needs to cover scholarship that is important to the issue you are writing about; sometimes it will also cover key sources that informed your research methodology.
Lit reviews can also be standalone pieces, either as assignments in a class or as publications. In a class, a lit review may be assigned to help students familiarize themselves with a topic and with scholarship in their field, get an idea of the other researchers working on the topic they’re interested in, find gaps in existing research in order to propose new projects, and/or develop a theoretical framework and methodology for later research. As a publication, a lit review usually is meant to help make other scholars’ lives easier by collecting and summarizing, synthesizing, and analyzing existing research on a topic. This can be especially helpful for students or scholars getting into a new research area, or for directing an entire community of scholars toward questions that have not yet been answered.
What are the parts of a lit review?
Most lit reviews use a basic introduction-body-conclusion structure; if your lit review is part of a larger paper, the introduction and conclusion pieces may be just a few sentences while you focus most of your attention on the body. If your lit review is a standalone piece, the introduction and conclusion take up more space and give you a place to discuss your goals, research methods, and conclusions separately from where you discuss the literature itself.
Introduction:
- An introductory paragraph that explains what your working topic and thesis is
- A forecast of key topics or texts that will appear in the review
- Potentially, a description of how you found sources and how you analyzed them for inclusion and discussion in the review (more often found in published, standalone literature reviews than in lit review sections in an article or research paper)
- Summarize and synthesize: Give an overview of the main points of each source and combine them into a coherent whole
- Analyze and interpret: Don’t just paraphrase other researchers – add your own interpretations where possible, discussing the significance of findings in relation to the literature as a whole
- Critically Evaluate: Mention the strengths and weaknesses of your sources
- Write in well-structured paragraphs: Use transition words and topic sentence to draw connections, comparisons, and contrasts.
Conclusion:
- Summarize the key findings you have taken from the literature and emphasize their significance
- Connect it back to your primary research question
How should I organize my lit review?
Lit reviews can take many different organizational patterns depending on what you are trying to accomplish with the review. Here are some examples:
- Chronological : The simplest approach is to trace the development of the topic over time, which helps familiarize the audience with the topic (for instance if you are introducing something that is not commonly known in your field). If you choose this strategy, be careful to avoid simply listing and summarizing sources in order. Try to analyze the patterns, turning points, and key debates that have shaped the direction of the field. Give your interpretation of how and why certain developments occurred (as mentioned previously, this may not be appropriate in your discipline — check with a teacher or mentor if you’re unsure).
- Thematic : If you have found some recurring central themes that you will continue working with throughout your piece, you can organize your literature review into subsections that address different aspects of the topic. For example, if you are reviewing literature about women and religion, key themes can include the role of women in churches and the religious attitude towards women.
- Qualitative versus quantitative research
- Empirical versus theoretical scholarship
- Divide the research by sociological, historical, or cultural sources
- Theoretical : In many humanities articles, the literature review is the foundation for the theoretical framework. You can use it to discuss various theories, models, and definitions of key concepts. You can argue for the relevance of a specific theoretical approach or combine various theorical concepts to create a framework for your research.
What are some strategies or tips I can use while writing my lit review?
Any lit review is only as good as the research it discusses; make sure your sources are well-chosen and your research is thorough. Don’t be afraid to do more research if you discover a new thread as you’re writing. More info on the research process is available in our "Conducting Research" resources .
As you’re doing your research, create an annotated bibliography ( see our page on the this type of document ). Much of the information used in an annotated bibliography can be used also in a literature review, so you’ll be not only partially drafting your lit review as you research, but also developing your sense of the larger conversation going on among scholars, professionals, and any other stakeholders in your topic.
Usually you will need to synthesize research rather than just summarizing it. This means drawing connections between sources to create a picture of the scholarly conversation on a topic over time. Many student writers struggle to synthesize because they feel they don’t have anything to add to the scholars they are citing; here are some strategies to help you:
- It often helps to remember that the point of these kinds of syntheses is to show your readers how you understand your research, to help them read the rest of your paper.
- Writing teachers often say synthesis is like hosting a dinner party: imagine all your sources are together in a room, discussing your topic. What are they saying to each other?
- Look at the in-text citations in each paragraph. Are you citing just one source for each paragraph? This usually indicates summary only. When you have multiple sources cited in a paragraph, you are more likely to be synthesizing them (not always, but often
- Read more about synthesis here.
The most interesting literature reviews are often written as arguments (again, as mentioned at the beginning of the page, this is discipline-specific and doesn’t work for all situations). Often, the literature review is where you can establish your research as filling a particular gap or as relevant in a particular way. You have some chance to do this in your introduction in an article, but the literature review section gives a more extended opportunity to establish the conversation in the way you would like your readers to see it. You can choose the intellectual lineage you would like to be part of and whose definitions matter most to your thinking (mostly humanities-specific, but this goes for sciences as well). In addressing these points, you argue for your place in the conversation, which tends to make the lit review more compelling than a simple reporting of other sources.
- Resources Home 🏠
- Try SciSpace Copilot
- Search research papers
- Add Copilot Extension
- Try AI Detector
- Try Paraphraser
- Try Citation Generator
- April Papers
- June Papers
- July Papers

How To Write A Literature Review - A Complete Guide

Table of Contents
A literature review is much more than just another section in your research paper. It forms the very foundation of your research. It is a formal piece of writing where you analyze the existing theoretical framework, principles, and assumptions and use that as a base to shape your approach to the research question.
Curating and drafting a solid literature review section not only lends more credibility to your research paper but also makes your research tighter and better focused. But, writing literature reviews is a difficult task. It requires extensive reading, plus you have to consider market trends and technological and political changes, which tend to change in the blink of an eye.
Now streamline your literature review process with the help of SciSpace Copilot. With this AI research assistant, you can efficiently synthesize and analyze a vast amount of information, identify key themes and trends, and uncover gaps in the existing research. Get real-time explanations, summaries, and answers to your questions for the paper you're reviewing, making navigating and understanding the complex literature landscape easier.
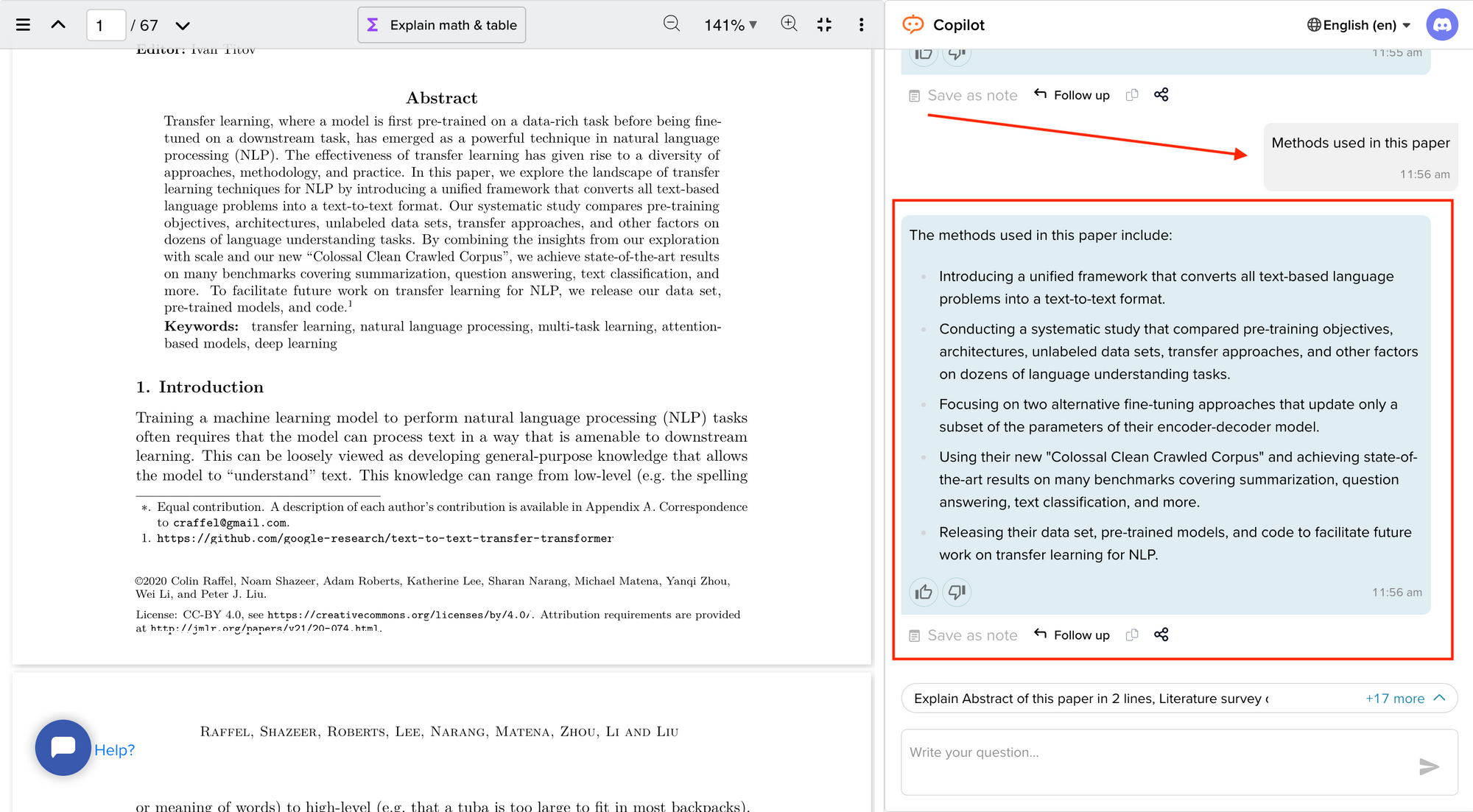
In this comprehensive guide, we will explore everything from the definition of a literature review, its appropriate length, various types of literature reviews, and how to write one.
What is a literature review?
A literature review is a collation of survey, research, critical evaluation, and assessment of the existing literature in a preferred domain.
Eminent researcher and academic Arlene Fink, in her book Conducting Research Literature Reviews , defines it as the following:
“A literature review surveys books, scholarly articles, and any other sources relevant to a particular issue, area of research, or theory, and by so doing, provides a description, summary, and critical evaluation of these works in relation to the research problem being investigated.
Literature reviews are designed to provide an overview of sources you have explored while researching a particular topic, and to demonstrate to your readers how your research fits within a larger field of study.”
Simply put, a literature review can be defined as a critical discussion of relevant pre-existing research around your research question and carving out a definitive place for your study in the existing body of knowledge. Literature reviews can be presented in multiple ways: a section of an article, the whole research paper itself, or a chapter of your thesis.
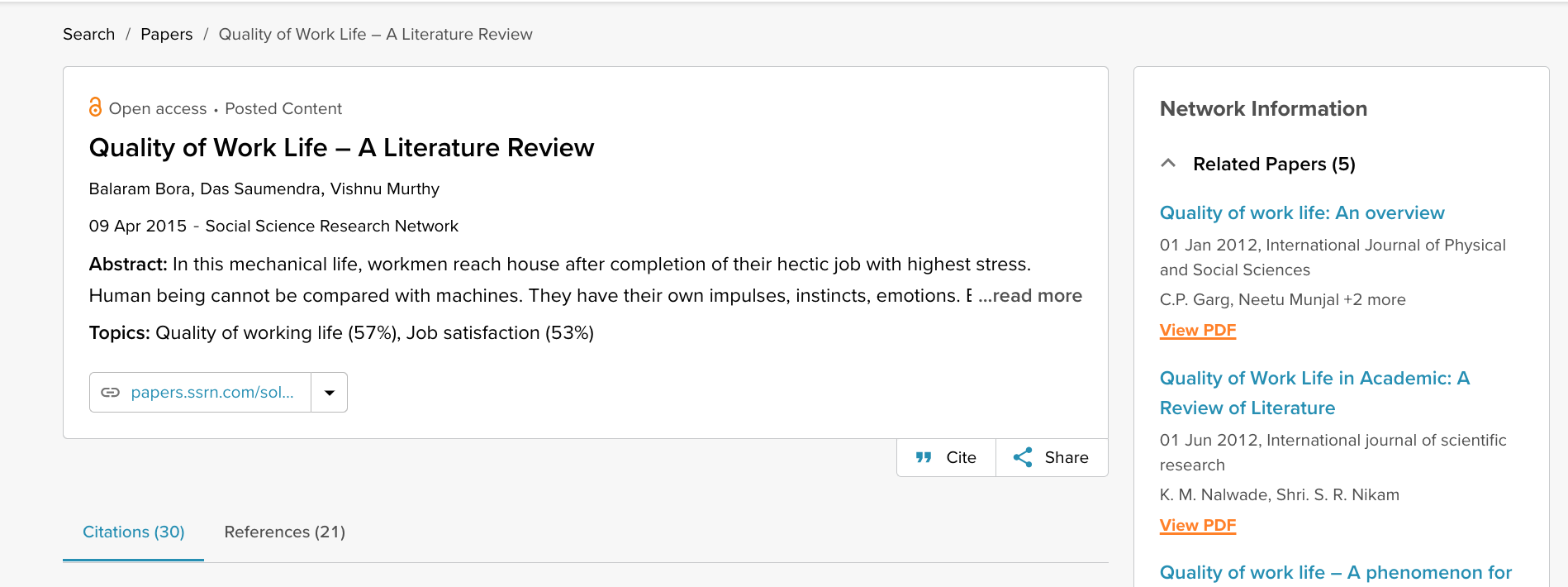
A literature review does function as a summary of sources, but it also allows you to analyze further, interpret, and examine the stated theories, methods, viewpoints, and, of course, the gaps in the existing content.
As an author, you can discuss and interpret the research question and its various aspects and debate your adopted methods to support the claim.
What is the purpose of a literature review?
A literature review is meant to help your readers understand the relevance of your research question and where it fits within the existing body of knowledge. As a researcher, you should use it to set the context, build your argument, and establish the need for your study.
What is the importance of a literature review?
The literature review is a critical part of research papers because it helps you:
- Gain an in-depth understanding of your research question and the surrounding area
- Convey that you have a thorough understanding of your research area and are up-to-date with the latest changes and advancements
- Establish how your research is connected or builds on the existing body of knowledge and how it could contribute to further research
- Elaborate on the validity and suitability of your theoretical framework and research methodology
- Identify and highlight gaps and shortcomings in the existing body of knowledge and how things need to change
- Convey to readers how your study is different or how it contributes to the research area
How long should a literature review be?
Ideally, the literature review should take up 15%-40% of the total length of your manuscript. So, if you have a 10,000-word research paper, the minimum word count could be 1500.
Your literature review format depends heavily on the kind of manuscript you are writing — an entire chapter in case of doctoral theses, a part of the introductory section in a research article, to a full-fledged review article that examines the previously published research on a topic.
Another determining factor is the type of research you are doing. The literature review section tends to be longer for secondary research projects than primary research projects.
What are the different types of literature reviews?
All literature reviews are not the same. There are a variety of possible approaches that you can take. It all depends on the type of research you are pursuing.
Here are the different types of literature reviews:
Argumentative review
It is called an argumentative review when you carefully present literature that only supports or counters a specific argument or premise to establish a viewpoint.
Integrative review
It is a type of literature review focused on building a comprehensive understanding of a topic by combining available theoretical frameworks and empirical evidence.
Methodological review
This approach delves into the ''how'' and the ''what" of the research question — you cannot look at the outcome in isolation; you should also review the methodology used.
Systematic review
This form consists of an overview of existing evidence pertinent to a clearly formulated research question, which uses pre-specified and standardized methods to identify and critically appraise relevant research and collect, report, and analyze data from the studies included in the review.
Meta-analysis review
Meta-analysis uses statistical methods to summarize the results of independent studies. By combining information from all relevant studies, meta-analysis can provide more precise estimates of the effects than those derived from the individual studies included within a review.
Historical review
Historical literature reviews focus on examining research throughout a period, often starting with the first time an issue, concept, theory, or phenomenon emerged in the literature, then tracing its evolution within the scholarship of a discipline. The purpose is to place research in a historical context to show familiarity with state-of-the-art developments and identify future research's likely directions.
Theoretical Review
This form aims to examine the corpus of theory accumulated regarding an issue, concept, theory, and phenomenon. The theoretical literature review helps to establish what theories exist, the relationships between them, the degree the existing approaches have been investigated, and to develop new hypotheses to be tested.
Scoping Review
The Scoping Review is often used at the beginning of an article, dissertation, or research proposal. It is conducted before the research to highlight gaps in the existing body of knowledge and explains why the project should be greenlit.
State-of-the-Art Review
The State-of-the-Art review is conducted periodically, focusing on the most recent research. It describes what is currently known, understood, or agreed upon regarding the research topic and highlights where there are still disagreements.
Can you use the first person in a literature review?
When writing literature reviews, you should avoid the usage of first-person pronouns. It means that instead of "I argue that" or "we argue that," the appropriate expression would be "this research paper argues that."
Do you need an abstract for a literature review?
Ideally, yes. It is always good to have a condensed summary that is self-contained and independent of the rest of your review. As for how to draft one, you can follow the same fundamental idea when preparing an abstract for a literature review. It should also include:
- The research topic and your motivation behind selecting it
- A one-sentence thesis statement
- An explanation of the kinds of literature featured in the review
- Summary of what you've learned
- Conclusions you drew from the literature you reviewed
- Potential implications and future scope for research
Here's an example of the abstract of a literature review
Is a literature review written in the past tense?
Yes, the literature review should ideally be written in the past tense. You should not use the present or future tense when writing one. The exceptions are when you have statements describing events that happened earlier than the literature you are reviewing or events that are currently occurring; then, you can use the past perfect or present perfect tenses.
How many sources for a literature review?
There are multiple approaches to deciding how many sources to include in a literature review section. The first approach would be to look level you are at as a researcher. For instance, a doctoral thesis might need 60+ sources. In contrast, you might only need to refer to 5-15 sources at the undergraduate level.
The second approach is based on the kind of literature review you are doing — whether it is merely a chapter of your paper or if it is a self-contained paper in itself. When it is just a chapter, sources should equal the total number of pages in your article's body. In the second scenario, you need at least three times as many sources as there are pages in your work.
Quick tips on how to write a literature review
To know how to write a literature review, you must clearly understand its impact and role in establishing your work as substantive research material.
You need to follow the below-mentioned steps, to write a literature review:
- Outline the purpose behind the literature review
- Search relevant literature
- Examine and assess the relevant resources
- Discover connections by drawing deep insights from the resources
- Structure planning to write a good literature review
1. Outline and identify the purpose of a literature review
As a first step on how to write a literature review, you must know what the research question or topic is and what shape you want your literature review to take. Ensure you understand the research topic inside out, or else seek clarifications. You must be able to the answer below questions before you start:
- How many sources do I need to include?
- What kind of sources should I analyze?
- How much should I critically evaluate each source?
- Should I summarize, synthesize or offer a critique of the sources?
- Do I need to include any background information or definitions?
Additionally, you should know that the narrower your research topic is, the swifter it will be for you to restrict the number of sources to be analyzed.
2. Search relevant literature
Dig deeper into search engines to discover what has already been published around your chosen topic. Make sure you thoroughly go through appropriate reference sources like books, reports, journal articles, government docs, and web-based resources.
You must prepare a list of keywords and their different variations. You can start your search from any library’s catalog, provided you are an active member of that institution. The exact keywords can be extended to widen your research over other databases and academic search engines like:
- Google Scholar
- Microsoft Academic
- Science.gov
Besides, it is not advisable to go through every resource word by word. Alternatively, what you can do is you can start by reading the abstract and then decide whether that source is relevant to your research or not.
Additionally, you must spend surplus time assessing the quality and relevance of resources. It would help if you tried preparing a list of citations to ensure that there lies no repetition of authors, publications, or articles in the literature review.
3. Examine and assess the sources
It is nearly impossible for you to go through every detail in the research article. So rather than trying to fetch every detail, you have to analyze and decide which research sources resemble closest and appear relevant to your chosen domain.
While analyzing the sources, you should look to find out answers to questions like:
- What question or problem has the author been describing and debating?
- What is the definition of critical aspects?
- How well the theories, approach, and methodology have been explained?
- Whether the research theory used some conventional or new innovative approach?
- How relevant are the key findings of the work?
- In what ways does it relate to other sources on the same topic?
- What challenges does this research paper pose to the existing theory
- What are the possible contributions or benefits it adds to the subject domain?
Be always mindful that you refer only to credible and authentic resources. It would be best if you always take references from different publications to validate your theory.
Always keep track of important information or data you can present in your literature review right from the beginning. It will help steer your path from any threats of plagiarism and also make it easier to curate an annotated bibliography or reference section.
4. Discover connections
At this stage, you must start deciding on the argument and structure of your literature review. To accomplish this, you must discover and identify the relations and connections between various resources while drafting your abstract.
A few aspects that you should be aware of while writing a literature review include:
- Rise to prominence: Theories and methods that have gained reputation and supporters over time.
- Constant scrutiny: Concepts or theories that repeatedly went under examination.
- Contradictions and conflicts: Theories, both the supporting and the contradictory ones, for the research topic.
- Knowledge gaps: What exactly does it fail to address, and how to bridge them with further research?
- Influential resources: Significant research projects available that have been upheld as milestones or perhaps, something that can modify the current trends
Once you join the dots between various past research works, it will be easier for you to draw a conclusion and identify your contribution to the existing knowledge base.
5. Structure planning to write a good literature review
There exist different ways towards planning and executing the structure of a literature review. The format of a literature review varies and depends upon the length of the research.
Like any other research paper, the literature review format must contain three sections: introduction, body, and conclusion. The goals and objectives of the research question determine what goes inside these three sections.
Nevertheless, a good literature review can be structured according to the chronological, thematic, methodological, or theoretical framework approach.
Literature review samples
1. Standalone
2. As a section of a research paper
How SciSpace Discover makes literature review a breeze?
SciSpace Discover is a one-stop solution to do an effective literature search and get barrier-free access to scientific knowledge. It is an excellent repository where you can find millions of only peer-reviewed articles and full-text PDF files. Here’s more on how you can use it:
Find the right information
Find what you want quickly and easily with comprehensive search filters that let you narrow down papers according to PDF availability, year of publishing, document type, and affiliated institution. Moreover, you can sort the results based on the publishing date, citation count, and relevance.
Assess credibility of papers quickly
When doing the literature review, it is critical to establish the quality of your sources. They form the foundation of your research. SciSpace Discover helps you assess the quality of a source by providing an overview of its references, citations, and performance metrics.
Get the complete picture in no time
SciSpace Discover’s personalized suggestion engine helps you stay on course and get the complete picture of the topic from one place. Every time you visit an article page, it provides you links to related papers. Besides that, it helps you understand what’s trending, who are the top authors, and who are the leading publishers on a topic.
Make referring sources super easy
To ensure you don't lose track of your sources, you must start noting down your references when doing the literature review. SciSpace Discover makes this step effortless. Click the 'cite' button on an article page, and you will receive preloaded citation text in multiple styles — all you've to do is copy-paste it into your manuscript.
Final tips on how to write a literature review
A massive chunk of time and effort is required to write a good literature review. But, if you go about it systematically, you'll be able to save a ton of time and build a solid foundation for your research.
We hope this guide has helped you answer several key questions you have about writing literature reviews.
Would you like to explore SciSpace Discover and kick off your literature search right away? You can get started here .
Frequently Asked Questions (FAQs)
1. how to start a literature review.
• What questions do you want to answer?
• What sources do you need to answer these questions?
• What information do these sources contain?
• How can you use this information to answer your questions?
2. What to include in a literature review?
• A brief background of the problem or issue
• What has previously been done to address the problem or issue
• A description of what you will do in your project
• How this study will contribute to research on the subject
3. Why literature review is important?
The literature review is an important part of any research project because it allows the writer to look at previous studies on a topic and determine existing gaps in the literature, as well as what has already been done. It will also help them to choose the most appropriate method for their own study.
4. How to cite a literature review in APA format?
To cite a literature review in APA style, you need to provide the author's name, the title of the article, and the year of publication. For example: Patel, A. B., & Stokes, G. S. (2012). The relationship between personality and intelligence: A meta-analysis of longitudinal research. Personality and Individual Differences, 53(1), 16-21
5. What are the components of a literature review?
• A brief introduction to the topic, including its background and context. The introduction should also include a rationale for why the study is being conducted and what it will accomplish.
• A description of the methodologies used in the study. This can include information about data collection methods, sample size, and statistical analyses.
• A presentation of the findings in an organized format that helps readers follow along with the author's conclusions.
6. What are common errors in writing literature review?
• Not spending enough time to critically evaluate the relevance of resources, observations and conclusions.
• Totally relying on secondary data while ignoring primary data.
• Letting your personal bias seep into your interpretation of existing literature.
• No detailed explanation of the procedure to discover and identify an appropriate literature review.
7. What are the 5 C's of writing literature review?
• Cite - the sources you utilized and referenced in your research.
• Compare - existing arguments, hypotheses, methodologies, and conclusions found in the knowledge base.
• Contrast - the arguments, topics, methodologies, approaches, and disputes that may be found in the literature.
• Critique - the literature and describe the ideas and opinions you find more convincing and why.
• Connect - the various studies you reviewed in your research.
8. How many sources should a literature review have?
When it is just a chapter, sources should equal the total number of pages in your article's body. if it is a self-contained paper in itself, you need at least three times as many sources as there are pages in your work.
9. Can literature review have diagrams?
• To represent an abstract idea or concept
• To explain the steps of a process or procedure
• To help readers understand the relationships between different concepts
10. How old should sources be in a literature review?
Sources for a literature review should be as current as possible or not older than ten years. The only exception to this rule is if you are reviewing a historical topic and need to use older sources.
11. What are the types of literature review?
• Argumentative review
• Integrative review
• Methodological review
• Systematic review
• Meta-analysis review
• Historical review
• Theoretical review
• Scoping review
• State-of-the-Art review
12. Is a literature review mandatory?
Yes. Literature review is a mandatory part of any research project. It is a critical step in the process that allows you to establish the scope of your research, and provide a background for the rest of your work.
But before you go,
- Six Online Tools for Easy Literature Review
- Evaluating literature review: systematic vs. scoping reviews
- Systematic Approaches to a Successful Literature Review
- Writing Integrative Literature Reviews: Guidelines and Examples
You might also like

Consensus GPT vs. SciSpace GPT: Choose the Best GPT for Research
Literature Review and Theoretical Framework: Understanding the Differences

Types of Essays in Academic Writing - Quick Guide (2024)
Thank you for visiting nature.com. You are using a browser version with limited support for CSS. To obtain the best experience, we recommend you use a more up to date browser (or turn off compatibility mode in Internet Explorer). In the meantime, to ensure continued support, we are displaying the site without styles and JavaScript.
- View all journals
- Explore content
- About the journal
- Publish with us
- Sign up for alerts
- CAREER FEATURE
- 04 December 2020
- Correction 09 December 2020
How to write a superb literature review
Andy Tay is a freelance writer based in Singapore.
You can also search for this author in PubMed Google Scholar
Literature reviews are important resources for scientists. They provide historical context for a field while offering opinions on its future trajectory. Creating them can provide inspiration for one’s own research, as well as some practice in writing. But few scientists are trained in how to write a review — or in what constitutes an excellent one. Even picking the appropriate software to use can be an involved decision (see ‘Tools and techniques’). So Nature asked editors and working scientists with well-cited reviews for their tips.
Access options
Access Nature and 54 other Nature Portfolio journals
Get Nature+, our best-value online-access subscription
24,99 € / 30 days
cancel any time
Subscribe to this journal
Receive 51 print issues and online access
185,98 € per year
only 3,65 € per issue
Rent or buy this article
Prices vary by article type
Prices may be subject to local taxes which are calculated during checkout
doi: https://doi.org/10.1038/d41586-020-03422-x
Interviews have been edited for length and clarity.
Updates & Corrections
Correction 09 December 2020 : An earlier version of the tables in this article included some incorrect details about the programs Zotero, Endnote and Manubot. These have now been corrected.
Hsing, I.-M., Xu, Y. & Zhao, W. Electroanalysis 19 , 755–768 (2007).
Article Google Scholar
Ledesma, H. A. et al. Nature Nanotechnol. 14 , 645–657 (2019).
Article PubMed Google Scholar
Brahlek, M., Koirala, N., Bansal, N. & Oh, S. Solid State Commun. 215–216 , 54–62 (2015).
Choi, Y. & Lee, S. Y. Nature Rev. Chem . https://doi.org/10.1038/s41570-020-00221-w (2020).
Download references
Related Articles
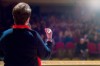
- Research management
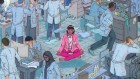
How PhD students and other academics are fighting the mental-health crisis in science
News Feature 09 JUL 24
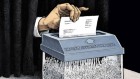
How can I break into industry if my CV keeps disappearing into a black hole?
Career Feature 08 JUL 24
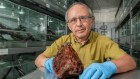
Harrowing trends: how endangered-species researchers find hope in the dark
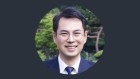
Is your research a trade secret? South Korean data-sharing case is a wake-up call
World View 03 JUL 24
To regain lost public trust, incorporate research ethics into graduate training
Correspondence 02 JUL 24
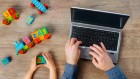
What it means to be a successful male academic
Career Column 26 JUN 24
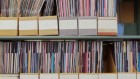
Western scientists more likely to get rejected papers published — and do it faster
News 02 JUL 24
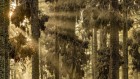
Spy on millions of sleeping butterflies and more — June’s best science images
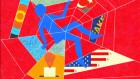
‘It can feel like there’s no way out’ — political scientists face pushback on their work
News Feature 19 JUN 24
Postdoctoral Fellow - Boyi Gan lab
New postdoctoral positions are open in a cancer research laboratory located within The University of Texas MD Anderson Cancer Center. The lab curre...
Houston, Texas (US)
The University of Texas MD Anderson Cancer Center - Experimental Radiation Oncology
Senior Research Associates x 3 – Bioinformatician Team
The Genomics and Bioinformatics Core (GBC) within the Institute of Metabolic Science – Metabolic Research Laboratories at the Clinical School, Univers
Cambridge, Cambridgeshire
University of Cambridge
Al Medical Engineering at School of Biomedical Engineering
Tsinghua BME offers faculty positions in the emerging research direction of AI Medical Engineering
Beijing, China
Tsinghua University
Shanghai Jiao Tong University Global Recruitment
Interested applicants can send CV to the relevant department/school.
Shanghai, China
Shanghai Jiao Tong University
Faculty Positions in School of Engineering, Westlake University
The School of Engineering (SOE) at Westlake University is seeking to fill multiple tenured or tenure-track faculty positions in all ranks.
Hangzhou, Zhejiang, China
Westlake University
Sign up for the Nature Briefing newsletter — what matters in science, free to your inbox daily.
Quick links
- Explore articles by subject
- Guide to authors
- Editorial policies

An official website of the United States government
The .gov means it’s official. Federal government websites often end in .gov or .mil. Before sharing sensitive information, make sure you’re on a federal government site.
The site is secure. The https:// ensures that you are connecting to the official website and that any information you provide is encrypted and transmitted securely.
- Publications
- Account settings
Preview improvements coming to the PMC website in October 2024. Learn More or Try it out now .
- Advanced Search
- Journal List
- Perspect Med Educ
- v.7(1); 2018 Feb

Writing an effective literature review
Lorelei lingard.
Schulich School of Medicine & Dentistry, Health Sciences Addition, Western University, London, Ontario Canada
In the Writer’s Craft section we offer simple tips to improve your writing in one of three areas: Energy, Clarity and Persuasiveness. Each entry focuses on a key writing feature or strategy, illustrates how it commonly goes wrong, teaches the grammatical underpinnings necessary to understand it and offers suggestions to wield it effectively. We encourage readers to share comments on or suggestions for this section on Twitter, using the hashtag: #how’syourwriting?
This Writer’s Craft instalment is the first in a two-part series that offers strategies for effectively presenting the literature review section of a research manuscript. This piece alerts writers to the importance of not only summarizing what is known but also identifying precisely what is not, in order to explicitly signal the relevance of their research. In this instalment, I will introduce readers to the mapping the gap metaphor, the knowledge claims heuristic, and the need to characterize the gap.
Mapping the gap
The purpose of the literature review section of a manuscript is not to report what is known about your topic. The purpose is to identify what remains unknown— what academic writing scholar Janet Giltrow has called the ‘knowledge deficit’ — thus establishing the need for your research study [ 1 ]. In an earlier Writer’s Craft instalment, the Problem-Gap-Hook heuristic was introduced as a way of opening your paper with a clear statement of the problem that your work grapples with, the gap in our current knowledge about that problem, and the reason the gap matters [ 2 ]. This article explains how to use the literature review section of your paper to build and characterize the Gap claim in your Problem-Gap-Hook. The metaphor of ‘mapping the gap’ is a way of thinking about how to select and arrange your review of the existing literature so that readers can recognize why your research needed to be done, and why its results constitute a meaningful advance on what was already known about the topic.
Many writers have learned that the literature review should describe what is known. The trouble with this approach is that it can produce a laundry list of facts-in-the-world that does not persuade the reader that the current study is a necessary next step. Instead, think of your literature review as painting in a map of your research domain: as you review existing knowledge, you are painting in sections of the map, but your goal is not to end with the whole map fully painted. That would mean there is nothing more we need to know about the topic, and that leaves no room for your research. What you want to end up with is a map in which painted sections surround and emphasize a white space, a gap in what is known that matters. Conceptualizing your literature review this way helps to ensure that it achieves its dual goal: of presenting what is known and pointing out what is not—the latter of these goals is necessary for your literature review to establish the necessity and importance of the research you are about to describe in the methods section which will immediately follow the literature review.
To a novice researcher or graduate student, this may seem counterintuitive. Hopefully you have invested significant time in reading the existing literature, and you are understandably keen to demonstrate that you’ve read everything ever published about your topic! Be careful, though, not to use the literature review section to regurgitate all of your reading in manuscript form. For one thing, it creates a laundry list of facts that makes for horrible reading. But there are three other reasons for avoiding this approach. First, you don’t have the space. In published medical education research papers, the literature review is quite short, ranging from a few paragraphs to a few pages, so you can’t summarize everything you’ve read. Second, you’re preaching to the converted. If you approach your paper as a contribution to an ongoing scholarly conversation,[ 2 ] then your literature review should summarize just the aspects of that conversation that are required to situate your conversational turn as informed and relevant. Third, the key to relevance is to point to a gap in what is known. To do so, you summarize what is known for the express purpose of identifying what is not known . Seen this way, the literature review should exert a gravitational pull on the reader, leading them inexorably to the white space on the map of knowledge you’ve painted for them. That white space is the space that your research fills.
Knowledge claims
To help writers move beyond the laundry list, the notion of ‘knowledge claims’ can be useful. A knowledge claim is a way of presenting the growing understanding of the community of researchers who have been exploring your topic. These are not disembodied facts, but rather incremental insights that some in the field may agree with and some may not, depending on their different methodological and disciplinary approaches to the topic. Treating the literature review as a story of the knowledge claims being made by researchers in the field can help writers with one of the most sophisticated aspects of a literature review—locating the knowledge being reviewed. Where does it come from? What is debated? How do different methodologies influence the knowledge being accumulated? And so on.
Consider this example of the knowledge claims (KC), Gap and Hook for the literature review section of a research paper on distributed healthcare teamwork:
KC: We know that poor team communication can cause errors. KC: And we know that team training can be effective in improving team communication. KC: This knowledge has prompted a push to incorporate teamwork training principles into health professions education curricula. KC: However, most of what we know about team training research has come from research with co-located teams—i. e., teams whose members work together in time and space. Gap: Little is known about how teamwork training principles would apply in distributed teams, whose members work asynchronously and are spread across different locations. Hook: Given that much healthcare teamwork is distributed rather than co-located, our curricula will be severely lacking until we create refined teamwork training principles that reflect distributed as well as co-located work contexts.
The ‘We know that …’ structure illustrated in this example is a template for helping you draft and organize. In your final version, your knowledge claims will be expressed with more sophistication. For instance, ‘We know that poor team communication can cause errors’ will become something like ‘Over a decade of patient safety research has demonstrated that poor team communication is the dominant cause of medical errors.’ This simple template of knowledge claims, though, provides an outline for the paragraphs in your literature review, each of which will provide detailed evidence to illustrate a knowledge claim. Using this approach, the order of the paragraphs in the literature review is strategic and persuasive, leading the reader to the gap claim that positions the relevance of the current study. To expand your vocabulary for creating such knowledge claims, linking them logically and positioning yourself amid them, I highly recommend Graff and Birkenstein’s little handbook of ‘templates’ [ 3 ].
As you organize your knowledge claims, you will also want to consider whether you are trying to map the gap in a well-studied field, or a relatively understudied one. The rhetorical challenge is different in each case. In a well-studied field, like professionalism in medical education, you must make a strong, explicit case for the existence of a gap. Readers may come to your paper tired of hearing about this topic and tempted to think we can’t possibly need more knowledge about it. Listing the knowledge claims can help you organize them most effectively and determine which pieces of knowledge may be unnecessary to map the white space your research attempts to fill. This does not mean that you leave out relevant information: your literature review must still be accurate. But, since you will not be able to include everything, selecting carefully among the possible knowledge claims is essential to producing a coherent, well-argued literature review.
Characterizing the gap
Once you’ve identified the gap, your literature review must characterize it. What kind of gap have you found? There are many ways to characterize a gap, but some of the more common include:
- a pure knowledge deficit—‘no one has looked at the relationship between longitudinal integrated clerkships and medical student abuse’
- a shortcoming in the scholarship, often due to philosophical or methodological tendencies and oversights—‘scholars have interpreted x from a cognitivist perspective, but ignored the humanist perspective’ or ‘to date, we have surveyed the frequency of medical errors committed by residents, but we have not explored their subjective experience of such errors’
- a controversy—‘scholars disagree on the definition of professionalism in medicine …’
- a pervasive and unproven assumption—‘the theme of technological heroism—technology will solve what ails teamwork—is ubiquitous in the literature, but what is that belief based on?’
To characterize the kind of gap, you need to know the literature thoroughly. That means more than understanding each paper individually; you also need to be placing each paper in relation to others. This may require changing your note-taking technique while you’re reading; take notes on what each paper contributes to knowledge, but also on how it relates to other papers you’ve read, and what it suggests about the kind of gap that is emerging.
In summary, think of your literature review as mapping the gap rather than simply summarizing the known. And pay attention to characterizing the kind of gap you’ve mapped. This strategy can help to make your literature review into a compelling argument rather than a list of facts. It can remind you of the danger of describing so fully what is known that the reader is left with the sense that there is no pressing need to know more. And it can help you to establish a coherence between the kind of gap you’ve identified and the study methodology you will use to fill it.
Acknowledgements
Thanks to Mark Goldszmidt for his feedback on an early version of this manuscript.
PhD, is director of the Centre for Education Research & Innovation at Schulich School of Medicine & Dentistry, and professor for the Department of Medicine at Western University in London, Ontario, Canada.
Have a language expert improve your writing
Run a free plagiarism check in 10 minutes, automatically generate references for free.
- Knowledge Base
- Dissertation
- What is a Literature Review? | Guide, Template, & Examples
What is a Literature Review? | Guide, Template, & Examples
Published on 22 February 2022 by Shona McCombes . Revised on 7 June 2022.
What is a literature review? A literature review is a survey of scholarly sources on a specific topic. It provides an overview of current knowledge, allowing you to identify relevant theories, methods, and gaps in the existing research.
There are five key steps to writing a literature review:
- Search for relevant literature
- Evaluate sources
- Identify themes, debates and gaps
- Outline the structure
- Write your literature review
A good literature review doesn’t just summarise sources – it analyses, synthesises, and critically evaluates to give a clear picture of the state of knowledge on the subject.
Instantly correct all language mistakes in your text
Be assured that you'll submit flawless writing. Upload your document to correct all your mistakes.
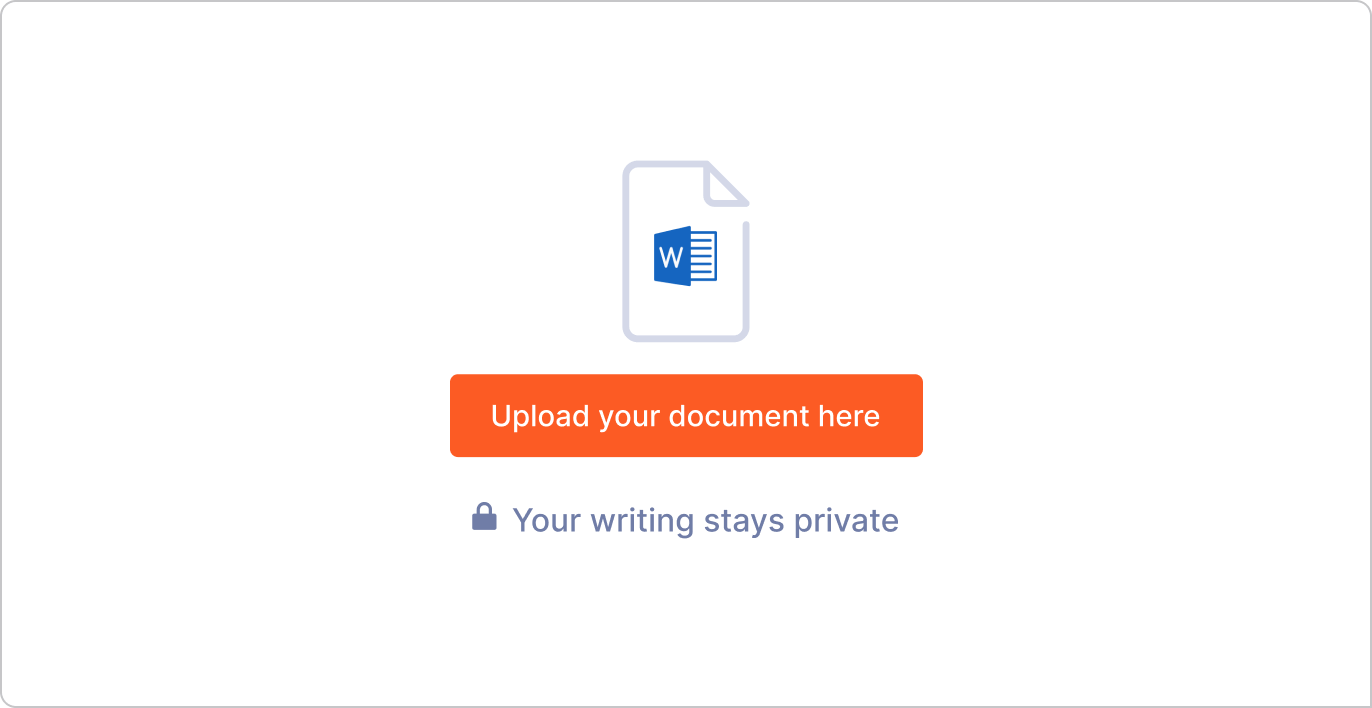
Table of contents
Why write a literature review, examples of literature reviews, step 1: search for relevant literature, step 2: evaluate and select sources, step 3: identify themes, debates and gaps, step 4: outline your literature review’s structure, step 5: write your literature review, frequently asked questions about literature reviews, introduction.
- Quick Run-through
- Step 1 & 2
When you write a dissertation or thesis, you will have to conduct a literature review to situate your research within existing knowledge. The literature review gives you a chance to:
- Demonstrate your familiarity with the topic and scholarly context
- Develop a theoretical framework and methodology for your research
- Position yourself in relation to other researchers and theorists
- Show how your dissertation addresses a gap or contributes to a debate
You might also have to write a literature review as a stand-alone assignment. In this case, the purpose is to evaluate the current state of research and demonstrate your knowledge of scholarly debates around a topic.
The content will look slightly different in each case, but the process of conducting a literature review follows the same steps. We’ve written a step-by-step guide that you can follow below.
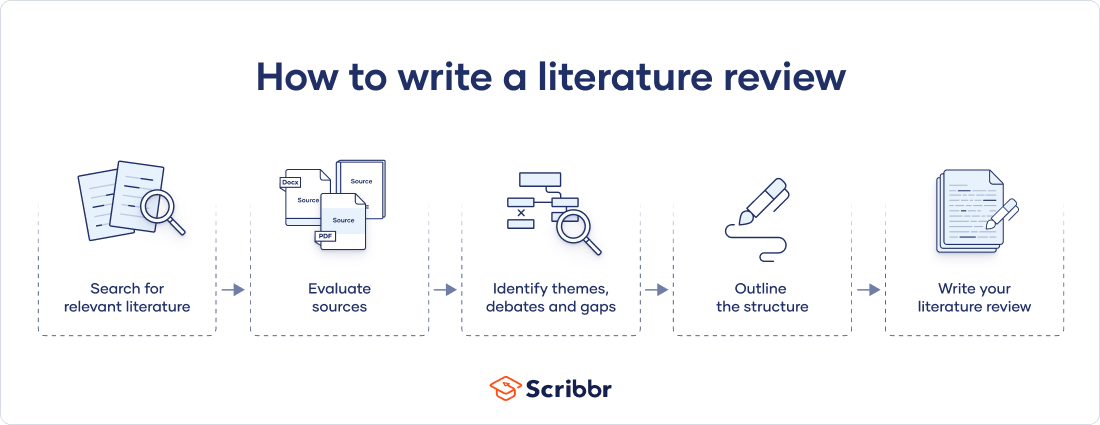
The only proofreading tool specialized in correcting academic writing
The academic proofreading tool has been trained on 1000s of academic texts and by native English editors. Making it the most accurate and reliable proofreading tool for students.
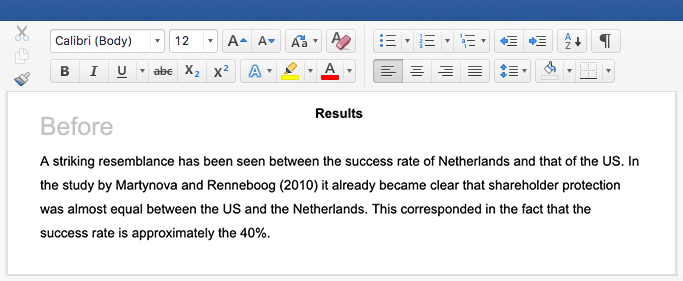
Correct my document today
Writing literature reviews can be quite challenging! A good starting point could be to look at some examples, depending on what kind of literature review you’d like to write.
- Example literature review #1: “Why Do People Migrate? A Review of the Theoretical Literature” ( Theoretical literature review about the development of economic migration theory from the 1950s to today.)
- Example literature review #2: “Literature review as a research methodology: An overview and guidelines” ( Methodological literature review about interdisciplinary knowledge acquisition and production.)
- Example literature review #3: “The Use of Technology in English Language Learning: A Literature Review” ( Thematic literature review about the effects of technology on language acquisition.)
- Example literature review #4: “Learners’ Listening Comprehension Difficulties in English Language Learning: A Literature Review” ( Chronological literature review about how the concept of listening skills has changed over time.)
You can also check out our templates with literature review examples and sample outlines at the links below.
Download Word doc Download Google doc
Before you begin searching for literature, you need a clearly defined topic .
If you are writing the literature review section of a dissertation or research paper, you will search for literature related to your research objectives and questions .
If you are writing a literature review as a stand-alone assignment, you will have to choose a focus and develop a central question to direct your search. Unlike a dissertation research question, this question has to be answerable without collecting original data. You should be able to answer it based only on a review of existing publications.
Make a list of keywords
Start by creating a list of keywords related to your research topic. Include each of the key concepts or variables you’re interested in, and list any synonyms and related terms. You can add to this list if you discover new keywords in the process of your literature search.
- Social media, Facebook, Instagram, Twitter, Snapchat, TikTok
- Body image, self-perception, self-esteem, mental health
- Generation Z, teenagers, adolescents, youth
Search for relevant sources
Use your keywords to begin searching for sources. Some databases to search for journals and articles include:
- Your university’s library catalogue
- Google Scholar
- Project Muse (humanities and social sciences)
- Medline (life sciences and biomedicine)
- EconLit (economics)
- Inspec (physics, engineering and computer science)
You can use boolean operators to help narrow down your search:
Read the abstract to find out whether an article is relevant to your question. When you find a useful book or article, you can check the bibliography to find other relevant sources.
To identify the most important publications on your topic, take note of recurring citations. If the same authors, books or articles keep appearing in your reading, make sure to seek them out.
You probably won’t be able to read absolutely everything that has been written on the topic – you’ll have to evaluate which sources are most relevant to your questions.
For each publication, ask yourself:
- What question or problem is the author addressing?
- What are the key concepts and how are they defined?
- What are the key theories, models and methods? Does the research use established frameworks or take an innovative approach?
- What are the results and conclusions of the study?
- How does the publication relate to other literature in the field? Does it confirm, add to, or challenge established knowledge?
- How does the publication contribute to your understanding of the topic? What are its key insights and arguments?
- What are the strengths and weaknesses of the research?
Make sure the sources you use are credible, and make sure you read any landmark studies and major theories in your field of research.
You can find out how many times an article has been cited on Google Scholar – a high citation count means the article has been influential in the field, and should certainly be included in your literature review.
The scope of your review will depend on your topic and discipline: in the sciences you usually only review recent literature, but in the humanities you might take a long historical perspective (for example, to trace how a concept has changed in meaning over time).
Remember that you can use our template to summarise and evaluate sources you’re thinking about using!
Take notes and cite your sources
As you read, you should also begin the writing process. Take notes that you can later incorporate into the text of your literature review.
It’s important to keep track of your sources with references to avoid plagiarism . It can be helpful to make an annotated bibliography, where you compile full reference information and write a paragraph of summary and analysis for each source. This helps you remember what you read and saves time later in the process.
You can use our free APA Reference Generator for quick, correct, consistent citations.
Prevent plagiarism, run a free check.
To begin organising your literature review’s argument and structure, you need to understand the connections and relationships between the sources you’ve read. Based on your reading and notes, you can look for:
- Trends and patterns (in theory, method or results): do certain approaches become more or less popular over time?
- Themes: what questions or concepts recur across the literature?
- Debates, conflicts and contradictions: where do sources disagree?
- Pivotal publications: are there any influential theories or studies that changed the direction of the field?
- Gaps: what is missing from the literature? Are there weaknesses that need to be addressed?
This step will help you work out the structure of your literature review and (if applicable) show how your own research will contribute to existing knowledge.
- Most research has focused on young women.
- There is an increasing interest in the visual aspects of social media.
- But there is still a lack of robust research on highly-visual platforms like Instagram and Snapchat – this is a gap that you could address in your own research.
There are various approaches to organising the body of a literature review. You should have a rough idea of your strategy before you start writing.
Depending on the length of your literature review, you can combine several of these strategies (for example, your overall structure might be thematic, but each theme is discussed chronologically).
Chronological
The simplest approach is to trace the development of the topic over time. However, if you choose this strategy, be careful to avoid simply listing and summarising sources in order.
Try to analyse patterns, turning points and key debates that have shaped the direction of the field. Give your interpretation of how and why certain developments occurred.
If you have found some recurring central themes, you can organise your literature review into subsections that address different aspects of the topic.
For example, if you are reviewing literature about inequalities in migrant health outcomes, key themes might include healthcare policy, language barriers, cultural attitudes, legal status, and economic access.
Methodological
If you draw your sources from different disciplines or fields that use a variety of research methods , you might want to compare the results and conclusions that emerge from different approaches. For example:
- Look at what results have emerged in qualitative versus quantitative research
- Discuss how the topic has been approached by empirical versus theoretical scholarship
- Divide the literature into sociological, historical, and cultural sources
Theoretical
A literature review is often the foundation for a theoretical framework . You can use it to discuss various theories, models, and definitions of key concepts.
You might argue for the relevance of a specific theoretical approach, or combine various theoretical concepts to create a framework for your research.
Like any other academic text, your literature review should have an introduction , a main body, and a conclusion . What you include in each depends on the objective of your literature review.
The introduction should clearly establish the focus and purpose of the literature review.
If you are writing the literature review as part of your dissertation or thesis, reiterate your central problem or research question and give a brief summary of the scholarly context. You can emphasise the timeliness of the topic (“many recent studies have focused on the problem of x”) or highlight a gap in the literature (“while there has been much research on x, few researchers have taken y into consideration”).
Depending on the length of your literature review, you might want to divide the body into subsections. You can use a subheading for each theme, time period, or methodological approach.
As you write, make sure to follow these tips:
- Summarise and synthesise: give an overview of the main points of each source and combine them into a coherent whole.
- Analyse and interpret: don’t just paraphrase other researchers – add your own interpretations, discussing the significance of findings in relation to the literature as a whole.
- Critically evaluate: mention the strengths and weaknesses of your sources.
- Write in well-structured paragraphs: use transitions and topic sentences to draw connections, comparisons and contrasts.
In the conclusion, you should summarise the key findings you have taken from the literature and emphasise their significance.
If the literature review is part of your dissertation or thesis, reiterate how your research addresses gaps and contributes new knowledge, or discuss how you have drawn on existing theories and methods to build a framework for your research. This can lead directly into your methodology section.
A literature review is a survey of scholarly sources (such as books, journal articles, and theses) related to a specific topic or research question .
It is often written as part of a dissertation , thesis, research paper , or proposal .
There are several reasons to conduct a literature review at the beginning of a research project:
- To familiarise yourself with the current state of knowledge on your topic
- To ensure that you’re not just repeating what others have already done
- To identify gaps in knowledge and unresolved problems that your research can address
- To develop your theoretical framework and methodology
- To provide an overview of the key findings and debates on the topic
Writing the literature review shows your reader how your work relates to existing research and what new insights it will contribute.
The literature review usually comes near the beginning of your dissertation . After the introduction , it grounds your research in a scholarly field and leads directly to your theoretical framework or methodology .
Cite this Scribbr article
If you want to cite this source, you can copy and paste the citation or click the ‘Cite this Scribbr article’ button to automatically add the citation to our free Reference Generator.
McCombes, S. (2022, June 07). What is a Literature Review? | Guide, Template, & Examples. Scribbr. Retrieved 8 July 2024, from https://www.scribbr.co.uk/thesis-dissertation/literature-review/
Is this article helpful?
Shona McCombes
Other students also liked, how to write a dissertation proposal | a step-by-step guide, what is a theoretical framework | a step-by-step guide, what is a research methodology | steps & tips.
Reference management. Clean and simple.
How to write a literature review in 6 steps
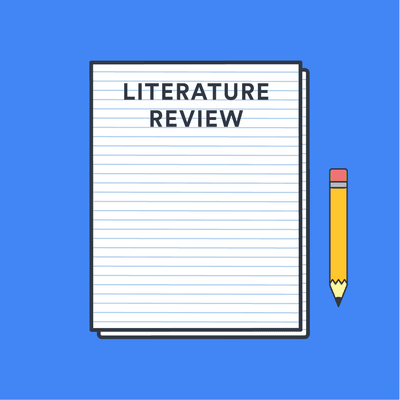
What is a literature review?
How to write a literature review, 1. determine the purpose of your literature review, 2. do an extensive search, 3. evaluate and select literature, 4. analyze the literature, 5. plan the structure of your literature review, 6. write your literature review, other resources to help you write a successful literature review, frequently asked questions about writing a literature review, related articles.
A literature review is an assessment of the sources in a chosen topic of research.
A good literature review does not just summarize sources. It analyzes the state of the field on a given topic and creates a scholarly foundation for you to make your own intervention. It demonstrates to your readers how your research fits within a larger field of study.
In a thesis, a literature review is part of the introduction, but it can also be a separate section. In research papers, a literature review may have its own section or it may be integrated into the introduction, depending on the field.
➡️ Our guide on what is a literature review covers additional basics about literature reviews.
- Identify the main purpose of the literature review.
- Do extensive research.
- Evaluate and select relevant sources.
- Analyze the sources.
- Plan a structure.
- Write the review.
In this section, we review each step of the process of creating a literature review.
In the first step, make sure you know specifically what the assignment is and what form your literature review should take. Read your assignment carefully and seek clarification from your professor or instructor if needed. You should be able to answer the following questions:
- How many sources do I need to include?
- What types of sources should I review?
- Should I evaluate the sources?
- Should I summarize, synthesize or critique sources?
- Do I need to provide any definitions or background information?
In addition to that, be aware that the narrower your topic, the easier it will be to limit the number of sources you need to read in order to get a good overview of the topic.
Now you need to find out what has been written on the topic and search for literature related to your research topic. Make sure to select appropriate source material, which means using academic or scholarly sources , including books, reports, journal articles , government documents and web resources.
➡️ If you’re unsure about how to tell if a source is scholarly, take a look at our guide on how to identify a scholarly source .
Come up with a list of relevant keywords and then start your search with your institution's library catalog, and extend it to other useful databases and academic search engines like:
- Google Scholar
- Science.gov
➡️ Our guide on how to collect data for your thesis might be helpful at this stage of your research as well as the top list of academic search engines .
Once you find a useful article, check out the reference list. It should provide you with even more relevant sources. Also, keep a note of the:
- authors' names
- page numbers
Keeping track of the bibliographic information for each source will save you time when you’re ready to create citations. You could also use a reference manager like Paperpile to automatically save, manage, and cite your references.
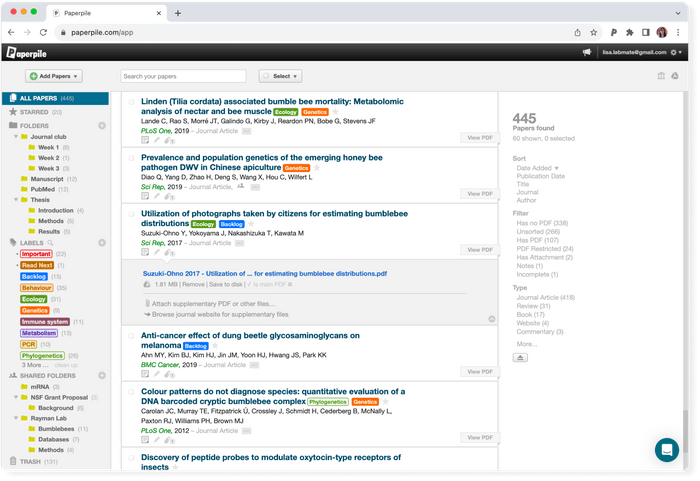
Read the literature. You will most likely not be able to read absolutely everything that is out there on the topic. Therefore, read the abstract first to determine whether the rest of the source is worth your time. If the source is relevant for your topic:
- Read it critically.
- Look for the main arguments.
- Take notes as you read.
- Organize your notes using a table, mind map, or other technique.
Now you are ready to analyze the literature you have gathered. While your are working on your analysis, you should ask the following questions:
- What are the key terms, concepts and problems addressed by the author?
- How is this source relevant for my specific topic?
- How is the article structured? What are the major trends and findings?
- What are the conclusions of the study?
- How are the results presented? Is the source credible?
- When comparing different sources, how do they relate to each other? What are the similarities, what are the differences?
- Does the study help me understand the topic better?
- Are there any gaps in the research that need to be filled? How can I further my research as a result of the review?
Tip: Decide on the structure of your literature review before you start writing.
There are various ways to organize your literature review:
- Chronological method : Writing in the chronological method means you are presenting the materials according to when they were published. Follow this approach only if a clear path of research can be identified.
- Thematic review : A thematic review of literature is organized around a topic or issue, rather than the progression of time.
- Publication-based : You can order your sources by publication, if the way you present the order of your sources demonstrates a more important trend. This is the case when a progression revealed from study to study and the practices of researchers have changed and adapted due to the new revelations.
- Methodological approach : A methodological approach focuses on the methods used by the researcher. If you have used sources from different disciplines that use a variety of research methods, you might want to compare the results in light of the different methods and discuss how the topic has been approached from different sides.
Regardless of the structure you chose, a review should always include the following three sections:
- An introduction, which should give the reader an outline of why you are writing the review and explain the relevance of the topic.
- A body, which divides your literature review into different sections. Write in well-structured paragraphs, use transitions and topic sentences and critically analyze each source for how it contributes to the themes you are researching.
- A conclusion , which summarizes the key findings, the main agreements and disagreements in the literature, your overall perspective, and any gaps or areas for further research.
➡️ If your literature review is part of a longer paper, visit our guide on what is a research paper for additional tips.
➡️ UNC writing center: Literature reviews
➡️ How to write a literature review in 3 steps
➡️ How to write a literature review in 30 minutes or less
The goal of a literature review is to asses the state of the field on a given topic in preparation for making an intervention.
A literature review should have its own independent section. You should indicate clearly in the table of contents where it can be found, and address this section as “Literature Review.”
There is no set amount of words for a literature review; the length depends on the research. If you are working with a large amount of sources, then it will be long. If your paper does not depend entirely on references, then it will be short.
Most research papers include a literature review. By assessing the available sources in your field of research, you will be able to make a more confident argument about the topic.
Literature reviews are most commonly found in theses and dissertations. However, you find them in research papers as well.
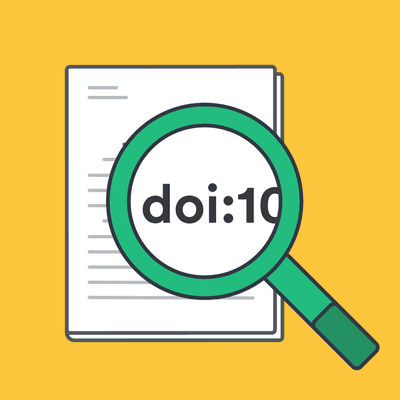

Literature Reviews: 5. Write the Review
- Library Basics
- 1. Choose Your Topic
- How to Find Books
- Types of Clinical Study Designs
- Types of Literature
- 3. Search the Literature
- 4. Read & Analyze the Literature
- 5. Write the Review
- Keeping Track of Information
- Style Guides
- Books, Tutorials & Examples
Qualities of A Good Lit Review
| A good literature review is NOT simply a list describing or summarizing several articles; a literature review is discursive prose which proceeds to a conclusion by reason or argument. A good literature review shows signs of synthesis and understanding of the topic. There should be strong evidence of analytical thinking shown through the connections you make between the literature being reviewed. |
Create an Outline, Then Summarize & Synthesize
Draft an outline for your review. Read more about developing an outline here at the Purdue OWL site.
Summarize & Synthesize
- Place each work in the context of its contribution to the understanding of the topic.
- Describe the relationship of each work to the others under consideration, noting contradictory studies.
- Identify areas of prior scholarship to prevent duplication of effort.
- Point the way forward for further research.
Choose How to Organize Your Paper
Before writing, you should consider a few different ways of organizing or categorizing the literature you've looked at and consider prioritizing the readings, or grouping them by methodology or theme.
1. Chronologically – Organizing your sources by the date of publication can show how scholarly perspective on a topic has changed over time.
2. Thematically – Organizing by theme puts all of the sources with a similar focus together, making it very easy to see where differences in perspective emerge.
3. Methodologically – Organizing by method, much like organizing by theme, puts similar sources together and illustrates what effect method has on final product.
At a loss for words?
- Academic Phrasebank Check out this phrasebank of terms and phrases to use in your research papers.
Elements of the Literature Review
There are many different ways to organize your references in a literature review, but most reviews contain certain basic elements.
Objectives - Clearly describe the purpose of the paper and state your objectives in completing the literature review.
Background/Introduction – Give an overview of your research topic and what prompted it.
Methods - Describe step by step how your performed your evaluation of the materials.
Discussion/Body - The body contains the evaluation or synthesis of the materials. Discuss and compare common themes and gaps in the literature. You may also want to include a section on "questions for further research" and discuss what issues the review has sparked about the topic/field or offer suggestions for future studies that build on your current findings.
Conclusion – A summary of your analysis and evaluation of the reviewed works and how it is related to its parent discipline, scientific endeavor, or profession.
Bibliography - A list of the papers you discussed, aka References. To learn more about different citation styles, visit the "Manage References and Citations" tab.
Writing Tips
Once you actually begin to write the review, stick to your outline and keep these tips in mind:
- Consider your audience. Are you engaging with specialists in one discipline? Or generalists in more than one discipline?
- When writing for a more general audience, avoid jargon and strive for "plain English."
- When writing for a very specialized audience, consider using "plain English" anyway.
- Short paragraphs are easier to read than long paragraphs.
- Subheadings and subsections can help to underscore the structure of your review.
- Do more than just summarize the readings. A lit review is not an annotated bibliography.
- Resist the temptation to refer to *all* the readings you've evaluated. To begin with, focus on readings you've identified as essential or representative.
- Tell the reader how the literature intersects with your project -- how your project complements the existing literature.
- Be objective.
- << Previous: 4. Read & Analyze the Literature
- Next: Keeping Track of Information >>
- Last Updated: Dec 29, 2023 11:41 AM
- URL: https://research.library.gsu.edu/litrev


Literature Review - what is a Literature Review, why it is important and how it is done
- Strategies to Find Sources
Evaluating Literature Reviews and Sources
Reading critically, tips to evaluate sources.
- Tips for Writing Literature Reviews
- Writing Literature Review: Useful Sites
- Citation Resources
- Other Academic Writings
- Useful Resources
A good literature review evaluates a wide variety of sources (academic articles, scholarly books, government/NGO reports). It also evaluates literature reviews that study similar topics. This page offers you a list of resources and tips on how to evaluate the sources that you may use to write your review.
- A Closer Look at Evaluating Literature Reviews Excerpt from the book chapter, “Evaluating Introductions and Literature Reviews” in Fred Pyrczak’s Evaluating Research in Academic Journals: A Practical Guide to Realistic Evaluation , (Chapter 4 and 5). This PDF discusses and offers great advice on how to evaluate "Introductions" and "Literature Reviews" by listing questions and tips. First part focus on Introductions and in page 10 in the PDF, 37 in the text, it focus on "literature reviews".
- Tips for Evaluating Sources (Print vs. Internet Sources) Excellent page that will guide you on what to ask to determine if your source is a reliable one. Check the other topics in the guide: Evaluating Bibliographic Citations and Evaluation During Reading on the left side menu.
To be able to write a good Literature Review, you need to be able to read critically. Below are some tips that will help you evaluate the sources for your paper.
Reading critically (summary from How to Read Academic Texts Critically)
- Who is the author? What is his/her standing in the field.
- What is the author’s purpose? To offer advice, make practical suggestions, solve a specific problem, to critique or clarify?
- Note the experts in the field: are there specific names/labs that are frequently cited?
- Pay attention to methodology: is it sound? what testing procedures, subjects, materials were used?
- Note conflicting theories, methodologies and results. Are there any assumptions being made by most/some researchers?
- Theories: have they evolved overtime?
- Evaluate and synthesize the findings and conclusions. How does this study contribute to your project?
Useful links:
- How to Read a Paper (University of Waterloo, Canada) This is an excellent paper that teach you how to read an academic paper, how to determine if it is something to set aside, or something to read deeply. Good advice to organize your literature for the Literature Review or just reading for classes.
Criteria to evaluate sources:
- Authority : Who is the author? what is his/her credentials--what university he/she is affliliated? Is his/her area of expertise?
- Usefulness : How this source related to your topic? How current or relevant it is to your topic?
- Reliability : Does the information comes from a reliable, trusted source such as an academic journal?
Useful site - Critically Analyzing Information Sources (Cornell University Library)
- << Previous: Strategies to Find Sources
- Next: Tips for Writing Literature Reviews >>
- Last Updated: Jul 3, 2024 10:56 AM
- URL: https://lit.libguides.com/Literature-Review
The Library, Technological University of the Shannon: Midwest

How To Find A-Grade Literature For Review
Sourcing, evaluating and organising.
By: David Phair (PhD) and Peter Quella (PhD) | January 2022
As we’ve discussed previously on our blog and YouTube channel, the first step of the literature review process is to source high-quality , relevant resources for your review, and to catalogue these pieces of literature in a systematic way so that you can digest and synthesise all the content efficiently.
In this article, we’ll look discuss 6 important things to keep in mind for the initial stage of your literature review so that you can source high-quality, relevant resources, quickly and efficiently. Let’s get started!
Overview: Literature Review Sourcing
- Develop and follow a clear literature search strategy
- Understand and use different types of literature correctly
- Carefully evaluate the quality of your potential sources
- Use a reference manager and a literature catalogue
- Read as broadly and comprehensively as possible
- Keep your golden thread front of mind throughout the process
1. Have a clear literature search strategy
As with any task in the research process, you need to have a clear plan of action before you get started, or you’ll end up wasting a lot of time and energy. So, before you begin your literature review , it’s useful to develop a simple search strategy . Broadly speaking, a good literature search strategy should include the following steps:
Step one – Clearly identify your golden thread
Your golden thread consists of your research aims , research objectives and research questions . These three components should be tightly aligned to form the focus of your research. If you’re unclear what your research aims and research questions are, you’re not going to have a clear direction when trying to source literature. As a result, you’re going to waste a lot of time reviewing irrelevant resources.
So, make sure that you have clarity regarding your golden thread before you start searching for literature. Of course, your research aims, objectives and questions may evolve or shift as a result of the literature review process (in fact, this is quite common), but you still need to have a clear focus to get things started.
Step two – Develop a keyword/keyphrase list
Once you’ve clearly articulated your golden thread in terms of the research aims, objectives and questions, the next step is to develop a list of keywords or keyphrases, based on these three elements (the golden thread). You’ll also want to include synonyms and alternative spellings (for example, American vs British English) in your list.
For example, if your research aims and research questions involve investigating organisational trust , your keyword list might include:
- Organisational trust
- Organizational trust (US spelling)
- Consumer trust
- Brand trust
- Online trust
When it comes to brainstorming keywords, the more the better . Don’t hold back at this stage. You’ll quickly find out which ones are useful, and which aren’t when you start searching. So, it’s best to just go as broad as possible here to ensure you cast a wide net.
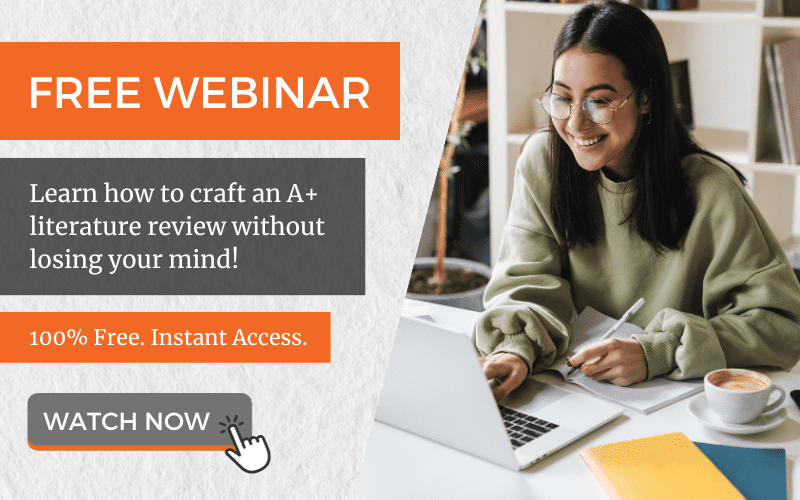
Step three – Identify the relevant databases
Now that you’ve got a comprehensive set of keywords, the next step is to identify which literature databases will be most useful and relevant for your particular study. There are hundreds, if not thousands of databases out there, and they are often subject or discipline-specific . For example, within the medicine space, Medline is a popular one.
To identify relevant databases, it’s best to speak to your research advisor/supervisor, Grad Coach or a librarian at your university library. Oftentimes, a quick chat with a skilled librarian can yield tremendous insight. Don’t be shy to ask – chances are, they’ll be thrilled that you asked!
At this stage, you might be asking, “why not just use Google Scholar?”. Of course, an academic search engine like Google Scholar will be useful in terms of getting started and finding a broad range of resources, but it won’t always present every possible resource or the best quality resources. It also has limited filtering options compared to some of the specialist databases, so you shouldn’t rely purely on Google Scholar.
Step four – Use Boolean operators to refine your search
Once you’ve identified your keywords and databases, it’s time to start searching for literature – hooray! However, you’ll quickly find that there is a seemingly endless number of journal articles to sift through, and you have limited time to work through the literature. So, you’ll need to get smart about how you use these databases – enter Boolean operators.
Boolean operators are special characters that allow you to refine your search. Common operators include:
- AND – only show results that contain both X and Y
- OR – show results that contain X or Y
- NOT – show results that include X, but not Y
These operators are incredibly useful, especially when there are topics that are very similar to yours but are not relevant . For example, if you’re researching something about the growth of apples, you’ll want to exclude all literature related to Apple, the company. Boolean operators allow you to cut out the irrelevant content and improve the signal to noise ratio in your search.
Need a helping hand?
2. Use different types of literature correctly
Once you start searching for literature, you’ll quickly notice that there are different “types” of resources that come up. It’s important to understand the different types of literature available to you and how to use each of them appropriately.
Generally speaking, you’ll find three categories of literature:
Primary literature
Secondary literature
Tertiary literature
Primary literature refers to journal articles , typically peer reviewed, which document a study that was undertaken, where data were collected and analysed, and findings were discussed. For example, a journal article that involves the collection and analysis of survey data to identify differences in personality between two groups of people.
Primary literature should, ideally, form the foundation of your literature review – the bread and butter, so to speak. You’ll likely refer to many of the arguments made and findings identified in these types of articles to build your own arguments throughout your literature review. You’ll also rely on these types of articles for theoretical models and frameworks, which may form the foundation of your own proposed framework, depending on the nature of your research.
Lastly, primary literature can be a useful source of measurement scales for quantitative studies. For example, many journal articles will include a copy of the survey measures they used at the end of the article, which will typically be reliable and valid. You can either use these “as is” or as a foundation for your own survey measures .
So, long story short, you’ll need a good stockpile of these types of resources. They are, admittedly, more “dense” and challenging to digest than the other types of literature, but taking the time to work through them will pay off greatly.
Secondary literature refers to journal articles that summarise and integrate the findings from primary literature. For example, you’ll likely find “review of the literature” type journal articles which provide an overview of the current state of the research (at the time of publication, of course).
Secondary literature is very useful for orienting yourself with regards to the current state of knowledge and identifying key researchers , seminal works and so on. In other words, they’re a good tool to make sure you’ve got a broad, comprehensive view of what all is out there. They’re not going to give you the level of detail that primary literature will (and they’ll likely be a bit outdated), but they’ll point you in the right direction.
In practical terms, it’s a good idea to start by reviewing secondary literature-type articles to help you get a bird’s eye view of the landscape and then dive deeper into the primary literature to get a grasp of the specifics and to bring your knowledge up to date with the most current studies.
The final category of literature refers to sources that would be considered less academic and scientifically rigorous in nature, but up to date and highly relevant. For example, sources such as current industry and country reports published by management consulting groups, news articles, blog posts and so on.
While these sources are not as credible and trustworthy as journal articles (especially peer-reviewed ones), they can provide very up to date information , whereas academic research tends to roll out quite slowly. Therefore, they can be very useful for contextualising your research topic and/or demonstrating a current trend. Quite often, you’d cite these types of sources in your introduction chapter rather than your literature review chapter, but you may still have use for them in the latter.
In summary, it’s important to understand the three different types of literature – primary, secondary and tertiary, and use them appropriately in your dissertation, thesis or research project.
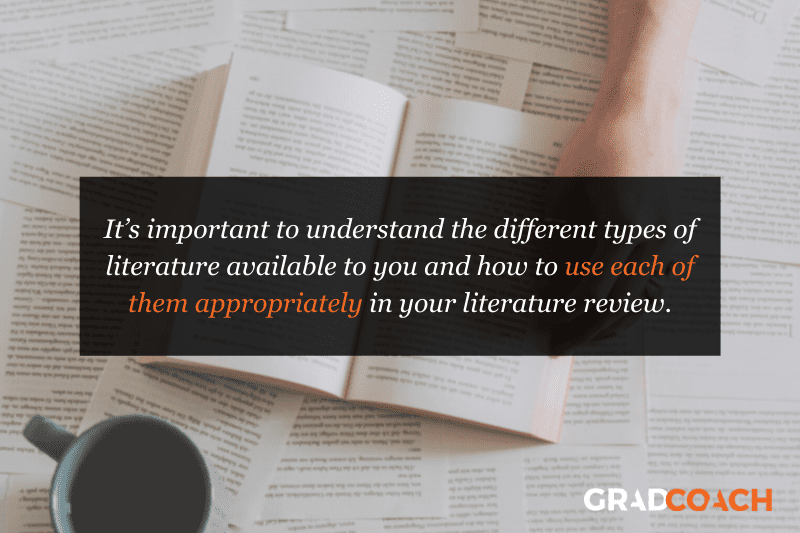
3. Carefully evaluate the quality of your sources
As we’ve alluded to, not all literature is created equally. Not only does literature vary in terms of type (i.e., primary vs secondary), it also varies in terms of overall quality .
Simply put, all sources exist on a quality spectrum . On the high end of the spectrum are peer-reviewed articles published in popular, credible journals. Next are journal articles that are not peer-reviewed, or that are published in lower quality or lesser-known journals. In the middle are sources like textbooks and reports by professional organisations (e.g., management consulting firms). On the low end are sources like newspapers, blog posts and social media posts.
As you can probably see, this loosely reflects the categories we mentioned previously (primary, secondary and tertiary literature), so there is once again a trade-off between quality and recency . Therefore, you need to carefully evaluate the quality of each potential source and let this inform how you use it in your literature review. Importantly, this doesn’t mean that you can’t include a newspaper article or blog post as a source – it just means that you shouldn’t rely too heavily on these types of courses as the core of your argument.
When evaluating journal articles, you can consider their citation count (i.e., the number of other articles that reference them) as a quality indicator. But keep in mind that citation count is a product of many factors , including the popularity of the article, the popularity of the research field and most importantly, time. In other words, it’s natural for newer articles to have lower citation counts. This is useful to keep in mind, as you ideally want to focus on more recent literature (published within the last 3-5 years) in your literature review.
In summary, aim to focus on higher-quality literature , especially when you’re building core arguments in your literature review. You don’t, for example, want to make an argument regarding the importance and novelty of your research (i.e., its justification) based on some blogger’s opinion.
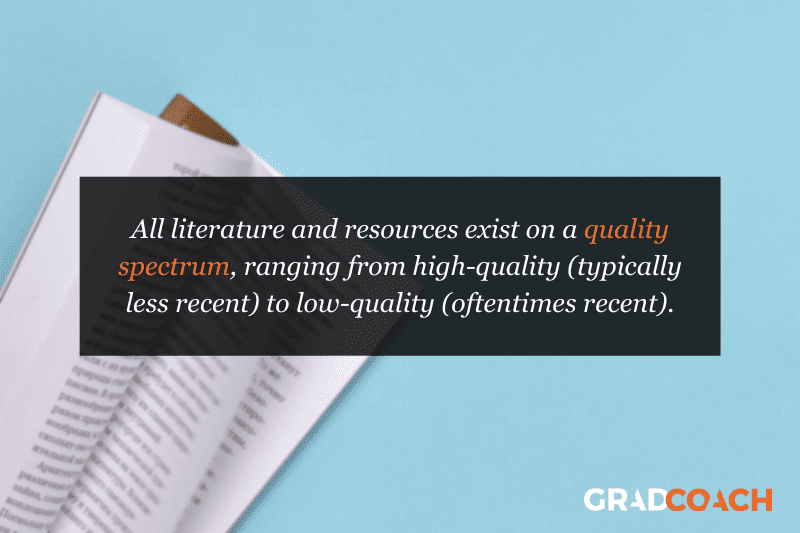
4. Use a reference manager and literature catalogue
As you review the literature and build your collection of potential sources, you’ll need a way to stay on top of all the details. To this end, it’s essential that you make use of both a reference manager and a literature catalogue . Let’s take a look at each of these.
The reference manager
Reference management software helps you store the reference information for each of your articles and manages the citation and reference list building task as you write up your actual literature review chapter. In other words, a reference manager ensures that your citations and reference list are correctly formatted in the reference style required by your university – e.g., Harvard, APA , MLA, etc.
Using a reference manager saves you the hassle of trying to manually type out your in-text citations and reference list, which you’re bound to mess up in some way. A simple comma out of place, incorrect italicisation or boldfacing can result in you losing marks, and that’s highly likely when you’re dealing with a large number of references. So, it just makes sense to use a piece of software for this task.
The good news is that there are loads of options , many of which are free . For new researchers, we usually recommend Mendeley or Zotero . So, don’t waste your time trying to manage your references manually – get yourself a reference manager ASAP.
The literature catalogue
The second tool you’ll need is a literature catalogue. This is simply an Excel document that you can easily compile yourself (or download our free one here ), where you list and categorise all your literature. You might doubt whether it’s really necessary to have a separate catalogue when you’ve already logged your reference data in a reference manager, but trust us, you’re going to need it. It’s quite common that throughout the literature review process, you’ll review hundreds of articles , so it’s simply impossible that you’ll remember all the details.
What makes a literature catalogue extremely powerful is that you can store as much information as you want for each piece of literature that you include (whereas a reference manager only includes basic fields). Typically, you would include things like:
- Title of the article
- One-line summary of the research
- Key findings and takeaways
- Context (i.e. where did it take place)
- Useful quotes
- Methodology (e.g. qualitative, quantitative or mixed methods)
- Category (you can customise as many categories as makes sense for you)
- Quality of resource
- Type of literature (e.g. primary, secondary or tertiary)
These are just some examples – ultimately you need to customise your catalogue to suit your needs. But, as you can see, the more detailed you get, the more useful your catalogue will become when it’s time to synthesise the research and write up your literature. For example, you could quickly filter the catalogue to display all papers that support a certain hypothesis, that argue in a specific direction, or that were written at a certain time.
5. Read widely (and efficiently)
As we’ve discussed in other posts , the purpose of the literature review chapter is to present and synthesise the current state of knowledge in relation to your research aims, objectives and research questions. To do this, you’ll need to read as broadly and comprehensively as possible. You’ll need to demonstrate to your marker that you “know your stuff” and have a strong understanding of the relevant literature.
Ideally, your literature review should include an eclectic mix of research that features multiple perspectives . In other words, you need to avoid getting tunnel vision and running down one narrow stream of literature. Ideally, you want to highlight both the agreements and disagreements in the literature to show that you’ve got a well-balanced view of the situation.
If your topic is particularly novel and there isn’t a lot of literature available, you can focus your efforts on adjacent literature . For example, if you’re researching factors that cultivate organisational trust in Germany, but there’s very little literature on this, you can draw on US and UK-based studies to form your theoretical foundation. Similarly, if you’re investigating an occurrence in an under-researched industry, you can look at other industries for literature.
As you read each journal article, be sure to scan the reference list for further reading (this technique is called “snowballing”). By doing this, you will quickly identify key literature within a topic area and fast-track your literature review process. You can also check which articles have cited any given article using Google Scholar, which will give you a “forward view” in terms of the progress of the literature.
Given that you’ll need to work through a large amount of literature, it’s useful to adopt a “strategic skimming ” approach when you’re initially assessing articles, so that you don’t need to read the entire journal article . In practical terms, this means you can focus on just the title and abstract at first, and if the article seems relevant based on those, you can jump to the findings section and limitations section . These sections will give you a solid indicator as to whether the resource is relevant to your study, which you can then shortlist for full reading.
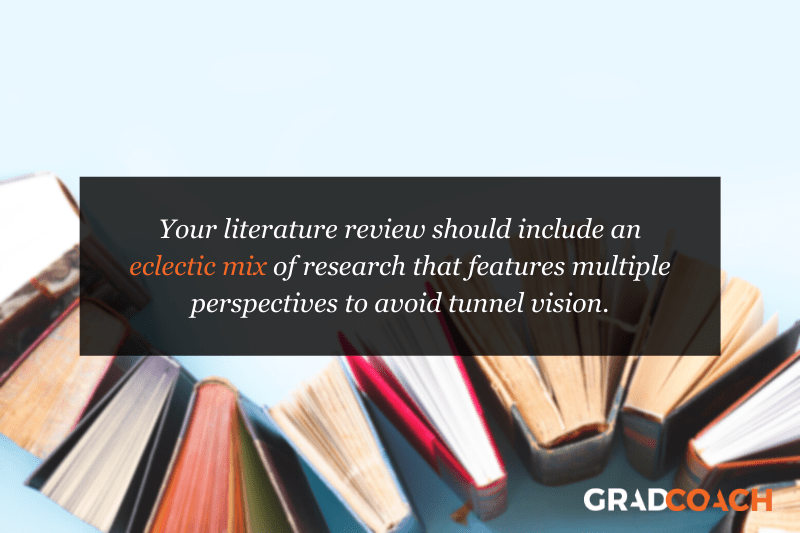
6. Keep your golden thread front of mind
Your golden thread (i.e., your research aims, objectives and research questions) needs to guide every decision you make throughout your dissertation, thesis, or research project. This is especially true in the literature review stage, as the golden thread should act as a litmus test for relevance whenever you’re reviewing potential articles or resources. In other words, if an article doesn’t relate to your golden thread, its probably not worth spending time on.
Keep in mind that your research aims, objectives and research questions may evolve as a result of the literature review process. For example, you may find that after reviewing the literature in more depth, your topic focus is not as novel as you originally thought, or that there’s an adjacent area that is more deserving of investigation. This is perfectly natural, so don’t be surprised if your focus shifts somewhat during the review process. Just remember to update your literature review in this case and be sure to update any previous chapters so that your document has a consistent focus throughout.
Wrapping up
In this article, we covered 6 pointers to help you find and evaluate high-quality resources for your literature review. To recap:
- Understand and use different types of literature for the right purpose
If you have any questions, please feel free to leave a comment . Alternatively, if you’d like hands-on help with your literature review, be sure to check out our 1-on-1 private coaching services here.
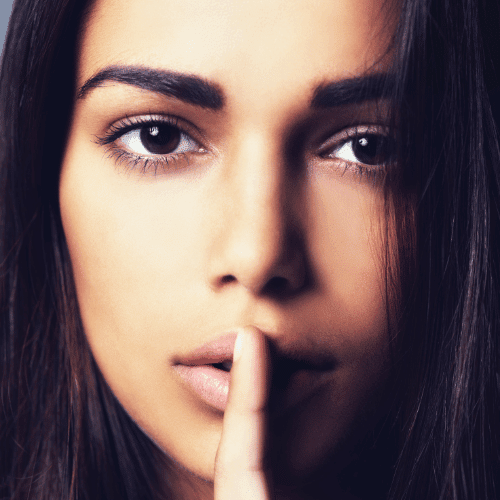
Psst… there’s more!
This post is an extract from our bestselling short course, Literature Review Bootcamp . If you want to work smart, you don't want to miss this .
You Might Also Like:
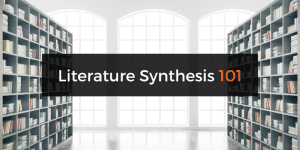
this is very helpful to any researcher, I am learning this for the benefit of myself and overs as a library staff
Of course this is useful to most of researchers. I have learnt a lot issues which are relevant to teaching research. Surely they will enjoy my research sessions.
Thank you. Very clear and concise
Submit a Comment Cancel reply
Your email address will not be published. Required fields are marked *
Save my name, email, and website in this browser for the next time I comment.
- Print Friendly
Research in International Management |

Doing a literature review: an 8-step process
Overview of my presentation in the Middlesex University PhD coursework - with embedded videos of the 8 steps
Anne-Wil Harzing - Mon 1 Jan 2024 09:31 (updated Tue 2 Jan 2024 08:14)
Copyright 2024 Anne-Wil Harzing. All rights reserved. First version, 2 January 2024

Step 1: Information management strategies
Step 2: situating the literature review, step 3: sources of literature, step 4: keeping current, step 5: how much is enough, step 6: different types of papers.
- Step 7a: Seven criteria to evaluate coverage
- Step 7b: Twelve guidelines to evaluate references
Step 8: The literature review in your thesis
Your challenge is very different from what mine was during my PhD. I completed my PhD before the internet was available. So my challenge was to get access to information and find the time and money to do so. Oftentimes, this involved traveling to various libraries across the country.
Your challenge is to manage the wealth of information you have easy access to, but not to waste too much time on completely irrelevant information. This step provides you with some tips on how to approach this.
In this step, you learn why a literature review is important in no less than six of the nine stages of the research process. Before watching it, try and list the stages where you think it might be important.
In this step, we review the various sources you can use for literature reviews: books, journal articles, government and industry resources, working papers, and conference papers. I show you the relative merits of each of these.
Here I share my top tips on how to keep up to date with new publications. You can find more information about this here: How to keep up-to-date with the literature, but avoid information overload? .
Note that my tips focus on the "old-fashioned" tried-and-tested approaches. This process has now been facilitated by many dedicated tools, often using artificial intelligence. I can't say I like working with these as they do not facilitate the deep engagement that I think is needed for academic research, but they might well work for you.
The next step is deciding when to stop. How do you know you have "enough"? When can you stop? Well obviously, you never completely stop reviewing the literature, as it is important in so many stages of the review process (see step 2).
But in deciding when you can start writing up, I do suggest the use of a relevance tree in this video. I also show how you can use tables to effectively summarise literature. Further tips on how many references to use in writing up can be found here: How many references is enough?
In this step, I review three types of papers to look out for to maximise the effectiveness of your literature review: review papers, star papers, model papers.
Step 7a: 7 criteria to evaluate coverage
How do you evaluate whether all the literature you have collected is actually useful for your thesis or article?
In this step I go through seven criteria you can use to evaluate coverage of the collected literature: relevance, currency, reliability, audience, accuracy, scope, and objectivity.
Step 7b: 12 guidelines to evaluate references
If you are going to use the literature to reference arguments in your thesis, this step covers twelve guidelines you can use to evaluate other academics' referencing practices as well as to make sure you do this right. They are also described in detail in this blogpost: Are referencing errors undermining our scholarship and credibility?
My twelve guidelines are based on research in my own PhD, written up as a paper that turned out to be very hard to publish. If you are interested, you can read the full story here: How to publish an unusual paper? Referencing errors, scholarship & credibility .
This last step reveals what criteria are used to evaluate the literature review in your own thesis: synthesis, critical appraisal, and application to the research question. I also explain what your literature should not look like and why a good literature review helps you to get papers published.
Related blogposts
- Want to publish a literature review? Think of it as an empirical paper
- Do you really want to publish your literature review? Advice for PhD students
- How to keep up-to-date with the literature, but avoid information overload?
- Is a literature review publication a low-cost project?
- Using Publish or Perish to do a literature review
- How to conduct a longitudinal literature review?
- New: Publish or Perish now also exports abstracts
- A framework for your literature review article: where to find one?
Find the resources on my website useful?
I cover all the expenses of operating my website privately. If you enjoyed this post and want to support me in maintaining my website, consider buying a copy of one of my books (see below) or supporting the Publish or Perish software .
Aug 2022: | Nov 2022: | Feb 2023: | May 2023: |
Copyright © 2024 Anne-Wil Harzing . All rights reserved. Page last modified on Tue 2 Jan 2024 08:14

Anne-Wil Harzing's profile and contact details >>

15 Literature Review Examples
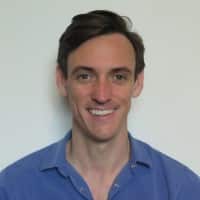
Chris Drew (PhD)
Dr. Chris Drew is the founder of the Helpful Professor. He holds a PhD in education and has published over 20 articles in scholarly journals. He is the former editor of the Journal of Learning Development in Higher Education. [Image Descriptor: Photo of Chris]
Learn about our Editorial Process
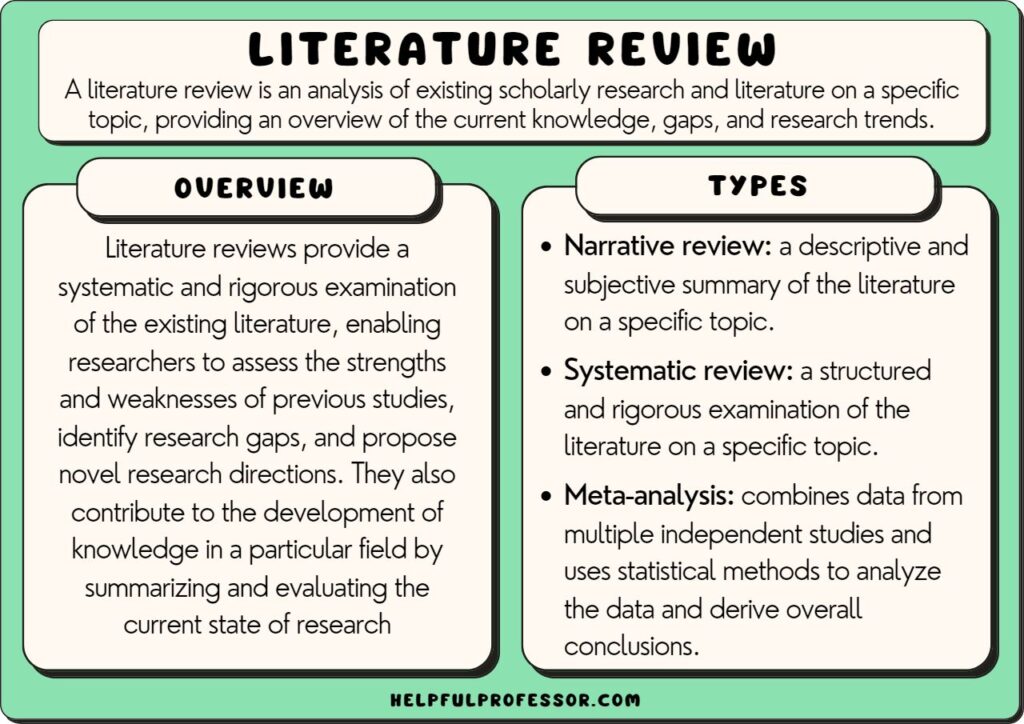
Literature reviews are a necessary step in a research process and often required when writing your research proposal . They involve gathering, analyzing, and evaluating existing knowledge about a topic in order to find gaps in the literature where future studies will be needed.
Ideally, once you have completed your literature review, you will be able to identify how your research project can build upon and extend existing knowledge in your area of study.
Generally, for my undergraduate research students, I recommend a narrative review, where themes can be generated in order for the students to develop sufficient understanding of the topic so they can build upon the themes using unique methods or novel research questions.
If you’re in the process of writing a literature review, I have developed a literature review template for you to use – it’s a huge time-saver and walks you through how to write a literature review step-by-step:
Get your time-saving templates here to write your own literature review.
Literature Review Examples
For the following types of literature review, I present an explanation and overview of the type, followed by links to some real-life literature reviews on the topics.
1. Narrative Review Examples
Also known as a traditional literature review, the narrative review provides a broad overview of the studies done on a particular topic.
It often includes both qualitative and quantitative studies and may cover a wide range of years.
The narrative review’s purpose is to identify commonalities, gaps, and contradictions in the literature .
I recommend to my students that they should gather their studies together, take notes on each study, then try to group them by themes that form the basis for the review (see my step-by-step instructions at the end of the article).
Example Study
Title: Communication in healthcare: a narrative review of the literature and practical recommendations
Citation: Vermeir, P., Vandijck, D., Degroote, S., Peleman, R., Verhaeghe, R., Mortier, E., … & Vogelaers, D. (2015). Communication in healthcare: a narrative review of the literature and practical recommendations. International journal of clinical practice , 69 (11), 1257-1267.
Source: https://onlinelibrary.wiley.com/doi/pdf/10.1111/ijcp.12686
Overview: This narrative review analyzed themes emerging from 69 articles about communication in healthcare contexts. Five key themes were found in the literature: poor communication can lead to various negative outcomes, discontinuity of care, compromise of patient safety, patient dissatisfaction, and inefficient use of resources. After presenting the key themes, the authors recommend that practitioners need to approach healthcare communication in a more structured way, such as by ensuring there is a clear understanding of who is in charge of ensuring effective communication in clinical settings.
Other Examples
- Burnout in United States Healthcare Professionals: A Narrative Review (Reith, 2018) – read here
- Examining the Presence, Consequences, and Reduction of Implicit Bias in Health Care: A Narrative Review (Zestcott, Blair & Stone, 2016) – read here
- A Narrative Review of School-Based Physical Activity for Enhancing Cognition and Learning (Mavilidi et al., 2018) – read here
- A narrative review on burnout experienced by medical students and residents (Dyrbye & Shanafelt, 2015) – read here
2. Systematic Review Examples
This type of literature review is more structured and rigorous than a narrative review. It involves a detailed and comprehensive plan and search strategy derived from a set of specified research questions.
The key way you’d know a systematic review compared to a narrative review is in the methodology: the systematic review will likely have a very clear criteria for how the studies were collected, and clear explanations of exclusion/inclusion criteria.
The goal is to gather the maximum amount of valid literature on the topic, filter out invalid or low-quality reviews, and minimize bias. Ideally, this will provide more reliable findings, leading to higher-quality conclusions and recommendations for further research.
You may note from the examples below that the ‘method’ sections in systematic reviews tend to be much more explicit, often noting rigid inclusion/exclusion criteria and exact keywords used in searches.
Title: The importance of food naturalness for consumers: Results of a systematic review
Citation: Roman, S., Sánchez-Siles, L. M., & Siegrist, M. (2017). The importance of food naturalness for consumers: Results of a systematic review. Trends in food science & technology , 67 , 44-57.
Source: https://www.sciencedirect.com/science/article/pii/S092422441730122X
Overview: This systematic review included 72 studies of food naturalness to explore trends in the literature about its importance for consumers. Keywords used in the data search included: food, naturalness, natural content, and natural ingredients. Studies were included if they examined consumers’ preference for food naturalness and contained empirical data. The authors found that the literature lacks clarity about how naturalness is defined and measured, but also found that food consumption is significantly influenced by perceived naturalness of goods.
- A systematic review of research on online teaching and learning from 2009 to 2018 (Martin, Sun & Westine, 2020) – read here
- Where Is Current Research on Blockchain Technology? (Yli-Huumo et al., 2016) – read here
- Universities—industry collaboration: A systematic review (Ankrah & Al-Tabbaa, 2015) – read here
- Internet of Things Applications: A Systematic Review (Asghari, Rahmani & Javadi, 2019) – read here
3. Meta-analysis
This is a type of systematic review that uses statistical methods to combine and summarize the results of several studies.
Due to its robust methodology, a meta-analysis is often considered the ‘gold standard’ of secondary research , as it provides a more precise estimate of a treatment effect than any individual study contributing to the pooled analysis.
Furthermore, by aggregating data from a range of studies, a meta-analysis can identify patterns, disagreements, or other interesting relationships that may have been hidden in individual studies.
This helps to enhance the generalizability of findings, making the conclusions drawn from a meta-analysis particularly powerful and informative for policy and practice.
Title: Cholesterol and Alzheimer’s Disease Risk: A Meta-Meta-Analysis
Citation: Sáiz-Vazquez, O., Puente-Martínez, A., Ubillos-Landa, S., Pacheco-Bonrostro, J., & Santabárbara, J. (2020). Cholesterol and Alzheimer’s disease risk: a meta-meta-analysis. Brain sciences, 10(6), 386.
Source: https://doi.org/10.3390/brainsci10060386
O verview: This study examines the relationship between cholesterol and Alzheimer’s disease (AD). Researchers conducted a systematic search of meta-analyses and reviewed several databases, collecting 100 primary studies and five meta-analyses to analyze the connection between cholesterol and Alzheimer’s disease. They find that the literature compellingly demonstrates that low-density lipoprotein cholesterol (LDL-C) levels significantly influence the development of Alzheimer’s disease.
- The power of feedback revisited: A meta-analysis of educational feedback research (Wisniewski, Zierer & Hattie, 2020) – read here
- How Much Does Education Improve Intelligence? A Meta-Analysis (Ritchie & Tucker-Drob, 2018) – read here
- A meta-analysis of factors related to recycling (Geiger et al., 2019) – read here
- Stress management interventions for police officers and recruits (Patterson, Chung & Swan, 2014) – read here
Other Types of Reviews
- Scoping Review: This type of review is used to map the key concepts underpinning a research area and the main sources and types of evidence available. It can be undertaken as stand-alone projects in their own right, or as a precursor to a systematic review.
- Rapid Review: This type of review accelerates the systematic review process in order to produce information in a timely manner. This is achieved by simplifying or omitting stages of the systematic review process.
- Integrative Review: This review method is more inclusive than others, allowing for the simultaneous inclusion of experimental and non-experimental research. The goal is to more comprehensively understand a particular phenomenon.
- Critical Review: This is similar to a narrative review but requires a robust understanding of both the subject and the existing literature. In a critical review, the reviewer not only summarizes the existing literature, but also evaluates its strengths and weaknesses. This is common in the social sciences and humanities .
- State-of-the-Art Review: This considers the current level of advancement in a field or topic and makes recommendations for future research directions. This type of review is common in technological and scientific fields but can be applied to any discipline.
How to Write a Narrative Review (Tips for Undergrad Students)
Most undergraduate students conducting a capstone research project will be writing narrative reviews. Below is a five-step process for conducting a simple review of the literature for your project.
- Search for Relevant Literature: Use scholarly databases related to your field of study, provided by your university library, along with appropriate search terms to identify key scholarly articles that have been published on your topic.
- Evaluate and Select Sources: Filter the source list by selecting studies that are directly relevant and of sufficient quality, considering factors like credibility , objectivity, accuracy, and validity.
- Analyze and Synthesize: Review each source and summarize the main arguments in one paragraph (or more, for postgrad). Keep these summaries in a table.
- Identify Themes: With all studies summarized, group studies that share common themes, such as studies that have similar findings or methodologies.
- Write the Review: Write your review based upon the themes or subtopics you have identified. Give a thorough overview of each theme, integrating source data, and conclude with a summary of the current state of knowledge then suggestions for future research based upon your evaluation of what is lacking in the literature.
Literature reviews don’t have to be as scary as they seem. Yes, they are difficult and require a strong degree of comprehension of academic studies. But it can be feasibly done through following a structured approach to data collection and analysis. With my undergraduate research students (who tend to conduct small-scale qualitative studies ), I encourage them to conduct a narrative literature review whereby they can identify key themes in the literature. Within each theme, students can critique key studies and their strengths and limitations , in order to get a lay of the land and come to a point where they can identify ways to contribute new insights to the existing academic conversation on their topic.
Ankrah, S., & Omar, A. T. (2015). Universities–industry collaboration: A systematic review. Scandinavian Journal of Management, 31(3), 387-408.
Asghari, P., Rahmani, A. M., & Javadi, H. H. S. (2019). Internet of Things applications: A systematic review. Computer Networks , 148 , 241-261.
Dyrbye, L., & Shanafelt, T. (2016). A narrative review on burnout experienced by medical students and residents. Medical education , 50 (1), 132-149.
Geiger, J. L., Steg, L., Van Der Werff, E., & Ünal, A. B. (2019). A meta-analysis of factors related to recycling. Journal of environmental psychology , 64 , 78-97.
Martin, F., Sun, T., & Westine, C. D. (2020). A systematic review of research on online teaching and learning from 2009 to 2018. Computers & education , 159 , 104009.
Mavilidi, M. F., Ruiter, M., Schmidt, M., Okely, A. D., Loyens, S., Chandler, P., & Paas, F. (2018). A narrative review of school-based physical activity for enhancing cognition and learning: The importance of relevancy and integration. Frontiers in psychology , 2079.
Patterson, G. T., Chung, I. W., & Swan, P. W. (2014). Stress management interventions for police officers and recruits: A meta-analysis. Journal of experimental criminology , 10 , 487-513.
Reith, T. P. (2018). Burnout in United States healthcare professionals: a narrative review. Cureus , 10 (12).
Ritchie, S. J., & Tucker-Drob, E. M. (2018). How much does education improve intelligence? A meta-analysis. Psychological science , 29 (8), 1358-1369.
Roman, S., Sánchez-Siles, L. M., & Siegrist, M. (2017). The importance of food naturalness for consumers: Results of a systematic review. Trends in food science & technology , 67 , 44-57.
Sáiz-Vazquez, O., Puente-Martínez, A., Ubillos-Landa, S., Pacheco-Bonrostro, J., & Santabárbara, J. (2020). Cholesterol and Alzheimer’s disease risk: a meta-meta-analysis. Brain sciences, 10(6), 386.
Vermeir, P., Vandijck, D., Degroote, S., Peleman, R., Verhaeghe, R., Mortier, E., … & Vogelaers, D. (2015). Communication in healthcare: a narrative review of the literature and practical recommendations. International journal of clinical practice , 69 (11), 1257-1267.
Wisniewski, B., Zierer, K., & Hattie, J. (2020). The power of feedback revisited: A meta-analysis of educational feedback research. Frontiers in Psychology , 10 , 3087.
Yli-Huumo, J., Ko, D., Choi, S., Park, S., & Smolander, K. (2016). Where is current research on blockchain technology?—a systematic review. PloS one , 11 (10), e0163477.
Zestcott, C. A., Blair, I. V., & Stone, J. (2016). Examining the presence, consequences, and reduction of implicit bias in health care: a narrative review. Group Processes & Intergroup Relations , 19 (4), 528-542
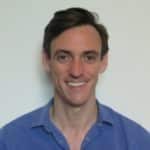
- Chris Drew (PhD) https://helpfulprofessor.com/author/chris-drew-phd-2/ 25 Classroom Wall Decoration Ideas
- Chris Drew (PhD) https://helpfulprofessor.com/author/chris-drew-phd-2/ 31 Cute & Cozy Play Corner Ideas
- Chris Drew (PhD) https://helpfulprofessor.com/author/chris-drew-phd-2/ 24 Steiner-Waldorf Classroom Design Ideas
- Chris Drew (PhD) https://helpfulprofessor.com/author/chris-drew-phd-2/ 25 Kindergarten Decoration Ideas
Leave a Comment Cancel Reply
Your email address will not be published. Required fields are marked *
- UWF Libraries
Literature Review: Conducting & Writing
- Sample Literature Reviews
- Steps for Conducting a Lit Review
- Finding "The Literature"
- Organizing/Writing
- APA Style This link opens in a new window
- Chicago: Notes Bibliography This link opens in a new window
- MLA Style This link opens in a new window
Sample Lit Reviews from Communication Arts
Have an exemplary literature review.
- Literature Review Sample 1
- Literature Review Sample 2
- Literature Review Sample 3
Have you written a stellar literature review you care to share for teaching purposes?
Are you an instructor who has received an exemplary literature review and have permission from the student to post?
Please contact Britt McGowan at [email protected] for inclusion in this guide. All disciplines welcome and encouraged.
- << Previous: MLA Style
- Next: Get Help! >>
- Last Updated: Mar 22, 2024 9:37 AM
- URL: https://libguides.uwf.edu/litreview

Home » Purdue University » What Makes A Good Literature Review?
What Makes A Good Literature Review?
Table of Contents
A good literature review shows signs of synthesis and understanding of the topic . There should be strong evidence of analytical thinking shown through the connections you make between the literature being reviewed.
What are the 5 characteristics of a good literature review?
Literature Review Format
- Provide an overview of the topic, theme, or issue.
- Identify your specific area of focus.
- Describe your methodology and rationale.
- Briefly discuss the overall trends in the published scholarship in this area.
- Establish your reason for writing the review.
What are the 5 C’s of writing literature review?
Is including the five C’s( Cite, Compare, Contrast, Critique and Connect ) really important in writing a literature review for your research project?
Why makes a good literature review?
A literature review establishes familiarity with and understanding of current research in a particular field before carrying out a new investigation . Conducting a literature review should enable you to find out what research has already been done and identify what is unknown within your topic.
What are the 4 main functions of literature review?
In relation to your own study, the literature review can help in four ways. It can: 1 bring clarity and focus to your research problem; 2 improve your research methodology; 3 broaden your knowledge base in your research area; and 4 contextualise your findings .
What are the 4 stages of literature review?
Literature search—finding materials relevant to the subject being explored. Data evaluation—determining which literature makes a significant contribution to the understanding of the topic. Analysis and interpretation—discussing the findings and conclusions of pertinent literature.
What are some of the most critical components of a good literature review?
The basic components of a literature review include:
- a description of the publication;
- a summary of the publication’s main points;
- a discussion of gaps in research;
- an evaluation of the publication’s contribution to the topic.
How do we write a literature review?
Write a Literature Review
- Narrow your topic and select papers accordingly.
- Search for literature.
- Read the selected articles thoroughly and evaluate them.
- Organize the selected papers by looking for patterns and by developing subtopics.
- Develop a thesis or purpose statement.
- Write the paper.
- Review your work.
How do you write a literature review example?
There are five key steps to writing a literature review:
- Search for relevant literature.
- Evaluate sources.
- Identify themes, debates and gaps.
- Outline the structure.
- Write your literature review.
What are the seven qualities of great literature?
The seven literary standards are: artistry, suggestiveness, intellectual value, spiritual value, permanence, universality and style . These are a set of characteristics to determine whether or not a work is literary. The criteria was developed by writer William J.
What makes good literature?
Great literature is based on ideas that are startling, unexpected, unusual, weighty. or new . Great literature makes us see or think things we never did before. The ideas underpinning the work challenge our accustomed categories and ways of thinking, putting minds on edge.
What are three six ways to write literature review?
6 Steps to Writing A Literature Review
- Synthesize.
What are the 6 steps in writing a literature review?
Organized around a proven six-step model and incorporating technology into all of the steps, the book provides examples, strategies, and exercises that take students step by step through the entire process: (1) Selecting a topic; (2) Searching the literature; (3) Developing arguments; (4) Surveying the literature; (5)
What makes a literature review fail?
There are several mistakes that may happen while writing an effective literature review includes no proper lines like dispute statement, absences of appropriate research, indicating the sources incorrectly, the poor formation of paper, plagiarism checking .
What are the 3 parts of literature review?
Just like most academic papers, literature reviews also must contain at least three basic elements: an introduction or background information section; the body of the review containing the discussion of sources; and, finally, a conclusion and/or recommendations section to end the paper .
What are the ten simple rules in writing literature?
Ten Simple Rules for Writing a Literature Review
- Rule 1: Define a Topic and Audience.
- Rule 2: Search and Re-search the Literature.
- Rule 3: Take Notes While Reading.
- Rule 4: Choose the Type of Review You Wish to Write.
- Rule 5: Keep the Review Focused, but Make It of Broad Interest.
- Rule 6: Be Critical and Consistent.
When starting your literature review what is the first step?
1. Define your topic . The first step is defining your task — choosing a topic and noting the questions you have about the topic. This will provide a focus that guides your strategy in step II and will provide potential words to use in searches in step III.
How many words should a literature review be?
In a PhD thesis, the literature review typically comprises one chapter (perhaps 8-10,000 words), for a Masters dissertation it may be around 2-3,000 words, and for an undergraduate dissertation it may be no more than 2,000 words.
How many sources should a literature review have?
The number of sources you will need will depend upon your assignment, professor, and level of study. In general, undergraduate students will usually be required to use somewhere between 5 and 20 sources; graduate students typically will need between 20 and 40 .
How many pages should literature review be?
The length of a literature review varies depending on its purpose and audience. In a thesis or dissertation, the review is usually a full chapter ( at least 20 pages), but for an assignment it may only be a few pages . There are several ways to organize and structure a literature review.
What are the qualities that make a piece of writing be considered literature?
Main Characteristics:
- usually fiction that displays a sense of reality.
- tension or conflict.
- artistic unity (a main idea is conveyed)
- figurative language (similes, metaphors, irony, symbolism, analogy)

By Travis Thornton
Travis Thornton is an education expert who has dedicated his life to helping students achieve their academic goals. He has worked as a teacher, tutor, and administrator in both public and private schools, and he currently serves as the dean of admissions at a prestigious university.
Travis believes that every student has the potential to succeed, and he tirelessly works to help them reach their full potential. He is a passionate advocate for education, and he believes that every student should have access to a quality education.
Travis is also a father of three young children, and he loves spending time with his family. He enjoys playing sports and watching movies together.
You might also like:
Can freshman have cars at purdue, what cities are near purdue university, is purdue close to notre dame.
OPINION article
Enhancing the quality and efficiency of regulatory science literature reviews through innovation and collaboration with library and information science experts.
- 1 Department of Population Health, New York University Grossman School of Medicine, New York, NY, United States
- 2 Health Sciences Library, New York University Grossman School of Medicine, New York, NY, United States
Introduction
The importance of literature reviews as part of regulatory science is widely understood. From large trials to follow-up case studies, capturing existing research is key to creating safe and effective regulatory policies. With a shift toward incorporating additional evidence-types—beyond randomized controlled trials (RCTs)—into regulatory decisions and the potential for literature reviews themselves to generate new data ( 1 – 7 ), the importance of high-quality literature reviews is increasing. However, rigorous and comprehensive literature reviews—that ensure important data are not missed ( 8 )—take substantial effort and can be difficult to do well. To encourage both comprehensiveness and efficiency in regulatory science literature reviews, additional focus is needed to integrate rigorous information science methodologies into regulatory science search strategies, as well as to develop innovative tools and strategies that can augment traditional search methods.
Meta-research (research on research) provides a way to examine the efficiency, quality, and potential bias in the overall research ecosystem ( 9 ). By applying a meta-research lens to literature reviews in regulatory science, we seek to elaborate on the challenges to performing high quality literature review data retrieval in regulatory science and identify potential strategies that may be explored to improve literature review quality and efficiency. Specifically, we hope to highlight the value of engaging library and information science expertise in the data retrieval process, including the strategic development of new controlled vocabulary and enhancement of conventional search techniques with novel digital technologies.
Challenges facing literature review in regulatory science
Due to the scope of potentially relevant materials, regulatory expectations, and an increasingly large body of research ( 10 ), regulatory science researchers face particular challenges to successfully identifying and retrieving the data they need. Identifying the potential obstacles to effective and high-quality literature review, both unique to regulatory research, as well as those experienced by other disciplines, is important for determining potential solutions to these barriers. The challenges identified fall into two main categories: diversity of potential data sources and breadth of potential search terms ( 11 ). These challenges are then overlain by the various stipulations put forth by regulatory bodies, such as post-marketing safety reports ( 12 ), thus, contributing to the importance of rapid turn around and the need for repeated searches ( 13 ).
Data relevant to a specific regulatory science research question may be found throughout a multitude of databases and repositories that house peer-reviewed research studies and/or gray literature ( 14 ). Some databases have a broad topical and geographic focus, whereas others may be more specialized or include language-specific resources. While there is often significant overlap among database contents, some materials will only be found in select databases; this necessitates a detailed knowledge of database characteristics to identify relevant search locations, as well as a search strategy that spans multiple databases ( 15 ).
Gray literature can require an additional level of awareness and expertise to identify, as these non-peer-reviewed data sources are often not indexed in the same manner as peer-reviewed literature. Conference proceedings, institutional reports, interim results, and other types of gray literature may be the first data sources to publish important adverse reaction outcomes and therefore may be necessary to identify. These materials, however, are found in a diverse set of platforms that are not always well-known or easily searchable ( 16 ). Similarly, relevant data may be found as secondary analyses within published clinical studies. Thus, additional expertise may be required to search beyond a title or abstract to identify potentially relevant literature. Furthermore, not all published materials are of equivalent quality, and in the age of paper mills, predatory journals ( 17 ), fraudulent citations ( 18 ), and paper retractions ( 19 ), having the expertise to discern which sources are reliable is needed to produce a high quality regulatory literature review ( 20 , 21 ).
In addition to the diverse locations in which it can be found, identifying relevant regulatory data can require an extensive list of search terms, as there can be inconsistency across geography, disciplines, and historically in how similar topics are described. To capture literature relevant for answering a research question, identifying an appropriate set of search terms is a critical step ( 22 , 23 ). Additionally, databases often employ a controlled vocabulary for indexing articles (such as PubMed's Medical Subject Headings, MeSH). A search strategy encompassing multiple databases will often require translating the search into the controlled vocabulary of each database ( 24 ). Defining search terms, can also pose a particular challenge depending on whether a topic has differing terminology in multiple disciplines, terminology that has changed over time, or a limited maturity of the research field leading to less well-characterized key terms ( 25 , 26 ).
Regulatory bodies often have requirements for the collection of data published in other countries, all drug or device formulations, and across the development spectrum. This can contribute to the need to identify appropriate vocabulary that accounts for foreign languages, spans all stages of development from pre-clinical to clinical studies, includes synonyms and older terms that have been replaced by more current terms, and captures all ways in which related products may be labeled. Furthermore, as the field of regulatory science has sought to include non-RCT evidence ( 1 – 7 ), additional vocabulary is needed to identify alternate study designs and data sources.
A role for library and information science experts
While in the age of rapid online literature searching the role of a librarian or other information science experts in literature reviews may feel unnecessary, the role of these experts has become even more vital to the production of high quality, thorough, and expedient regulatory science literature reviews. Finding all of the existing relevant research is a key component in performing effective regulatory research. However, with a multitude of databases and variations in search terms, understanding how and where to identify relevant data is not a simple task and requires strategy and knowledge of data systems. Therefore, studies that choose to include those with library and information science expertise on their teams are at a significant advantage for producing high quality literature reviews with less overall effort ( 27 – 29 ).
Indeed, a whole field of library and information science has evolved to develop methods for the management and discoverability of research data for research synthesis, whether in the published literature or in data repositories ( 30 ). Those skilled in information science can provide invaluable insights into the best approaches to identify potentially relevant literature ( 27 – 29 ). Information science experts can improve how controlled vocabulary can be used or improved for a specific research topic, as well as generate high quality search strategies.
Potential solutions to reduce the burden of information retrieval and maximize the quality of regulatory science literature reviews may be found in the expanded use of existing controlled vocabulary and strategic development of new controlled vocabulary. The use of controlled vocabulary, or indexing terms, can facilitate and improve the accuracy of literature searches being performed ( 31 , 32 ), and are a powerful tool that can overcome some of the limitations seen in a basic keyword search. Without the use of controlled vocabulary, search results would be limited to specific keywords or phrases used in the text, which could lead to irrelevant or incomplete results due to inconsistences in terminology used or alternate spellings. Thus, the standardization provided by controlled vocabulary helps ensure research is discoverable and accessible to a wider audience. Therefore, having knowledge of the controlled vocabulary for each database is a valuable skillset that can improve the comprehensiveness of a literature review. However, controlled vocabulary does have its limitations and not all important areas of research have an existing controlled vocabulary available ( 33 , 34 ). Furthermore, gray literature sources are typically not indexed with controlled vocabulary. Librarians or those with an information science expertise can assist in search strategies to overcome the shortcomings of controlled vocabulary, as well as help advance the strategic development of new controlled vocabulary specific to regulatory research ( 35 , 36 ).
A role for technological innovation
Even with a meticulously designed search strategy, the sheer amount of research findings in existence creates an important challenge to successfully and efficiently identifying all relevant research. Technological innovations, such as natural language processing (NLP) and generative artificial intelligence (GenAI), have the potential to provide alternative or supplementary approaches for identifying relevant research studies to address gaps and inefficiencies in information retrieval. Automation may help address the high volume of items in need of screening due to search term limitations by increasing search specificity and reducing the workload burden associated with manually screening all potentially appropriate items identified during literature search ( 37 ). However, there is significant work to be done before automation tools are viewed as successfully replacing traditional search methods ( 38 , 39 ).
During the literature search process, a balance must be struck between achieving the sensitivity necessary to identify all relevant documents while maintaining specificity to reduce the amount of irrelevant data collected. When using classic systematic search strategies aimed to maximize sensitivity, this can result in a large number of irrelevant documents that must be screened manually. Despite the importance of comprehensive information retrieval for high quality literature reviews, much of the effort placed into automating regulatory science data retrieval has been focused on enhancing the efficiency of article screening and data extraction ( 40 ), rather than improving the comprehensiveness and quality of data being collected in the literature search itself. Efforts for automating the review need to not just decrease workload but also improve the quality of the data being identified ( 41 ).
For topics and data sources that do not have an extensive controlled vocabulary, NLP tools can potentially assist researchers by providing an alternate method for identifying relevant literature. NLP combines computational linguistics, machine learning, and deep learning models to process human language to allow a computer program to understand human language as it is spoken and written, rather than relying on predefined vocabulary or the presence of specific phrases or terms within the text. This type of automation can equip a researcher to increase the specificity of their search strategy while maintaining sensitivity, as NLP can capture a broader range of vocabulary combinations, while providing phrase interpretation to eliminate data sources that use similar vocabulary, but in irrelevant contexts.
During the regulatory research literature review process, additional sources of information may be gleamed from within the reference lists of identified documents ( 42 ). But identifying and evaluating these citations can be time consuming and existing technology approaches have demonstrated suboptimal performance ( 38 , 43 , 44 ). GenAI tools can be developed to scan through research documents to identify and extract potentially relevant citations from a document's reference section for further review ( 43 ). Furthermore, the summarization capabilities of GenAI can reduce the burden of the screening process. Relevant data in a document may be contained within secondary analyses or sub-studies that are not reported in the document title or abstract, making the identification of these data sources time consuming, as they require a full-text review. GenAI tools can be developed to analyze the full text and provide a summary of the data to minimize the effort required to screen these sources. While some screening and summarization tools are available, many have not been validated for the literature review data extraction and screening process ( 39 , 45 – 49 ). Collaboration with an information science expert may improve the process of developing tools with improved validity.
To truly make these technologies work for regulatory science research, however, concerted investment is needed to develop and adapt these tools to meet the needs of the regulatory science researcher and meet systematic review standards ( 39 ). A collaboration between regulatory scientists and information science experts can serve to facilitate the development of effective and relevant review tools. To ensure reliability, GenAI and NLP approaches need to be validated on search use cases. However, the creation of datasets for training and validation can be time intensive and requires existing literature search expertise ( 50 ). In additional to collaborating on tool development, librarians and data science experts can be an important resource to evaluate the validity and utility of tools being developed within industry. Furthermore, attention must be given to the current limitations of using even “well-trained” GenAI. While adept at information generation and summarization, GenAI cannot discern the quality of the data sources it pulls from ( 51 ). Therefore, additional training will likely be necessary for a GenAI model to distinguish high- and low-quality data sources from an information science perspective (e.g., predatory journals) as well as discern what is a high-quality data source as per standards set forth by regulatory bodies.
Finding all existing relevant research is a key component to performing effective regulatory science research. However, regulatory science researchers face particular challenges to identifying and retrieving relevant data in an increasingly large body of research findings. With a high burden associated with sifting through irrelevant sources and a growing number of resources that may not be identified when using traditional or less sophisticated search strategies, performing high quality and efficient regulatory science reviews is a challenge. To address these barriers, further efforts are needed to increase the integration of rigorous information science expertise into regulatory science search strategies, as well as to develop innovative tools and strategies that can augment traditional search methods.
Author contributions
ES: Conceptualization, Methodology, Writing – original draft. GL: Conceptualization, Methodology, Writing – review & editing.
The author(s) declare financial support was received for the research, authorship, and/or publication of this article. This work was funded by NIA 1K01AG075169-01A1 (ES). The study sponsor was not involved in the conception or preparation of this manuscript.
Conflict of interest
The authors declare that the research was conducted in the absence of any commercial or financial relationships that could be construed as a potential conflict of interest.
Publisher's note
All claims expressed in this article are solely those of the authors and do not necessarily represent those of their affiliated organizations, or those of the publisher, the editors and the reviewers. Any product that may be evaluated in this article, or claim that may be made by its manufacturer, is not guaranteed or endorsed by the publisher.
1. Barbui C, Addis A, Amato L, Traversa G, Garattini S. Can systematic reviews contribute to regulatory decisions? Eur J Clin Pharmacol. (2017) 73:507–9. doi: 10.1007/s00228-016-2194-y
PubMed Abstract | Crossref Full Text | Google Scholar
2. U.S. Food and Drug Administration. 21st Century Cures Act . (2020). Available online at: https://www.fda.gov/regulatory-information/selected-amendments-fdc-act/21st-century-cures-act (accessed June 17, 2024).
Google Scholar
3. European Food Safety Authority EFS. Dealing With Evidence . (2024). Available online at: https://www.efsa.europa.eu/en/methodology/evidence (accessed June 17, 2024).
4. . Regulatory Science Research Needs 1.0 . (2022). Available online at: https://www.ema.europa.eu/en/documents/other/regulatory-science-research-needs_en.pdf (accessed June 17, 2024).
5. Burns L, Roux NL, Kalesnik-Orszulak R, Christian J, Hukkelhoven M, Rockhold F, et al. Real-world evidence for regulatory decision-making: guidance from around the world. Clin Ther. (2022) 44:420–37. doi: 10.1016/j.clinthera.2022.01.012
6. Beaulieu-Jones BK, Finlayson SG, Yuan W, Altman RB, Kohane IS, Prasad V, et al. Examining the use of real-world evidence in the regulatory process. Clin Pharmacol Ther. (2020) 107:843–52. doi: 10.1002/cpt.1658
7. Barbui C, Patten SB. Safety of psychotropic medicines: looking beyond randomised evidence. Epidemiol Psychiatr Sci. (2018) 27:529–30. doi: 10.1017/S2045796018000306
8. Ewald H, Klerings I, Wagner G, Heise TL, Stratil JM, Lhachimi SK, et al. Searching two or more databases decreased the risk of missing relevant studies: a metaresearch study. J Clin Epidemiol. (2022) 149:154–64. doi: 10.1016/j.jclinepi.2022.05.022
9. Harpe SE. Meta-research in pharmacy: time for a look in the mirror. Res Social Adm Pharm . (2021) 17:2028–35. doi: 10.1016/j.sapharm.2021.04.006
10. National Science Board NSF. Publications Output: U.S. Trends and International Comparisons . Science and Engineering Indicators (2024). NSB-2023-33; Alexandria, VA. Available online at: https://ncses.nsf.gov/pubs/nsb202333/ (accessed June 17, 2024).
11. MacFarlane A, Russell-Rose T, Shokraneh F. Search strategy formulation for systematic reviews: Issues, challenges and opportunities. Intell Syst Appl. (2022) 15:200091. doi: 10.1016/j.iswa.2022.200091
12. U.S. Food and Drug Administration. Postmarketing Safety Reporting for Human Drug and Biological Products Including Vaccines . (2020). Available online at: https://www.fda.gov/regulatory-information/search-fda-guidance-documents/postmarketing-safety-reporting-human-drug-and-biological-products-including-vaccines (accessed June 17, 2024).
13. Honig P, Zhang L. Regulation and innovation: role of regulatory science in facilitating pharmaceutical innovation. Clin Pharmacol Ther. (2019) 105:778–81. doi: 10.1002/cpt.1367
14. Gusenbauer M. The age of abundant scholarly information and its synthesis– A time when ‘just google it' is no longer enough. Res Synth Methods. (2021) 12:684–91. doi: 10.1002/jrsm.1520
15. Wanyama SB, McQuaid RW, Kittler M. Where you search determines what you find: the effects of bibliographic databases on systematic reviews. Int J Soc Res Methodol. (2022) 25:409–22. doi: 10.1080/13645579.2021.1892378
Crossref Full Text | Google Scholar
16. Saleh AA, Ratajeski MA, Bertolet M. Grey literature searching for health sciences systematic reviews: a prospective study of time spent and resources utilized. Evid Based Libr Inf Pract. (2014) 9:28–50. doi: 10.18438/B8DW3K
17. Richtig G, Berger M, Lange-Asschenfeldt B, Aberer W, Richtig E. Problems and challenges of predatory journals. J Eur Acad Dermatol Venereol. (2018) 32:1441–9. doi: 10.1111/jdv.15039
18. Májovský M, Cerný M, Kasal M, Komarc M, Netuka D. Artificial intelligence can generate fraudulent but authentic-looking scientific medical articles: pandora's box has been opened. J Med Int Res. (2023) 25:e46924. doi: 10.2196/46924
19. Van Noorden R. More than 10,000 research papers were retracted in 2023—a new record. Nature. (2023) 624:479–81. doi: 10.1038/d41586-023-03974-8
20. Gerberi D, Taylor JM, Beeler CJ. Educating authors and users of the literature to increase vigilance of predatory publishing. J Hosp Librariansh. (2021) 21:207–16. doi: 10.1080/15323269.2021.1942691
21. Ross-White A, Godfrey CM, Sears KA, Wilson R. Predatory publications in evidence syntheses. J Med Libr Assoc. (2019) 107:57–61. doi: 10.5195/jmla.2019.491
22. Bramer WM, de Jonge GB, Rethlefsen ML, Mast F, Kleijnen J. A systematic approach to searching: an efficient and complete method to develop literature searches. J Med Libr Assoc. (2018) 106:531–41. doi: 10.5195/jmla.2018.283
23. Higgins JP, Thomas J, Chandler J, Cumpston M, Li T, Page MJ, et al. Cochrane Handbook for Systematic Reviews of Interventions . Chichester: John Wiley & Sons (2019).
24. Parker RMN, Neilson MJ. Lost in Translation: supporting learners to search comprehensively across databases. J Can Health Librar. (2015) 36:54–8. doi: 10.5596/c15-014
25. Paul J, Barari M. Meta-analysis and traditional systematic literature reviews—What, why, when, where, and how? Psychol Market. (2022) 39:1099–115. doi: 10.1002/mar.21657
26. Card NA. Applied Meta-Analysis for Social Science Research . New York, NY: Guilford Publications (2015).
27. Rethlefsen ML, Farrell AM, Osterhaus Trzasko LC, Brigham TJ. Librarian co-authors correlated with higher quality reported search strategies in general internal medicine systematic reviews. J Clin Epidemiol. (2015) 68:617–26. doi: 10.1016/j.jclinepi.2014.11.025
28. Aamodt M, Huurdeman HC, Strømme H. Librarian co-authored systematic reviews are associated with lower risk of bias compared to systematic reviews with acknowledgement of librarians or no participation by librarians. Evid Based Libr Inf Pract . (2019) 14, 103–127. doi: 10.18438/eblip29601
29. Ibragimova I, Fulbright H. Librarians and information specialists as methodological peer-reviewers: a case-study of the International Journal of Health Governance. Res Integr Peer Rev. (2024) 9:1. doi: 10.1186/s41073-023-00142-4
30. Smith LC. Reviews and reviewing: approaches to research synthesis. An Annual Review of Information Science and Technology (ARIST) paper. J Assoc Inf Sci Technol. (2024) 75:245–67. doi: 10.1002/asi.24851
31. Lindberg DA, Humphreys BL, McCray AT. The unified medical language system. Methods Inf Med. (1993) 32:281–91. doi: 10.1055/s-0038-1634945
32. Doig GS, Simpson F. Efficient literature searching: a core skill for the practice of evidence-based medicine. Intens Care Med. (2003) 29:2119–27. doi: 10.1007/s00134-003-1942-5
33. Bass MB, Molina RL, Reardon EE, Porcello L, Goldberg J, Nickum A, et al. Trends in…controlled vocabulary and health equity. Med Ref Serv Q. (2022) 41:185–201. doi: 10.1080/02763869.2022.2060638
34. McTavish JR, Rasmussen Neal D, Wathen CN. Is what you see what you get? Medical subject headings and their organizing work in the violence against women research literature article. Knowl Org. (2011) 38:381–97. doi: 10.5771/0943-7444-2011-5-381
35. Stevens EL. Recognizing the value of meta-research and making it easier to find. J Med Libr Assoc . (2023) 111:1758. doi: 10.5195/jmla.2023.1758
36. Logan J. Why do researchers co-author evidence syntheses with librarians? A mixed-methods study. Res Synth Methods. (2023) 14:489–503. doi: 10.1002/jrsm.1629
37. Tang F-SK-B, Bukowski M, Schmitz-Rode T, Farkas R. Guidance for Clinical evaluation under the medical device regulation through automated scoping searches. Appl Sci. (2023) 13:7639. doi: 10.3390/app13137639
38. O'Connor AM, Tsafnat G, Thomas J, Glasziou P, Gilbert SB, Hutton B, et al. Question of trust: can we build an evidence base to gain trust in systematic review automation technologies? Syst Rev. (2019) 8:143. doi: 10.1186/s13643-019-1062-0
39. O'Connor AM, Glasziou P, Taylor M, Thomas J, Spijker R, Wolfe MS, et al. Focus on cross-purpose tools, automated recognition of study design in multiple disciplines, and evaluation of automation tools: a summary of significant discussions at the fourth meeting of the International Collaboration for Automation of Systematic Reviews (ICASR). Syst Rev. (2020) 9:100. doi: 10.1186/s13643-020-01351-4
40. Halamoda-Kenzaoui B, Rolland E, Piovesan J, Puertas Gallardo A, Bremer-Hoffmann S. Toxic effects of nanomaterials for health applications: how automation can support a systematic review of the literature? J Appl Toxicol. (2022) 42:41–51. doi: 10.1002/jat.4204
41. Laynor G. Can systematic reviews be automated? J Electron Resour Med Libr. (2022) 19:101–6. doi: 10.1080/15424065.2022.2113350
42. Wohlin C, Kalinowski M, Romero Felizardo K, Mendes E. Successful combination of database search and snowballing for identification of primary studies in systematic literature studies. Inf Softw Technol. (2022) 147:106908. doi: 10.1016/j.infsof.2022.106908
43. Bakker C, Theis-Mahon N, Brown SJ. Evaluating the accuracy of scite, a smart citation index. Hypothesis . (2023) 35:26528. doi: 10.18060/26528
44. Allot A, Lee K, Chen Q, Luo L, Lu Z. LitSuggest: a web-based system for literature recommendation and curation using machine learning. Nucleic Acids Res. (2021) 49:W352–8. doi: 10.1093/nar/gkab326
45. Goodwin Burri K. Intelligent use of artificial intelligence for systematic reviews of medical devices. Article Med Writing. (2019) 28:22–6.
46. Gramoun A. Digital tools for the clinical evaluation of medical devices: a guide to empower regulatory writers. Med Writing. (2023) 32:16–21. doi: 10.56012/hmts9332
47. Perlman-Arrow S, Loo N, Bobrovitz N, Yan T, Arora RK. A real-world evaluation of the implementation of NLP technology in abstract screening of a systematic review. Res Synth Methods. (2023) 14:608–21. doi: 10.1002/jrsm.1636
48. Yao X, Kumar MV, Su E, Flores Miranda A, Saha A, Sussman J. Evaluating the efficacy of artificial intelligence tools for the automation of systematic reviews in cancer research: a systematic review. Cancer Epidemiol. (2024) 88:102511. doi: 10.1016/j.canep.2023.102511
49. Marshall IJ, Trikalinos TA, Soboczenski F, Yun HS, Kell G, Marshall R, et al. In a pilot study, automated real-time systematic review updates were feasible, accurate, and work-saving. J Clin Epidemiol. (2023) 153:26–33. doi: 10.1016/j.jclinepi.2022.08.013
50. Wu L, Chen S, Guo L, Shpyleva S, Harris K, Fahmi T, et al. Development of benchmark datasets for text mining and sentiment analysis to accelerate regulatory literature review. Regul Toxicol Pharmacol. (2023) 137:105287. doi: 10.1016/j.yrtph.2022.105287
51. Hersh W. Search still matters: information retrieval in the era of generative AI. J Am Med Inform Assoc. (2024) ocae014. doi: 10.1093/jamia/ocae014
Keywords: meta-research, literature review methods, information retrieval, regulatory science, collaboration
Citation: Stevens ER and Laynor G (2024) Enhancing the quality and efficiency of regulatory science literature reviews through innovation and collaboration with library and information science experts. Front. Med. 11:1434427. doi: 10.3389/fmed.2024.1434427
Received: 17 May 2024; Accepted: 24 June 2024; Published: 03 July 2024.
Reviewed by:
Copyright © 2024 Stevens and Laynor. This is an open-access article distributed under the terms of the Creative Commons Attribution License (CC BY) . The use, distribution or reproduction in other forums is permitted, provided the original author(s) and the copyright owner(s) are credited and that the original publication in this journal is cited, in accordance with accepted academic practice. No use, distribution or reproduction is permitted which does not comply with these terms.
*Correspondence: Elizabeth R. Stevens, elizabeth.stevens@nyulangone.org
Disclaimer: All claims expressed in this article are solely those of the authors and do not necessarily represent those of their affiliated organizations, or those of the publisher, the editors and the reviewers. Any product that may be evaluated in this article or claim that may be made by its manufacturer is not guaranteed or endorsed by the publisher.
- Open access
- Published: 09 July 2024
The incidence of fractures in children under two years of age: a systematic review
- Karen Rosendahl 1 , 2 ,
- Laura Tanturri de Horatio 1 , 2 , 3 ,
- Celine Habre 4 ,
- Susan C. Shelmerdine 5 , 6 , 7 , 8 ,
- Janina Patsch 9 ,
- Ola Kvist 10 , 11 ,
- Regina K. Lein 12 ,
- Domen Plut 13 , 14 ,
- Edvard J. Enoksen 1 ,
- Rien Avenarius 1 ,
- Lene B. Laborie 15 , 16 ,
- Thomas A. Augdal 1 , 2 ,
- Paolo Simoni 17 ,
- Rick R. van Rijn 18 &
- Amaka C. Offiah 19
on behalf of the European Society of Paediatric Radiology (ESPR) Musculoskeletal and Child Abuse Task Forces
BMC Musculoskeletal Disorders volume 25 , Article number: 528 ( 2024 ) Cite this article
Metrics details
Epidemiological research on fractures in children under the age of two is of great importance to help understand differences between accidental and abusive trauma.
This systematic review aimed to evaluate studies reporting on the incidence of fractures in children under two years of age, excluding birth injuries. Secondary outcome measures included fracture location, mechanisms of injury and fracture characteristics.
A systematic literature review (1946 to February 7th 2024), including prospective and retrospective cohort studies and cross-sectional cohort studies, was performed. Studies including children from other age groups were included if the actual measures for those aged 0–2 years could be extracted. We also included studies restricted to infants. Annual incidence rates of fractures were extracted and reported as the main result. Critical appraisal of was performed using the Appraisal tool for Cross-Sectional Studies.
Twelve moderate to good quality studies met eligibility criteria, of which seven were based on data from medical records and five were registry studies. Studies investigated different aspects of fractures, making comprehensive synthesis challenging. There was an overall annual fracture incidence rate of 5.3 to 9.5 per 1,000 children from 0–2 years of age; with commonest sites being the radius/ulna (25.2–40.0%), followed by tibia/fibula (17.3–27.6%) and the clavicle (14.6–14.8%) (location based on 3 studies with a total of 407 patients). In infants, the reported incidence ranged between 0.7 to 4.6 per 1,000 (based on 3 studies), with involvement of the clavicle in 22.2% and the distal humerus in 22.2% of cases (based on 1 study). Only a single metaphyseal lesion was reported (proximal humerus of an 11-month-old infant). Fracture mechanisms were detailed in four studies, with fall from chair, bed, table, own height or fall following indoor activities causing 50–60% of fractures.
Conclusions
There is a paucity of good quality data on fracture incidence in children under the age of two. Larger, prospective and unbiased studies would be helpful in determining normal pattern of injuries, so that differences from abusive trauma may be better understood.
Peer Review reports
Introduction
In children under the age of two, fractures are rare, particularly in non-ambulatory infants, with a predilection for the clavicle and skull in those under 8 months of age [ 1 ]. In toddlers between 9 and 24 months of age, forearm and lower leg fractures predominate [ 1 , 2 ]. The incidence and pattern of fractures in children under the age of two is, however, poorly described in the literature. This age group is particularly vulnerable to inflicted injury, which may be difficult to detect. Both under- and overdiagnosis occur, in part due to limited knowledge of variations in normal growth that may mimic pathology [ 3 , 4 , 5 ], limited experience, and subtlety of fractures of immature bone. Although the Royal College of Paediatrics and Child Health website provides important knowledge for those dealing with potential abusive trauma ( https://childprotection.rcpch.ac.uk/child-protection-evidence/fractures-systematic-review/ ), it mainly focuses on fractures indicative of abuse, fracture dating, and rib fractures secondary to cardiopulmonary resuscitation.
In this novel systematic review, we aim to identify and summarise all epidemiological studies which have reported on the incidence of fractures in children under the age of two. Secondary outcome measures include fracture location, mechanisms of injury and fracture characteristics.
A systematic literature review was performed in accordance with the Preferred Reporting Items for Systematic Reviews and Meta-Analyses guidelines [ 6 ]. The protocol was registered in the International prospective register of systematic reviews (PROSPERO reg. number CRD42022355938). Ethical approval was not required for this review of publicly available data.
Eligibility criteria
The review includes all studies which have attempted to quantitatively assess the incidence of fractures in children under two years of age; thus, the outcome of interest was the annual incidence rates, with secondary measures being localization, fracture characteristics and mechanisms.
Inclusion criteria applied to identified studies were epidemiological studies, written in English, which attempt to quantitatively assess the incidence of fractures in children under two years of age, including prospective and retrospective cohort studies and cross-sectional cohort studies. Studies including children from other age groups were included if the actual measures for those aged 0–2 years could be extracted. We also included studies restricted to infants (0–1-year-olds).
Excluded were non-primary research, systematic literature reviews, animal studies, in-vitro studies, interventional studies, single case reports, editorials/comments, and clinical guidelines; studies lacking full text or relevant data on outcomes, studies addressing birth injuries alone, studies addressing child abuse alone, studies of children with underlying disease (e.g., osteogenesis imperfecta, leukaemia, metabolic bone disease etc.) and studies restricted to sites other than the limbs or ribs. When studies reported findings from the same population, we selected only the most relevant study based on date, sample size, and reported analysis of data.
Information sources and search strategy
We comprehensively searched Medline (Ovid), Embase (Ovid), the Cochrane Library, Cinahl (Ebco) and Web-of-Science (Clarivate) for full text articles published in English between 1946 and 7th of February 2024 (RKL/KR, the latter with 35 years of experience in paediatric radiology). Both subject headings and free text words were used for the following concepts: bone fracture, incidence, and children under 2 years of age (detailed search strategies are listed in Additional file 1). We also searched the reference lists of the included articles.
Screening, study selection and data extraction
Search results were exported through EndNote, version 20 (Clarivate, Philadelphia, US) duplicates were removed, and all eligible studies were imported to Rayyan [ 7 ]. Titles and abstracts were screened by one investigator (KR) for possible inclusion according to the pre-specified eligibility criteria [ 8 , 9 ]. A random sample of 35% of titles and abstracts were double screened by one of two investigators (SCS/LTdH) to ensure high levels of agreement. Any article which the investigator was unsure about was included in the list of full text articles to be reviewed in a second stage. Full text articles were retrieved and assessed for final eligibility by one investigator (KR), and if doubt, in consensus with a second reviewer (RRvR). From the included studies, two reviewers (KR/RRvR) independently extracted relevant data and populated a project-specific Microsoft Excel spreadsheet. Discrepancies between values were discussed and resolved between the reviewers and/or by involving a third reviewer (TAA). The following data were collected: study details (first author, publication year and country), recruitment setting (sample description/hospital/year), study design, sample size (number of children under two years of age/number of fractures), sex and outcome measures (annual incidence rates (per 1,000), location (five most common fracture sites as reported in each paper), mechanism, fracture type (transverse, spiral etc.) and whether the fracture was acute or healing.
Strategy for data synthesis
The data synthesis was through a narrative analysis method of incidence. Annual incidence rates of fractures (per 1,000) were extracted, and reported as the main result (in total, and by sex / location / mechanism).
Assessment of methodological quality
Critical appraisal was performed independently by three reviewers (OK, CH, JP, with 7, 5 and 10 years of experience in paediatric radiology, respectively) to assess the quality of included studies and provide context for the interpretation of the findings. Each of the selected studies was evaluated with the Appraisal tool for Cross-Sectional Studies (AXIS) (Additional file 2), focusing on the presented aims, methods and analysis of what is reported [ 10 ]. As the tool does not provide a numerical scale for assessing the quality of a study, a degree of subjectivity was used to classify the studies into poor, fair, moderate or good quality [ 10 ]. When studies included multiple analyses aimed at answering several research questions within the same study, quality assessments were only applied to the analyses relevant to this systematic review.
A total of 10,341 references were found following the literature search (Fig. 1 ). After removal of duplicates, 6,644 titles/abstracts were screened for relevance, of which 6,507 were excluded. After a full-text review of the remaining 136 studies, 12 were eventually included (Fig. 1 ).
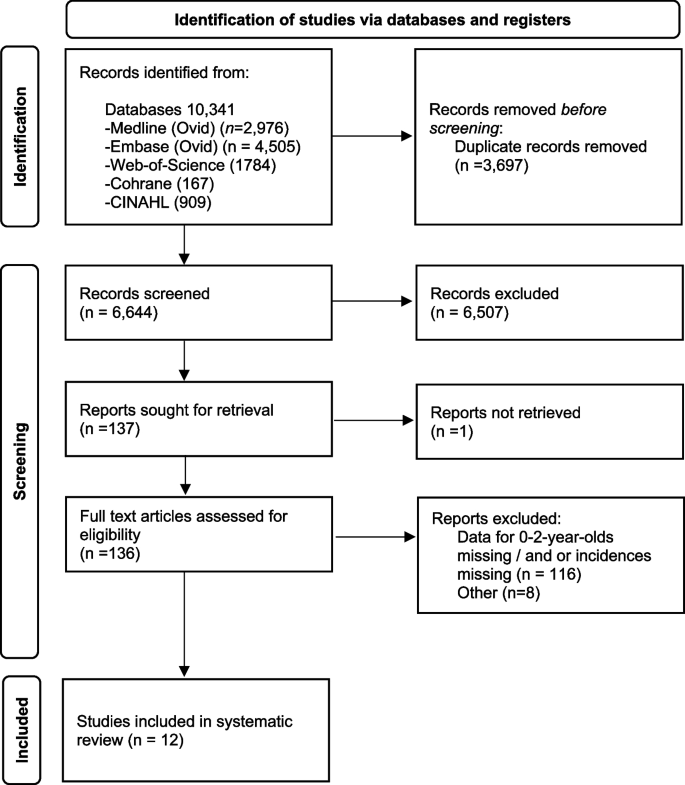
PRISMA flow diagram outlining the process by which articles were screened
Characteristics of studies
Of the 12 included studies, 7 were based on review of medical records; of which 5 were single hospital studies [ 1 , 2 , 11 , 12 , 13 ], 1 was based on medical records from two paediatric trauma units [ 12 ] and 1 on data from 27 hospitals and 126 clinics [ 14 ]. Five were registry studies [ 15 , 16 , 17 , 18 , 19 ].
Two were prospective [ 12 , 13 ], 5 were retrospective cohort studies [ 1 , 2 , 11 , 14 , 20 ], and 5 were retrospective registry studies. In 3 of the studies, all radiographs were re-assessed by a radiologist or by an orthopaedic surgeon to minimize misdiagnosis [ 2 , 13 , 20 ] (Table 1 ).
All 12 studies were performed in, or using data from cities and/or rural areas; 4 studies in the UK [ 1 , 13 , 15 , 17 ], 2 in the US [ 16 , 19 ], 2 in Scotland [ 12 , 20 ], 2 in Sweden [ 11 , 18 ], 1 in Norway [ 2 ], and 1 in Japan [ 14 ]. Sample size was given for 5 out of 8 studies on children < 2 years of age (mean 178 fractures, range 122–245) and for 1 of 4 studies including infants (Table 1 ).
Four studies included all relevant fracture locations [ 1 , 2 , 11 , 20 ], while the remainder reported on the incidence of fractures to the appendicular skeleton [ 12 , 19 ], to the femur [ 15 , 16 , 17 , 18 ] or to the distal radius [ 13 , 14 ] (Table 1 ).
All studies were considered of moderate to good quality based on the AXIS system, although several were lacking population denominator and census-based demographic data necessary to generate true incidence rates (Table 1 ). Study design limitations were mainly due to potential selection bias or unadjusted confounders. Important potential confounders, such as socioeconomic status or additional comorbidities were not accounted for in any of the analyses.
Incidence estimates
Study results are summarized in Tables 1 and 2 . The overall annual fracture incidence rates for children under two years of age was reported at 5.3 to 9.5 per 1,000 [ 1 , 2 , 11 ], while the incidence for children under the age of one ranged from 0.7 to 4.6 per 1,000 [ 2 , 12 , 20 ]. The incidence of limb fractures was reported at 4.6 per 1,000 amongst infants, rising to 7.3 per 1,000 for those between one and two years of age [ 12 ].
Femur fractures had an incidence rate range of 0.07–0.2 per 1,000 for infants [ 15 , 18 ], increasing to 0.3–0.5 per 1,000 for 0–2-year-olds [ 16 , 17 ]. For 1–2-year-olds, the corresponding figure was 12.1 per 1,000 [ 15 ].
Three studies reported on sex distribution, of which two found fractures to be equally distributed between sexes; one addressing all except high energy traumas fractures in children 0–2 years of age [ 2 ] and the other addressing fractures to the distal radius in infants [ 14 ]. The third study reported on more fractures in girls than in boys; 62.6% vs 37.4% [ 1 ].
Most common fracture locations
Three papers reported on the most common fracture sites; in 0–2-year-olds the radius/ulna (25.2–40% of all fractures), followed by the tibia/fibula (17.3–27.6%), and the clavicle (14.6–14.8%) [ 1 , 2 , 12 ] (Table 2 ). In infants, the most common fracture sites were the clavicle and distal humerus (22.2% each of all fractures) [ 20 ].
Fracture mechanisms
Fracture mechanisms were reported in 7 studies, of which 2 of the 3 studies including all locations in 0–2-year-olds, described fall from low height (chair, bed, table, own height) to cause 50–70% of fractures [ 1 , 2 ], while a third study described fall, without specifying height, as the cause in 52% [ 12 ] (Table 2 ). Five studies reported on abuse as a potential mechanism in 4.1%—12.2% of the cases [ 1 , 2 , 12 , 16 , 17 ]. As for fractures to the femur, falls were the reported mechanism in 24–77% of the cases [ 15 , 16 , 17 , 18 ], of which two studies specified the height [ 15 , 18 ]. In the Swedish registry study from 2011 including 313 infants with femur fractures, birth injuries excluded, the authors found that 70 (22.4%) out of 313 fractures were caused by a fall, of which 31 from a height < 1 m, 19 from a height > 1 m, whilst the remainder 20 were unspecified [ 18 ]. In the study from Talbot et al., the most common mechanism was fall of less than two meters [ 15 ].
Type of fractures
Two studies reported on fracture type [ 2 , 12 ], 31–32% being of the buckle/greenstick type (Table 2 ). Only a single classical metaphyseal lesion (CML) (in a proximal humerus of an 11-month-old infant) was reported [ 2 ]. The fracture was initially missed, but diagnosed during the retrospective review of the radiographs. The child refused to use the arm, however, there was no mention of trauma in the medical notes.
Acute/healing fracture
The incidence of healing fractures was reported at 0.3 per 1,000 in children under two years of age [ 2 ]. This information could not be extracted for those under 1 year of age.
The purpose of this review was to systematically investigate the existing literature to determine the population-based fracture incidence in children under the age of two years. Although there was a vast body of literature reporting fractures in children, most papers did not report figures for 0–2-year-olds specifically. Moreover, studies were lacking the appropriate population denominator and census-based demographic data necessary to generate true incidence rates rather than frequencies or proportions. Studies differed in design; methods to secure a population-based cohort; type of health service where the study was undertaken; and clinical setting. The degree of variation across the studies, combined with our quality findings that most studies were at risk of bias, meant that it was not appropriate to pool the results in a meta-analysis.
Most included studies were based on researcher-collected data from medical records, while five were registry based. Despite the increasing use, no developed methodological literature on use and evaluation of population based registers is available [ 21 ]. Although complete study populations minimize selection bias, registry studies are limited by missing data, lack of data quality, confounder information, and the risk of data dredging. On the other hand, data retrospectively collected from medical records suffer similar limitations, underscoring the need for prospective studies and validated research databases.
Knowledge of fractures in children has typically come from Northern European population studies reported in the late 1970s through the 80 s and 90 s [ 22 , 23 , 24 , 25 ], however, most of these have provided pooled data from birth until school-age or until skeletal maturity without focusing on the youngest age group. Despite performing an extensive literature search, we identified only three studies reporting true, population-based incidences in 0–2-year-olds [ 1 , 2 , 11 ]. The reported incidences were relatively similar, ranging from 5.3 to 9.5 per 1,000, of which the latter comes with a caveat being deduced from a figure in the original paper.
Three studies addressed infants, with reported fracture incidences ranging from 0.7 per 1,000 in a Norwegian study [ 2 ] to 3.6 and 4.6 per 1,000 in two studies from Scotland [ 12 , 20 ]. The differences may in part be due to selection bias, as one study excluded high energy trauma [ 2 ], another excluded skull and rib fractures [ 20 ] and a third excluded the axial skeleton, pelvis and chest, but included the clavicles [ 12 ]. The many different methodological and reporting approaches highlights the challenges of synthesising results. Although there was high heterogeneity of the studies included, two studies concurred and showed significantly higher fracture rates in 1–2-year-olds compared to infants [ 2 , 12 ]. This finding seems reasonable, as fractures are less likely to occur in non-ambulatory infants.
Our review found that fractures to the forearm constituted up to 50% of all fractures in children aged 0–2 years, as compared to around 20% in infants. However, the number of studies is low, reducing confidence in this finding. Interestingly, only a single CML (of the proximal humerus of an 11-month-old infant) was reported despite the thousands reviewed. The child was brought to the emergency out-patient clinic because he refused to use his left arm, with no history of trauma. Unfortunately, the fracture was missed during the initial visit, thus the finding did not trigger a more extensive work-up. In retrospect, the authors speculate that the fracture might have represented a missed, inflicted injury [ 2 , 3 ].
In terms of fracture mechanisms, insignificant injury or fall from low height such as chair, bed, table or own height, was the reported mechanism in 50–60% of all fractures amongst 0–2-year-olds, while this was the case for one tenth of femur fractures in infants. However, these results must be interpreted with care, as none of the studies registered fracture mechanisms in a detailed, prospective manner. Moreover, a significant proportion of the injuries were not observed by the caretakers or by other adults, thus, the figures given include potentially abusive fractures. However, it was not the purpose of this review to examine the incidence of inflicted injury.
The strengths of this systematic review include the rigorous methodological approach employed using an established methodological framework. A comprehensive search strategy was used, with broad inclusion criteria. Three independent reviewers were involved in the screening process to identify papers for full-text reading, and a fourth reviewer was included in data extraction. Moreover, the search was repeated at the time of manuscript preparation to capture recent and relevant studies.
There are some limitations to the present study. First, the number of studies was low with varying quality, and many did not report essential data, such as incidences by sex. Second, given the limitations of the reported data, the risk of bias among the included studies and the wide heterogeneity between them, we were unable to combine data in a meta-analysis, and instead results were reported as a narrative summary. Thirdly, we included articles written in English only. We also planned to assess publication bias but were unable to do so owing to the wide heterogeneity between the included studies. The generalisability of these findings may be uncertain.
There is a paucity of good quality data on fracture incidence in children under the age of two. This systematic review of the literature found only 12 studies over the last 78 years that met the eligibility criteria, however, due to data inhomogeneity a meta-analysis could not be calculated. From the limited, potentially biased data available, we calculated the following: an overall incidence of fractures of around 1% in children under 2-year-olds, most of which were lower leg or forearm fractures, and a lower incidence in infants (under 1-year-olds) being a maximum of 0.5%, most of which were clavicle and humeral fractures. The low frequency of CMLs and absence of rib fractures may be differentiating features from inflicted injury.
Availability of data and materials
The datasets used and/or analysed during the current study are available from the corresponding author on reasonable request.
Clarke NM, Shelton FR, Taylor CC, Khan T, Needhirajan S. The incidence of fractures in children under the age of 24 months–in relation to non-accidental injury. Injury. 2012;43(6):762–5.
Article PubMed Google Scholar
Rosendahl K, Myklebust R, Ulriksen KF, Nottveit A, Eide P, Djuve A, Brudvik C. Incidence, pattern and mechanisms of injuries and fractures in children under two years of age. BMC Musculoskelet Disord. 2021;22(1):555.
Article PubMed PubMed Central Google Scholar
Eide P, Djuve A, Myklebust R, Forseth KF, Nottveit A, Brudvik C, Rosendahl K. Prevalence of metaphyseal injury and its mimickers in otherwise healthy children under two years of age. Pediatr Radiol. 2019;49(8):1051–5.
Keats TE. Some new normal roentgen variants that may simulate disease. Curr Probl Diagn Radiol. 1981;10(3):1–52.
CAS PubMed Google Scholar
Keats TE. Atlas of normal roentgen variants that may simulate disease. 9th ed. Philadelphia: Elsevier; 2013.
Google Scholar
Moher D, Shamseer L, Clarke M, Ghersi D, Liberati A, Petticrew M, Shekelle P, Stewart LA, Group PP. Preferred reporting items for systematic review and meta-analysis protocols (PRISMA-P) 2015 statement. Syst Rev. 2015;4:1.
Article Google Scholar
Ouzzani M, Hammady H, Fedorowicz Z, Elmagarmid A. Rayyan-a web and mobile app for systematic reviews. Syst Rev. 2016;5(1):210.
Shemilt I, Khan N, Park S, Thomas J. Use of cost-effectiveness analysis to compare the efficiency of study identification methods in systematic reviews. Syst Rev. 2016;5(1):140.
Mathieu E, Noguchi N, Li T, Barratt AL, Hersch JK, De Bock GH, Wylie EJ, Houssami N. Health benefits and harms of mammography screening in older women (75+ years)-a systematic review. Br J Cancer. 2024;130(2):275–96.
Downes MJ, Brennan ML, Williams HC, Dean RS. Development of a critical appraisal tool to assess the quality of cross-sectional studies (AXIS). BMJ Open. 2016;6(12):e011458.
Tiderius CJ, Landin L, Duppe H. Decreasing incidence of fractures in children: an epidemiological analysis of 1,673 fractures in Malmo, Sweden, 1993–1994. Acta Orthop Scand. 1999;70(6):622–6.
Article CAS PubMed Google Scholar
Hansoti B, Beattie TF. Limb fractures and nonaccidental injury in children less than 24 months of age. Eur J Emerg Med. 2008;15(2):63–6.
Mamoowala N, Johnson NA, Dias JJ. Trends in paediatric distal radius fractures: an eight-year review from a large UK trauma unit. Ann R Coll Surg Engl. 2019;101(4):297–303.
Article CAS PubMed PubMed Central Google Scholar
Hagino H, Yamamoto K, Ohshiro H, Nose T. Increasing incidence of distal radius fractures in Japanese children and adolescents. J Orthop Sci. 2000;5(4):356–60.
Talbot C, Davis N, Majid I, Young M, Bouamra O, Lecky FE, Jones S. Fractures of the femoral shaft in children: national epidemiology and treatment trends in England following activation of major trauma networks. Bone Joint J. 2018;100-B(1):109–18.
Hinton RY, Lincoln A, Crockett MM, Sponseller P, Smith G. Fractures of the femoral shaft in children. Incidence, mechanisms, and sociodemographic risk factors. J Bone Joint Surg Am. 1999;81(4):500–9.
Bridgman S, Wilson R. Epidemiology of femoral fractures in children in the West Midlands region of England 1991 to 2001. J Bone Joint Surg Br. 2004;86(8):1152–7.
Heideken J, Svensson T, Blomqvist P, Haglund-Akerlind Y, Janarv PM. Incidence and trends in femur shaft fractures in Swedish children between 1987 and 2005. J Pediatr Orthop. 2011;31(5):512–9.
Powell EC, Tanz RR. Adjusting our view of injury risk: the burden of nonfatal injuries in infancy. Pediatrics. 2002;110(4):792–6.
Rennie L, Court-Brown CM, Mok JY, Beattie TF. The epidemiology of fractures in children. Injury. 2007;38(8):913–22.
Thygesen LC, Ersboll AK. When the entire population is the sample: strengths and limitations in register-based epidemiology. Eur J Epidemiol. 2014;29(8):551–8.
Landin LA. Fracture patterns in children. Analysis of 8,682 fractures with special reference to incidence, etiology and secular changes in a Swedish urban population 1950–1979. Acta Orthop Scand Suppl. 1983;202:1–109.
Brudvik C, Hove LM. Childhood fractures in Bergen, Norway: identifying high-risk groups and activities. J Pediatr Orthop. 2003;23(5):629–34.
Lyons RA, Delahunty AM, Kraus D, Heaven M, McCabe M, Allen H, Nash P. Children’s fractures: a population based study. Inj Prev. 1999;5(2):129–32.
Walsh SS, Jarvis SN, Towner EM, Aynsley-Green A. Annual incidence of unintentional injury among 54,000 children. Inj Prev. 1996;2(1):16–20.
Download references
Acknowledgements
Open access funding provided by UiT The Arctic University of Norway (incl University Hospital of North Norway) UiT the Arctic University of Norway funded costs for publication.
Author information
Authors and affiliations.
Department of Clinical Medicine, UiT the Artic University of Norway, Tromsø, Norway
Karen Rosendahl, Laura Tanturri de Horatio, Edvard J. Enoksen, Rien Avenarius & Thomas A. Augdal
Department of Radiology, University Hospital of North Norway, Pb 100, Tromsø, 9038, Norway
Karen Rosendahl, Laura Tanturri de Horatio & Thomas A. Augdal
Department of Imaging, IRCCS Bambino Gesù Children‘s Hospital, Rome, Italy
Laura Tanturri de Horatio
Pediatric Radiology Unit, Radiology Division, Diagnostic Department, University Hospitals of Geneva, Geneva, Switzerland
Celine Habre
Department of Clinical Radiology, Great Ormond Street Hospital for Children NHS Foundation Trust, Great Ormond Street, London, England
Susan C. Shelmerdine
Great Ormond Street Hospital for Children, UCL Great Ormond Street Institute of Child Health, London, England
NIHR Great Ormond Street Hospital Biomedical Research Centre, Bloomsbury, London, England
Department of Radiology, St. George’s Hospital, London, England
Division of General and Pediatric Radiology, Department of Biomedical Imaging and Image-Guided Therapy, Medical University of Vienna, Wien, Austria
Janina Patsch
Department of Pediatric Radiology, Karolinska University Hospital, Stockholm, Sweden
Department of Women’s and Children’s Health, Karolinska Institute, Solna, Sweden
University Library, Bergen University, Bergen, Norway
Regina K. Lein
Clinical Radiology Institute, University Medical Centre Ljubljana, Ljubljana, Slovenia
Faculty of Medicine, University of Ljubljana, Ljubljana, Slovenia
Section for Paediatric Radiology, Department of Radiology, Haukeland University Hospital, Bergen, Norway
Lene B. Laborie
Department of Clinical Medicine, University of Bergen, Bergen, Norway
Department of Radiology, “Reine Fabiola” Children’s University Hospital Université Libre de Bruxelles, Brussels, Belgium
Paolo Simoni
Department of Radiology and Nuclear Medicine, UMC, University of Amsterdam, Amsterdam, Netherlands
Rick R. van Rijn
Department of Oncology & Metabolism, University of Sheffield, Sheffield, England
Amaka C. Offiah
You can also search for this author in PubMed Google Scholar
Contributions
All conceptualized the study, R.K.L performed the literature search, K.R., S.S and L.T.d.H. screened the titles and abstracts, R.v.R and K.R. extracted data from the included papers, O.K, J.P and C.H performed the quality check using AXIS and K.R. drafted a first version of the manuscript. All authors reviewed the manuscript.
Corresponding author
Correspondence to Karen Rosendahl .
Ethics declarations
Ethics approval and consent to participate.
Ethical approval was not required for this review of publicly available data.
Consent for publication
All authors consented to publication.
Competing interests
The authors declare no competing interests.
Additional information
Publisher's note.
Springer Nature remains neutral with regard to jurisdictional claims in published maps and institutional affiliations.
Supplementary Information
Supplementary material 1., supplementary material 2., rights and permissions.
Open Access This article is licensed under a Creative Commons Attribution 4.0 International License, which permits use, sharing, adaptation, distribution and reproduction in any medium or format, as long as you give appropriate credit to the original author(s) and the source, provide a link to the Creative Commons licence, and indicate if changes were made. The images or other third party material in this article are included in the article's Creative Commons licence, unless indicated otherwise in a credit line to the material. If material is not included in the article's Creative Commons licence and your intended use is not permitted by statutory regulation or exceeds the permitted use, you will need to obtain permission directly from the copyright holder. To view a copy of this licence, visit http://creativecommons.org/licenses/by/4.0/ .
Reprints and permissions
About this article
Cite this article.
Rosendahl, K., de Horatio, L.T., Habre, C. et al. The incidence of fractures in children under two years of age: a systematic review . BMC Musculoskelet Disord 25 , 528 (2024). https://doi.org/10.1186/s12891-024-07633-5
Download citation
Received : 13 April 2023
Accepted : 27 June 2024
Published : 09 July 2024
DOI : https://doi.org/10.1186/s12891-024-07633-5
Share this article
Anyone you share the following link with will be able to read this content:
Sorry, a shareable link is not currently available for this article.
Provided by the Springer Nature SharedIt content-sharing initiative
BMC Musculoskeletal Disorders
ISSN: 1471-2474
- Submission enquiries: [email protected]
- General enquiries: [email protected]
Deep learning for lungs cancer detection: a review
- Open access
- Published: 08 July 2024
- Volume 57 , article number 197 , ( 2024 )
Cite this article
You have full access to this open access article
- Rabia Javed 1 ,
- Tahir Abbas 1 ,
- Ali Haider Khan 2 ,
- Ali Daud 3 ,
- Amal Bukhari 4 &
- Riad Alharbey 4
Abstract
Although lung cancer has been recognized to be the deadliest type of cancer, a good prognosis and efficient treatment depend on early detection. Medical practitioners’ burden is reduced by deep learning techniques, especially Deep Convolutional Neural Networks (DCNN), which are essential in automating the diagnosis and classification of diseases. In this study, we use a variety of medical imaging modalities, including X-rays, WSI, CT scans, and MRI, to thoroughly investigate the use of deep learning techniques in the field of lung cancer diagnosis and classification. This study conducts a comprehensive Systematic Literature Review (SLR) using deep learning techniques for lung cancer research, providing a comprehensive overview of the methodology, cutting-edge developments, quality assessments, and customized deep learning approaches. It presents data from reputable journals and concentrates on the years 2015–2024. Deep learning techniques solve the difficulty of manually identifying and selecting abstract features from lung cancer images. This study includes a wide range of deep learning methods for classifying lung cancer but focuses especially on the most popular method, the Convolutional Neural Network (CNN). CNN can achieve maximum accuracy because of its multi-layer structure, automatic learning of weights, and capacity to communicate local weights. Various algorithms are shown with performance measures like precision, accuracy, specificity, sensitivity, and AUC; CNN consistently shows the greatest accuracy. The findings highlight the important contributions of DCNN in improving lung cancer detection and classification, making them an invaluable resource for researchers looking to gain a greater knowledge of deep learning’s function in medical applications.
Avoid common mistakes on your manuscript.
1 Introduction
Cancer refers to the growth of abnormal tissues that are unwanted, it’s uncontrollable, and it spreads speedily in the body; if it is not treated well at the start, it spreads and affects other body organs also. In the health sector, the use of modern technology has contributed a lot, especially in the detection of lungs cancer. It helps the doctors to identify as well as properly treat a disease. Many deaths occur in the whole world due to lung cancer and due to this, it is one of the deadliest diseases known in the world. In 2020, according to the research, approximately 2.21 million cases were detected, and 1.8 million mortalities were caused by lungs cancer (Sharma 2022 ). The report presented by the World Health Organization (WHO) in 2020, shows that lungs cancer is the deadliest among all kinds of cancers, that is said according to the death rate that is calculated as 1.80 million (World Health Organization 2022 ). Figure 1 shows the details of extinction because of cancer in 2020 according to WHO Lungs cancer is one of those diseases in which early-stage diagnosis and disease management play a crucial role in proper treatment.
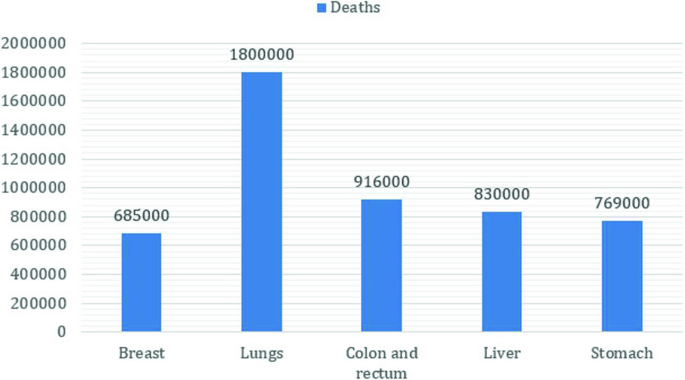
Global Distribution of Cancer-Related Deaths in 2020
Just like other cancers, the early detection of lungs cancer is mandatory due to which the chances of survival increase (Pathak et al. 2018 ). A large number of people affected by lung cancer cannot survive due to the delay in early detection, the overall survival rate of the patient is five years which is less than 20% (Roointan et al. 2019 ). Age is not a vital prognostic factor when it comes to the survival of patients (Hurria and Kris 2003 ). Both males and females fall prey to it. Men are more prone to lungs cancer than females According to research, the death rate in men due to lungs cancer is higher than females. (Chen et al. 2016 ). Factors contributing to increased lungs cancer cases include tobacco, smoke, viral infection, ionizing radiation (Cook et al. 1993 ; Esposito et al. 2010 ) air pollution, and unhealthy lifestyle. (Strak et al. 2017 ) History of chronic obstructive pulmonary disease (COPD) is the main factor that contributes to lungs cancer (Parris et al. 2019 ). The drastic increase in vehicles and bad smoking habits also play a crucial role. As tobacco is a primary factor, driving lungs cancer trend (Parascandola and Xiao 2019 ) so, the death toll due to lung cancer can be reduced by controlling tobacco usage (Field et al. 2013 ). Its symptoms include fatigue, difficulty in breathing, and persistent cough (Corner 2005 ; Mayo Clinic 2022 ). Apart from common symptoms, its symptoms vary from person to person, making its diagnosis quite tricky. It might be asymptomatic, and a person may have cancer without any sign (Quadrelli et al. 2015 ). Lack of symptoms at early stages leads to a late diagnosis of lungs cancer (Goebel et al. 2019 ). One of the most important cornerstones of human civilization is maintaining one's health, hence modern approaches to medical issues are required. The amount of information available in the form of lab tests, research papers, clinic reports, and other documents has increased due to advancements in the biomedical area (Riad Alharbey et al. 2022 ).
A lot of research has been performed by many researchers in different fields so that the accurate prediction and classification of lungs cancer can be increased. In recent studies, Initial screening of disease is performed by exhaled breath analysis which is non-invasive and inexpensive (Nardi-Agmon and Peled 2017 ). Different methods are used for the prediction of lungs cancer. In the detection process X-rays, CT, and MRI& PET scans are most used.
The classification of lungs cancer in its early stages (Basak and Nath 2017 ), and the chance of survival of the patient is opposite and inversely proportional to the disease. Tumor size determines the cancer stage. The cancer stage is measured by its spread in the body. The more the spread, the higher the stage. Mostly it’s not quite visible in the early stages; so, detection in the early stage is difficult (Das et al. 2020 ). But it’s quite easy to deal with it in the early stages as the disease progresses, it becomes complex to cope with it Fig. 2 . Depicts the stages of cancer.
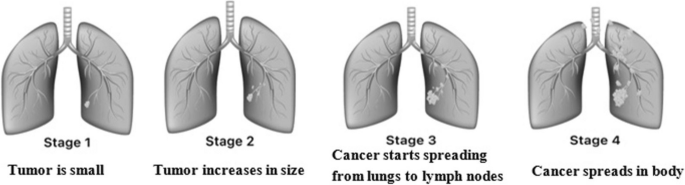
Progression stages of lung cancer—illustrating the different developmental phases"
Analysis of visual images is an efficient way to investigate the lungs tissues identify the stages of lungs cancer and classify these stages. However, it is difficult to categorize it by stages.However, by the usage of advanced deep learning methods, lungs cancer can be classified accurately. Figure 2 effectively depicts the progression of lungs cancer and categorizes it into stages. Deep learning algorithms are implemented to identify different types of lung cancer and categorize them. The most important and effective method to diagnose and the treatment of lung cancer is made possible by the initial step of disease detection within the lung tissue. Subsequently, various classifiers are used to accurately classify the identified cases into their respective stages Fig. 3 . Depicts how classification and prediction of lungs cancer.is performed using deep learning.
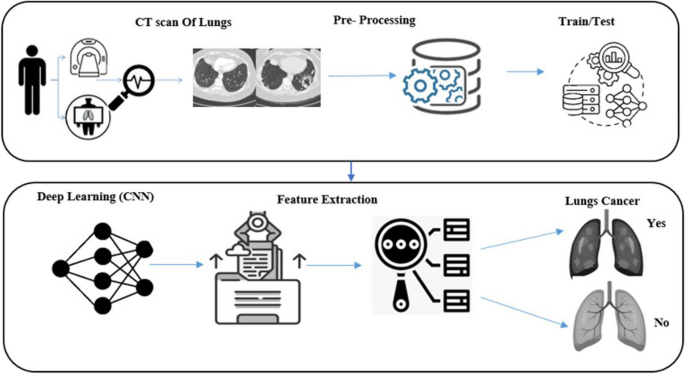
Deep Learning for the classification and detection of lungs cancer
Different therapies like chemotherapy and radiotherapy are performed for their treatment, but advanced lungs cancer is quite complex. CT is widely and commonly used for the detection purpose of lungs tumors, but it’s been closely observed that small nodules are mostly not predictive for lungs cancer (Horeweg et al. 2014 ). These are comparatively tricky and complicated to detect and treat. When it comes to classifying benign & malignant lesions CT has limited ability (Lardinois et al. 2003 ) Small lesions, having minimal contact with the chest wall are complicated and dealt with technically (Middleton et al. 2006 ). Chest wall structure, small blood vessels, airway walls, pulmonary structures (Lu et al. 2015 ), and tissues that are pretty like nodule makes the detection difficult, so it’s rather difficult to perform biopsy that leads to detection. A biopsy is performed for the evaluation of nodules (Lowe et al. 1998 ). The disease’s complexity and poor CT resolution sometimes lead to re-biopsy. Nodules are classified according to their type, size, and growth rate; it’s essential because it sets the direction of treatment. Nodules can be classified as the cavity, calcified & non-calcified (El-Baz et al. 2013 ), or as solid, non-solid, partially solid, and calcified (Massimo 2012 ). A partially solid tumor is a combination of non-solid and solid, the solidified lung tumors consist of a solid internal core. Lung nodules can also be classified as Benign and Malignant (Dhara et al. 2016 ; Silvestri MD et al. n.d. ; Wu et al. 2020 ; Zhang et al. 2019 )
Deep learning is contributing tremendously to healthcare (Esteva et al. 2019 ; Miotto et al. 2018 ; Mittal and Hasija 2019 ). Deep learning paves the way for fast and accurate detection and diagnosis of diseases (Mishra et al. 2020 ), leading to precise and exact treatment. Research shows that the algorithm of deep learning has shown its significance in the prediction, detection, and diagnosis with the classification of lung cancer; pulmonary nodules are closely observed. By incorporating different deep-learning approaches, the tumor, and the nodule features are captured and classified (Wu and Qian 2019 ). Big Data refers to incredibly massive data collections that are amenable to analysis to identify trends and patterns. Deep Learning is one method for data analysis that can be utilized to discover abstract patterns in large amounts of data (Gheisari et al. 2017 ). Advanced data representations and knowledge can be extracted with the help of deep learning (DL). Highly efficient DL methods aid in uncovering more buried information (Gheisari et al. 2023 ). CAD (Computer-aided design) is used for the screening of cancer in the early stages (Traverso et al. 2017 ). It is proven as a helping hand for doctors and radiologists (Yuan et al. 2006 ). State-of-the-art methods have been designed to develop automated processes. Coherent Anti-Stroke Raman Scattering (CARS) technique is used for sensitive investigations (Müller and Zumbusch 2007 ), and is also there to scan the lungs that capture the molecular movement and produce an image that helps to detect diseases accurately. Researchers have defined different classification models to detect & classify lungs cancer automatically (Nasrullah et al. 2019 ).
Using a deep learning algorithm, other techniques have been made to read and learn data representation from the unorganized (raw) data. Inner body details are examined, and valuable information is extracted from this data. Deep learning models, algorithms, and methods play a tremendous role in increasing accuracy and decreasing error in the classification of lungs cancer. Deep learning-based automatic segmentation is better than manual in many aspects (Liu et al. 2021 ) Deep learning helps to avoid misclassification, reduces error rate, provides high-quality images, and accurately predicts cancer. False-positive nodules are filtered out using different classifiers (Jiang et al. 2022 ). Accurate and high-quality images are directly proportional to the radiologist’s fast and accurate diagnostic decision. Deep learning methods are also incorporated to predict lungs cancer (Banerjee and Das 2021 ). Training images are provided, and features are extracted automatically. Comparatively, deep learning costs less than conventional CAD frameworks. Deep learning offers HD representation of the given input data, making the detection and identification process efficient and helping the radiologist. The image’s pixel directly contributes to cancer detection, as cancerous and non-cancerous areas are determined on the base of pixels. So, to diagnose accurately and the classification of disease, deep learning assists medical professionals in serving the healthcare system better. It helps to make accurate decisions regarding the disease. CNN design consists of multiple tiers, one of which being Convolutional Layers (CLs). By employing different kinds of convolution filters, the CL layers can extract distinct information from the images of cancer cells that are supplied to them (Manjula Devi et al. 2023 ). Under the methodology that is being described, the first step in the process is image processing, where preprocessing methods are used to improve the quality of medical images. The improved pictures next go through segmentation, which is an essential stage in identifying pertinent areas within lung imaging. Following identification, the regions are subjected to feature extraction, a process that involves the extraction of significant features to identify crucial patterns suggestive of lung cancer. The classification step, which uses a complex architecture called the Deep Convolutional Neural Network (DCNN), is where the classification process is most centrally located. To dynamically learn hierarchical features, this DCNN is composed of several convolutional layers, each of which has filters, activation functions, and pooling operations. Dense layers known as fully connected layers are another component of the architecture that handles the high-level characteristics that the convolutional layers have learned. The final output shows the findings of the categorization, which differentiates between various lung cancer classifications. The DCNN is an effective technique for accurately classifying lung cancer because its convolutional layers are essential for automatically learning complex patterns.
This study involves the following contributions to the field of medical science especially to the detection of lungs cancer in its early stages:
Provides the solution for the detection of lungs cancer in the field of healthcare.
Discussed different existing techniques and procedures.
Implemented deep learning algorithm and compared with the existing machine learning algorithms and compared the performance with the developed algorithm.
The designed technique is implemented on a large dataset and shows how to classify the features.
It shows the usability of Convolutional Neural Networks (CNN) in the field of artificial intelligence.
For future research it provides useful implementation and development techniques for the early detection of cancer disease.
The other parts of the literature survey are defined in the following sections. Imaging techniques for lungs cancer detection are presented in Section 2 . Section 3 includes the latest trends in lungs cancer detection, Section 4 offers the Research methodology of the opted research and the process of selecting research articles is given in Section 4 . Section 5 refers to the deep learning contribution towards lungs cancer classification. Section 6 refers to the Literature sources, and the research community’s contribution to the current field covering primary techniques and models used to classify, detect, and predict lungs cancer. Results that are obtained from the selected and extracted data are presented in Section 7 . Section 8 refers to the conclusion in which the state-of-the-art deep learning contribution towards lungs cancer classification is presented.
2 Imaging techniques for detection of lungs cancer
Different screening approaches are employed for the identification and screening of lung cancer (Schaefer-Prokop and Prokop 2002 ). These aid in the doctor's ability to see internal bodily processes and to gain an understanding of how internal organs function. To check for lung anomalies. There are several multimodality imaging techniques including positron emission tomography (PET), computer tomography (CT), Ultrasound, chest radiography (X-Ray), and magnetic resonance imaging (MRI) scans (Laal 2013 ; Tariq Hussain n.d. ) Fig. 4 shows a few techniques of Imaging.
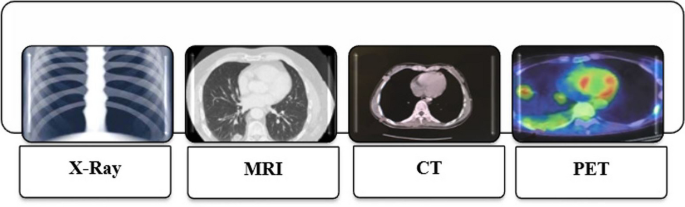
Modalities in lung cancer detection—a visual exploration of imaging techniques
3 Latest trends in lungs cancer detection
The primary purpose of the designed research is to demonstrate the methods and strategies employed in the deep learning categorization of lung cancer. Deep Learning algorithm is the most recent technique that helps medical professionals diagnose diseases and helps radiologists find difficult-to-diagnose conditions like lung cancer. The chosen articles demonstrate the most recent deep learning algorithms and their efficacy in the prediction and categorization of cancer. This paper presents different techniques defined in deep learning algorithms and concepts for this (Fig. 5 ).
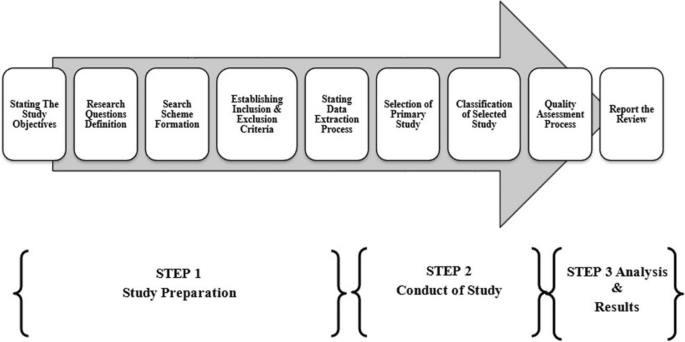
Sequential process of study execution
Convolutional Neural Network (CNN) consists of multiple layers. Convolutional layer that extracts features of image pooling layer which selects the feature. The third one is the fully connected or FC layer, its work is to combine those extracted features. Recurrent Neural Network (RNN) is suitable for sequential data and is mostly used for audio, video, and text. Deep Belief Network (DBN) consists of multiple RBMs. These are probabilistic generative models. DBN has many variants. Support Vector Machine (SVM) is a statistical theory-based algorithm. Artificial Neural Network (ANN) is structured just like human brains in which neurons are involved, that’s why it is known as a biological-inspired network. Deep Neural Network (DNN) is a new and advanced technique in the field of artificial intelligence, as it can also work for a complex nonlinear relationship. DNA-binding proteins have a close relationship with several human disorders, including AIDS, cancer, and asthma (Ali et al. 2022b ). DBP-DeepCNN would be beneficial in developing more promising therapeutic approaches for the management of chronic diseases (Ali et al. 2022a ) while patients with chronic depressive illness experience confusion in their social lives (Gheisari 2016 ). Integrating CC technology with wireless body area networks WBANs systems to create sensor-cloud infrastructure (S-CI) is helping the healthcare industry by enabling early detection of diseases and real-time patient monitoring (Masood et al. 2023 , 2018a ) while patient privacy should preserved (Masood et al. 2018b ). If a deep learning model is developed well, it may help prevent misdiagnosis and waste of time (Javed et al. 2023 ). Deep machine learning could be applied to the initial processing of images, the segmentation of images to emphasize the diagnostic objects under investigation, and the classification of these objects to ascertain their benign or malignant nature (Jamshaid Iqbal Janjua et al. 2022 ). It is challenging to predict human diseases, especially cancer, in order to deliver more effective and timely care. Cancer is a potentially deadly disease that affects the human body's many organs and systems (Abbas et al. 2023b )
4 Research methodology
For the selected research, a mapping study “Classification (lungs cancer)” analysis is chosen as a research methodology. Figure 6 illustrates the mapping process that has been followed. It consists of three steps that are as follows:
Step-I: Study Preparation
Step-II: Conduct of Study
Step-III: Analysis and Results of the Study
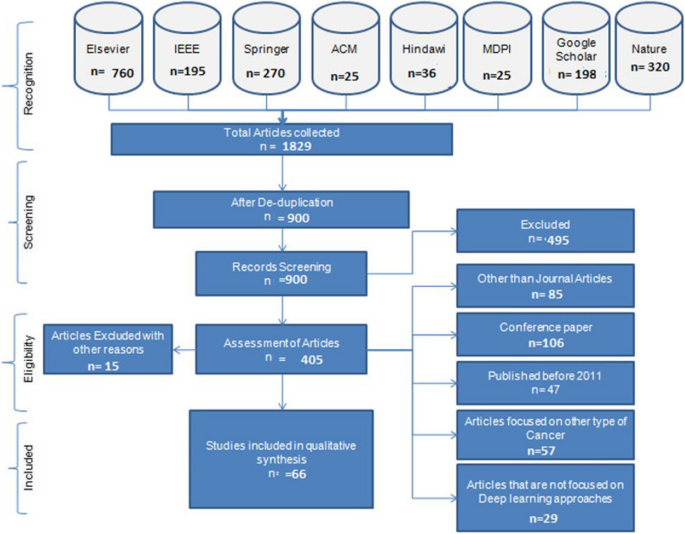
Visual representation of the systematic article selection process
In this study, a mapping study methodology is employed to conduct a systematic exploration of the literature on lung cancer classification using deep learning approaches. The mapping process, illustrated in Fig. 5 , is structured into three distinct steps: Study Preparation, Conduct of Study, and Analysis and Results of the Study. The main contribution of this paper is described below.
Conducted a comprehensive Systematic Literature Review (SLR) using deep learning approaches, which included a detailed analysis of pertinent literature in the field of lung cancer.
Categorized and synthesized the overall methodologies observed in the literature, offering readers a systematic overview of the strategies adopted in the domain of deep learning for lung cancer detection and analysis
Outlined the current state-of-the-art and the latest advancements in deep learning methodologies applied to lung cancer research, providing insights into cutting-edge techniques and emerging trends in the field.
Conducted a comprehensive Quality Assessment of the approaches used in the examined papers, guaranteeing a strong assessment framework to gauge the validity and dependability of the deep learning methods applied in lung cancer research.
Provided a comprehensive overview of deep learning methodologies specifically tailored to lung cancer research, consolidating the collective knowledge and advancements in the area for the benefit of researchers, practitioners, and stakeholders.
Study preparation, the first step, entails defining the research's scope, creating inclusion and exclusion criteria, and choosing a search technique. The implementation of the literature search, data extraction, classification, and synthesis of pertinent literature are then included in the Conduct of Study phase. Lastly, analyzing the identified literature, gauging the caliber of the included research, and extracting significant findings to guide the systematic review are all part of the Analysis and Results of the Study phase.
4.1 Research objectives
The main purpose of this research is to provide the scientific community with a systematic step-by-step review of the current research on lungs cancer by using the technique known as deep learning just like the recurrent neural networks (RNN), deep belief network (DBN), support vector machine (SVM), convolution neural networks (CNN) and the deep neural networks (DNN) etc.
4.2 Research questions
As part of this procedure, the questions related to this research are listed in Table 1 and are defined step by step to provide a more thorough understanding of the investigation. These research questions are accompanied by their motivations.
4.3 Search scheme
Following databases and scientific resources have been searched to get and gather the most relevant research papers and articles IEEE Digital Library, Springer, Elsevier, ACM Digital Library, Science Direct, and Google Scholar are the main repositories that were used to get the most relevant research articles.
4.4 Search string
The following search string was used to conduct the automatic search in the selected databases/scientific sources.
(“Classification” OR “Detection” OR “Prediction” OR “Diagnosis” OR “Analysis”) AND (“Lungs Cancer” OR “Lung Cancer” OR “Pulmonary Nodule” OR “Lungs Tumor” OR “Lung Nodule”) AND “Deep Learning” also known as “Deep Neural Network” alternate “DNN” also written as “DL”
4.5 Study selection procedure
The selection procedure is focused on identifying and recognizing those articles that effectively meet the goal of the study. These articles have been searched and gathered from different sources, so if the article is present in more than one source it is counted just once. After comprehensively investigating and examining titles, abstracts, and keywords, each paper is evaluated and its candidature in a study is determined. The search string is considered in deciding the inclusion and exclusion criteria. Duplicates are removed and articles not observing the search string are excluded.
4.6 Inclusion & exclusion principles for the research studies
For the chosen research Table 2 listed the inclusion and exclusion principles. Articles from journals focused on the classification of lungs cancer where deep learning algorithms, are incorporated, and published between 2015-2024 are collected While Articles that are focused on other types of cancer and do not incorporate deep learning are not included.
Research articles are collected from different geographical locations through a combination of online databases, we gathered articles for our study from various geographic places. Using precise terms and search parameters linked to our research topic, we conducted in-depth searches on academic databases including IEEE, Nature, Google Scholar, etc. Table 3 depicts the geographical locations of the articles selected for the study.
The research process is conducted according to the given flow diagram in Fig. 6 , which depicts the steps of gathering the research material, from identifying articles to selecting articles for further analysis.
It starts by gathering articles from well-reputed databases. Then the overall number is calculated. After that, the duplicate articles are removed, and initial screening is performed.
Articles that are not in the English language are excluded, and further assessment is performed in this step different criteria of exclusion are applied. The articles that are not from journals are excluded, conference papers, papers published before 2015, papers that are focused on other types of cancer, and articles that are not focused on deep learning are excluded. After excluding the papers with justification, 66 articles were chosen for further investigation.
Figure 7 displays a graphical depiction of the scientific databases where the search term was used, and articles were chosen.
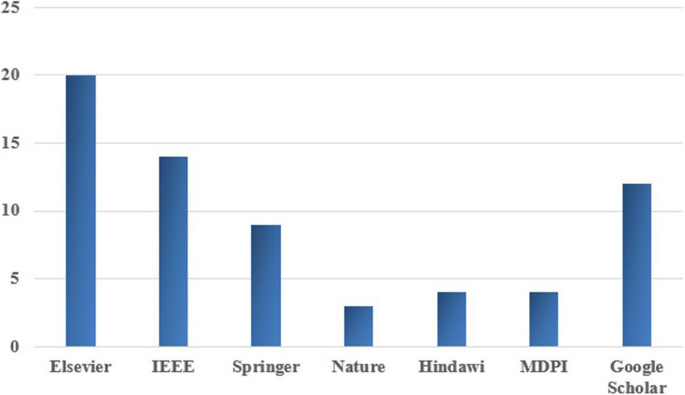
Distribution of articles selected from scientific databases
5 Quality assessment of study
Quality assessment is important in systematic reviews of literature as it determines the quality of the study that is included.
The solution to the problem is presented clearly in the paper. The answer could be yes (+ 1), No (0), somehow (0.5)
The contribution of the paper regarding the issue “Classification of lung cancer using deep learning is presented clearly. The answer could be yes (+ 1), No (0), somehow (0.5)
Limitations and future study are presented and defined clearly the answer could be yes (+ 1), No (0), somehow (0.5)
Result parameters are presented clearly, the answer could be yes (+ 1), No (0), somehow (0.5)
Table 4 presents a detailed Quality assessment score. In which selected articles along with their reference number are presented. These are evaluated based on the solution of the problem, contribution, limitation, future work, and results. Each question possesses one score. A total of 4, each article is evaluated and graded.
Table 5 presents a summary of the total scores. There is one paper that possesses a score of 2, 7 articles with 2.5. There are 13 articles with a score of 3, 25 articles with a score of 3.5, and 20 articles with a score of 4
6 Literature sources
To look over and explore the detection, classification, and prediction of lung cancer using deep learning, 66 relevant articles published by reliable sources were examined.
To ensure the credibility of the systematic literature review, credible journals are selected as sources and data collection. Moreover, a large number of adequate literature surveys exist for that kind of review Fig. 8 portrays the year-wise detail of collected articles.
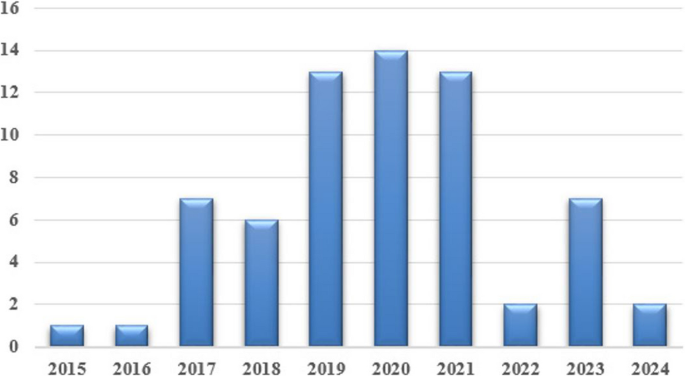
Annual article selection overview (2015–2024)
It’s evident that the selected articles are from 2015 to 2024 and the chart represents that 2020 is the maximum contributing year because the maximum number of articles falls in 2020.
The nature of the review was to present the work done on the topic of the classification of lungs cancer using different deep-learning methodologies. According to the collected data, it is observed that the most frequently used method is the convolutional Neural Network) CNN. Convolution neural networks (CNN) is also a technique used to solve deep learning problems; it consists of multiple layers. CNN is contributing a lot to Image processing and computer vision (Liu et al. 2019a ). It is to be noted that the article review was quite prejudiced to the articles published (2015–2024), and the articles that have “Deep Learning” used in titles. It is observed that multiple data sets have been used in research.
This shows that many diversities in data when it comes to training and testing on CNN. MRI, thoracic surgery data, X-ray, CT, PET image data, CARS images, breathing data, thoracic MR images, Whole Slide Imaging (WSI), Lymph Node Slides, H & E slides, and Histopathological. Table 6 presents the summary of the selected research articles. The datasets used in the research, and their descriptions are presented. The parameters that decide the form of classification, applied method or approach, and the feature extraction techniques used are provided after thoroughly examining the selected research articles.
Cancer image data is collected and presented in forms. It is observed that a CT scan is the most frequently used type of data.
The management of input image sizes is a critical consideration in deep learning field for lung CT-scan processing. Some models require a specific input size, which calls for preprocessing operations like scaling or cropping to fit the data into this preset dimension. Such modifications, however, run the danger of information loss or image distortion, which reduces the model's effectiveness. In contrast, to solve these problems more advanced and sophisticated methods are developed. Models can adjust to different image sizes using techniques like padding or spatial pyramid pooling, but they may also introduce noise or artifacts. The idea of picture pyramids also produces numerous image copies at varying scales, facilitating feature capture at varied levels of detail. Fully Convolutional Networks (FCNs) are also specially designed to support arbitrary input sizes. The decision between these methods depends on the particular deep learning architecture and the actual requirements of the task of medical image analysis, taking into account elements like computational complexity and the requirement to adapt to various input dimensions.
The process of lungs cancer/nodules detection or classification goes as follows: -
Pre-Processing: it is the first step which is used to take inputs in the form of MRI, Thoracic Surgery Data, X-ray, CT, PET Image Data, Breathing Data, Thoracic MR Images, Whole Slide, Imaging (WSI), Lymph Node Slides, Histopathological Cancer Images, CARS Images, H&E. Different Image processing/ feature extraction techniques are applied on the Input. Table 7 lists the various data types used in the reviewed studies.
Computed Tomography is used most of the time, because it’s been frequently used in our review techniques used for preprocessing includes FPSOCNN, Wavelet-Transform-Based Features, SIFT, LBP, ABF Zernike Moment, and HE, inceptionv3, LDA, ODNN, Novel Nodule Candidate Detection Method, Single-level discrete Two-Dimensional Wavelet Transform (2D-DWT), Fractional-Order Darwinian Particle Swarm Optimization, Two-Dimensional Discrete Fourier transform (2D-DFT), U-Net, Back-Propagation, Deep Learning Architectures, Gradient Descent, SIFT + LBP, Swarm Algorithms, Bipartite Undirected Graphical Models (RBMs), including Alex, Deep (ConvNet),Intensity features + SVM Support Vector Machines, Transfer Learning Networks, Hybrid Geometric Texture Feature Descriptor, FODPSO CARS, VGG16,Wiener Filter, VGG19, Three-Dimensional (3D) CNN model, CNN Region-Of-Interest (ROI), Median Filter, Gaussian Filter, Gabor Filter, Knowledge-Based Collaborative (KBC) sub-mode, ResNet-50 networks, ProNet, RadNet, UB open-source software ITK-Snap, 3D Stereoscopic Planning System, IMR, Maximum Intensity Projection Technique, Based On Lung-RADS version 1.1, Three Reconstruction Kernels. Multi-Scale Dilated Residual Representation Block Size-Related (SR-DiRes) &Multi-Mask Convolution Representation Block (ConvRB), Multi-Scale LBP, AP Algorithms, Affinity Propagation (AP) Algorithm, Deep Convolution Neural Network (DCNN) Architecture, Radiomics-Based Analysis, Hot-Spot ROI-based DCE Kinetic Analysis, Deep Dueling Q-network, Hierarchical Deep Q-Networks, Radiomics Deep Q-Network, Weighted Mean Histogram Equalization, Dense PriNet, Multi-and single model method, Pixel-Wise Segmentation based On FCN ,Multi-Model, Weakly Supervised Learning Methods, HALO Tissue Classifier Analysis Module (Random Forest Algorithm), HALO AI (CNN, VGG network),Context-Aware Feature Selection And Aggregation, Graph Regularized Sparse MTL,K-means Algorithm, Extensive Data Augmentation,LUNA16 Pulmonary Nodule Annotations, Multi-Group Patch-Based Learning System, Diffusion (VED) and a Vessel filter, Integrated Deep Learning , Region Growth Algorithm, fuzzy logic and a NN, HE hybrid learning algorithm, ACC, VEL, Correlation Analysis Algorithm, PCA, (GSEA)Gene-Set Enrichment Analysis, ReLU Activation Function, CVAE-GAN, DenseNet using CoxPH, CNN model, ImageNet, Score-CAM Maximum Intensity Projection (MIP).,Multi-Scale Convolution Image Feature, Novel 15-Layer 2D Deep CNN architecture Hyperparameter Tuning, vector Quantization(VQ) algorithm, Gaussian noise model-based collaborative Wiener Filtering (GNM-CWF),Self-Adaptive Online VQ algorithm Residual Learning Denoising Model (DR-Net),Feature fusion strategy called DCA, Curriculum Learning, And Transfer Learning, Rival Convolutional Neural Network Models (setio-CNN and Over Feat), Taguchi-based CNN, combination of Deep Residual Learning, The Trial And Error Method, ACL algorithm, Converged Search and Rescue (CSAR) Algorithm, Novel Wilcoxon Signed-Rank Gain Preprocessing, Hybrid Spiral Optimization Intelligent-Generalized Rough Set approach, AI-based noninvasive radiomics biomarkers, SVM Principal Component Analysis (PCA), Multilevel Brightness-Preserving approach,. Numerous segmentation methods and approaches are then applied.
Segmentation contributes to the feature extraction process; it prepares the extracted data for classification.
Classification: - Classification is the phase where the prepared, feature extracted and segmented data is classified into different categories that may be abnormal or normal, benign, or malignant, LUAD or LUSC. After the detection of cancer nodules or pulmonary nodules which confirm that the disease is present or not of lungs cancer further classification is performed to classify these nodules into solid, calcified, partially solid, perifissural, and speculated.
Several classifiers are used in our study which includes; Fuzzy Particle Swarm Optimization (FPSO),Confusion matrix, Forest Classifiers, Back-Propagation, SVM or Naïve Bayes classifiers, Cross Entropy loss and Transfer Learning, RMS Prop-optimization method, Modified Gravitational Search Algorithm (MGSA),Multi-Channel CNN, Deep Learning and Swarm Intelligence, DBN and CNN Deep Learning, Convolutional Network Architecture, FODPSO, CARS & Deep learning, GoogleNet Inception v3 CNN architecture, Deep Learning Approach Based On Stacked Autoencoder and Softmax, CADe systemVGG19architectureand SVM classifier, Multi-View Knowledge-Based Collaborative (MV-KBC) Deep Model, Long Short-Term Memory/LSTM, Recurrent Neural Network/RNN,CNN Cancer Risk Prediction Model, triplet, DCNN AlexNet, watershed segmentation Banarization, Auto Encoder/AE &General Adversarial Networks/GAN, Deep Belief Network/DBN, Random Forest Classifier, Deep Quality Model, GG-net architecture, Convolutional Neural Networks with a U-net architecture, Recurrent Neural Networks (RNN), 3D Deep Learning and Radiomics, Core-Ring Blocks Residual Estimation with size-related damper block deep prediction model, GG-net architecture, Conditional Random Framework, SVM anti-PD-1 response prediction by H&E,DCNN CAD system, DCE Kinetic Parameters, Convolutional Long Short Term Memory (CLSTM) Network, DCE-MRI,Value-based Reinforcement Learning Approach, DSRL, Deep Successor Q-Network, Profuse Clustering Technique (IPCT),Deep. Learning Instantaneously Trained Neural Network (DITNN), deep Q-network and hierarchical deep Q-network, Explosion-Trained Deep Learning Neural Network (DITNN),CADe / CADx models, Deep Learning Classifier (Lymphoid Follicle CNN - LFCNN),3D Neural Network, Fully Convolutional Network (FCN),FCN, ScanNet, proportion-SVM, Adaptive Hierarchical Heuristic Mathematical Model (AHHMM),K-mean algorithm, 3D fully CNN based on the V-Net architecture, Four-Channel CNN, corrective lung contour, Wilcoxon Signed Generative DL (WS-GDL)Hyper-Parameter Tuning Algorithm, Multi-Scene Deep Learning Framework (MSDLF),Normalized Spherical Sampling, LSTM algorithms, NFNet, Fast R-CNN, Intra- And Inter-Fraction Fuzzy Deep Learning (IIFDL),deep learning-based radiogenomic framework-net, EfficientNet, CoxPH and CoxCC, LdcNet, Converged Search and Rescue Algorithm, Deep Gaussian Mixture Model in region-based CNN[DGMM-RBCNN], Lung-Deep System, Novel Nodule CADeCNN, INC classification, (DFD-Net), Two-path CNN with feature fusion DFD-Nets, Curriculum learning, 3D (DCNNs), Taguchi-based CNN, Fuzzy C-Ordered Means (FCOM) with ECN, Enhanced Capsule Networks (ECN), Lung Cancer Prediction (LCP-CNN), Generative Deep Learning, Survival Neural Network model, machine learning peculated known as k-nearest, KNN neighbors and SVM on CNN Ensemble Classifier and Improved DNN.
7 Literature synthesis: unveiling patterns and insights
Deep Convolutional Neural Networks (DCNN) is the best technique to detect lungs cancer in the field of machine learning. The highest levels of accurate lung cancer case classification were continuously attained using DCNN. The ability to make accurate diagnoses, a critical component in healthcare, is shown by this improved accuracy. Additionally, DCNN demonstrated exceptional specificity, reducing the incidence of false positives. In healthcare contexts, this precision is crucial since it lessens the possibility of misdiagnosing non-cancerous patients as cancer. The remarkable sensitivity of DCNN enabled the identification of sizable actual lung cancer cases. The potential for early identification and intervention, which are essential for enhancing patient outcomes, is increased by this high sensitivity. Finally, DCNN demonstrated impressive accuracy in detecting lung cancer. Finally, DCNN demonstrated remarkable accuracy in diagnosing lung cancer, reducing the possibility of incorrect diagnosis. These findings demonstrate how well DCNN performs in automatically extracting complex patterns from medical images, which helps in the accurate and reliable identification of lung cancer. DCNN stands out as the leading machine learning technology, to improve the accuracy and reliability of lung cancer detection in clinical practice due to its exceptional performance in accuracy, specificity, sensitivity, and precision. To promote medical diagnostics in this crucial area, we encourage continued investigation and development of DCNN-based techniques.
In the study, 66 research articles were carefully examined and their methods were analyzed. In Table 8 , the results of this evaluation procedure are collated and summarized. Important details including reference numbers, research methodology, and performance measures like the F1 score, accuracy, precision, sensitivity, and AUC are included in this table. Table 8 is a useful tool for comparing and assessing the research findings because these metrics are significant indicators of the efficacy and dependability of the various methodologies covered in the examined papers. The major limitation of current studies is mostly the size of the data sets. There is potential for improvement because there isn't a finished product or global standard for cancer detection and prediction. To ascertain the accuracy of these models, researchers need to gather up-to-date and new data, employ various deep learning and machine learning techniques, and combine new and old data. Early cancer detection can benefit millions of people. To detect cancer, there is no established standard or finished product. While deep learning has promising opportunities for lung cancer detection, there are important gaps that need to be filled. The generalizability of current findings is frequently problematic while accurate feature extraction is also crucial to be handled. Certain populations in the actual world may not respond well to models that were trained on their particular datasets. Furthermore, characterization may be eclipsed by an emphasis on detection. Some models are quite good at detecting nodules, but they may not give information about the tumor, which makes it more difficult to diagnose patients early and accurately. In addition, the "black box" nature of sophisticated deep learning models and worries about data security and privacy persist, making it challenging to comprehend how these models make decisions. Most of the research frequently runs into issues with feature extraction, making it difficult to identify pertinent elements that are essential for accurate prediction. These restrictions make it more difficult to do the thorough study needed to produce accurate and trustworthy detection results. In order to advance deep learning models' efficacy and dependability in lung cancer detection and eventually enhance patient outcomes and diagnostic accuracy, it is imperative that these issues be resolved. Despite these shortcomings, scientists are working hard to fill in the gaps Closing the existing gaps is critical to the future of deep learning for lung cancer detection. Researchers are focusing on tumor characterization rather than merely detection, enhancing generalizability through transfer learning, and advanced approaches. Strong data security protocols and resolving any biases in training data are also essential. Lastly, to stay up to date with the changing features of cancer, ongoing learning will be crucial. Deep learning has the potential to transform lung cancer detection and result in earlier diagnoses and better patient outcomes by overcoming these obstacles.
Different algorithms used for classification and detection purposes perform differently in terms of performance CNN surpasses the others.
8 Conclusion
Lung cancer is a serious and sometimes fatal disease that necessitates early discovery to effectively treat it. This work emphasizes how deep learning—specifically, the application of Convolutional Neural Networks, or CNN—is essential to changing the face of medical diagnosis. Deep learning techniques reduce the workload of healthcare professionals by automating the identification and categorization of lung cancer. This technological advancement improves the precision and efficacy of diagnosis. In the context of lung cancer research, this paper offers an extensive Systematic Literature Review (SLR) that makes use of deep learning approaches. It benefits researchers, practitioners, and stakeholders by classifying and synthesizing methodologies, outlining the state-of-the-art, conducting a thorough Quality Assessment, and offering a customized overview of deep learning approaches for lung cancer research. Our thorough analysis examines the several deep learning methods used in the identification and categorization of lung cancer across a range of imaging modalities, including MRIs, CT scans, and X-rays. Notably, we ensure a current overview by concentrating on the years 2015 to 2024 and obtaining information from credible journals. The study highlights the critical function that Deep Convolutional Neural Networks (DCNN) fulfill in the field of deep learning techniques. In particular, DCNN is the recommended method in the Convolutional Neural Network (CNN) architecture because of its unique multi-layered architecture, which makes direct feature learning from lung nodule images possible. The main reason why DCNN is so effective at obtaining the best accuracy is that it has the innate capacity to learn weights automatically. These models can significantly boost the efficiency and accuracy of lung cancer classification by the automatic learning of pertinent features, which improves diagnostic procedures in clinical settings. The research thoroughly assesses several performance measures, such as AUC, sensitivity, specificity, accuracy, and precision. When it comes to the classification of lung cancer, DCNN frequently performs more accurately than other algorithms. Even with these noteworthy successes, there are still difficulties, especially when managing large-volume datasets. This study presents the classification process on multiple data sets and multiple classifiers are used. The focus of the study is to present different deep learning algorithms and approaches to classify lungs cancer Nevertheless, there are still some challenges that still exist, most of them are related to the size of datasets. This study will help the researchers to better understand the existing deep-learning techniques and procedures to classify lungs cancer. This work lays the groundwork for future advancements in the field by providing a comprehensive grasp of the state-of-the-art deep learning methods for the categorization of lung cancer. Lung cancer diagnosis could be revolutionized by deep learning, however there remain obstacles because of the scale of data sets and the absence of an international standard. Current data must be gathered, deep learning and machine learning methods must be used, and both new and old data must be combined. Generalizability, characterization, data security, and privacy issues are lacking, nonetheless. Notwithstanding these obstacles, researchers are concentrating on tumor characterization, improving generalizability via transfer learning, and addressing biases in training data in an effort to close these gaps. Continual learning is also crucial to stay updated with changing cancer features.
References
Abbas S, Issa GF, Fatima A et al (2023) Fused Weighted Federated Deep Extreme Machine Learning Based on Intelligent Lung Cancer Disease Prediction Model for Healthcare 5.0. Int J Intell Syst 2023:1–14. https://doi.org/10.1155/2023/2599161
Article Google Scholar
Abbas T, Fatima A, Shahzad T et al (2023) Secure IoMT for Disease Prediction Empowered With Transfer Learning in Healthcare 5.0, the Concept and Case Study. IEEE Access 11:39418–39430. https://doi.org/10.1109/access.2023.3266156
Abbas Q (2017) Lung-Deep: a Computerized Tool for detection of lung nodule patterns using deep learning algorithms detection of lung nodules patterns. Int J Adv Comput Sci Appl 8. https://doi.org/10.14569/ijacsa.2017.081015
Affonso C, de Nedjah NL (2020) Detection and classification of pulmonary nodules using deep learning and swarm intelligence. Multimedia Tools Appl 79:15437–15465. https://doi.org/10.1007/s11042-019-7473-z
Alharbey R, Kim JI, Daud A et al (2022) Indexing important drugs from medical literature. Scientometrics 127:2661–2681. https://doi.org/10.1007/s11192-022-04340-7
Ali F, Kumar H, Patil S et al (2022) DBP-DeepCNN: Prediction of DNA-binding proteins using wavelet-based denoising and deep learning. Chemom Intell Lab Syst 229:104639–104639. https://doi.org/10.1016/j.chemolab.2022.104639
Ali F, Kumar H, Patil S et al (2022) Target-DBPPred: An intelligent model for prediction of DNA-binding proteins using discrete wavelet transform based compression and light eXtreme gradient boosting. Comput Biol Med 145:105533–105533. https://doi.org/10.1016/j.compbiomed.2022.105533
Ardila D, Kiraly AP, Bharadwaj S et al (2019) Author Correction: End-to-end lung cancer screening with three-dimensional deep learning on low-dose chest computed tomography. Nat Med 25:1319–1319. https://doi.org/10.1038/s41591-019-0536-x
Asuntha A, Srinivasan A (2020) Deep learning for lung Cancer detection and classification. Multimedia Tools Appl 79:7731–7762. https://doi.org/10.1007/s11042-019-08394-3
Banerjee N, Das S (2021) Lung cancer prediction in deep learning perspective
Basak P, Nath A (2017) Detection of different stages of lungs cancer in CT-scan images using image processing techniques. Int J Innov Res Comput Commun Eng 2320–9798
Chae KJ, Jin GY, Ko SB et al (2020) Deep Learning for the Classification of Small (≤2 cm) Pulmonary Nodules on CT Imaging: A Preliminary Study. Acad Radiol 27:e55–e63. https://doi.org/10.1016/j.acra.2019.05.018
Chaunzwa TL, Hosny A, Xu Y et al (2021) Deep learning classification of lung cancer histology using CT images. Sci Rep 11. https://doi.org/10.1038/s41598-021-84630-x
Chen W, Zheng R, Baade PD et al (2016) Cancer statistics in China, 2015. CA: A Cancer J Clin 66:115–132. https://doi.org/10.3322/caac.21338
Ciompi F, Chung K, van Riel SJ et al (2017) Towards automatic pulmonary nodule management in lung cancer screening with deep learning. Scientific Reports 7. https://doi.org/10.1038/srep46479
Cook RM, Miller YE, Bunn PA (1993) Small cell lung cancer: etiology, biology, clinical features, staging, and treatment. Curr Probl Cancer 17:69–141. https://doi.org/10.1016/0147-0272(93)90010-y
Corner J (2005) Is late diagnosis of lung cancer inevitable? Interview study of patients’ recollections of symptoms before diagnosis. Thorax 60:314–319. https://doi.org/10.1136/thx.2004.029264
Coudray N, Ocampo PS, Sakellaropoulos T et al (2018) Classification and mutation prediction from non–small cell lung cancer histopathology images using deep learning. Nat Med 24:1559–1567. https://doi.org/10.1038/s41591-018-0177-5
Cui X, Zheng S, Heuvelmans MA et al (2022) Performance of a deep learning-based lung nodule detection system as an alternative reader in a Chinese lung cancer screening program. Eur J Radiol 146:110068–110068. https://doi.org/10.1016/j.ejrad.2021.110068
Das P, Das B, Dutta HS (2020) Prediction of lungs cancer using machine learning
Dhara AK, Mukhopadhyay S, Dutta A et al (2016) A Combination of Shape and Texture Features for Classification of Pulmonary Nodules in Lung CT Images. J Digit Imaging 29:466–475. https://doi.org/10.1007/s10278-015-9857-6
Doppalapudi S, Qiu RG, Badr Y (2021) Lung cancer survival period prediction and understanding: Deep learning approaches. Int J Med Informatics 148:104371. https://doi.org/10.1016/j.ijmedinf.2020.104371
El-Baz A, Elnakib A, Abou El-Ghar M et al (2013) Automatic Detection of 2D and 3D Lung Nodules in Chest Spiral CT Scans. Int J Biomed Imaging 2013:1–11. https://doi.org/10.1155/2013/517632
Elnakib A, Amer HM, Abou-Chadi FE (2020) Early Lung Cancer Detection using Deep Learning Optimization. Int J Online Biomed Eng (iJOE) 16:82. https://doi.org/10.3991/ijoe.v16i06.13657
Esposito L, Conti D, Ailavajhala R et al (2010) Lung Cancer: Are we up to the Challenge? Curr Genom 11:513–518. https://doi.org/10.2174/138920210793175903
Esteva A, Robicquet A, Ramsundar B et al (2019) A guide to deep learning in healthcare. Nat Med 25:24–29. https://doi.org/10.1038/s41591-018-0316-z
Field JK, Oudkerk M, Pedersen JH, Duffy SW (2013) Prospects for population screening and diagnosis of lung cancer. The Lancet 382:732–741. https://doi.org/10.1016/s0140-6736(13)61614-1
Gheisari M, Wang G, Alam Bhuiyan MdZ (2017) A Survey on deep learning in big data. https://doi.org/10.1109/CSE-EUC.2017.215
Gheisari M, Ebrahimzadeh F, Rahimi M et al (2023) Deep learning: applications, architectures, models, tools, and frameworks: a comprehensive survey. CAAI Trans Intell Technol. https://doi.org/10.1049/cit2.12180
Gheisari M (2016) The Effectiveness of schema therapy integrated with neurological rehabilitation methods to improve executive functions in patients with chronic depression. Health Sci J 10
Goebel C, Louden CL, Mckenna R et al (2019) Diagnosis of Non-small Cell Lung Cancer for Early Stage Asymptomatic Patients. Cancer Genom - Proteom 16:229–244. https://doi.org/10.21873/cgp.20128
Gu Y, Chi J, Liu J et al (2021) A survey of computer-aided diagnosis of lung nodules from CT scans using deep learning. Comput Biol Med 137:104806. https://doi.org/10.1016/j.compbiomed.2021.104806
Guo Y, Song Q, Jiang M et al (2021) Histological Subtypes Classification of Lung Cancers on CT Images Using 3D Deep Learning and Radiomics. Acad Radiol 28:e258–e266. https://doi.org/10.1016/j.acra.2020.06.010
Heuvelmans MA, van Ooijen PMA, Ather S et al (2021) Lung cancer prediction by Deep Learning to identify benign lung nodules. Lung Cancer 154:1–4. https://doi.org/10.1016/j.lungcan.2021.01.027
Hoang Ngoc Pham H, Futakuchi M, Bychkov A et al (2019) Detection of Lung Cancer Lymph Node Metastases from Whole-Slide Histopathologic Images Using a Two-Step Deep Learning Approach. Am J Pathol 189:2428–2439. https://doi.org/10.1016/j.ajpath.2019.08.014
Horeweg N, van Rosmalen J, Heuvelmans MA et al (2014) Lung cancer probability in patients with CT-detected pulmonary nodules: a prespecified analysis of data from the NELSON trial of low-dose CT screening. Lancet Oncol 15:1332–1341. https://doi.org/10.1016/S1470-2045(14)70389-4
Hu J, Cui C, Yang W et al (2021) Using deep learning to predict anti-PD-1 response in melanoma and lung cancer patients from histopathology images. Translational Oncol 14:100921. https://doi.org/10.1016/j.tranon.2020.100921
Hurria A, Kris MG (2003) Management of Lung Cancer in Older Adults. CA: A Cancer J Clin 53:325–341. https://doi.org/10.3322/canjclin.53.6.325
Hussein S, Kandel P, Bolan CW et al (2019) Lung and Pancreatic Tumor Characterization in the Deep Learning Era: Novel Supervised and Unsupervised Learning Approaches. IEEE Trans Med Imaging 38:1777–1787. https://doi.org/10.1109/tmi.2019.2894349
Jamshaid Iqbal Janjua, Tahir Abbas Khan, Nadeem M (2022) Chest x-ray anomalous object detection and classification framework for medical diagnosis. 2022 International conference on information networking (ICOIN). https://doi.org/10.1109/icoin53446.2022.9687110
Javed R, Abbas T, Jamshaid Iqbal Janjua et al (2023) wrist fracture prediction using transfer learning, a case study. J Popul Ther Clin Pharmacol 30. https://doi.org/10.53555/jptcp.v30i18.3161
Jena SR, George ST, Ponraj DN (2021) Lung cancer detection and classification with DGMM-RBCNN technique. 33:15601–15617. https://doi.org/10.1007/s00521-021-06182-5
Jiang H, Ma H, Qian W et al (2017) An Automatic Detection System of Lung Nodule Based on Multigroup Patch-Based Deep Learning Network. IEEE J Biomed Health Inform 22:1227–1237. https://doi.org/10.1109/JBHI.2017.2725903
Jiang W, Zeng G, Wang S et al (2022) Application of Deep Learning in Lung Cancer Imaging Diagnosis. J Healthc Eng 2022:1–12. https://doi.org/10.1155/2022/6107940
Jung H, Kim B, Lee I et al (2018) Classification of lung nodules in CT scans using three-dimensional deep convolutional neural networks with a checkpoint ensemble method. BMC Med Imaging 18. https://doi.org/10.1186/s12880-018-0286-0
Kumar V, Bakariya B (2021) Classification of malignant lung cancer using deep learning. J Med Eng Technol 45:85–93. https://doi.org/10.1080/03091902.2020.1853837
Kumar Swain A, Swetapadma A, Kumar Rout J, Kumar Balabantaray B (2024) Classification of non-small cell lung cancer types using sparse deep neural network features. Biomed Signal Process Control 87. https://doi.org/10.1016/j.bspc.2023.105485
Laal M (2013) Innovation Process in Medical Imaging. Procedia Soc Behav Sci 81:60–64. https://doi.org/10.1016/j.sbspro.2013.06.388
Lakshmanaprabu SK, Mohanty SN, Shankar K et al (2019) Optimal deep learning model for classification of lung cancer on CT images. Future Gener Comput Syst 92:374–382. https://doi.org/10.1016/j.future.2018.10.009
Lang N, Zhang Y, Zhang E et al (2019) Differentiation of spinal metastases originated from lung and other cancers using radiomics and deep learning based on DCE-MRI. Magn Reson Imaging 64:4–12. https://doi.org/10.1016/j.mri.2019.02.013
Lanjewar MG, Panchbhai KG, Charanarur P (2023) Lung Cancer detection from CT scans using modified DenseNet with feature selection methods and ML classifiers. Expert Syst Appl 119961. https://doi.org/10.1016/j.eswa.2023.119961
Lardinois D, Weder W, Hany TF et al (2003) Staging of Non–Small-Cell Lung Cancer with Integrated Positron-Emission Tomography and Computed Tomography. N Engl J Med 348:2500–2507. https://doi.org/10.1056/nejmoa022136
Li Y, Zhang L, Chen H, Yang N (2019) Lung Nodule Detection With Deep Learning in 3D Thoracic MR Images. IEEE Access 7:37822–37832. https://doi.org/10.1109/access.2019.2905574
Li Z, Zhang J, Tan T et al (2020) Deep learning methods for lung cancer segmentation in whole-slide histopathology images -- the ACDC@LungHP Challenge 2019. arXiv (Cornell University). https://doi.org/10.48550/arxiv.2008.09352
Lin C-J, Li Y-C (2020) Lung Nodule Classification Using Taguchi-Based Convolutional Neural Networks for Computer Tomography Images. Electronics 9:1066. https://doi.org/10.3390/electronics9071066
Liu Y, Wang H, Gu Y, Lv X (2019) Image classification toward lung cancer recognition by learning deep quality model. J Vis Commun Image Represent 63:102570. https://doi.org/10.1016/j.jvcir.2019.06.012
Liu Z, Yao C, Yu H, Wu T (2019) Deep reinforcement learning with its application for lung cancer detection in medical Internet of Things. Futur Gener Comput Syst 97:1–9. https://doi.org/10.1016/j.future.2019.02.068
Liu S, Yao W (2022) Prediction of lung cancer using gene expression and deep learning with KL divergence gene selection. BMC Bioinformatics 23. https://doi.org/10.1186/s12859-022-04689-9
Liu X, Li K-W, Yang R, Geng L-S (2021) Review of deep learning based automatic segmentation for lung cancer Radiotherapy. Front Oncol 11. https://doi.org/10.3389/fonc.2021.717039
Lowe VJ, Fletcher JW, Gobar L et al (1998) Prospective investigation of positron emission tomography in lung nodules. J Clin Oncol 16:1075–1084. https://doi.org/10.1200/jco.1998.16.3.1075
Lu L, Tan Y, Schwartz LH, Zhao B (2015) Hybrid detection of lung nodules on CT scan images. Med Phys 42:5042–5054. https://doi.org/10.1118/1.4927573
Manjula Devi R, Dhanaraj RK, Pani SK et al (2023) An improved deep convolutionary neural network for bone marrow cancer detection using image processing. Inf Med Unlocked 101233. https://doi.org/10.1016/j.imu.2023.101233
Masood I, Wang Y, Daud A et al (2018) Towards Smart Healthcare: Patient Data Privacy and Security in Sensor-Cloud Infrastructure. Wirel Commun Mob Comput 2018:1–23. https://doi.org/10.1155/2018/2143897
Masood I, Wang Y, Daud A et al (2018) Privacy management of patient physiological parameters. Telematics Inform 35:677–701. https://doi.org/10.1016/j.tele.2017.12.020
Masood I, Daud A, Wang Y et al (2023) A blockchain-based system for patient data privacy and security. Multimedia Tools Appl. https://doi.org/10.1007/s11042-023-17941-y
Massimo B (2012) A classification of pulmonary nodules by CT scan. https://doi.org/10.3332/ecancer.2012.260
Masud M, Sikder N, Nahid A-A et al (2021) A Machine Learning Approach to Diagnosing Lung and Colon Cancer Using a Deep Learning-Based Classification Framework. Sensors 21:748. https://doi.org/10.3390/s21030748
Mayo Clinic (2022) Cancer - Symptoms and Causes. In: Mayo Clinic. https://www.mayoclinic.org/diseases-conditions/cancer/symptoms-causes/syc-20370588
Middleton WD, Teefey SA, Dahiya N (2006) Ultrasound-Guided Chest Biopsies. Ultrasound Q 22:241–252. https://doi.org/10.1097/01.ruq.0000237258.48756.94
Miotto R, Wang F, Wang S et al (2018) Deep Learning for healthcare: review, Opportunities and Challenges. Brief Bioinform 19:1236–1246. https://doi.org/10.1093/bib/bbx044
Mishra S, Dash A, Jena L (2020) Use of deep learning for disease detection and diagnosis. 903. https://doi.org/10.1007/978-981-15-5495-7_10
Mittal S, Hasija Y (2019) Deep Learning Techniques for Biomedical and Health Informatics. Stud Big Data 68:57–77. https://doi.org/10.1007/978-3-030-33966-1_4
Müller M, Zumbusch A (2007) Coherent anti-Stokes Raman Scattering Microscopy. ChemPhysChem 8:2156–2170. https://doi.org/10.1002/cphc.200700202
Nam JG, Park S, Hwang EJ et al (2019) Development and Validation of Deep Learning–based Automatic Detection Algorithm for Malignant Pulmonary Nodules on Chest Radiographs. Radiology 290:218–228. https://doi.org/10.1148/radiol.2018180237
Naqi SM, Sharif M, Jaffar A (2018) Lung nodule detection and classification based on geometric fit in parametric form and deep learning. Neural Comput Appl 32:4629–4647. https://doi.org/10.1007/s00521-018-3773-x
Nardi-Agmon I, Peled N (2017) Exhaled breath analysis for the early detection of lung cancer: recent developments and future prospects. Lung Cancer: Targets Ther 8:31–38. https://doi.org/10.2147/lctt.s104205
Nasrullah, Sang J, Mohammad Khursheed Alam, Xiang H (2019) Automated detection and classification for early stage lung cancer on CT images using deep learning. https://doi.org/10.1117/12.2520333
Nibali A, He Z, Wollersheim D (2017) Pulmonary nodule classification with deep residual networks. Int J Comput Assist Radiol Surg 12:1799–1808. https://doi.org/10.1007/s11548-017-1605-6
Obulesu O, Kallam S, Dhiman G et al (2021) Adaptive diagnosis of lung cancer by deep learning classification using Wilcoxon gain and generator. 2021:1–13. https://doi.org/10.1155/2021/5912051
Oh S, Im J, Kang S-R et al (2021) PET-Based Deep-Learning Model for Predicting Prognosis of Patients With Non-Small Cell Lung Cancer. IEEE Access 9:138753–138761. https://doi.org/10.1109/access.2021.3115486
Ozdemir O, Russell RL, Berlin AA (2020) A 3D Probabilistic Deep Learning System for Detection and Diagnosis of Lung Cancer Using Low-Dose CT Scans. IEEE Trans Med Imaging 39:1419–1429. https://doi.org/10.1109/tmi.2019.2947595
Pandit BR, Alsadoon A, Prasad PWC et al (2022) Deep learning neural network for lung cancer classification: enhanced optimization function. Multimedia Tools Appl. https://doi.org/10.1007/s11042-022-13566-9
Parascandola M, Xiao L (2019) Tobacco and the lung cancer epidemic in China. Translational Lung Cancer Res 8:S21–S30. https://doi.org/10.21037/tlcr.2019.03.12
Park S, Jin Lee S, Weiss E, Motai Y (2016) Intra- and Inter-Fractional Variation Prediction of Lung Tumors Using Fuzzy Deep Learning. IEEE J Translational Eng Health Med 4:4300112. https://doi.org/10.1109/JTEHM.2016.2516005
Parris BA, O’Farrell HE, Fong KM, Yang IA (2019) Chronic obstructive pulmonary disease (COPD) and lung cancer: common pathways for pathogenesis. J Thoracic Dis\ 11:S2155–S2172. https://doi.org/10.21037/jtd.2019.10.54
Pathak H, Manoj Kumar Pandey, Kaur J (2018) Detection and feature extraction of cancer nodules in lung CT image. J Emerging Technol Innov Res
Quadrelli S, Lyons G, Colt H et al (2015) Clinical Characteristics and Prognosis of Incidentally Detected Lung Cancers. Int J Surg Oncol 2015:1–6. https://doi.org/10.1155/2015/287604
Roointan A, Ahmad Mir T, Ibrahim Wani S et al (2019) Early detection of lung cancer biomarkers through biosensor technology: A review. J Pharm Biomed Anal 164:93–103. https://doi.org/10.1016/j.jpba.2018.10.017
Said Y, Alsheikhy AA, Shawly T, Lahza H (2023) Medical Images Segmentation for Lung Cancer Diagnosis Based on Deep Learning Architectures. Diagnostics 13:546–546. https://doi.org/10.3390/diagnostics13030546
Savitha G, Jidesh P (2020) A holistic deep learning approach for identification and classification of sub-solid lung nodules in computed tomographic scans. Comput Electr Eng 84:106626. https://doi.org/10.1016/j.compeleceng.2020.106626
Schaefer-Prokop C, Prokop M (2002) New imaging techniques in the treatment guidelines for lung cancer. Eur Respir J 19:71S-83S. https://doi.org/10.1183/09031936.02.00277902
Shah AA, Malik HAM, Muhammad A et al (2023) Deep learning ensemble 2D CNN approach towards the detection of lung cancer. Sci Rep 13. https://doi.org/10.1038/s41598-023-29656-z
Shakeel PM, Burhanuddin MA, Desa MI (2019) Lung cancer detection from CT image using improved profuse clustering and deep learning instantaneously trained neural networks. Measurement 145:702–712. https://doi.org/10.1016/j.measurement.2019.05.027
Shakeel PM, Burhanuddin MA, Desa MI (2020) Automatic lung cancer detection from CT image using improved deep neural network and ensemble classifier. Neural Comput Appl. https://doi.org/10.1007/s00521-020-04842-6
Shakeel PM, Burhanuddin MA, Desa MI (2022) Automatic lung cancer detection from CT image using improved deep neural network and ensemble classifier. Neural Comput Appl 7731–7762. https://doi.org/10.1007/s00521-020-04842-6
Sharma R (2022) Mapping of global, regional and national incidence, mortality and mortality-to-incidence ratio of lung cancer in 2020 and 2050. Int J Clin Oncol 27: https://doi.org/10.1007/s10147-021-02108-2
Siddiqui EA, Chaurasia V, Shandilya M (2023) Detection and classification of lung cancer computed tomography images using a novel improved deep belief network with Gabor filters. Chemom Intell Lab Syst 235:104763. https://doi.org/10.1016/j.chemolab.2023.104763
Silvestri MD GA, Tanner MD NT, Kearney P et al (n.d.) Assessment of plasma proteomics biomarker’s ability to distinguish benign from malignant lung nodules: results of the PANOPTIC (Pulmonary Nodule Plasma Proteomic Classifier). Trial Chest 154:491–500. https://doi.org/10.1016/j.chest.2018.02.012
Song Q, Zhao L, Luo X, Dou X (2017) Using Deep Learning for Classification of Lung Nodules on Computed Tomography Images. J Healthc Eng 2017:1–7. https://doi.org/10.1155/2017/8314740
Sori WJ, Feng J, Godana AW et al (2020) DFD-Net: lung cancer detection from denoised CT scan image using deep learning. Front Comput Sci 15: https://doi.org/10.1007/s11704-020-9050-z
Strak M, Janssen N, Beelen R et al (2017) Associations between lifestyle and air pollution exposure: Potential for confounding in large administrative data cohorts. Environ Res 156:364–373. https://doi.org/10.1016/j.envres.2017.03.050
Sui D, Guo M, Ma X et al (2021) Image bio-markers and gene expression data correlation framework for lung cancer radio-genomics analysis based on deep learning. Res Square (Res Square). https://doi.org/10.21203/rs.3.rs-144196/v1
Tan J, Huo Y, Liang Z, Li L (2019) Expert knowledge-infused deep learning for automatic lung nodule detection. J Xray Sci Technol 27:17–35. https://doi.org/10.3233/xst-180426
Tariq Hussain S (n.d.) The journey: from X-rays to PET-MRI. Indian J Nucl Med
Tian Q, Wu Y, Ren X, Razmjooy N (2021) A New optimized sequential method for lung tumor diagnosis based on deep learning and converged search and rescue algorithm. Biomed Signal Process Control 68:102761. https://doi.org/10.1016/j.bspc.2021.102761
Tran GS, Nghiem TP, Nguyen VT et al (2019) Improving Accuracy of Lung Nodule Classification Using Deep Learning with Focal Loss. J Healthc Eng 2019:1–9. https://doi.org/10.1155/2019/5156416
Traverso A, Lopez Torres E, Fantacci ME, Cerello P (2017) Computer-aided detection systems to improve lung cancer early diagnosis: state-of-the-art and challenges. J Phys: Conf Ser 841:012013. https://doi.org/10.1088/1742-6596/841/1/012013
Wang X, Chen H, Gan C et al (2020) Weakly Supervised Deep Learning for Whole Slide Lung Cancer Image Analysis. IEEE Trans Cybern 50:3950–3962. https://doi.org/10.1109/tcyb.2019.2935141
Wang Y-W, Chen C-J, Huang H-C et al (2021) Dual energy CT image prediction on primary tumor of lung cancer for nodal metastasis using deep learning. Comput Med Imaging Graph 91:101935–101935. https://doi.org/10.1016/j.compmedimag.2021.101935
Wang W, Liu F, Zhi X et al (2020a) An Integrated deep learning algorithm for detecting lung nodules with low-dose CT and its application in 6G-enabled internet of medical things. IEEE Internet Things J 1–1. https://doi.org/10.1109/jiot.2020.3023436
Wani NA, Kumar R, Bedi J (2023) DeepXplainer: an interpretable deep learning based approach for lung cancer detection using explainable artificial intelligence. Comput Methods Programs Biomed 243:107879. https://doi.org/10.1016/j.cmpb.2023.107879
Wankhade S, Vigneshwari S (2023) Lung cell cancer identification mechanism using deep learning approach. Soft Computing. https://doi.org/10.1007/s00500-023-08661-4
Weng S, Xu X, Li J, Wong STC (2017) Combining deep learning and coherent anti-Stokes Raman scattering imaging for automated differential diagnosis of lung cancer. J Biomed Opt 22:1. https://doi.org/10.1117/1.jbo.22.10.106017
World Health Organization (2022) Cancer. In: World Health Organization. https://www.who.int/news-room/fact-sheets/detail/cancer . Accessed 22 Jan 2024
Wu P, Sun X, Zhao Z et al (2020) Classification of lung nodules based on deep residual networks and migration learning. 2020:1–10. https://doi.org/10.1155/2020/8975078
Wu J, Qian T (2019) A survey of pulmonary nodule detection, segmentation and classification in computed tomography with deep learning techniques. J Med Artif Intell 2:8–8. https://doi.org/10.21037/jmai.2019.04.01
Xie Y, Xia Y, Zhang J et al (2019) Knowledge-based Collaborative Deep Learning for Benign-Malignant Lung Nodule Classification on Chest CT. IEEE Trans Med Imaging 38:991–1004. https://doi.org/10.1109/tmi.2018.2876510
Xu Y, Hosny A, Zeleznik R et al (2019) Deep Learning Predicts Lung Cancer Treatment Response from Serial Medical Imaging. Clin Cancer Res 25:3266–3275. https://doi.org/10.1158/1078-0432.ccr-18-2495
Yu H, Zhou Z, Wang Q (2020) Deep Learning Assisted Predict of Lung Cancer on Computed Tomography Images Using the Adaptive Hierarchical Heuristic Mathematical Model. IEEE Access 8:86400–86410. https://doi.org/10.1109/access.2020.2992645
Yuan R, Vos PM, Cooperberg PL (2006) Computer-Aided Detection in Screening CT for Pulmonary Nodules. Am J Roentgenol 186:1280–1287. https://doi.org/10.2214/ajr.04.1969
Yu-Jen Chen Y-J, Hua K-L, Hsu C-H et al (2015) Computer-aided classification of lung nodules on computed tomography images via deep learning technique. OncoTargets Ther 2015. https://doi.org/10.2147/ott.s80733
Zhang Q, Kong X (2020) Design of Automatic Lung Nodule Detection System Based on Multi-Scene Deep Learning Framework. IEEE Access 8:90380–90389. https://doi.org/10.1109/access.2020.2993872
Zhang G, Yang Z, Gong L et al (2019) Classification of benign and malignant lung nodules from CT images based on hybrid features. Phys Med Biol 64:125011. https://doi.org/10.1088/1361-6560/ab2544
Zhao X, Wang X, Xia W et al (2020) A cross-modal 3D deep learning for accurate lymph node metastasis prediction in clinical stage T1 lung adenocarcinoma. Lung Cancer (amsterdam, Netherlands) 145:10–17. https://doi.org/10.1016/j.lungcan.2020.04.014
Download references
Author information
Authors and affiliations.
Department of Computer Science, TIMES Institute Multan, Multan, 60000, Pakistan
Rabia Javed & Tahir Abbas
Department of Software Engineering, Faculty of Computer Science, Lahore Garrison University, Lahore, 54000, Pakistan
Ali Haider Khan
Faculty of Resilience, Rabdan Academy, Abu Dhabi, United Arab Emirates
Department of Information Systems and Technology, College of Computer Science and Engineering, University of Jeddah, Jeddah, Saudi Arabia
Amal Bukhari & Riad Alharbey
You can also search for this author in PubMed Google Scholar
Contributions
Rabia and Ali Haider have written a major part of the paper under the supervision of Tahir and Ali Daud. Tahir and Ali Daud have helped design and improve the methodology and wrote the paper initial draft with Rabia and Ali Haider. Riad and Amal have helped in improving the paper sections, such as, review methodology, datasets, performance evaluation and challenges and future directions. Ali, Amal and Riad have improved the technical writing of paper. All authors are involved in revising the manuscript critically and have approved the final version of the manuscript.
Corresponding author
Correspondence to Ali Daud .
Ethics declarations
Competing interests.
The authors declare no competing interests.
Additional information
Publisher's note.
Springer Nature remains neutral with regard to jurisdictional claims in published maps and institutional affiliations.
Rights and permissions
Open Access This article is licensed under a Creative Commons Attribution 4.0 International License, which permits use, sharing, adaptation, distribution and reproduction in any medium or format, as long as you give appropriate credit to the original author(s) and the source, provide a link to the Creative Commons licence, and indicate if changes were made. The images or other third party material in this article are included in the article's Creative Commons licence, unless indicated otherwise in a credit line to the material. If material is not included in the article's Creative Commons licence and your intended use is not permitted by statutory regulation or exceeds the permitted use, you will need to obtain permission directly from the copyright holder. To view a copy of this licence, visit http://creativecommons.org/licenses/by/4.0/ .
Reprints and permissions
About this article
Javed, R., Abbas, T., Khan, A.H. et al. Deep learning for lungs cancer detection: a review. Artif Intell Rev 57 , 197 (2024). https://doi.org/10.1007/s10462-024-10807-1
Download citation
Accepted : 16 May 2024
Published : 08 July 2024
DOI : https://doi.org/10.1007/s10462-024-10807-1
Share this article
Anyone you share the following link with will be able to read this content:
Sorry, a shareable link is not currently available for this article.
Provided by the Springer Nature SharedIt content-sharing initiative
- Lungs Cancer
- Deep learning
- Classification
- Segmentation
- Find a journal
- Publish with us
- Track your research
Public finance and budgets
Countries across the OECD are facing long-term fiscal pressures in areas such as health, ageing, climate change, and defence. At the same time, governments must grapple with mounting debt levels, rising interest rates and high levels of uncertainty. In this increasingly constrained fiscal environment, reconciling new and emerging spending pressures with already stretched public finances requires high-quality budget institutions and processes.

Select a language
Key messages, oecd countries are facing long-term fiscal pressures..
The long-term fiscal pressures associated with climate change and reducing greenhouse gas emissions; ageing populations and shrinking labour supply; and rising health care and social care costs continued to mount. Interest expenditures are now increasing significantly. The current geopolitical tensions are adding further new spending pressures, including in the defence area, as well as greater economic uncertainty.
Reconciling these pressures with already stretched public finances requires high quality budget institutions and strengthened public understanding
Budgets are about more than money. They are a statement of a nation’s priorities. Engagement and oversight of the budget process by Independent Fiscal Institutions, parliaments and the public is fundamental to democratic governance and trust in government. Empowering the public to understand fiscal challenges is essential for generating the will to solve them
Governments must have credible public financial management frameworks to build trust in budgetary governance and maintain enough fiscal space to be able to finance crisis responses when needed.
Governments must have credible public financial management frameworks to build trust in budgetary governance and maintain enough fiscal space to finance crisis responses when needed.
Each of the crises of recent years has shown the importance of preserving the resilience of public finances; countries need to be able to finance large and unexpected expenditures, such as in the aftermath of major natural disasters, to support a distressed sector or to address the consequences of a major pandemic. However, debt levels in OECD countries have risen significantly in recent years.
General government expenditures amounted to 46.3% of GDP on average across OECD countries in 2021
Between 2019 and 2021 general government expenditures as a percentage of GDP increased by 5.4 percentage points, from 40.9% in 2019. This increase is largely explained by the COVID-19 pandemic, which led to significant economic disruption. This prompted large-scale fiscal stimuluses, including increased spending on healthcare, social welfare programmes, and support for businesses and individuals affected by the pandemic, while at the same time GDP was falling.
General Fiscal Balance
The fiscal balance is the difference between a government’s revenues and its expenditures. It signals if public accounts are balanced or if there are surpluses or deficits. Recurrent deficits over time imply the accumulation of public debt and may send worrying signals to consumers and investors about the sustainability of public accounts which, in turn, may deter consumption or investment decisions. Nonetheless, if debt is kept at a sustainable level, deficits can help to finance necessary public investment, or in exceptional circumstances, such as unexpected external shocks (e.g. pandemics, wars or natural disasters), can contribute to maintaining living conditions and preserving social stability.
Related data
Related publications.

Related policy issues
- Fiscal Frameworks Fiscal frameworks outline the government's fiscal intentions and explain how these will be implemented concretely. Well-designed fiscal frameworks provide clarity and stability in government fiscal operations, ensuring that spending on policy priorities of governments, like healthcare, education, and climate adaptation, are funded and sustainable. Additionally, they build resilience by helping governments prepare effectively for economic challenges. Learn more
- Fiscal federalism network The OECD Network on Fiscal Relations across Levels of Government, also known as the “Fiscal Network”, provides a platform for countries to engage on intergovernmental fiscal relations and fiscal decentralisation policy issues. Its core mission is to improve the efficiency, equity and stability of fiscal systems through cross-country policy analysis and international comparisons. The Network facilitates best practice sharing through high-level meetings and maintaining a comprehensive decentralisation database, informing policymaking and reforms. Through collaborative efforts like workshops and the Fiscal Federalism publication series, the Network enables policymakers to access and contribute to research and insights on managing financial relationships across government levels. Supported by a multidisciplinary OECD team, the Network emphasises concrete outcomes, offering members a structured environment to learn, share and apply successful policy strategies. Learn more
- Gender budgeting Gender budgeting is a public governance tool that governments can use to assess how budget decisions impact gender equality. When implemented effectively, gender budgeting helps expose how gender inequalities may have inadvertently become embedded in public policies and the allocation of resources and promotes budget measures that will be effective at closing gender gaps. Learn more
- Green budgeting Green budgeting uses the tools of budgetary policy making to provide policy makers with a clearer understanding of the environmental and climate impacts of budgeting choices, while bringing evidence together in a systematic and co-ordinated manner for more informed decision making to fulfil national and international commitments. Learn more
- Health budgeting Without a major policy shift, health spending is projected to outstrip both expected growth in the overall economy and in government revenues across OECD countries. Competing priorities for government spending are also squeezing health budgets. Urgent action is therefore needed to finance more resilient health systems while ensuring the fiscal sustainability of health systems. Learn more
- Parliamentary budget offices and independent fiscal institutions Our work with parliaments and independent fiscal institutions (IFIs) supports fiscal transparency and accountability. At a time when the sustainability of public finances is under close scrutiny, these oversight institutions play a crucial role in raising the quality of the debate on fiscal policy and ensuring that public budgets are managed effectively. Learn more
- Performance budgeting In an environment of budget constraints and high citizen expectations it is necessary to demonstrate that public expenditure is providing value for money and delivering on performance. The availability of good-quality performance information not only assists policymakers in making more informed budgetary decisions but also enables the broader public to hold the government accountable for delivering the outcomes promised to citizens. Learn more
- Public accounts Good management of public money is vital for good governance, ensuring essential services like healthcare and education run smoothly. Public accounts track government income and spending, they show how money is managed and if the government can fund these crucial services. Learn more
- Public debt management Prudent public debt management is critical for well-functioning national financial systems and helps to reinforce sound fiscal and monetary policies. Public debt portfolios, both in terms of their size and composition, have the potential to generate substantial risk to countries’ balance sheets and overall financial stability. The OECD promotes good practices in public debt and risk management and provides recommendations to assist policy makers in their efforts to adopt and implement prudent debt management policies. Learn more
- Spending Reviews Spending reviews are tools for systematically analysing the government’s existing expenditure. The OECD has found that spending reviews have proved to be an important tool for governments, not only to control total expenditure by making space for more resources, but also to align spending allocations with government priorities and to improve the effectiveness of policies and programmes. Learn more

IMAGES
VIDEO
COMMENTS
Examples of literature reviews. Step 1 - Search for relevant literature. Step 2 - Evaluate and select sources. Step 3 - Identify themes, debates, and gaps. Step 4 - Outline your literature review's structure. Step 5 - Write your literature review.
Literature reviews are in great demand in most scientific fields. ... Feedback is vital to writing a good review, and should be sought from a variety of colleagues, so as to obtain a diversity of views on the draft. ... Maier HR (2013) What constitutes a good literature review and why does its quality matter? Environ Model Softw 43: 3-4 doi ...
A literature review is a document or section of a document that collects key sources on a topic and discusses those sources in conversation with each other (also called synthesis ). The lit review is an important genre in many disciplines, not just literature (i.e., the study of works of literature such as novels and plays).
1. Outline and identify the purpose of a literature review. As a first step on how to write a literature review, you must know what the research question or topic is and what shape you want your literature review to take. Ensure you understand the research topic inside out, or else seek clarifications.
The best proposals are timely and clearly explain why readers should pay attention to the proposed topic. It is not enough for a review to be a summary of the latest growth in the literature: the ...
Mapping the gap. The purpose of the literature review section of a manuscript is not to report what is known about your topic. The purpose is to identify what remains unknown—what academic writing scholar Janet Giltrow has called the 'knowledge deficit'—thus establishing the need for your research study [].In an earlier Writer's Craft instalment, the Problem-Gap-Hook heuristic was ...
A literature review is a survey of scholarly sources on a specific topic. It provides an overview of current knowledge, allowing you to identify relevant theories, methods, and gaps in the existing research. There are five key steps to writing a literature review: Search for relevant literature. Evaluate sources. Identify themes, debates and gaps.
classification, and comparison of prior research studies, reviews of literature, and theoretical articles • To emphasize the credibility of the writer in their field • To provide a solid background for a research paper's investigation A GOOD LITERATURE REVIEW SHOULD… • Be organized around a thesis statement or research question(s)
Quality research is about building onto the existing work of others, "standing on the shoulders of giants", as Newton put it.The literature review chapter of your dissertation, thesis or research project is where you synthesise this prior work and lay the theoretical foundation for your own research.. Long story short, this chapter is a pretty big deal, which is why you want to make sure ...
3. Evaluate and select literature. 4. Analyze the literature. 5. Plan the structure of your literature review. 6. Write your literature review. Other resources to help you write a successful literature review.
To understand better how to achieve a good quality literature review, it is helpful to look at the specific processes of the archetypes of literature reviews introduced in Section 2.5; they can also be found in Fig. 3.1, which presents a further classification of the protocol-driven approaches to literature reviews.Even though different archetypes serve different purposes, there are similar ...
The word "literature review" can refer to two related things that are part of the broader literature review process. The first is the task of reviewing the literature - i.e. sourcing and reading through the existing research relating to your research topic. The second is the actual chapter that you write up in your dissertation, thesis or ...
In addition, even when the methodology of the reviews is valid, there are often issues with what constitutes a good contribution. Of course, there already exist some guidelines for conducting literature reviews that suggest different types of reviews, ... (2018) suggest that a quality literature review must have both depth and rigor, that is, ...
2. MOTIVATE YOUR RESEARCH in addition to providing useful information about your topic, your literature review must tell a story about how your project relates to existing literature. popular literature review narratives include: ¡ plugging a gap / filling a hole within an incomplete literature ¡ building a bridge between two "siloed" literatures, putting literatures "in conversation"
Important aspects of a systematic literature review (SLR) include a structured method for conducting the study and significant transparency of the approaches used for summarizing the literature (Hiebl, 2023).The inspection of existing scientific literature is a valuable tool for (a) developing best practices and (b) resolving issues or controversies over a single study (Gupta et al., 2018).
Your first draft is a big accomplishment, especially if the literature review is very long and part of a much larger study or paper such as a dissertation or similar work. 7. Revise and Edit. While your first draft includes all the studies you wanted to talk about, your literature review isn't done yet.
A good literature review is NOT simply a list describing or summarizing several articles; a literature review is discursive prose which proceeds to a conclusion by reason or argument. A good literature review shows signs of synthesis and understanding of the topic. There should be strong evidence of analytical thinking shown through the ...
A good literature review evaluates a wide variety of sources (academic articles, scholarly books, government/NGO reports). It also evaluates literature reviews that study similar topics. This page offers you a list of resources and tips on how to evaluate the sources that you may use to write your review.
In this article, we covered 6 pointers to help you find and evaluate high-quality resources for your literature review. To recap: Develop and follow a clear literature search strategy. Understand and use different types of literature for the right purpose. Carefully evaluate the quality of your potential sources.
Step 8: The literature review in your thesis. This last step reveals what criteria are used to evaluate the literature review in your own thesis: synthesis, critical appraisal, and application to the research question. I also explain what your literature should not look like and why a good literature review helps you to get papers published.
15 Literature Review Examples. Literature reviews are a necessary step in a research process and often required when writing your research proposal. They involve gathering, analyzing, and evaluating existing knowledge about a topic in order to find gaps in the literature where future studies will be needed. Ideally, once you have completed your ...
Steps for Conducting a Lit Review; Finding "The Literature" Organizing/Writing; APA Style This link opens in a new window; Chicago: Notes Bibliography This link opens in a new window; MLA Style This link opens in a new window; Sample Literature Reviews. Sample Lit Reviews from Communication Arts; Have an exemplary literature review? Get Help!
Ten Simple Rules for Writing a Literature Review. Rule 1: Define a Topic and Audience. Rule 2: Search and Re-search the Literature. Rule 3: Take Notes While Reading. Rule 4: Choose the Type of Review You Wish to Write. Rule 5: Keep the Review Focused, but Make It of Broad Interest. Rule 6: Be Critical and Consistent.
Keywords: meta-research, literature review methods, information retrieval, regulatory science, collaboration. Citation: Stevens ER and Laynor G (2024) Enhancing the quality and efficiency of regulatory science literature reviews through innovation and collaboration with library and information science experts. Front.
There is a paucity of good quality data on fracture incidence in children under the age of two. This systematic review of the literature found only 12 studies over the last 78 years that met the eligibility criteria, however, due to data inhomogeneity a meta-analysis could not be calculated.
Although lung cancer has been recognized to be the deadliest type of cancer, a good prognosis and efficient treatment depend on early detection. Medical practitioners' burden is reduced by deep learning techniques, especially Deep Convolutional Neural Networks (DCNN), which are essential in automating the diagnosis and classification of diseases. In this study, we use a variety of medical ...
Public finance is the economic field focusing on the financial activities of government entities at various levels. Our work examines government expenditures, including public services, infrastructure, social welfare, defence, education, healthcare, and more. These are outlined in the national budget, reflecting financial commitments to meet obligations and provide essential services. Our ...