Kolb’s Learning Styles and Experiential Learning Cycle
Saul Mcleod, PhD
Editor-in-Chief for Simply Psychology
BSc (Hons) Psychology, MRes, PhD, University of Manchester
Saul Mcleod, PhD., is a qualified psychology teacher with over 18 years of experience in further and higher education. He has been published in peer-reviewed journals, including the Journal of Clinical Psychology.
Learn about our Editorial Process
Olivia Guy-Evans, MSc
Associate Editor for Simply Psychology
BSc (Hons) Psychology, MSc Psychology of Education
Olivia Guy-Evans is a writer and associate editor for Simply Psychology. She has previously worked in healthcare and educational sectors.
On This Page:
David Kolb published his learning styles model in 1984, from which he developed his learning style inventory.
Kolb’s experiential learning theory works on two levels: a four-stage learning cycle and four separate learning styles. Much of Kolb’s theory concerns the learner’s internal cognitive processes.
Kolb states that learning involves the acquisition of abstract concepts that can be applied flexibly in a range of situations. In Kolb’s theory, the impetus for the development of new concepts is provided by new experiences.
“Learning is the process whereby knowledge is created through the transformation of experience” (Kolb, 1984, p. 38).

The Experiential Learning Cycle
Kolb’s experiential learning style theory is typically represented by a four-stage learning cycle in which the learner “touches all the bases”:
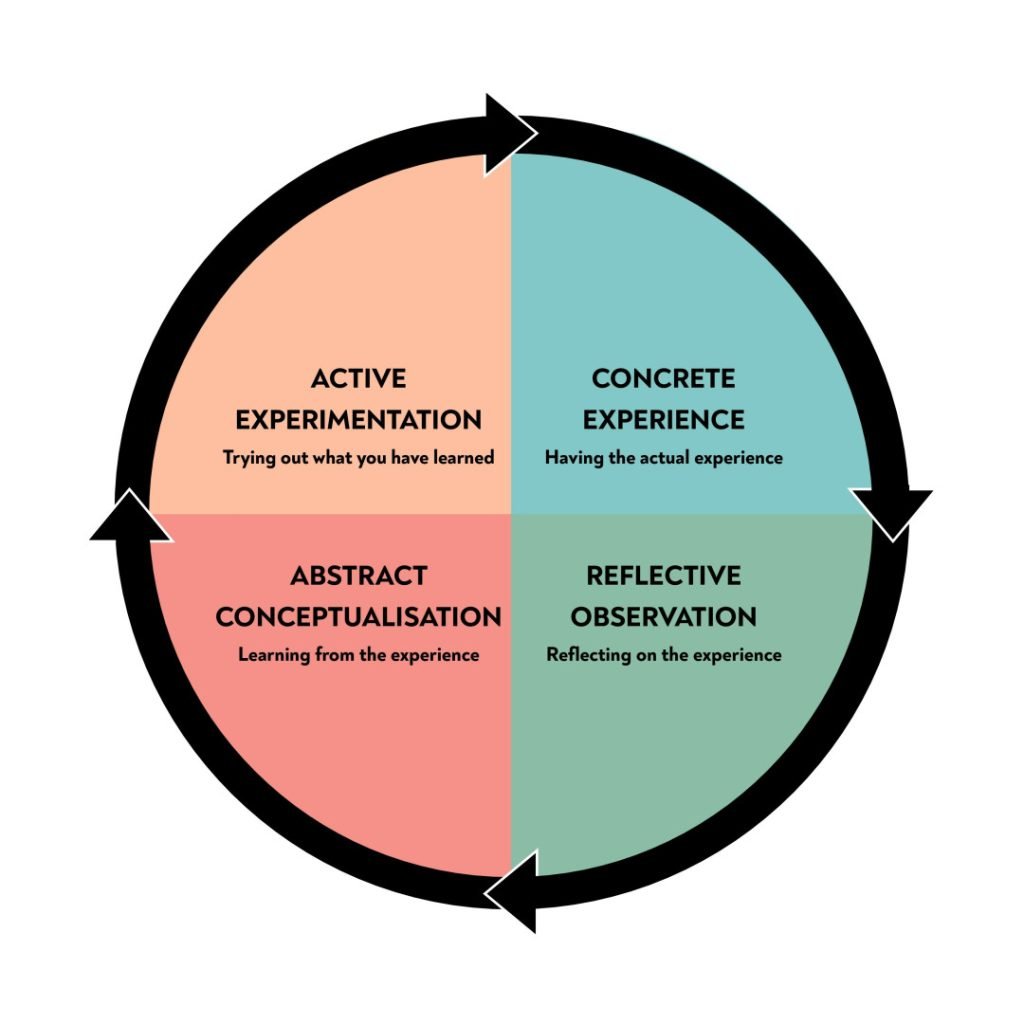
The terms “Reflective Cycle” and “Experiential Learning Cycle” are often used interchangeably when referring to this four-stage learning process. The main idea behind both terms is that effective learning occurs through a continuous cycle of experience, reflection, conceptualization, and experimentation.
- Concrete Experience – the learner encounters a concrete experience. This might be a new experience or situation, or a reinterpretation of existing experience in the light of new concepts.
- Reflective Observation of the New Experience – the learner reflects on the new experience in the light of their existing knowledge. Of particular importance are any inconsistencies between experience and understanding.
- Abstract Conceptualization – reflection gives rise to a new idea, or a modification of an existing abstract concept (the person has learned from their experience).
- Active Experimentation – the newly created or modified concepts give rise to experimentation. The learner applies their idea(s) to the world around them to see what happens.
Effective learning is seen when a person progresses through a cycle of four stages: of (1) having a concrete experience followed by (2) observation of and reflection on that experience which leads to (3) the formation of abstract concepts (analysis) and generalizations (conclusions) which are then (4) used to test a hypothesis in future situations, resulting in new experiences.
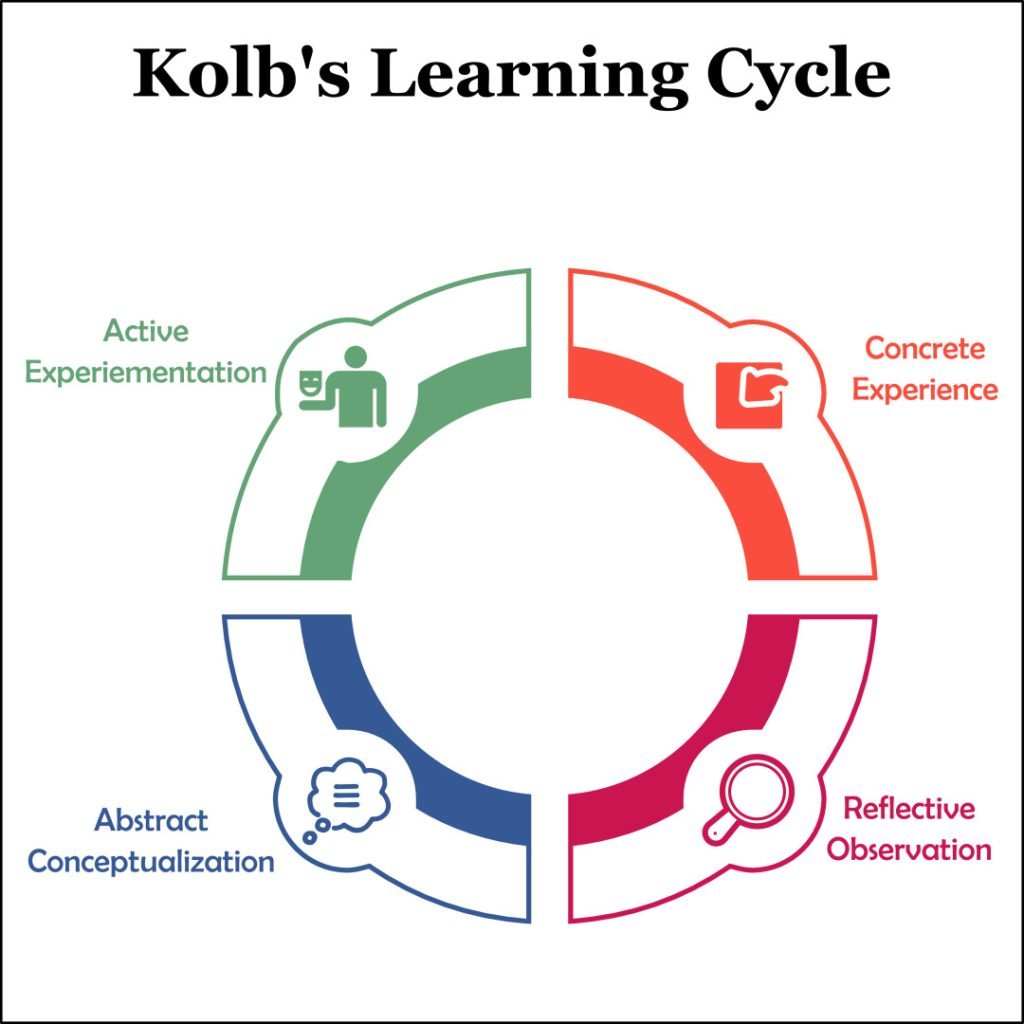
Kolb (1984) views learning as an integrated process, with each stage mutually supporting and feeding into the next. It is possible to enter the cycle at any stage and follow it through its logical sequence.
However, effective learning only occurs when a learner can execute all four stages of the model. Therefore, no one stage of the cycle is effective as a learning procedure on its own.
The process of going through the cycle results in the formation of increasingly complex and abstract ‘mental models’ of whatever the learner is learning about.
Learning Styles
Kolb’s learning theory (1984) sets out four distinct learning styles, which are based on a four-stage learning cycle (see above). Kolb explains that different people naturally prefer a certain single different learning style.
Various factors influence a person’s preferred style. For example, social environment, educational experiences, or the basic cognitive structure of the individual.
Whatever influences the choice of style, the learning style preference itself is actually the product of two pairs of variables, or two separate “choices” that we make, which Kolb presented as lines of an axis, each with “conflicting” modes at either end.
A typical presentation of Kolb’s two continuums is that the east-west axis is called the Processing Continuum (how we approach a task), and the north-south axis is called the Perception Continuum (our emotional response, or how we think or feel about it).
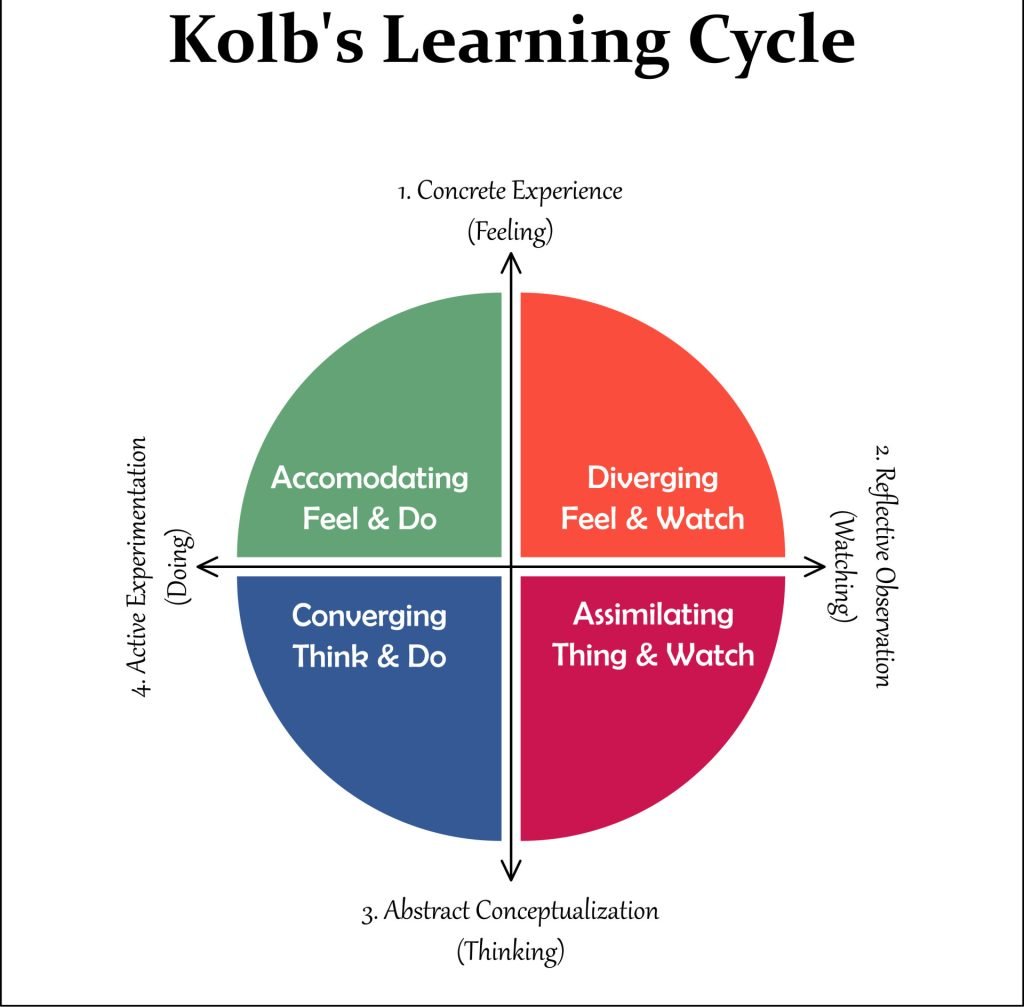
Kolb believed that we cannot perform both variables on a single axis simultaneously (e.g., think and feel). Our learning style is a product of these two choice decisions.
It’s often easier to see the construction of Kolb’s learning styles in terms of a two-by-two matrix. Each learning style represents a combination of two preferred styles.
The matrix also highlights Kolb’s terminology for the four learning styles; diverging, assimilating, and converging, accommodating:
Active Experimentation (Doing) | Reflective Observation (Watching) | |
---|---|---|
Concrete Experience (Feeling) | Accommodating (CE/AE) | Diverging (CE/RO) |
Abstract Conceptualization (Thinking) | Converging (AC/AE) | Assimilating (AC/RO) |
Knowing a person’s (and your own) learning style enables learning to be orientated according to the preferred method.
That said, everyone responds to and needs the stimulus of all types of learning styles to one extent or another – it’s a matter of using emphasis that fits best with the given situation and a person’s learning style preferences.
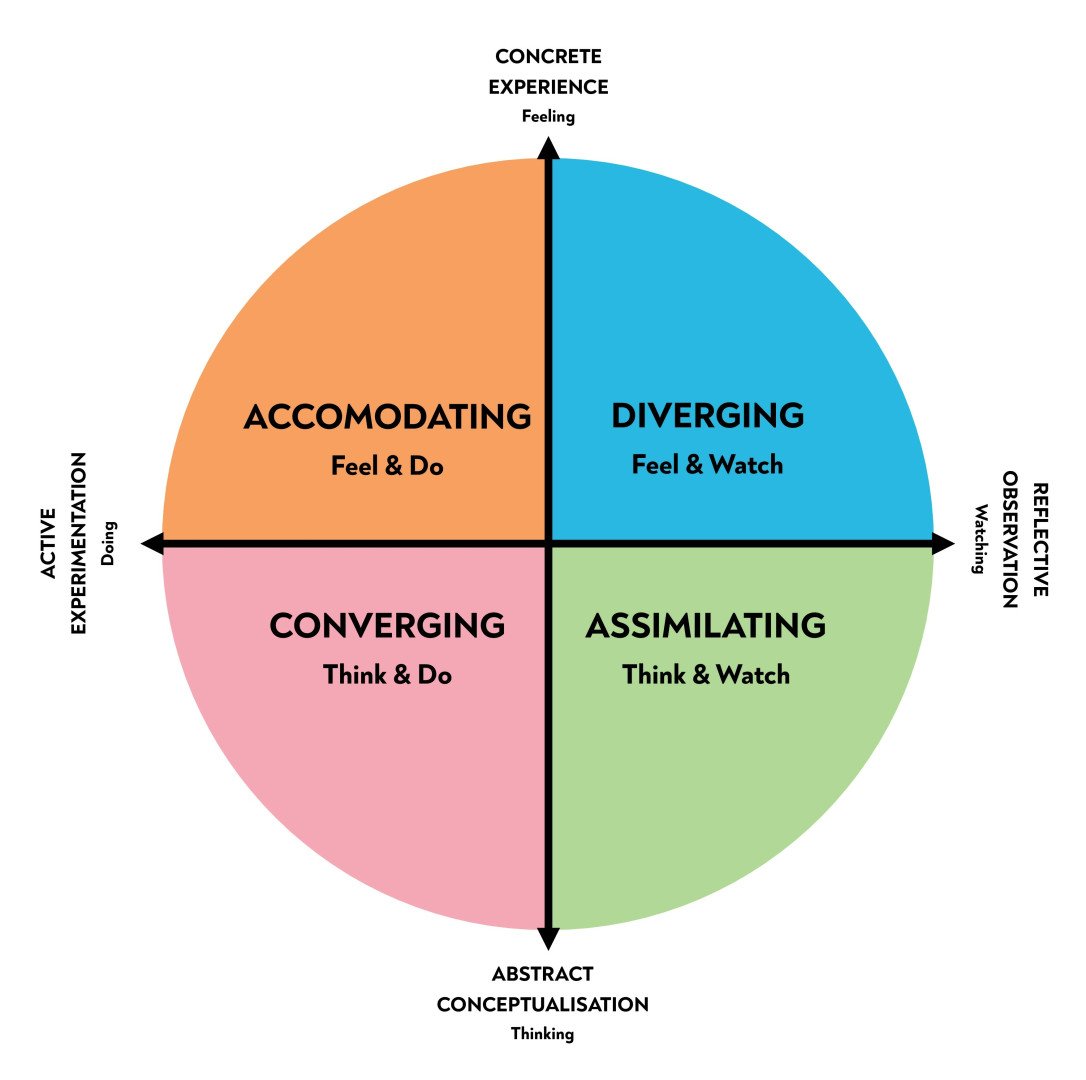
Here are brief descriptions of the four Kolb learning styles:
Diverging (feeling and watching – CE/RO)
These people are able to look at things from different perspectives. They are sensitive. They prefer to watch rather than do, tending to gather information and use imagination to solve problems. They are best at viewing concrete situations from several different viewpoints.
Kolb called this style “diverging” because these people perform better in situations that require ideas-generation, for example, brainstorming. People with a diverging learning style have broad cultural interests and like to gather information.
They are interested in people, tend to be imaginative and emotional, and tend to be strong in the arts. People with the diverging style prefer to work in groups, to listen with an open mind and to receive personal feedback.
Assimilating (watching and thinking – AC/RO)
The assimilating learning preference involves a concise, logical approach. Ideas and concepts are more important than people.
These people require good, clear explanations rather than a practical opportunity. They excel at understanding wide-ranging information and organizing it in a clear, logical format.
People with an assimilating learning style are less focused on people and more interested in ideas and abstract concepts. People with this style are more attracted to logically sound theories than approaches based on practical value.
This learning style is important for effectiveness in information and science careers. In formal learning situations, people with this style prefer readings, lectures, exploring analytical models, and having time to think things through.
Converging (doing and thinking – AC/AE)
People with a converging learning style can solve problems and will use their learning to find solutions to practical issues. They prefer technical tasks, and are less concerned with people and interpersonal aspects.
People with a converging learning style are best at finding practical uses for ideas and theories. They can solve problems and make decisions by finding solutions to questions and problems.
People with a converging learning style are more attracted to technical tasks and problems than social or interpersonal issues. A converging learning style enables specialist and technology abilities.
People with a converging style like to experiment with new ideas, to simulate, and to work with practical applications.
Accommodating (doing and feeling – CE/AE)
The Accommodating learning style is “hands-on,” and relies on intuition rather than logic. These people use other people’s analysis, and prefer to take a practical, experiential approach. They are attracted to new challenges and experiences, and to carrying out plans.
They commonly act on “gut” instinct rather than logical analysis. People with an accommodating learning style will tend to rely on others for information than carry out their own analysis. This learning style is prevalent within the general population.
Educational Implications
Both Kolb’s (1984) learning stages and the cycle could be used by teachers to critically evaluate the learning provision typically available to students, and to develop more appropriate learning opportunities.
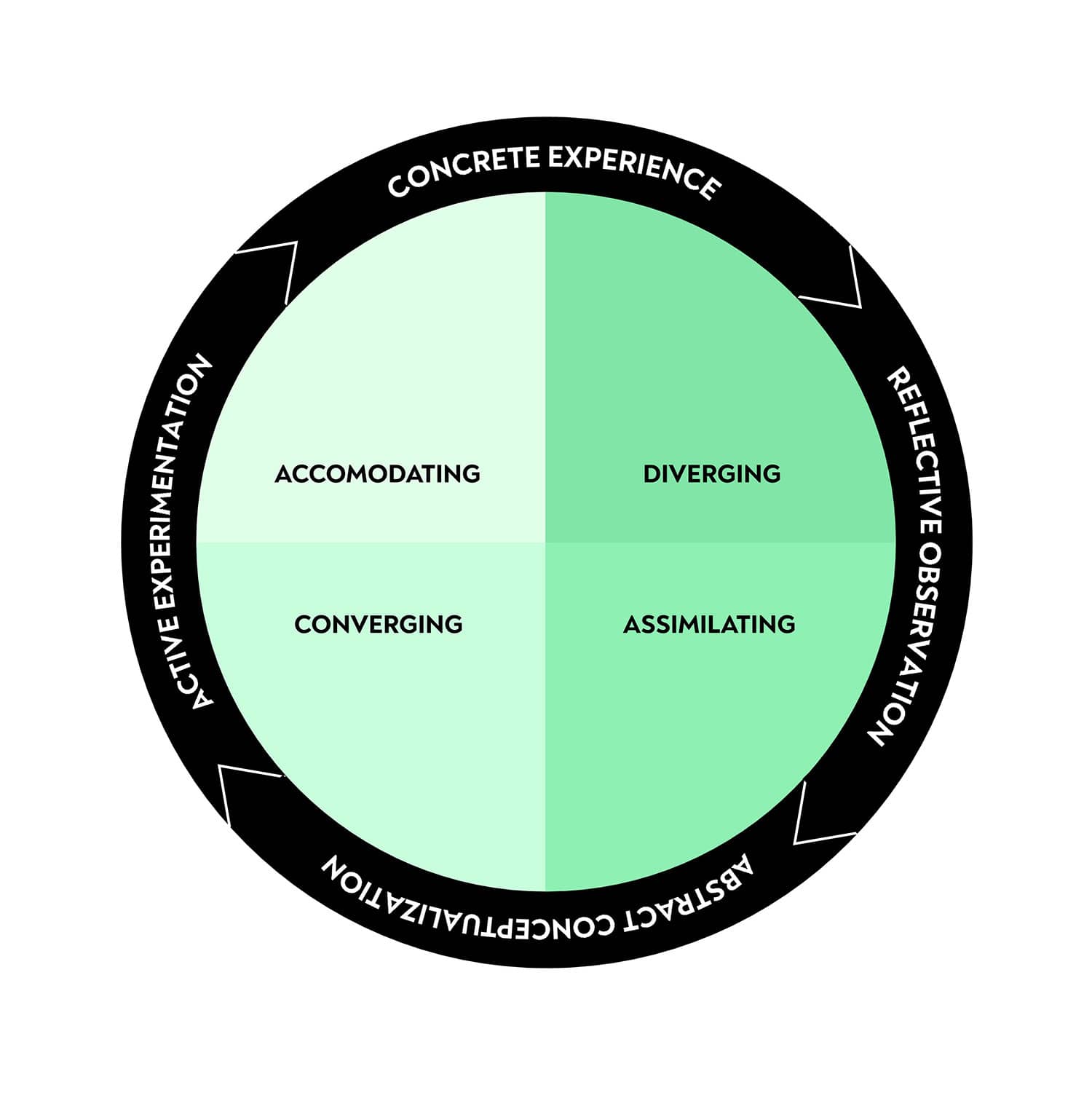
Educators should ensure that activities are designed and carried out in ways that offer each learner the chance to engage in the manner that suits them best.
Also, individuals can be helped to learn more effectively by the identification of their lesser preferred learning styles and the strengthening of these through the application of the experiential learning cycle.
Ideally, activities and material should be developed in ways that draw on abilities from each stage of the experiential learning cycle and take the students through the whole process in sequence.
Kolb, D. A. (1976). The Learning Style Inventory: Technical Manual . Boston, MA: McBer.
Kolb, D.A. (1981). Learning styles and disciplinary differences, in: A.W. Chickering (Ed.) The Modern American College (pp. 232–255). San Francisco, LA: Jossey-Bass.
Kolb, D. A. (1984). Experiential learning: Experience as the source of learning and development (Vol. 1). Englewood Cliffs, NJ: Prentice-Hall.
Kolb, D. A., & Fry, R. (1975). Toward an applied theory of experiential learning. In C. Cooper (Ed.), Studies of group process (pp. 33–57). New York: Wiley.
Kolb, D. A., Rubin, I. M., & McIntyre, J. M. (1984). Organizational psychology: readings on human behavior in organizations . Englewood Cliffs, NJ: Prentice-Hall.
Further Reading
- How to Write a Psychology Essay
- David Kolb’s Website
- Pashler, H., McDaniel, M., Rohrer, D., & Bjork, R. (2008). Learning styles: Concepts and evidence. Psychological science in the public interest, 9(3) , 105-119.

- Spring 2024
- Spring 2023
- Spring 2022
- Spring 2021
- Spring 2020
- Summer 2019
- Spring 2019
- Summer 2018
- Spring 2018
- Spring 2017
- Spring 2016
- Staff and Contact
- Julia Robinson Math Festival
- Teachers’ Circle
- The Three Stages of the Problem-Solving Cycle
Essentially every problem-solving heuristic in mathematics goes back to George Polya’s How to Solve It ; my approach is no exception. However, this cyclic description might help to keep the process cognitively present.
A few months ago, I produced a video describing this the three stages of the problem-solving cycle: Understand, Strategize, and Implement. That is, we must first understand the problem, then we think of strategies that might help solve the problem, and finally we implement those strategies and see where they lead us. During two decades of observing myself and others in the teaching and learning process, I’ve noticed that the most neglected phase is often the first one—understanding the problem.

The Three Stages Explained
- What am I looking for?
- What is the unknown?
- Do I understand every word and concept in the problem?
- Am I familiar with the units in which measurements are given?
- Is there information that seems missing?
- Is there information that seems superfluous?
- Is the source of information bona fide? (Think about those instances when a friend gives you a puzzle to solve and you suspect there’s something wrong with the way the puzzle is posed.)
- Logical reasoning
- Pattern recognition
- Working backwards
- Adopting a different point of view
- Considering extreme cases
- Solving a simpler analogous problem
- Organizing data
- Making a visual representation
- Accounting for all possibilities
- Intelligent guessing and testing
I have produced videos explaining each one of these strategies individually using problems we have solved at the Chapel Hill Math Circle.
- Implementing : We now implement our strategy or set of strategies. As we progress, we check our reasoning and computations (if any). Many novice problem-solvers make the mistake of “doing something” before understanding (or at least thinking they understand) the problem. For instance, if you ask them “What are you looking for?”, they might not be able to answer. Certainly, it is possible to have an incorrect understanding of the problem, but that is different from not even realizing that we have to understand the problem before we attempt to solve it!
As we implement our strategies, we might not be able to solve the problem, but we might refine our understanding of the problem. As we refine our understanding of the problem, we can refine our strategy. As we refine our strategy and implement a new approach, we get closer to solving the problem, and so on. Of course, even after several iterations of this cycle spanning across hours, days, or even years, one may still not be able to solve a particular problem. That’s part of the enchanting beauty of mathematics.
I invite you to observe your own thinking—and that of your students—as you move along the problem-solving cycle!
[1] Problem-Solving Strategies in Mathematics , Posamentier and Krulik, 2015.
About the author: You may contact Hector Rosario at [email protected].
Share this:
1 response to the three stages of the problem-solving cycle.
Pingback: When (Not) to Give a Hint | Chapel Hill Math Circle
Leave a comment Cancel reply
- Search for:
Recent Posts
- When (Not) to Give a Hint
- Making “Maths” Make Sense
- Problem-Solving Pedagogy
Recent Comments
on |
- September 2016
- Uncategorized
- Entries feed
- Comments feed
- WordPress.com

- Already have a WordPress.com account? Log in now.
- Subscribe Subscribed
- Copy shortlink
- Report this content
- View post in Reader
- Manage subscriptions
- Collapse this bar
- Bipolar Disorder
- Therapy Center
- When To See a Therapist
- Types of Therapy
- Best Online Therapy
- Best Couples Therapy
- Best Family Therapy
- Managing Stress
- Sleep and Dreaming
- Understanding Emotions
- Self-Improvement
- Healthy Relationships
- Student Resources
- Personality Types
- Guided Meditations
- Verywell Mind Insights
- 2024 Verywell Mind 25
- Mental Health in the Classroom
- Editorial Process
- Meet Our Review Board
- Crisis Support
Problem-Solving Strategies and Obstacles
Kendra Cherry, MS, is a psychosocial rehabilitation specialist, psychology educator, and author of the "Everything Psychology Book."
:max_bytes(150000):strip_icc():format(webp)/IMG_9791-89504ab694d54b66bbd72cb84ffb860e.jpg)
Sean is a fact-checker and researcher with experience in sociology, field research, and data analytics.
:max_bytes(150000):strip_icc():format(webp)/Sean-Blackburn-1000-a8b2229366944421bc4b2f2ba26a1003.jpg)
JGI / Jamie Grill / Getty Images
- Application
- Improvement
From deciding what to eat for dinner to considering whether it's the right time to buy a house, problem-solving is a large part of our daily lives. Learn some of the problem-solving strategies that exist and how to use them in real life, along with ways to overcome obstacles that are making it harder to resolve the issues you face.
What Is Problem-Solving?
In cognitive psychology , the term 'problem-solving' refers to the mental process that people go through to discover, analyze, and solve problems.
A problem exists when there is a goal that we want to achieve but the process by which we will achieve it is not obvious to us. Put another way, there is something that we want to occur in our life, yet we are not immediately certain how to make it happen.
Maybe you want a better relationship with your spouse or another family member but you're not sure how to improve it. Or you want to start a business but are unsure what steps to take. Problem-solving helps you figure out how to achieve these desires.
The problem-solving process involves:
- Discovery of the problem
- Deciding to tackle the issue
- Seeking to understand the problem more fully
- Researching available options or solutions
- Taking action to resolve the issue
Before problem-solving can occur, it is important to first understand the exact nature of the problem itself. If your understanding of the issue is faulty, your attempts to resolve it will also be incorrect or flawed.
Problem-Solving Mental Processes
Several mental processes are at work during problem-solving. Among them are:
- Perceptually recognizing the problem
- Representing the problem in memory
- Considering relevant information that applies to the problem
- Identifying different aspects of the problem
- Labeling and describing the problem
Problem-Solving Strategies
There are many ways to go about solving a problem. Some of these strategies might be used on their own, or you may decide to employ multiple approaches when working to figure out and fix a problem.
An algorithm is a step-by-step procedure that, by following certain "rules" produces a solution. Algorithms are commonly used in mathematics to solve division or multiplication problems. But they can be used in other fields as well.
In psychology, algorithms can be used to help identify individuals with a greater risk of mental health issues. For instance, research suggests that certain algorithms might help us recognize children with an elevated risk of suicide or self-harm.
One benefit of algorithms is that they guarantee an accurate answer. However, they aren't always the best approach to problem-solving, in part because detecting patterns can be incredibly time-consuming.
There are also concerns when machine learning is involved—also known as artificial intelligence (AI)—such as whether they can accurately predict human behaviors.
Heuristics are shortcut strategies that people can use to solve a problem at hand. These "rule of thumb" approaches allow you to simplify complex problems, reducing the total number of possible solutions to a more manageable set.
If you find yourself sitting in a traffic jam, for example, you may quickly consider other routes, taking one to get moving once again. When shopping for a new car, you might think back to a prior experience when negotiating got you a lower price, then employ the same tactics.
While heuristics may be helpful when facing smaller issues, major decisions shouldn't necessarily be made using a shortcut approach. Heuristics also don't guarantee an effective solution, such as when trying to drive around a traffic jam only to find yourself on an equally crowded route.
Trial and Error
A trial-and-error approach to problem-solving involves trying a number of potential solutions to a particular issue, then ruling out those that do not work. If you're not sure whether to buy a shirt in blue or green, for instance, you may try on each before deciding which one to purchase.
This can be a good strategy to use if you have a limited number of solutions available. But if there are many different choices available, narrowing down the possible options using another problem-solving technique can be helpful before attempting trial and error.
In some cases, the solution to a problem can appear as a sudden insight. You are facing an issue in a relationship or your career when, out of nowhere, the solution appears in your mind and you know exactly what to do.
Insight can occur when the problem in front of you is similar to an issue that you've dealt with in the past. Although, you may not recognize what is occurring since the underlying mental processes that lead to insight often happen outside of conscious awareness .
Research indicates that insight is most likely to occur during times when you are alone—such as when going on a walk by yourself, when you're in the shower, or when lying in bed after waking up.
How to Apply Problem-Solving Strategies in Real Life
If you're facing a problem, you can implement one or more of these strategies to find a potential solution. Here's how to use them in real life:
- Create a flow chart . If you have time, you can take advantage of the algorithm approach to problem-solving by sitting down and making a flow chart of each potential solution, its consequences, and what happens next.
- Recall your past experiences . When a problem needs to be solved fairly quickly, heuristics may be a better approach. Think back to when you faced a similar issue, then use your knowledge and experience to choose the best option possible.
- Start trying potential solutions . If your options are limited, start trying them one by one to see which solution is best for achieving your desired goal. If a particular solution doesn't work, move on to the next.
- Take some time alone . Since insight is often achieved when you're alone, carve out time to be by yourself for a while. The answer to your problem may come to you, seemingly out of the blue, if you spend some time away from others.
Obstacles to Problem-Solving
Problem-solving is not a flawless process as there are a number of obstacles that can interfere with our ability to solve a problem quickly and efficiently. These obstacles include:
- Assumptions: When dealing with a problem, people can make assumptions about the constraints and obstacles that prevent certain solutions. Thus, they may not even try some potential options.
- Functional fixedness : This term refers to the tendency to view problems only in their customary manner. Functional fixedness prevents people from fully seeing all of the different options that might be available to find a solution.
- Irrelevant or misleading information: When trying to solve a problem, it's important to distinguish between information that is relevant to the issue and irrelevant data that can lead to faulty solutions. The more complex the problem, the easier it is to focus on misleading or irrelevant information.
- Mental set: A mental set is a tendency to only use solutions that have worked in the past rather than looking for alternative ideas. A mental set can work as a heuristic, making it a useful problem-solving tool. However, mental sets can also lead to inflexibility, making it more difficult to find effective solutions.
How to Improve Your Problem-Solving Skills
In the end, if your goal is to become a better problem-solver, it's helpful to remember that this is a process. Thus, if you want to improve your problem-solving skills, following these steps can help lead you to your solution:
- Recognize that a problem exists . If you are facing a problem, there are generally signs. For instance, if you have a mental illness , you may experience excessive fear or sadness, mood changes, and changes in sleeping or eating habits. Recognizing these signs can help you realize that an issue exists.
- Decide to solve the problem . Make a conscious decision to solve the issue at hand. Commit to yourself that you will go through the steps necessary to find a solution.
- Seek to fully understand the issue . Analyze the problem you face, looking at it from all sides. If your problem is relationship-related, for instance, ask yourself how the other person may be interpreting the issue. You might also consider how your actions might be contributing to the situation.
- Research potential options . Using the problem-solving strategies mentioned, research potential solutions. Make a list of options, then consider each one individually. What are some pros and cons of taking the available routes? What would you need to do to make them happen?
- Take action . Select the best solution possible and take action. Action is one of the steps required for change . So, go through the motions needed to resolve the issue.
- Try another option, if needed . If the solution you chose didn't work, don't give up. Either go through the problem-solving process again or simply try another option.
You can find a way to solve your problems as long as you keep working toward this goal—even if the best solution is simply to let go because no other good solution exists.
Sarathy V. Real world problem-solving . Front Hum Neurosci . 2018;12:261. doi:10.3389/fnhum.2018.00261
Dunbar K. Problem solving . A Companion to Cognitive Science . 2017. doi:10.1002/9781405164535.ch20
Stewart SL, Celebre A, Hirdes JP, Poss JW. Risk of suicide and self-harm in kids: The development of an algorithm to identify high-risk individuals within the children's mental health system . Child Psychiat Human Develop . 2020;51:913-924. doi:10.1007/s10578-020-00968-9
Rosenbusch H, Soldner F, Evans AM, Zeelenberg M. Supervised machine learning methods in psychology: A practical introduction with annotated R code . Soc Personal Psychol Compass . 2021;15(2):e12579. doi:10.1111/spc3.12579
Mishra S. Decision-making under risk: Integrating perspectives from biology, economics, and psychology . Personal Soc Psychol Rev . 2014;18(3):280-307. doi:10.1177/1088868314530517
Csikszentmihalyi M, Sawyer K. Creative insight: The social dimension of a solitary moment . In: The Systems Model of Creativity . 2015:73-98. doi:10.1007/978-94-017-9085-7_7
Chrysikou EG, Motyka K, Nigro C, Yang SI, Thompson-Schill SL. Functional fixedness in creative thinking tasks depends on stimulus modality . Psychol Aesthet Creat Arts . 2016;10(4):425‐435. doi:10.1037/aca0000050
Huang F, Tang S, Hu Z. Unconditional perseveration of the short-term mental set in chunk decomposition . Front Psychol . 2018;9:2568. doi:10.3389/fpsyg.2018.02568
National Alliance on Mental Illness. Warning signs and symptoms .
Mayer RE. Thinking, problem solving, cognition, 2nd ed .
Schooler JW, Ohlsson S, Brooks K. Thoughts beyond words: When language overshadows insight. J Experiment Psychol: General . 1993;122:166-183. doi:10.1037/0096-3445.2.166
By Kendra Cherry, MSEd Kendra Cherry, MS, is a psychosocial rehabilitation specialist, psychology educator, and author of the "Everything Psychology Book."
- Action Learning

What is Action Learning?
Action Learning is a process that involves a small group working on real problems, taking action, and learning as individuals, as a team, and as an organization. It helps organizations develop creative, flexible and successful strategies to pressing problems.
Action Learning Solves Problems

Action Learning Components
Action Learning is a process of insightful questioning and reflective listening.
Action Learning tackles problems through a process of first asking questions to clarify the exact nature of the problem, reflecting and identifying possible solutions, and only then taking action. Questions build group dialogue and cohesiveness, generate innovative and systems thinking, and enhance learning results.
1. A Problem
2. an action learning group or team, 3. a process of insightful questioning and reflective listening, 4. an action taken on the problem, 5. a commitment to learning, 6. an action learning coach, does action learning interest you, looking for an action learning coach.
- Our Services
- Foundations
- CALC Courses
- In-House Programs
- WIAL-Endorsed Programs and Products
- Become a Coach
- Find a Coach
- Become an Affiliate
- Find an Affiliate
- Become a Partner
- Find a Partner
- 2023 Global Action Learning Week
- 2021 Global Conference
- Past Conferences
- Client Testimonials
- Share your Success Story
- WIAL Awards
- Award Nomination
- Previous WIAL Award Winners
- Better World Fund
- Better World Stories
- Share your Better World Story
- WIAL Gives Back
- Executive Committee
- Board of Directors
- Directors Emeritus
- Advisory Board
- Youth Cardio Equipment
- Challenge Courses and Climbing
- School Spotlights
- Research & Data
- Grants & Funding
- State Vendor No. & Contracts
Problem Solving

Problem Solving, what is it and why is it important?
Problem Solving takes information from what we already know and adds it together to make a sequence of events to solve a specific task.
Problem solving is a skill that must be taught, and practiced in order to develop the skills necessary for success throughout grade school, into adulthood.
We practice problem solving from a very young age. Learning how to problem solve applies to both academics, and real world situations. Students need problem solving skills to learn to navigate through different situations which will impact their ability to preform tasks, especially later on in life.
Problem solving involves much of the brain to help focus and attend to the information necessary to overcome challenges....... The prefrontal cortex controls executive functions like decision-making, problem solving, memory, language, emotions and attention. The cerebellum initiates putting patterns into a sequence, like letters into words, words into a sentences and numbers into order. Activities that activate the cerebellum while performing challenging cognitive skills strengthens areas in the brain for focus and attention.
If a child is lacking problem solving skills........ A prominent sign children are struggling with problem solving skills is their inability to understand a concept such as putting patterns into a sequence, how to handle a difficult situation, or having trouble working with others to solve a problem. If a child can't figure out how to put patterns into a sequence, they might struggle with where to start with steps to a task and how to stay on track with steps. Another situation might be them having trouble with classmates and not knowing how to handle it appropriately.
We can practice and strengthen problem solving skills through embodied cognition........... Embodied cognitions is using gestures, actions and emotions to help understand a concept.
-------------------
What's new at abl.
ACTION BASED LEARNING CERTIFICATIONS
ABL-LF Certified Action Based Learning Lab Faciliator
ABL-ST Certified Action Based Learning School Trainer
ABL-DT Certified Action Based Learning District Trainer
What is the difference between each certification?
The Lab facilitator certification is a specialized certification specifically for implementing, managing, and enhancing the effectiveness of your ABL Lab. This certification is designed for Lab Facilitators, or educators and administrators involved in creating and maintaining a successful lab in your school. The school and district certification on the other hand, extend well beyond the lab, to help you become an expert on all things movement and learning, including creating active classrooms and active schools, and overall culture of healthy, happy, engaged, using movement!
ABL PRODUCT TOP PICKS

NEW! ABL Academic Card Set Boxes include complete collections of Elementary Academic Station Cards for Math, ELAR, SS, Health and Wellness, and more!
VIEW ALL ACADEMIC BOXES


What is Problem Solving? (Steps, Techniques, Examples)
By Status.net Editorial Team on May 7, 2023 — 5 minutes to read
What Is Problem Solving?
Definition and importance.
Problem solving is the process of finding solutions to obstacles or challenges you encounter in your life or work. It is a crucial skill that allows you to tackle complex situations, adapt to changes, and overcome difficulties with ease. Mastering this ability will contribute to both your personal and professional growth, leading to more successful outcomes and better decision-making.
Problem-Solving Steps
The problem-solving process typically includes the following steps:
- Identify the issue : Recognize the problem that needs to be solved.
- Analyze the situation : Examine the issue in depth, gather all relevant information, and consider any limitations or constraints that may be present.
- Generate potential solutions : Brainstorm a list of possible solutions to the issue, without immediately judging or evaluating them.
- Evaluate options : Weigh the pros and cons of each potential solution, considering factors such as feasibility, effectiveness, and potential risks.
- Select the best solution : Choose the option that best addresses the problem and aligns with your objectives.
- Implement the solution : Put the selected solution into action and monitor the results to ensure it resolves the issue.
- Review and learn : Reflect on the problem-solving process, identify any improvements or adjustments that can be made, and apply these learnings to future situations.
Defining the Problem
To start tackling a problem, first, identify and understand it. Analyzing the issue thoroughly helps to clarify its scope and nature. Ask questions to gather information and consider the problem from various angles. Some strategies to define the problem include:
- Brainstorming with others
- Asking the 5 Ws and 1 H (Who, What, When, Where, Why, and How)
- Analyzing cause and effect
- Creating a problem statement
Generating Solutions
Once the problem is clearly understood, brainstorm possible solutions. Think creatively and keep an open mind, as well as considering lessons from past experiences. Consider:
- Creating a list of potential ideas to solve the problem
- Grouping and categorizing similar solutions
- Prioritizing potential solutions based on feasibility, cost, and resources required
- Involving others to share diverse opinions and inputs
Evaluating and Selecting Solutions
Evaluate each potential solution, weighing its pros and cons. To facilitate decision-making, use techniques such as:
- SWOT analysis (Strengths, Weaknesses, Opportunities, Threats)
- Decision-making matrices
- Pros and cons lists
- Risk assessments
After evaluating, choose the most suitable solution based on effectiveness, cost, and time constraints.
Implementing and Monitoring the Solution
Implement the chosen solution and monitor its progress. Key actions include:
- Communicating the solution to relevant parties
- Setting timelines and milestones
- Assigning tasks and responsibilities
- Monitoring the solution and making adjustments as necessary
- Evaluating the effectiveness of the solution after implementation
Utilize feedback from stakeholders and consider potential improvements. Remember that problem-solving is an ongoing process that can always be refined and enhanced.
Problem-Solving Techniques
During each step, you may find it helpful to utilize various problem-solving techniques, such as:
- Brainstorming : A free-flowing, open-minded session where ideas are generated and listed without judgment, to encourage creativity and innovative thinking.
- Root cause analysis : A method that explores the underlying causes of a problem to find the most effective solution rather than addressing superficial symptoms.
- SWOT analysis : A tool used to evaluate the strengths, weaknesses, opportunities, and threats related to a problem or decision, providing a comprehensive view of the situation.
- Mind mapping : A visual technique that uses diagrams to organize and connect ideas, helping to identify patterns, relationships, and possible solutions.
Brainstorming
When facing a problem, start by conducting a brainstorming session. Gather your team and encourage an open discussion where everyone contributes ideas, no matter how outlandish they may seem. This helps you:
- Generate a diverse range of solutions
- Encourage all team members to participate
- Foster creative thinking
When brainstorming, remember to:
- Reserve judgment until the session is over
- Encourage wild ideas
- Combine and improve upon ideas
Root Cause Analysis
For effective problem-solving, identifying the root cause of the issue at hand is crucial. Try these methods:
- 5 Whys : Ask “why” five times to get to the underlying cause.
- Fishbone Diagram : Create a diagram representing the problem and break it down into categories of potential causes.
- Pareto Analysis : Determine the few most significant causes underlying the majority of problems.
SWOT Analysis
SWOT analysis helps you examine the Strengths, Weaknesses, Opportunities, and Threats related to your problem. To perform a SWOT analysis:
- List your problem’s strengths, such as relevant resources or strong partnerships.
- Identify its weaknesses, such as knowledge gaps or limited resources.
- Explore opportunities, like trends or new technologies, that could help solve the problem.
- Recognize potential threats, like competition or regulatory barriers.
SWOT analysis aids in understanding the internal and external factors affecting the problem, which can help guide your solution.
Mind Mapping
A mind map is a visual representation of your problem and potential solutions. It enables you to organize information in a structured and intuitive manner. To create a mind map:
- Write the problem in the center of a blank page.
- Draw branches from the central problem to related sub-problems or contributing factors.
- Add more branches to represent potential solutions or further ideas.
Mind mapping allows you to visually see connections between ideas and promotes creativity in problem-solving.
Examples of Problem Solving in Various Contexts
In the business world, you might encounter problems related to finances, operations, or communication. Applying problem-solving skills in these situations could look like:
- Identifying areas of improvement in your company’s financial performance and implementing cost-saving measures
- Resolving internal conflicts among team members by listening and understanding different perspectives, then proposing and negotiating solutions
- Streamlining a process for better productivity by removing redundancies, automating tasks, or re-allocating resources
In educational contexts, problem-solving can be seen in various aspects, such as:
- Addressing a gap in students’ understanding by employing diverse teaching methods to cater to different learning styles
- Developing a strategy for successful time management to balance academic responsibilities and extracurricular activities
- Seeking resources and support to provide equal opportunities for learners with special needs or disabilities
Everyday life is full of challenges that require problem-solving skills. Some examples include:
- Overcoming a personal obstacle, such as improving your fitness level, by establishing achievable goals, measuring progress, and adjusting your approach accordingly
- Navigating a new environment or city by researching your surroundings, asking for directions, or using technology like GPS to guide you
- Dealing with a sudden change, like a change in your work schedule, by assessing the situation, identifying potential impacts, and adapting your plans to accommodate the change.
- How to Resolve Employee Conflict at Work [Steps, Tips, Examples]
- How to Write Inspiring Core Values? 5 Steps with Examples
- 30 Employee Feedback Examples (Positive & Negative)

In order to continue enjoying our site, we ask that you confirm your identity as a human. Thank you very much for your cooperation.

Center for Teaching
Teaching problem solving.
Print Version
Tips and Techniques
Expert vs. novice problem solvers, communicate.
- Have students identify specific problems, difficulties, or confusions . Don’t waste time working through problems that students already understand.
- If students are unable to articulate their concerns, determine where they are having trouble by asking them to identify the specific concepts or principles associated with the problem.
- In a one-on-one tutoring session, ask the student to work his/her problem out loud . This slows down the thinking process, making it more accurate and allowing you to access understanding.
- When working with larger groups you can ask students to provide a written “two-column solution.” Have students write up their solution to a problem by putting all their calculations in one column and all of their reasoning (in complete sentences) in the other column. This helps them to think critically about their own problem solving and helps you to more easily identify where they may be having problems. Two-Column Solution (Math) Two-Column Solution (Physics)
Encourage Independence
- Model the problem solving process rather than just giving students the answer. As you work through the problem, consider how a novice might struggle with the concepts and make your thinking clear
- Have students work through problems on their own. Ask directing questions or give helpful suggestions, but provide only minimal assistance and only when needed to overcome obstacles.
- Don’t fear group work ! Students can frequently help each other, and talking about a problem helps them think more critically about the steps needed to solve the problem. Additionally, group work helps students realize that problems often have multiple solution strategies, some that might be more effective than others
Be sensitive
- Frequently, when working problems, students are unsure of themselves. This lack of confidence may hamper their learning. It is important to recognize this when students come to us for help, and to give each student some feeling of mastery. Do this by providing positive reinforcement to let students know when they have mastered a new concept or skill.
Encourage Thoroughness and Patience
- Try to communicate that the process is more important than the answer so that the student learns that it is OK to not have an instant solution. This is learned through your acceptance of his/her pace of doing things, through your refusal to let anxiety pressure you into giving the right answer, and through your example of problem solving through a step-by step process.
Experts (teachers) in a particular field are often so fluent in solving problems from that field that they can find it difficult to articulate the problem solving principles and strategies they use to novices (students) in their field because these principles and strategies are second nature to the expert. To teach students problem solving skills, a teacher should be aware of principles and strategies of good problem solving in his or her discipline .
The mathematician George Polya captured the problem solving principles and strategies he used in his discipline in the book How to Solve It: A New Aspect of Mathematical Method (Princeton University Press, 1957). The book includes a summary of Polya’s problem solving heuristic as well as advice on the teaching of problem solving.
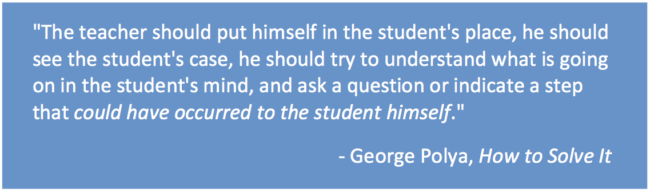
Teaching Guides
- Online Course Development Resources
- Principles & Frameworks
- Pedagogies & Strategies
- Reflecting & Assessing
- Challenges & Opportunities
- Populations & Contexts
Quick Links
- Services for Departments and Schools
- Examples of Online Instructional Modules
(Stanford users can avoid this Captcha by logging in.)
- Send to text email RefWorks EndNote printer
The ideal problem solver : a guide for improving thinking, learning, and creativity
Available online, at the library.

Law Library (Crown)
Call number | Note | Status |
---|---|---|
BF449 .B73 1993 | Unknown |
More options
- Find it at other libraries via WorldCat
- Contributors
Description
Creators/contributors, contents/summary.
- CHAPTER I THE IMPORTANCE OF PROBLEM SOLVING: New Views about Thinking and Problem Solving
- Some Common Approaches to Problems
- Menmi Escapes
- The Purpose and Structure of This Book
- PART I A FRAMEWORK FOR USING KNOWLEDGE MORE EFFECTIVELY: CHAPTER 2 A MODEL FOR IMPROVING PROBLEM-SOLVING SKILLS: The IDEAL Approach to Problem Solving
- Failure to Identify the Possibility of Future Problems
- The Importance of Conceptual Inventions
- The Importance of Systematic Analysis
- The Importance of Using External Representations
- Some Additional General Strategies
- The Importance of Specialized Concepts and Strategies
- The Importance of Acting on Strategies to Evaluate Outcomes
- The IDEAL Cycle
- Blocks to Creative Problem Solving
- CHAPTER 3 CREATIVITY AND THE IDEAL FRAMEWORK: Identify Problems and Opportunities
- Define Alternative Goals
- Explore Possible Strategies
- Anticipate and Act
- Look and Learn
- The IDEAL Cycle and Creativity
- CHAPTER 4 INTELLIGENT CRITICISM: The Importance of Evaluating Arguments
- Three Ways of Critically Evaluating Arguments
- The Analysis of Factual Claims
- Evaluating the Reasoning of Arguments
- The Analysis of Key Assumptions
- CHAPTER 5 EFFECTIVE COMMUNICATION: The Importance of Identifying Communication Problems
- Three Ways of Defining Communication Goals
- Presentation Medium
- Evaluating the Effectiveness of Your Attempts to Communicate
- PART 2 A MODEL FOR ACQUIRING NEW KNOWLEDGE: CHAPTER 6 IMPROVING MEMORY SKILLS: Remembering as Problem Solving
- Understanding Basic Memory Processes
- Strategies for Remembering
- Adapting and Inventing Memory Strategies
- CHAPTER 7 LEARNING WITH UNDERSTANDING : Comprehension as Problem Solving
- Learning about New Areas of Knowledge
- Anticipating Outcomes and Acting on Comprehension
- Looking at the Effects and Learning to Learn
- The IDEAL Cycle and Lifelong Learning
- CHAPTER 8 INSTRUCTION THAT FACILITATES PROBLEM SOLVING: Why Problem Solving Needs to Be an Integral Part of Education
- Problems with Traditional Approaches to Instruction
- Organizing Learning Around Problem Solving
- General Issues Related to Problem-Based Learning
- The Importance of Opportunities for Assessment
- CHAPTER 9 CONCLUDING REMARKS: The Development of Problem-Solving Skills
- Notes. APPENDIX A ANSWERS TO PROBLEMS IN TEXT. APPENDIX B ANSWERS TOEXERCISES. APPENDIX C IDEAL PROBLEM NAVIGATION GUIDE.
- (source: Nielsen Book Data)
Bibliographic information
Browse related items.

- Stanford Home
- Maps & Directions
- Search Stanford
- Emergency Info
- Terms of Use
- Non-Discrimination
- Accessibility
© Stanford University , Stanford , California 94305 .
- Open access
- Published: 03 March 2022
The cognitive principles of learning underlying the 5E Model of Instruction
- Héctor Ruiz-Martín ORCID: orcid.org/0000-0001-6264-0829 1 &
- Rodger W. Bybee 2
International Journal of STEM Education volume 9 , Article number: 21 ( 2022 ) Cite this article
36k Accesses
21 Altmetric
Metrics details
Over 34 years since its conception, research in educational settings has found evidence for the effectiveness of the 5E Instructional Model. Indeed, several studies have reported evidence of a better conceptual understanding of scientific ideas and models, positive effects on general achievement in science, and positive attitudes toward science. In this commentary, we would like to put forward the principles from cognitive sciences on how people learn which may underlie the 5E Model and that could theoretically contribute to the model’s effectiveness as a learning sequence. Connections to conceptual change theory are especially highlighted.
Introduction
The 5E Instructional Model is an approach to science teaching and learning developed by the Biological Sciences Curriculum Study (BSCS) in 1987. As a learning sequence, the 5E Model builds on the work of other instructional models, such as the Atkin and Karplus Learning Cycle or the Science Curriculum Improvement Study (SCIS) Learning Cycle, with which it shares some steps (Bybee, 2015 ; Bybee et al., 2006 ). The 5E Model consists of five phases: engagement, exploration, explanation, elaboration, and evaluation. A description of the phases can be found in Table 1 . Each phase has a specific function and overall they aim to help the teacher provide coherent instruction and the learner to engage in appropriate activities that will presumably promote a better understanding and retention of scientific knowledge.
The effectiveness of the 5E Model regarding the improvement of several science education outcomes has been supported by research conducted in schools over the last decades, mainly with primary and secondary school students from different socioeconomic backgrounds (for a review, see Bybee et al., 2006 and Bybee, 2015 ). Several studies have provided evidence of a better conceptual understanding of scientific ideas and models, long-term decreases in the prevalence of alternative conceptions, positive effects on general achievement in science, gains in students’ self-expressed interest and confidence in science and scientific careers, and positive attitudes toward science (Kilavuz, 2005 ; Bybee et al., 2006 ; Cardak et al., 2008 ; Garcia-Grau, 2021 ; Hokkanen, 2011 ).
In this commentary, we would like to put forward the cognitive principles of learning that may underlie the 5E Model and that could theoretically explain its effectiveness as a learning sequence, according to basic research in cognitive science of learning and memory. By 'cognitive principles of learning', we mean theories and models of how learning works that have been developed by research mainly in cognitive psychology and cognitive neuroscience, although we also draw on research from developmental psychology and other behavioral disciplines that assume the cognitivist paradigm. Thus, our dissertation includes references to socio-cognitive theories of motivation and the conceptual change theory, for example.
Next, we highlight potentially plausible connections between the 5E Model and research literature from cognitive sciences for each of the stages of the Model.
In the first stage of the 5E Model, called Engage, the teacher introduces a problem or a discrepant event in a familiar context that students cannot explain with their current knowledge because it falls too short or does not fit in with their new experiences. As a result of this, a cognitive conflict arises. This conflict plays a motivational role and provides an opportunity to activate and elicit the students’ prior knowledge.
From the cognitive perspective, the Engage stage of the 5E Model is exceptionally important because it addresses several processes which have proven critical for enhancing meaningful, durable, and transferable learning.
Firstly, research has shown that encouraging students to recall relevant knowledge from previous courses or their own lives (i.e., activating prior knowledge) can facilitate the integration of new material (Bransford & Johnson, 1972 ; Hattan et al., 2015 ; Peeck et al., 1982 ). In this regard, strategies such as asking students questions specifically designed to trigger recall or providing learners with a relevant context can help them use prior knowledge to aid the integration and retention of new information (Wetzels et al., 2011 ; Woloshyn et al., 1994 ).
Supported by decades of empirical research in psychology and neuroscience, the cognitive theories of learning state that prior knowledge plays an essential role in the processing and retention of new information (Ausubel, 2012 ; Brod et al., 2013 ; Shing & Brod, 2016 ). In short, that which students can learn depends greatly on what they already know because learning is a constructive process: much of what they learn is built on the prior knowledge in their long-term memory. But, according to this view, having relevant knowledge is not enough: it needs to be prompted to become useful during the learning episode. This means that it must be activated in long-term memory and enter working memory, the “mental space” where the learner holds and manipulates the information they attend to. When this happens, new learning is generated: some pieces of the new information are connected to semantically related pieces of knowledge that the learner had retrieved from long-term memory. Later, when the learner wants to retrieve what has been learned, they will reconstruct the memories using the related knowledge from different learning episodes. In other words, research suggests that human memory does not store redundant information. Instead, it uses information that is already consolidated in long-term memory to build on new memories that share those features. That is why learning would involve connecting prior knowledge to new information.
However, students may not spontaneously bring their prior knowledge to working memory in learning situations. In light of this, it has proved useful to provide explicit opportunities for students to activate their prior knowledge so they can build on it (Bransford & Johnson, 1972 ; Dooling & Lachman, 1971 ; Hattan et al., 2015 ). For instance, some students can spontaneously activate their prior knowledge while learning, while others need support and guidance (Carr & Thompson, 1996 ). These latter students can benefit from teacher prompting to activate prior knowledge (Carr & Thompson, 1996 ). The Engage stage of the 5E Model encourages teachers to assist their students to purposely and actively use their prior knowledge. In fact, since generating prior knowledge can be a double-edged sword, e.g., if the knowledge students generate is inappropriate for the context or inaccurate (Alvermann et al., 1985 ; van Loon et al., 2013 ), the role of the teacher as a guide is critical: they encourage students to openly voice their ideas, promote reflection, steer the students’ thoughts in the right direction, and guide classroom discussions. It is important not only to activate prior knowledge, but also to direct students’ attention to those aspects of their prior knowledge that will be relevant to the learning task.
Educational researchers and science educators do not only describe learning as an assimilation process, that is, as the incorporation of new information into the existing and appropriate knowledge structures without changing them (also known as knowledge accretion). Learning usually requires an accommodation process, where the pre-instructional conceptual structures of the learner need to be fundamentally restructured to allow understanding of the intended knowledge (Hanfstingl et al., 2021 ; Posner et al., 1982 ). Some authors in science education and developmental psychology refer to this as a ‘proper’ conceptual change (although most of them also consider knowledge accretion—assimilation—to be a lower level of conceptual change) (Harrison & Treagust, 2000 ). Cognitive scientists, on the other hand, refer to any change in prior knowledge as a memory updating process which involves changes in the way pieces of information in memory are connected to each other to build meaning and guide recalling (Bjork, 1978 ; Lee et al., 2017 ).
When learning requires an accommodation process, activation of prior knowledge becomes even more important. From the science education perspective it has been suggested that activating prior knowledge is key for triggering conceptual change due to motivational reasons: the learner realizes that they cannot use their current conceptions to explain the available data and this creates the need for a more adequate mental model. Posner et al. ( 1982 ) pointed to the detection of anomalies as the first step in conceptual change: “There must be dissatisfaction with existing conceptions. Students are unlikely to make major changes in their concepts until they believe that less radical changes will not work […]. An individual must have collected a store of […] anomalies and lost faith in the capacity of his current concepts to solve these problems.” However, although motivation is indeed a key mediator in the process of conceptual change (later we will discuss how the cognitive conflict implied in the Engage stage of the 5E Model can promote it), it should be noted that the activation of prior knowledge alone also contributes to conceptual change as a consequence of how memory works. Indeed, research in cognitive sciences suggests that activation of prior knowledge plays a key role in memory updating.
As said previously, activating prior knowledge appears to promote learning since, if interaction between new information and old knowledge is to take place, prior knowledge must be accessible. From the cognitive sciences perspective, activating prior knowledge may be essential for promoting conceptual change because only the memories that become activated are prone to change. We can even find evidence of this phenomenon in the cognitive neuroscience literature. For example, according to reconsolidation theory and the trace updating framework, when a memory is retrieved the synapses underlying the trace become unbound or weakened. Thus, reactivated memories are vulnerable to disruption and need to be reconsolidated (Finnie & Nader, 2012 ; Lee et al., 2017 ). However, since the retrieval episode is triggered and followed by related information in the environment, it provides an opportunity to update the memory trace: a reactivated trace is never just simply reconsolidated, it is modified to include information contained in the new experience (Dudai, 2004 , 2012 ; Hupbach et al., 2007 ).
Therefore, the Engage phase of the 5E Model, which is where the learning sequence begins, is seen as highly relevant from the cognitive perspective because it provides time and explicit opportunities for prior knowledge to be activated, which is essential for memory updating. However, this is just half of the story. As mentioned previously, the Engage stage also plays a significant role in promoting student motivation, which is another key factor for learning and triggering conceptual change (Pintrich et al., 1993 ; Sinatra & Pintrich, 2003 ). Indeed, students’ cognitive engagement is essential for attending to information, activating prior knowledge, monitoring comprehension, and persisting at the learning task. Regarding conceptual change, motivation is particularly important if the student is expected to abandon their prior conceptions and adopt new ones, a process that requires a substantial cognitive effort. That is why the Engage stage, as its name implies, aims to provide a motivational boost.
Indeed, the initial situation presented in the Engage stage involves two types of engagement: contextual and cognitive. Contextual engagement is based on the fact that students appreciate the real-life implications of the posed situation, that is, they recognize its instrumental value (Wigfield & Eccles, 2000 ). Clearly, learners are more motivated when they can see the usefulness of what they are learning (Maher & Midgley, 1991 ; McCombs, 1991 ). As for cognitive engagement, it is grounded in the curiosity that often arises when students realize that their current ideas cannot satisfactorily explain an observation that puzzles them, and therefore it is a consequence of the cognitive conflict or cognitive dissonance (Chaxel & Russo, 2015 ; Harmon-Jones et al., 2015 ; Kang et al., 2004 ; Posner et al., 1982 ). In a way, socio-cognitive theories of motivation suggest that people are motivated to learn from situations that pose problems that they believe they can solve (Barron & Hulleman, 2015 ; Wigfield & Eccles, 2000 ). At the same time, research showing that stories are “psychologically privileged”—because their structure enhances attention and memory—suggests that the introduction of a conflict to be solved is a key contributor to their effect (Ware & Young, 2010 ; Willingham, 2004 ). In sum, conflicts students believe they can solve are motivational.
The Engage stage is hugely important for the cognitive and the emotional (motivational) dimensions involved in the learning process. In addition, it also offers interesting opportunities for group discussions and cooperative learning, which, among others, are key socio-cognitive ways in which individuals learn and that may lead to more fruitful teaching and learning environments (Johnson & Johnson, 2009 ; Slavin, 2013 ).
The Explore stage consists of a guided inquiry activity that provides opportunities for students to address alternative conceptions and build new explanations that make sense to them. In the Explore stage students investigate phenomena, share their observations, suggest explanations, and discuss their interpretations. The role of the teacher is to act as a facilitator who guides and scaffolds students’ thinking.
After the activation of prior knowledge in the previous stage, the Explore stage aims to promote connections between students’ prior knowledge and the new information to be learned. To do so, the activities on this stage follow a guided inquiry-based approach (Gormally et al., 2009 ) that fosters thinking and sensemaking during the learning task. This contrasts with a type of learning that is only based on transmission, one in which the student often acts as a passive learner. From the cognitive perspective, this approach would be supported by one of the most basic findings of cognitive psychology: people learn and remember better when they think about what they are learning in terms of meaning, that is, when they are prompted to connect information in meaningful ways. This principle is related to the influential idea posited by Craik and Lockhart ( 1972 ), known as the levels of processing model , which describes memory encoding as a function of the depth of mental processing. According to this model, memory is the product of thought and deeper levels of processing during encoding produce more elaborate, longer-lasting, and stronger memory traces, where deep processing refers to greater degrees of semantic involvement—that is, thinking about meaning (for several perspectives on this topic, see Conway, 2002 ).
Regarding conceptual change, the Explore stage provides students with opportunities to reformulate their explanations by inferring them from new experiences and observations (Hewson, 1981 ). According to the classical model of conceptual change (Posner et al., 1982 ) after recognizing that prior conceptions are inconsistent in a given situation, students need to find an adequate new conception that successfully explains it. This new explanation must be “intelligible” (the learner must grasp its meaning) and “plausible” (the learner must see how it is consistent with other knowledge and explains the available data). Therefore, the Explore stage provides the chance for students to propose new explanations grounded on their understanding and test them empirically.
From the socio-cognitive perspective, in this stage, which can be accomplished with the whole class group or in small groups, the teacher’s role is to make sure students help one another solve problems by building on each other’s knowledge, by asking questions to clarify explanations, and by suggesting avenues that would move the group toward its goal (Brown & Campione, 1994 ). Research shows that both cooperation in problem-solving activities (Newstead & Evans, 1995 ) and argumentation (Youniss & Damon, 1992 ) among students enhance students’ cognitive development (i.e., their ability to learn, think, and reason).
Actually, the role of the teacher during the Explore stage is really important if the cognitive principles supporting learning are to be complied with. According to cognitive load theory (Sweller, 2011 ), inquiry-based learning activities must be carefully guided and extensively scaffolded by the teacher in order to facilitate students’ learning (Hmelo-Silver et al., 2007 ; Kirschner et al., 2006 ). Learning through investigations promotes students being cognitively engaged in sensemaking, developing evidence-based explanations, collaborating, and communicating their ideas. But it also places a huge burden on working memory. This working memory load does not contribute to the accumulation of knowledge in long-term memory because while working memory is being used to search for explanations, it is not available and cannot be used to learn (Kirschner et al., 2006 ). Scaffolded inquiry environments provide learners with opportunities to engage in complex tasks that would otherwise be beyond their current abilities and overwhelm working memory. Scaffolding keeps cognitive load at bay and makes the learning more manageable for students by modifying complex and difficult tasks in ways that make them within the student’s zone of proximal development (Vygotsky, 1978 ). In this regard, scaffolding is a key element of cognitive apprenticeship, whereby students become increasingly knowledgeable given structure and guidance from mentors who scaffold them through task structuring and sequencing, questions, and hints, but without explicitly giving students the final answers (Quintana et al., 2004 ).
Finally, the Explore stage provides students with opportunities to grapple with specific information relevant to the learning objectives, which is known to create a “time for telling”, that is, a situation that activates the students’ prior knowledge and that therefore enables them to learn much more from an organized explanation (Edelson, 2001 ; Schwartz & Bransford, 1998 ), such as those provided in the following Explain stage.
When students understand the necessity of new information and its relevance to their explorational practices, a just-in-time explanation can promote knowledge construction in a way that makes it available for future use in relevant contexts (Edelson, 2001 ). Therefore, the new concepts grasped in the Explore stage are formalized in the Explain stage, which provides opportunities for teachers or the curriculum to directly and formally introduce those concepts and help students organize their new knowledge in ways that facilitate encoding and later retrieval. The way students organize knowledge influences how they learn and apply what they know (Ambrose et al., 2010 ). Research shows that when students are provided with an organizational structure in which to fit new knowledge, they learn more effectively than when they are left to deduce this conceptual structure for themselves (Ausubel, 1960 , 1978 ; Bower et al., 1969 ; Kirschner et al., 2006 ). Of course, formal definitions and explanations of the intended models and concepts can also be cooperatively built by students with the close guidance of their teacher.
The Elaborate stage includes activities that require students to apply the concepts and procedures they have learned to solve new problems in new contexts. Here the new concepts will have the chance to prove they are “fruitful”, as Posner’s conceptual change model suggests: “if the new conception not only resolves its predecessor’s anomalies but also leads to new insights and discoveries, then the new conception will appear fruitful and the accommodation of it will seem persuasive” (Posner et al., 1982 ).
Therefore, the activities in the Elaborate stage provide opportunities for students to transfer their new knowledge to a wide diversity of contexts. Research suggests that exposing students to multiple contexts promotes deeper understanding, maybe because they are more likely to abstract the relevant features of concepts and to develop a flexible representation of knowledge (Bransford et al., 1990 ; Gentner et al., 1993 ; Gick & Holyoak, 1983 ; Kimball & Holyoak, 2000 ).
There often is a tendency to think that once students have the basic knowledge needed for an adequate understanding of a phenomenon, then it is relatively simple to apply that knowledge to the solution of practical problems. Unfortunately, transfer is not that straightforward. We’ve long known, since Thorndike and Woodworth’s pioneering experiments ( 1901a , b , c ), that transfer is very difficult to occur. The degree of similarity between learning and transfer contexts is critical (Barnett & Ceci, 2002 ). However, the way students learn is equally important in that it determines the potential for transfer to occur (Butler, 2010 ). If learning activities, by providing multiple contexts of applicability for the same concepts, create several retrieval routes to access the learned information, then the probability of finding a match between the cues given in the transfer task and the stored memory trace should be greater, thereby increasing the potential for transfer to occur. For example, asking students a series of questions that target the same concept and giving them feedback—hence encouraging students to think about that concept in different contexts—significantly increases the chances of transferring knowledge to new situations (Pan & Rickard, 2018 ).
To sum up, the Elaborate stage provides a space for extended practice applying the learned concepts and procedures in multiple situations. Extended practice is essential if something new is to be learned, especially if the goal is for that new knowledge to be retained over time and transferred to new situations (Healy et al., 1993 ).
Finally, in the Evaluate stage, the knowledge and abilities acquired by each student are assessed through an activity that challenges their understanding. Of course, evaluation can already take place during the previous stages of the instructional sequence as a formative assessment that provides opportunities for feedback throughout the whole learning process (Wiliam, 2011 ). Indeed, designers of the 5E Model recommend integrating the Evaluate stage throughout the whole learning sequence (Bybee, 2015 ). However, responding to a practical educational matter, science teachers must assess educational outcomes and assign students grades. Therefore, if this phase is applied at the end of the learning sequence, teachers can administer tests or other types of evaluative activities to determine the individual students’ level of understanding and abilities. This is also an important opportunity for students to use the skills they have acquired and evaluate their own understanding.
As a side note, it is important to underscore the fact that since the 5E Model aims to promote learning with understanding, the evaluative activities should be designed accordingly. In this regard, tests requiring transfer help assess the quality of students’ learning experiences. As stated in NRC’s How People Learn , a synthesis of decades of research across many different disciplines about the science of learning, “different kinds of learning experiences can look equivalent when tests of learning focus solely on remembering, but they can look quite different when tests of transfer are used” (Bransford et al., 2000 ). Therefore, the design of the assessment tool will be key to revealing students' genuine understanding and thus appreciating the contribution of the 5E sequence to learning.
From a cognitive perspective, evaluation is not only useful for assessing learning and providing timely feedback. Cognitive psychologists have shown that retrieving information from long-term memory is one of the most effective actions for strengthening learning, even more than restudying (i.e., reactivating the memory trace by reviewing the information) for an equivalent amount of time (Karpicke & Roediger, 2008 ; Rowland, 2014 ). Although testing is not usually understood as part of the learning process but as means to assess what has been learned, the act of retrieving information from memory actually changes memory, increasing the probability of successful retrieval in the future (Roediger & Butler, 2011 ). This was already noticed in 1890 by psychologist William James, who, probably relying on introspection, wrote:
A curious peculiarity of our memory is that things are impressed better by active than by passive repetition. I mean that in learning (by heart, for example), when we almost know the piece, it pays better to wait and recollect by an effort from within, than to look at the book again. If we recover the words in the former way, we shall probably know them the next time; if in the latter way, we shall very likely need the book once more.
Experimental reports from the beginning of the twentieth century through to today have proven him right: retrieval enhances retention (Karpicke & Roediger, 2008 ). Interestingly, according to several studies, retrieval may not merely produce transient, rote knowledge, but promote long-term, meaningful knowledge that can be retrieved flexibly and transferred to new situations (Karpicke, 2012 ; McDaniel et al., 2013 ). In addition, retrieval can help students organize information and form a coherent knowledge base (Roediger et al., 2011 ). Thus, the act of retrieval itself, which is central in an evaluative activity, is a powerful tool for enhancing learning. The 5E Evaluate phase, therefore, is also a learning phase, especially if it is applied not just at the end, but throughout the whole learning sequence.
It must be highlighted that, although evaluative activities can enhance learning by means of the retrieval practice effect (also known as the testing effect), tests can be stressful for students. This can have a negative impact on their memory and learning, which defeats the very purpose of assessing students for learning in the first place. In this regard, research suggests that the benefits of retrieval practice can be harnessed by using low stakes assessment activities (e.g., quizzing) that avoid the detrimental effects of stress associated with testing (McDaniel et al., 2011 ; Wenzel & Reinhard, 2021 ; Yang et al., 2021 ).
Neuroscientists have already suggested theoretical explanations of the neurocognitive mechanisms that may be underlying the retrieval effect (e.g., Antony et al., 2017 ). When an engram (the pattern of neural connections that represents a memory in the brain) is reactivated, it experiences a new round of consolidation processes. This eventually causes some strengthening of the original memory trace. However, it is also an opportunity for the trace to be modified since it becomes labile just before it is reconsolidated (Dudai, 2004 , 2012 ). Regarding these mechanisms, some neuroscientists have suggested that unlike restudying (i.e., encoding the same information again), which only reactivates the specific engrams corresponding to the material that was studied initially, retrieval is an imprecise process that coactivates memories that are semantically linked to the target memory, thereby affording an opportunity to integrate the original engram into the coactivated knowledge structures (Antony et al., 2017 ). Therefore, according to these hypothesis, not only does retrieving strengthen parts of the original engram, but it also promotes further connections to prior knowledge, increasing its transferability. The fact that retrieving knowledge changes that knowledge and its connections with other semantic-related knowledge may have evident consequences for conceptual change theory. Retrieval practice (including prior knowledge activation) may be essential for conceptual change.
Finally, retrieval practice can also improve the conceptual organization of practiced materials, especially when the posed questions are open-ended. As already posited by Gates as early as 1917, one of the reasons retrieval practice enhances knowledge organization is that it requires students to organize information more than does reading alone. When students actively recall information, they need to give it a coherent structure (Roediger et al., 2011 ).
Conclusions
The 5E Model of Instruction was designed in the late 1980s with the idea of translating decades of research in science education into a learning cycle that instructors could effectively use in their classrooms (Bybee et al., 2006 ; Trowbridge & Bybee, 1996 ). The cycle was based on a model of learning understood as a conceptual change and, therefore, on the constructivist theory of learning (Mayer, 2009 ). With this in mind, one could easily assume that several cognitive principles of learning had to underlie the design of the model. Indeed, some of these principles had already been identified by cognitive scientists by the time the 5E Model was designed, such as the levels of processing effect or the fact that memory works by connecting pieces of information related by meaning. In contrast, other principles were derived from later research or were at least supported by ample evidence found later, such as the role of retrieval in learning. These later principles also help us account for the model’s effectiveness.
Nevertheless, it should be highlighted that the learning principles that lay the foundations of the 5E Model are not only present in a collection of isolated actions that support learning, but also in the way they are sequenced. A quote from How People Learn (Bransford et al., 2000 ) seems especially germane to summarizing this point:
An alternative to simply progressing through a series of exercises that derive from a scope and sequence chart is to expose students to the major patterns of a subject domain as they arise naturally in problem situations. Activities can be structured so that students are able to explore, explain, extend, and evaluate their progress. Ideas are best introduced when students see a need or a reason for their use—this helps them see relevant uses of the knowledge to make sense of what they are learning. (p. 127)
Here is a research-based recommendation for a structure and sequence of instruction that exposes students to problem situations (i.e., engages student thinking) and then provides opportunities to explore, explain, extend, and evaluate their learning. This research summary from the NRC report supports the sequence of the 5E Instructional Model, and it even uses very similar terms.
Overall, one could argue that the 5E Model is effective because students are provided with time, prompts, and several opportunities to deeply engage with the learning object in a way that promotes connections between what is known and what is meant to be learned.
Availability of data and materials
Not applicable.
Alvermann, D. E., Smith, L. C., & Readence, J. E. (1985). Prior knowledge and the comprehension of compatible and incompatible text. Reading Research Quarterly, 20 , 420–436.
Google Scholar
Ambrose, S. A., Bridges, M. W., DiPietro, M., Lovett, M. C., & Norman, M. (2010). How learning works: seven research-based principles for smart teaching . Jossey-Bass.
Antony, J. W., Ferreira, C. S., Norman, K. A., & Wimber, M. (2017). Retrieval as a fast route to memory consolidation. Trends in Cognitive Sciences, 21 (8), 573–576.
Ausubel, D. P. (1960). The use of advance organizers in the learning and retention of meaningful verbal material. Journal of Educational Psychology, 51 , 267–272.
Ausubel, D. P. (1978). In defense of advance organizers: a reply to the critics. Review of Educational Research, 48 , 251–257.
Ausubel, D. P. (2012). The acquisition and retention of knowledge: a cognitive view . Springer Science Business Media.
Barnett, S. M., & Ceci, S. J. (2002). When and where do we apply what we learn? A taxonomy for far transfer. Psychological Bulletin, 128 (4), 612.
Barron, K. E., & Hulleman, C. S. (2015). Expectancy-value-cost model of motivation. Psychology, 84 , 261–271.
Bjork, R. A. (1978). The updating of human memory. In G. H. Bower (Ed.), The psychology of learning and motivation (Vol. 12, pp. 235–259). Academic Press.
Bower, G. H., Clark, M. C., Lesgold, A. M., & Winzenz, D. (1969). Hierarchical retrieval schemes in recall of categorical word lists. Journal of Verbal Learning and Verbal Behavior, 8 , 323–343.
Bransford, J. D., Brown, A. L., & Cocking, R. R. (2000). How people learn (Vol. 11). National Academy Press.
Bransford, J. D., & Johnson, M. K. (1972). Contextual prerequisites for understanding: some investigations of comprehension and recall. Journal of Verbal Learning and Verbal Behavior, 11 , 717–726.
Bransford, J. D., Vye, N., Kinzer, C. K., & Risko, V. (1990). Teaching thinking and content knowledge: toward an integrated approach. In B. F. Jones & L. Idol (Eds.), Dimensions of thinking and cognitive instruction (Vol. 1, pp. 381–413). Lawrence Erlbaum Associates.
Brod, G., Werkle-Bergner, M., & Shing, Y. L. (2013). The influence of prior knowledge on memory: a developmental cognitive neuroscience perspective. Frontiers in Behavioral Neuroscience, 7 , 139.
Brown A. L., Campione J. C. (1994). Guided Discovery In A Community of Learners, in K. Mc Gilly (Ed.), Classroom lesson: integrating cognitive theory and classroom practice. (pp. 229–270). MIT Press.
Butler, A. C. (2010). Repeated testing produces superior transfer of learning relative to repeated studying. Journal of Experimental Psychology: Learning, Memory, and Cognition, 36 (5), 1118.
Bybee, R. W. (2015). The BSCS 5E instructional model: creating teachable moments . NSTA Press.
Bybee, R. W., Taylor, J. A., Gardner, A., Van Scotter, P., Powell, J. C., Westbrook, A., & Landes, N. (2006). The BSCS 5E instructional model: origins and effectiveness. Colorado Springs Co: BSCS, 5 , 88–98.
Cardak, O., Dikmenli, M., & Saritas, O. (2008). Effect of 5E instructional model in student success in primary school 6th year circulatory system topic. In Asia-Pacific Forum on Science Learning & Teaching (Vol. 9, No. 2).
Carr, S. C., & Thompson, B. (1996). The effects of prior knowledge and schema activation strategies on the inferential reading comprehension of children with and without learning disabilities. Learning Disability Quarterly, 19 (1), 48–61.
Chaxel, A.-S., & Russo, J. E. (2015). Cognitive consistency: cognitive and motivational perspectives. In E. A. Wilhelms & V. F. Reyna (Eds.), Neuroeconomics, judgment, and decision making (pp. 29–48). Psychology Press.
Conway, M. A. (2002). Levels of processing 30 years on: a special issue of memory . Psychology Press.
Craik, F. I. M., & Lockhart, R. S. (1972). Levels of processing: a framework for memory research. Journal of Verbal Learning and Verbal Behavior, 11 (6), 671–684.
Dooling, D. J., & Lachman, R. (1971). Effects of comprehension on retention of prose. Journal of Experimental Psychology, 88 , 216–222.
Dudai, Y. (2004). The neurobiology of consolidations, or, how stable is the engram? Annual Review of Psychology, 55 , 51–86.
Dudai, Y. (2012). The restless engram: consolidations never end. Annual Review of Neuroscience, 35 , 227–247.
Edelson, D. C. (2001). Learning-for-use: a framework for integrating content and process learning in the design of inquiry activities. Journal of Research in Science Teaching, 38 , 355–385.
Finnie, P. S., & Nader, K. (2012). The role of metaplasticity mechanisms in regulating memory destabilization and reconsolidation. Neuroscience & Biobehavioral Reviews, 36 (7), 1667–1707.
Gentner, D., Rattermann, M. J., & Forbus, K. D. (1993). The roles of similarity in transfer: separating retrievability from inferential soundness. Cognitive Psychology, 25 (4), 431–467.
Gick, M. L., & Holyoak, K. J. (1983). Schema induction and analogical transfer. Cognitive Psychology, 15 (1), 1–38.
Gormally, C., Brickman, P., Hallar, B., & Armstrong, N. (2009). Effects of inquiry-based learning on students’ science literacy skills and confidence. International Journal for the Scholarship of Teaching and Learning, 3 (2), 16.
Garcia-Grau, F., Valls, C., Piqué, N., & Ruiz-Martín, H. (2021). The long-term effects of introducing the 5E model of instruction on students’ conceptual learning. International Journal of Science Education, 43 (9), 1441–1458.
Hanfstingl, B., Arzenšek, A., Apschner, J., & Gölly, K. I. (2021). Assimilation and accommodation. European Psychologist . https://doi.org/10.1027/1016-9040/a000463
Article Google Scholar
Harmon-Jones, E., Harmon-Jones, C., & Levy, N. (2015). An action-based model of cognitive-dissonance processes. Current Directions in Psychological Science, 24 (3), 184–189.
Harrison, A. G., & Treagust, D. F. (2000). Learning about atoms, molecules, and chemical bonds: a case study of multiple model use in grade 11 chemistry. Science Education, 84 (3), 352–381.
Hattan, C., Singer, L. M., Loughlin, S., & Alexander, P. A. (2015). Prior knowledge activation in design and in practice. Literacy Research: Theory, Method, and Practice, 64 (1), 478–497.
Healy, A. F., Clawson, D. M., McNamara, D. S., Marmie, W. R., Schneider, V. I., Rickard, T. C., Crutcher, R. J., King, C., Ericsson, K. A., & Bourne, L. E., Jr. (1993). The long-term retention of knowledge and skills. In D. Medin (Ed.), The psychology of learning and motivation: advances in research and theory (Vol. 30, pp. 135–164). Academic Press.
Hewson, P. W. (1981). A conceptual change approach to learning science. European Journal of Science Education, 3 (4), 383–396.
Hmelo-Silver, C. E., Duncan, R. G., & Chinn, C. A. (2007). Scaffolding and achievement in problem-based and inquiry learning: a response to Kirschner, Sweller and Clark. Educational Psychologist, 42 (2), 99–107.
Hokkanen, S. L. (2011). Improving student achievement, interest and confidence in science through the implementation of the 5E learning cycle in the middle grades of an urban school. Retrieved from: https://scholarworks.montana.edu/xmlui/bitstream/handle/1/1487/HokkanenS0811.pdf?sequence=1 (accessed 4 December 2021).
Hupbach, A., Gomez, R., Hardt, O., & Nadel, L. (2007). Reconsolidation of episodic memories: a subtle reminder triggers integration of new information. Learning & Memory, 14 (1–2), 47–53.
James, W. (1890). The principles of psychology . Holt.
Johnson, D. W., & Johnson, R. T. (2009). An educational psychology success story: social interdependence theory and cooperative learning. Educational Researcher, 38 (5), 365–379.
Kang, S., Scharmann, L. C., & Noh, T. (2004). Reexamining the role of cognitive conflict in science concept learning. Research in Science Education, 34 (1), 71–96.
Karpicke, J. D. (2012). Retrieval-based learning: active retrieval promotes meaningful learning. Current Directions in Psychological Science, 21 (3), 157–163.
Karpicke, J. D., & Roediger, H. L., III. (2008). The critical importance of retrieval for learning. Science, 319 , 966–968.
Kilavuz, Y. (2005). The effects of 5E learning cycle model based on constructivist theory on tenth grade students’ understanding of acid-base concepts . Middle East Technical University, Turkey. Retrieved from: https://citeseerx.ist.psu.edu/viewdoc/download?doi=10.1.1.633.4609&rep=rep1&type=pdf . Accessed 4 Dec 2021.
Kimball, D. R., & Holyoak, K. J. (2000). Transfer and expertise. The Oxford handbook of memory , 109–122.
Kirschner, P. A., Sweller, J., & Clark, R. E. (2006). Why minimal guidance during instruction does not work: an analysis of the failure of constructivist, discovery, problem-based, experiential, and inquiry-based teaching. Educational Psychologist, 41 (2), 75–86.
Lee, J. L., Nader, K., & Schiller, D. (2017). An update on memory reconsolidation updating. Trends in Cognitive Sciences, 21 (7), 531–545.
Maehr, M. L., & Midgley, C. (1991). Enhancing student motivation: a school-wide approach. Educational Psychologist, 26 , 399–427.
Mayer, R. E. (2009). Constructivism as a theory of learning versus constructivism as a prescription for instruction. In Constructivist Instruction (pp. 196–212). Routledge.
McCombs, B. L. (1991). Motivation and lifelong learning. Educational Psychologist, 26 (2), 117–127.
McDaniel, M. A., Agarwal, P. K., Huelser, B. J., McDermott, K. B., & Roediger, H. L., III. (2011). Test-enhanced learning in a middle school science classroom: the effects of quiz frequency and placement. Journal of Educational Psychology, 103 (2), 399.
McDaniel, M. A., Thomas, R. C., Agarwal, P. K., McDermott, K. B., & Roediger, H. L. (2013). Quizzing in middle-school science: successful transfer performance on classroom exams. Applied Cognitive Psychology, 27 (3), 360–372.
Newstead, S. E., Evans, J. St. B. T., eds. (1995). Perspectives on thinking and reasoning . Erlbaum.
Pan, S. C., & Rickard, T. C. (2018). Transfer of test-enhanced learning: meta-analytic review and synthesis. Psychological Bulletin, 144 (7), 710.
Peeck, J., Van Den Bosch, A. B., & Kruepeling, W. (1982). The effect of mobilizing prior knowledge on learning from text. Journal of Educational Psychology, 74 , 771–777.
Pintrich, P. R., Marx, R. W., & Boyle, R. A. (1993). Beyond cold conceptual change: the role of motivational beliefs and classroom contextual factors in the process of conceptual change. Review of Educational Research, 63 (2), 167–199.
Posner, G. J., Strike, K. A., Hewson, P. W., & Gertzog, W. A. (1982). Accommodation of a scientific conception: toward a theory of conceptual change. Science Education, 66 (2), 211–227.
Quintana, C., Reiser, B. J., Davis, E. A., Krajcik, J., Fretz, E., Duncan, R. G., et al. (2004). A scaffolding design framework for software to support science inquiry. Journal of the Learning Sciences, 13 , 337–386.
Roediger, H. L., III., & Butler, A. C. (2011). The critical role of retrieval practice in long-term retention. Trends in Cognitive Sciences, 15 (1), 20–27.
Roediger, H. L., III., Putnam, A. L., & Smith, M. A. (2011). Ten benefits of testing and their applications to educational practice. Psychology of Learning and Motivation, 55 , 1–36.
Rowland, C. A. (2014). The effect of testing versus restudy on retention: a meta-analytic review of the testing effect. Psychological Bulletin, 140 (6), 1432.
Schwartz, D. L., & Bransford, J. D. (1998). A time for telling. Cognition and Instruction, 16 (4), 475–5223.
Shing, Y. L., & Brod, G. (2016). Effects of prior knowledge on memory: implications for education. Mind, Brain, and Education, 10 (3), 153–161.
Sinatra, G. M., & Pintrich, P. R. (Eds.). (2003). Intentional conceptual change . Lawrence Erlbaum Associates.
Slavin, R. E. (2013). Cooperative learning and achievement: theory and research. In W. M. Reynolds, G. E. Miller, & I. B. Weiner (Eds.), Handbook of psychology (2nd ed., Vol. 7, pp. 199–212). Wiley.
Sweller, J. (2011). Cognitive load theory. In Psychology of learning and motivation (Vol. 55, pp. 37–76). Academic Press.
Thorndike, E. L., & Woodworth, R. S. (1901a). The influence of improvement in one mental function upon the efficiency of other functions: I. Psychological Review, 8 , 247–261.
Thorndike, E. L., & Woodworth, R. S. (1901b). The influence of improvement in one mental function upon the efficiency of other functions: II. The Estimation of Magnitudes. Psychological Review, 8 , 384–395.
Thorndike, E. L., & Woodworth, R. S. (1901c). The influence of improvement in one mental function upon the efficiency of other functions: III. Functions involving attention, observation, and discrimination. Psychological Review, 8 , 553–564.
Trowbridge L. W., & Bybee R. W. (1996). Models for effective science teaching. In: Teaching secondary school science: strategies for developing scientific literacy , Merrill, an Imprint of Prentice Hall, 213–221.
van Loon, M. H., de Bruin, A. B., van Gog, T., & van Merriënboer, J. J. (2013). Activation of inaccurate prior knowledge affects primary-school students’ metacognitive judgments and calibration. Learning and Instruction, 24 , 15–25.
Vygotsky, L. S. (1978). Mind in society . Harvard University Press.
Ware, S. G., & Young, R. M. (2010). Modeling narrative conflict to generate interesting stories. In Sixth Artificial Intelligence and Interactive Digital Entertainment Conference . Retrieved from: https://www.aaai.org/ocs/index.php/AIIDE/AIIDE10/paper/view/2136/2569 . Accessed 4 Dec 2021.
Wenzel, K., & Reinhard, M. A. (2021). Learning with a double-edged sword? beneficial and detrimental effects of learning tests—taking a first look at linkages among tests, later learning outcomes, stress perceptions, and intelligence. Frontiers in Psychology , 12.
Wetzels, S. A., Kester, L., & Van Merriënboer, J. J. (2011). Adapting prior knowledge activation: mobilisation, perspective taking, and learners’ prior knowledge. Computers in Human Behavior, 27 (1), 16–21.
Wigfield, A., & Eccles, J. (2000). Expectancy-value theory of achievement motivation. Contemporary Educational Psychology, 25 , 68–81.
Wiliam, D. (2011). Embedded formative assessment . Solution Tree Press.
Willingham, D. T. (2004). Ask the cognitive scientist. The privileged status of story. American Educator, 28 , 43–45.
Woloshyn, V., Paivio, A., & Pressley, M. (1994). Use of elaborative interrogation to help students acquire information consistent with prior knowledge and information inconsistent with prior knowledge. Journal of Educational Psychology, 86 , 79–89.
Yang, C., Luo, L., Vadillo, M. A., Yu, R., & Shanks, D. R. (2021). Testing (quizzing) boosts classroom learning: a systematic and meta-analytic review. Psychological Bulletin, 147 (4), 399.
Youniss, J., & Damon, W. (1992). Social construction in Piaget’s theory. In H. Beilin & P. Pufall (Eds.), Piaget’s theory: prospects and possibilities (pp. 267–286). Erlbaum.
Download references
Acknowledgements
This work received no specific Grant from any funding agency in the public, commercial, or not-for-profit sectors.
Author information
Authors and affiliations.
International Science Teaching Foundation, Brighton, UK
Héctor Ruiz-Martín
Golden, CO, USA
Rodger W. Bybee
You can also search for this author in PubMed Google Scholar
Contributions
Both authors read and approved the final manuscript.
Corresponding author
Correspondence to Héctor Ruiz-Martín .

Ethics declarations
Competing interests.
Héctor Ruiz-Martín is an independent researcher affiliated with the International Science Teaching Foundation (Brighton, UK), a nonprofit organization that develops research-informed resources for science teaching and learning. Some of these resources are based on the 5E Model of Instruction. Rodger W. Bybee led the team at the Biological Science Curriculum Study (BSCS) that designed and created the 5E Model of Instruction in 1987. The BSCS (Boulder, CO, USA) is an independent nonprofit organization offering science education research and leadership programs, and curriculum across the US.
Additional information
Publisher's note.
Springer Nature remains neutral with regard to jurisdictional claims in published maps and institutional affiliations.
Rights and permissions
Open Access This article is licensed under a Creative Commons Attribution 4.0 International License, which permits use, sharing, adaptation, distribution and reproduction in any medium or format, as long as you give appropriate credit to the original author(s) and the source, provide a link to the Creative Commons licence, and indicate if changes were made. The images or other third party material in this article are included in the article's Creative Commons licence, unless indicated otherwise in a credit line to the material. If material is not included in the article's Creative Commons licence and your intended use is not permitted by statutory regulation or exceeds the permitted use, you will need to obtain permission directly from the copyright holder. To view a copy of this licence, visit http://creativecommons.org/licenses/by/4.0/ .
Reprints and permissions
About this article
Cite this article.
Ruiz-Martín, H., Bybee, R.W. The cognitive principles of learning underlying the 5E Model of Instruction. IJ STEM Ed 9 , 21 (2022). https://doi.org/10.1186/s40594-022-00337-z
Download citation
Received : 29 December 2021
Accepted : 16 February 2022
Published : 03 March 2022
DOI : https://doi.org/10.1186/s40594-022-00337-z
Share this article
Anyone you share the following link with will be able to read this content:
Sorry, a shareable link is not currently available for this article.
Provided by the Springer Nature SharedIt content-sharing initiative
- Cognitive sciences
- Constructivism
- Conceptual change
- Learning theory
- Learning sequence

- SUGGESTED TOPICS
- The Magazine
- Newsletters
- Managing Yourself
- Managing Teams
- Work-life Balance
- The Big Idea
- Data & Visuals
- Reading Lists
- Case Selections
- HBR Learning
- Topic Feeds
- Account Settings
- Email Preferences
3 Steps to Cultivate an Innovator’s Mindset
- Soren Kaplan

Embracing curiosity, engaging in continuous learning, and fostering collaboration can help fast-track your career.
If you want to move up the corporate ladder, you need to do more than meet deadlines and produce strong results. Employees who quickly move up often have an innovator’s mindset. These people ultimately position themselves as valuable assets by questioning assumptions and pushing their organizations to stay competitive. By consistently bringing fresh ideas to the table and demonstrating a proactive approach to problem-solving, they naturally attract the attention of higher-ups. Anyone can build an innovator’s mindset by embracing curiosity, engaging in continuous learning, and fostering collaboration among their team members.
Imagine you’re playing a video game where you must overcome challenges and unlock rewards in order to move up to the next level. Climbing the corporate ladder at work is similar, but instead of battling dragons or building cities, you’re navigating office politics and aiming to deliver on goals. You’re also not going at it alone. Your peers are often competing alongside you for the next promotion.
- Soren Kaplan is the author of Experiential Intelligence , founder of InnovationPoint, and an affiliate at the Center for Effective Organizations at USC’s Marshall School of Business.
Partner Center
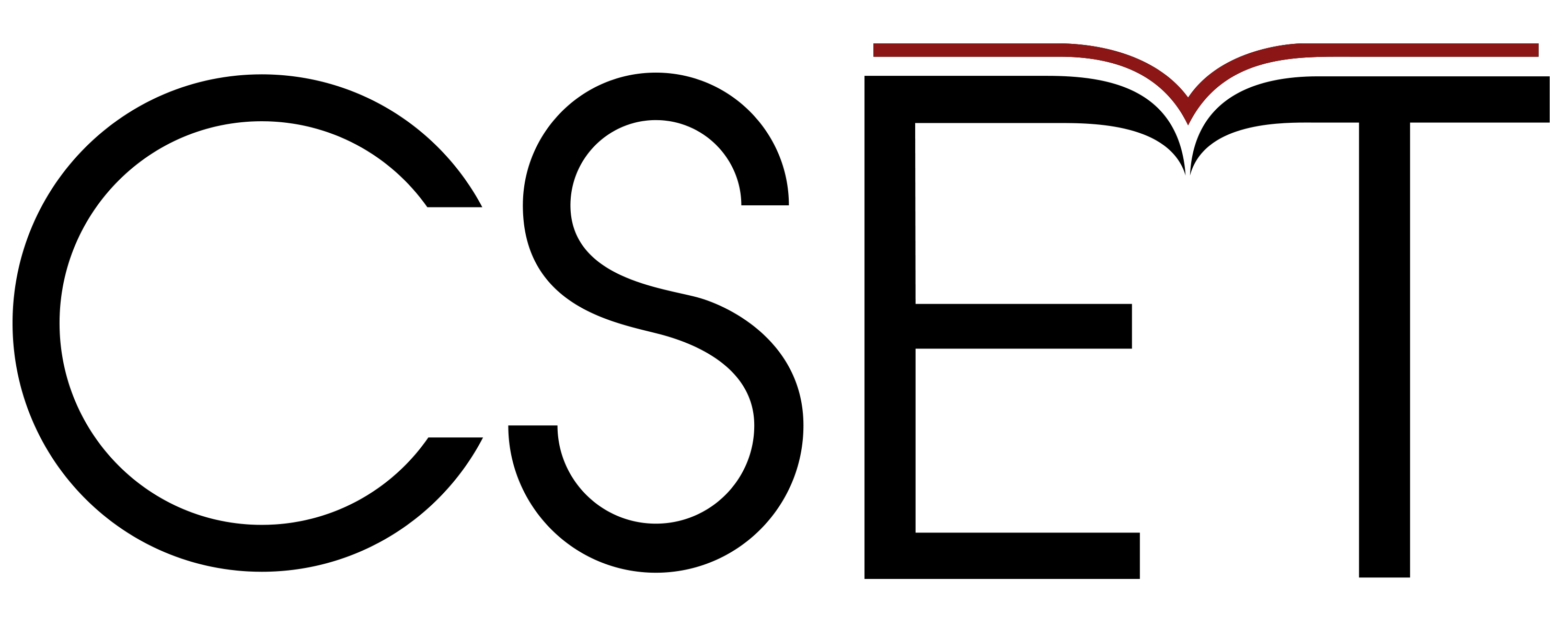
Donate Subscribe to our newsletter
Search form
Problem-solving cycle and teacher leadership preparation.
The Problem-Solving Cycle model and the Teacher Leadership Preparation model are part of a research-practice partnership to increase district capacity.

Introduction
All across the United States, mathematics teachers are being asked to implement new curriculum, interact with their students in different ways, and have a much deeper knowledge of mathematics content than ever before. The key is to professionalize the field of math teaching. We posit that teachers should be treated as professionals and, as such, have access to sustainable, long-term opportunities to expand and share their knowledge.
The Problem-Solving Cycle (PSC) model presents one such opportunity. When teachers participate in this highly adaptable model, they become part of a professional learning community. This community engages in mathematical problem solving, examines video of classroom teaching, and shares ideas about teaching and learning mathematics. By electing to take part in the PSC, teachers demonstrate their commitment to broadening their knowledge base and, consequently, to becoming better able to support their students’ learning of mathematics.
"After 20 years of teaching, it (PSC) has given me fresh eyes, like I’m a brand new teacher. The lens of the questions that I want to ask of my students and how they learn and why they’re doing things the way they do--I wasn't thinking that way. Maybe once in a while, but now it’s almost a constant lens. It’s due to these amazing workshops and the ability to practice this stuff with our peers in a safe environment."
--PSC Teacher Leader
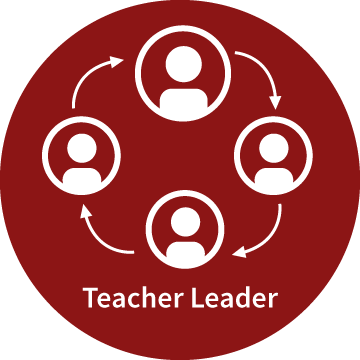
Teacher Leader
Find out what it means to be a Teacher Leader.
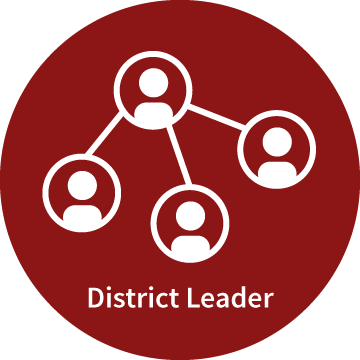
District Leader
Learn more about District Leadership in the Problem-Solving Cycle.
The Problem-Solving Cycle Facilitator's Guide explains each component of the cycle in more depth with examples, suggestions, and rationale. This guide is currently being finalized and will be made available shortly.
Principal Investigators and Leadership
Hilda Borko, PhD
Janet Carlson, PhD
Rebecca Deutscher, PhD
SFUSD Leaders

This work is supported by the National Science Foundation under Grant No DRL 1417261. Any opinions, findings, and conclusions or recommendations expressed in this material are those of the author(s) and do not necessarily reflect the views of the NSF.

- Stanford Home
- Maps & Directions
- Search Stanford
- Emergency Info
- Terms of Use
- Non-Discrimination
- Accessibility
© Stanford University , Stanford , California 94305 .
Ready or not? Investigating in-service teachers’ integration of learning analytics dashboard for assessing students’ collaborative problem solving in K–12 classrooms
- Open access
- Published: 10 July 2024
Cite this article
You have full access to this open access article
- Yiming Liu ORCID: orcid.org/0000-0003-2604-7993 1 ,
- Xiao Hu 1 ,
- Jeremy Tzi Dong Ng 1 ,
- Zhengyang Ma 2 &
- Xiaoyan Lai 3
Collaborative problem solving (CPS) has emerged as a crucial 21st century competence that benefits students’ studies, future careers, and general well-being, prevailing across disciplines and learning approaches. Given the complex and dynamic nature of CPS, teacher-facing learning analytics dashboards (LADs) have increasingly been adopted to support teachers’ CPS assessments by analysing and visualising various dimensions of students’ CPS. However, there is limited research investigating K-12 teachers’ integration of LADs for CPS assessments in authentic classrooms. In this study, a LAD was implemented to assist K-12 teachers in assessing students’ CPS skills in an educational game. Based on the person-environment fit theory, this study aimed to (1) examine the extent to which teachers’ environmental and personal factors influence LAD usage intention and behaviour and (2) identify personal factors mediating the relationships between environmental factors and LAD usage intention and behaviour. Survey data of 300 in-service teachers from ten Chinese K-12 schools were collected and analysed using partial least squares structural equation modelling (PLS-SEM). Results indicated that our proposed model showed strong in-sample explanatory power and out-of-sample predictive capability. Additionally, subjective norms affected technological pedagogical content knowledge (TPACK) and self-efficacy, while school support affected technostress and self-efficacy. Moreover, subjective norms, technostress, and self-efficacy predicted behavioural intention, while school support, TPACK, and behavioural intention predicted actual behaviour. As for mediation effects, school support indirectly affected behavioural intention through self-efficacy, while subjective norms indirectly affected behavioural intention through self-efficacy and affected actual behaviour through TPACK. This study makes theoretical, methodological, and practical contributions to technology integration in general and LAD implementation in particular.
Avoid common mistakes on your manuscript.
1 Introduction
Collaborative problem solving (CPS) is a socio-cognitive process in which group members utilise their shared knowledge, experiences, and skills, and navigate through a series of steps to reach a mutually agreed-upon solution to a particular problem (Fiore et al., 2017 ; Graesser et al., 2018 ; Griffin & Care, 2015 ; OECD, 2017a ). Scholars and educators recognise CPS as a critical competence for the younger generation in the 21st century (Cukurova et al., 2018 ; Fiore et al., 2018 ). As a domain-general competence (Graesser et al., 2017 ; Greiff et al., 2014 ), CPS skills are essential in various active learning approaches (e.g., project-based learning, inquiry-based learning) (Song, 2018 ; Saleh et al., 2022 ) and prevail in diverse disciplines (e.g., language, mathematics, and computer science) (see review by Baucal et al., 2023 and Tian & Zheng, 2023 ). More educational and governmental initiatives worldwide, including the Programme for International Student Assessment (PISA), Assessment and Teaching of 21st Century Skills (ATC21S), and Educational Testing Service (ETS), have increasingly emphasised the importance of students mastering CPS competence (von Davier & Halpin, 2013 ; Griffin & Care, 2015 ; OECD, 2017a ). Students competent in CPS are likely to not only excel academically but also become better equipped to effectively address communication issues and navigate interpersonal conflicts in future teamwork scenarios (Fiore et al., 2018 ; OECD, 2017b ; Sun et al., 2022 ). To achieve successful CPS, students are required to engage both effectively and collectively in the processes of identifying and representing problems, planning, execution, and monitoring (Hesse et al., 2015 ; OECD, 2017a ). Cognitive and social skills play pivotal roles during CPS activities, enabling teams to coordinate and communicate effectively and pool individual knowledge, experiences, and skills, thereby arriving at better solutions more efficiently than when individuals work alone (Andrews-Todd & Forsyth, 2020 ; Care et al., 2016 ; Hesse et al., 2015 ). However, given the interactive, interdependent, and temporal nature of CPS (Swiecki et al., 2020 ), it is challenging for teachers to assess and support students’ CPS skills and performance at both individual and group levels during their actual instruction (Scoular & Care, 2018 ), particularly in classroom settings (Care & Kim, 2018 ; Martinez-Maldonado, 2019 ).
In recent years, well-designed games have gained recognition as suitable vehicles for assessing and fostering students’ higher-order skills, including CPS (see review by Qian & Clark, 2016 and Gomez et al., 2022 ). As an active learning approach, digital game-based learning creates an immersive and playful environment, attracting students’ attention and promoting their engagement in learning tasks, thereby facilitating them to stay focused on the learning objectives (Hsu et al., 2021 ; Kaimara et al., 2021 ; Stieler-Hunt & Jones, 2017 ). More importantly, as students interact with games and teammates, they generate a substantial amount of multimodal gameplay data, such as clickstreams and conversations (Sun et al., 2022 ; see review by Tlili et al., 2021a ). Such multimodal gameplay data can be captured, analysed, and visualised through learning analytics dashboards (LADs), providing teachers with meaningful and actionable information about students’ demonstration of higher-order skills and learning attainments (Chen et al., 2022 ; Lee-Cultura et al., 2023 ; Ruipérez-Valiente et al., 2021 ; Tlili et al., 2021b ). LADs, harnessing the power of both learning analytics and visual analytics (Sun & Liu 2022 ), can illustrate various metrics that reflect different aspects of students’ CPS. Not only do they display students’ individual skills and contributions to collaborative learning along with their changes over time (Hu et al., 2022 ), but they also illuminate team performance and group dynamics during the collaborative learning processes (Liu et al., 2024 ; Bao et al., 2021 ; Zheng et al., 2021 ).
In CPS contexts, teachers play a guiding role in facilitating students’ collaboration and problem solving (Griffin, 2017 ). With the assistance of LADs, teachers can monitor students’ CPS progression and evaluate individual contributions and team performance more accurately (Liu et al., 2024 ; Martinez-Maldonado, 2019 ). This enables teachers to identify students who are struggling with CPS tasks or ‘gaming the system’ in a timely manner and provide actionable feedback as well as adaptive and personalised support (Chen et al., 2021 ; Huang et al., 2023 ). Teacher-facing LADs are widely regarded as effective tools that can aid teachers in facilitating students’ CPS practices (Kaliisa & Dolonen, 2023 ; Van Leeuwen et al., 2019 ). However, teachers’ sensemaking of the LADs relies on how they associate dashboard information with their own pedagogical decisions and actions (Van Leeuwen, 2019 ), and multiple factors can influence this sensemaking process. For example, existing studies (e.g., Zheng et al., 2021 ) have found that teachers often struggle with complex visualisations in LADs. Some have reported the misalignment between visual representations of LADs and teachers’ diagnoses (Li et al., 2022 ), whereas others have demonstrated that teachers might find it difficult to use LADs, suggesting the necessity of providing professional training and technological support for those working with LADs (Rienties et al., 2018 ).
Despite the substantial findings in LAD research, there exists a critical gap between the potential role of LADs designed for CPS assessments and their actual usage by teachers. According to person-environment fit theory (P-E fit theory), the degree of compatibility between a person’s individual characteristics and external environments can shape the person’s actual behaviour (Kristof-Brown et al., 2005 ; Kristof-Brown & Guay, 2011 ). Accordingly, this study posits that both teachers’ personal and environmental factors play critical roles in determining their usage behaviour of LADs for CPS assessments. Moreover, through reviewing previous literature on the P-E fit theory (e.g., Al-Fudail & Mellar, 2008 ; Chou & Chou, 2021 ; Dong et al., 2020 ; Govender & Mpungose, 2022 ) and determinants of teacher-facing LAD adoption (e.g., Kaliisa et al., 2021 ; Rienties et al., 2018 ; Van Leeuwen et al., 2021 ), this study identified several specific personal and environmental factors that may affect teachers’ LAD usage and constructed an integrated conceptual model (see Fig. 1 ). Specifically, teachers’ personal attributes include technological pedagogical content knowledge (TPACK), technostress, and self-efficacy, while the environmental factors include subjective norms and school support. In light of the potential of LAD for supporting teachers’ CPS assessments and the possible impacts of these factors on their LAD usage, this study aims to examine how these personal and environmental factors and their relationships shape the LAD usage intention and behaviour of K-12 in-service teachers in the classroom context of game-based CPS assessments.
Our paper is structured as follows: first, we define collaborative problem-solving, describe its prevalence in education across learning approaches and contexts, and introduce how educational games can develop and assess learners’ CPS competence. Then, we illustrate the potential of teacher-facing learning analytics dashboards (LADs) for supporting teachers’ assessment of learners’ CPS skills and problematize the gap between their potential and teachers’ actual usage. Next, we present our literature review on the affordances of teacher-facing LADs for facilitating computer-based collaborative learning and the person-environment fit theory, encompassing various environmental and personal factors influencing teachers’ technology integration. Then, we articulate our research aims, conceptual model and hypotheses, and our methodology for examining our model hypotheses and addressing our research objective. Next, we present the results of our analysis, including the structural and mediation effects of the environmental and personal factors on teachers’ LAD usage intention and behaviour. Finally, we discuss these results and make concluding remarks regarding the contributions of this study, its limitations, and our recommendations for future research.
2 Literature review
2.1 teacher-facing lads for computer-based collaborative learning.
Teacher-facing LADs function as a supportive tool, enabling teachers to assess and intervene in students’ learning processes effectively and efficiently based on timely visualizations (Van Leeuwen et al., 2019 ). These LADs provide fine-grained insights that empower teachers to understand, rationalise, and make informed decisions based on the complex data derived from student online learning trajectories and activities (Calvo-Morata et al., 2019 ; Li et al., 2022 ). Research has underscored the benefits of integrating LADs into computer-based collaborative learning. For instance, a study by Van Leeuwen et al. ( 2019 ) unveiled a teacher-centric LAD aimed at tracking students’ collaborative endeavours and providing indirect support within the classroom setting. The dashboard offers teachers an overview of students’ collaborative learning situations derived from computational analytics, assisting them in detecting students’ problematic learning situations. Kaliisa and Dolonen ( 2023 ) introduced university instructors to a LAD designed for online problem-oriented discussions. This dashboard, equipped with visualisations of team interaction and automated discourse analysis of students’ discussion content, facilitated teachers in interpreting students’ learning dynamics within collaborative discussions. While LADs were deemed beneficial for teachers to gain a deeper understanding of student learning and interactions within computer-supported collaborative learning environments, teachers might encounter multiple challenges when attempting to utilise LADs in their teaching (Zheng et al., 2021 ). For instance, Liu et al. ( 2023 ) found that teachers’ resistance to embracing LADs might result from a deficit in supportive knowledge and skills, such as data visualisation literacy, and possible stress and anxiety induced by technology adoption. Li et al. ( 2022 ) revealed that teachers viewed the complexity of visualisations from LADs and the inadequacy of LAD capabilities as barriers to interpreting their information. Thus, the identification of these challenges and the implementation of corresponding solutions are crucial in enhancing teachers’ capacity to integrate LADs into teaching practices.
2.2 Person-environment fit theory
Built on various theories and models of technology integration, previous studies have identified numerous factors that may facilitate or impede teachers’ effective use of LADs (e.g., Liu et al., 2023 , 2024 ; Li et al., 2022 ). Certain factors are tied to teachers’ personal attributes, encompassing their knowledge, skills, and literacy in relation to LADs, as well as psychological aspects like self-efficacy beliefs. On the other hand, some factors derive from external environments around teachers, such as the availability of school support or broader social conditions. To better conceptualise and situate these factors, we employ the P-E fit theory as the theoretical ground for this study. The P-E fit theory refers to the compatibility that arises when people and their environments are well matched (Kristof-Brown et al., 2005 ). It stresses that compatibility between individual and work environment characteristics influences behaviour and psychological functions, with a higher congruity associated with improved performance and increased productivity (Edwards et al., 1998 ; Kristof-Brown & Guay, 2011 ). In this study, the P-E fit theory offers a lens to investigate how individual and environmental factors influence teachers’ use of LADs for assessing and supporting students’ CPS skills. Past research has typically leveraged technology acceptance models (TAMs) to delve into various psychological drivers of technology adoption among teachers (Scherer et al., 2019 ). While both TAM and P-E fit theories underscore influential factors related to technology integration, P-E fit theory offers added value by clustering these factors and further clarifying the relationships between these clusters. In the following sections, we will elaborate on the personal and environmental factors influencing teachers’ technology integration into teaching practices.
2.3 Environmental factors: subjective norms and school support
Ajzen and Fishbein ( 1980 ) defined subjective norms (SN) as one’s normative belief that ‘specific individuals or groups think he should or should not perform the behaviour and his motivation to comply with the specific referents’ (p. 8). A systematic review conducted by Wijnen et al. ( 2021 ) highlighted the pivotal role of subjective norms in shaping teachers’ acceptance and adoption of technologies designed to foster primary school students’ higher-order thinking skills. Jeong and Kim ( 2017 ) found that teachers with a stronger sense of subjective norms were more inclined to utilise information and communication technologies (ICT) for instructional purposes in early childhood education. Shin’s ( 2015 ) study disclosed that a substantial proportion of elementary school teachers perceived the attitudes of administrators towards technology use as a vital determinant in achieving high-quality technology integration.
School support (SS) refers to material and psychological support provided by school administrators for teaching-related technology use (Chou & Chou, 2021 ). Empirical research has demonstrated that school support can facilitate teachers’ utilisation of technology (Atman Uslu & Usluel, 2019 ; Hew et al., 2017 ; Porter & Graham, 2016 ). For instance, Lam et al. ( 2010 ) discovered that secondary school teachers exhibited greater motivation and willingness to adopt technological innovations when they perceived their schools as being more supportive of their competence and autonomy. Koh et al. ( 2017 ) revealed that the primary school teachers’ integration of technology was fostered by peer support in teacher professional development activities organised by the schools. Regarding pre-service teachers, their intent to use technology was positively influenced by a range of facilitative conditions, such as the availability of infrastructure, technical assistance, and encouraging policies (Kaimara et al., 2021 ).
2.4 Personal factors: TPACK, self-efficacy, and technostress
Teachers’ technological, pedagogical, and content knowledge (TPACK) emphasises the affordances of using technologies to improve teaching practices (Archambault & Crippen, 2009 ; Schmidt et al., 2009 ). Well-developed TPACK is commonly associated with the successful integration of educational technologies in teaching and learning (Anthony et al., 2021 ; Koh et al., 2017 ), as TPACK not only informs teachers about what technologies to use but, more importantly, fosters teachers to think, analyse, and reflect on the use of technology (Huang et al., 2021a ). Consequently, TPACK is widely discussed when researchers evaluate teachers’ technology usage and integration. For instance, Schmid et al. ( 2021 ) revealed that pre-service teachers’ TPACK skills were associated with how they implemented technologies in lesson plans. Furthermore, previous research has emphasised the positive impact of TPACK on teachers’ attitudes, behavioural intentions, and actual behaviours towards technology integration among K-12 and higher education teachers (e.g., Hsu et al., 2021 ; Jung et al., 2019 ; Zhang & Chen, 2022 ). In other words, teachers with a high level of TPACK are more likely to form favourable perceptions of the value technology can add to teaching and learning. Conversely, the lack of TPACK often poses obstacles to successful technology integration (Liu et al., 2024 ).
Perceived self-efficacy (SE) was defined as ‘beliefs in one’s capabilities to organise and execute the courses of action required to produce given attainments’ (Bandura, 1997 , p. 3). Numerous studies have suggested that teachers’ self-efficacy positively influences their intentions to incorporate educational technologies into actual teaching (e.g., Panisoara et al., 2020 ; Wijnen et al., 2021 ). For instance, Joo et al. ( 2018 ) found that pre-service teachers’ perceived self-efficacy was positively related to their intention towards technology integration. The same positive relationship between self-efficacy and technology use can also be observed in K-12 educational contexts. Petko et al. ( 2018 ) reported that primary school teachers’ perceived technology-related beliefs strongly predicted their short-term and long-term technology use for teaching project-based learning. Similarly, Kwon et al. ( 2019 ) demonstrated that teachers’ self-efficacy for technology integration significantly affected their actual use of computing devices (e.g., smartphones and tablets) in secondary schools.
Technostress (TS), particularly in educational settings, has garnered significant research attention due to the pervasive infiltration of new technologies into classrooms spanning various subjects and educational levels (see review by Fernández-Batanero et al., 2021 ). Weil and Rosen ( 1997 ) defined technostress as ‘any negative impact on attitudes, thoughts, behaviors, or body psychology caused directly or indirectly by technology’ (p. 5). Ayyagari et al. ( 2011 ) suggested that a high level of technostress leads to users’ lower performance in their actual usage and diminished behavioural intention towards future adoption. Similar findings have likewise been noted within the realm of educational research (e.g., Chou & Chou, 2021 ; Joo et al., 2016 ). In particular, technostress could lead to K-12 teachers’ psychological frustration and an inability to cope with teaching tasks (Al-Fudail & Mellar, 2008 ). Similarly, a large-scale survey on the technology integration behaviour of K-12 teachers showed that teachers’ efforts to integrate technologies were hindered by technostress, which diminished satisfaction with educational technology usage and adversely affected their perceptions of using ICT for teaching (Wu et al., 2022 ).
2.5 The current study
With the complex and dynamic nature of CPS, there is a growing trend towards the development of teacher-facing LADs to analyse and visualise multimodal data related to students’ CPS skills (e.g., clickstreams, group conversations) collected from learning technologies, including educational games (Liu et al., 2024 ; Azevedo & Gašević, 2019 ; Chen et al., 2022 ; Tlili et al., 2021b ). Meanwhile, when attempting to adopt LADs for their teaching, teachers often encounter various barriers and challenges (Kaliisa et al., 2021 ; Lee-Cultura et al., 2023 ; Li et al., 2022 ), often due to the misfit between personal and environmental characteristics (Chou & Chou, 2021 ; Dong et al., 2020 ; Govender & Mpungose, 2022 ). The misfit in turn influences teachers’ technology usage intention and performance (Chou & Chou, 2021 ; Li & Wang, 2021 ; Joo et al., 2016 ). Consequently, to maximise the utilities of LADs and mitigate their stress from integrating LAD, it is necessary to identify personal and environmental stressors and predict teachers’ LAD usage from a person-environment fit perspective. Despite the substantial existing findings on the influence of personal and environmental factors on teacher’s technology acceptance and adoption, scant research has delved into how these factors affect K-12 teachers’ integration of LADs for CPS assessments in classroom settings. Therefore, in the current study, we implemented a teacher-facing LAD for assessing students’ CPS in K-12 classrooms, and examined the relationships between environmental and personal factors and how they shape K-12 teachers’ behavioural intention and actual usage of the LAD. The current study will extend our theoretical understanding of the P-E fit theory through its application in the context of technology-enhanced CPS assessment. Methodologically, although previous studies on P-E fit have proposed and validated diverse technology integration models, they focused on checking their models’ explanatory power (i.e., \({R}^{2}\) ) and offered limited evidence of the models’ predictive capability and external validity. Thus, whether our proposed model possesses strong out-of-sample predictive power warrants further investigation. Our research findings could shed light on the determinants that either facilitate or hinder teachers’ successful integration of LADs into teaching practices and offer recommendations on how to support teachers in leveraging LADs to foster students’ CPS skills.
2.6 Conceptual model and hypotheses
Based on the P-E fit theory and our literature review as discussed above, this study constructed an integrated conceptual model (see Fig. 1 ), where the hypothesised model relationships are as follows:
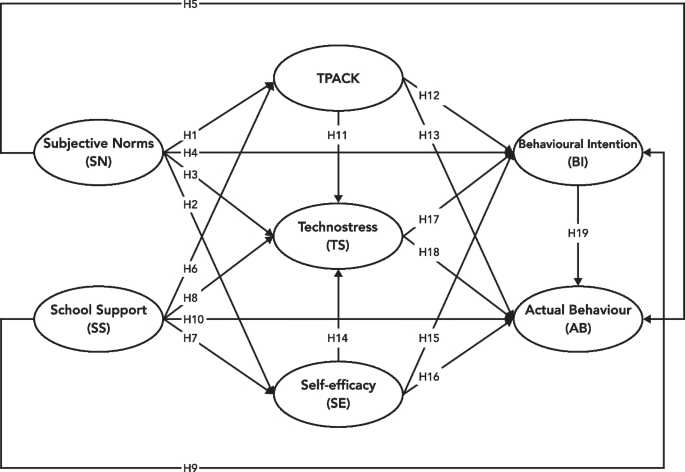
The integrated conceptual model
H 1 -H 5 : subjective norms (SN) affect TPACK, self-efficacy (SE), technostress (TS), behavioural intention (BI), and actual behaviour (AB) respectively.
H 6 -H 10 : school support (SS) affects TPACK, self-efficacy (SE), technostress (TS), behavioural intention (BI), and actual behaviour (AB) respectively.
H 11 -H 13 : TPACK affects technostress (TS), behavioural intention (BI), and actual behaviour (AB) respectively.
H 14 -H 16 : self-efficacy (SE) affects technostress (TS), behavioural intention (BI), and actual behaviour (AB) respectively.
H 17 -H 18 : technostress (TS) affects behavioural intention (BI) and actual behaviour (AB) respectively.
H 19 : behavioural intention (BI) affects actual behaviour (AB).
Additionally, we postulate that TPACK and self-efficacy indirectly affect behavioural intention and actual behaviour through technostress. Firstly, it was discovered that teachers’ TPACK abilities lessened their levels of technostress, which subsequently enhanced their intention to utilise educational technologies (Joo et al., 2016 ). Concerning the relationship between SE and technostress, in studies on K-12 and university teachers’ technostress towards online teaching tools, teachers’ self-efficacy reduced their technostress (Chou & Chou, 2021 ). Similarly, Dong et al. ( 2020 ) illustrated that self-efficacy mitigated technostress among K-12 in-service teachers during the integration of ICT into teaching activities. We therefore hypothesise:
H 20 -H 21 : technostress (TS) mediates the relations between TPACK and behavioural intention (BI) and actual behaviour (AB).
H 22 -H 23 : technostress (TS) mediates the relations between self-efficacy (SE) and behavioural intention (BI) and actual behaviour (AB).
We also propose that school support indirectly affects behavioural intention and actual behaviour through TPACK, self-efficacy, and technostress. School support has been identified as a crucial catalyst in motivating teachers to incorporate e-learning into their teaching practices (Atman Uslu & Usluel, 2019 ; Ifinedo & Kankaanranta, 2021 ; Liu et al., 2017 ). Both administrative and collegial support were found to positively influence teachers’ TPACK and computer self-efficacy within K-12 school settings (Dong et al., 2020 ). In the absence of sufficient school support, K-12 teachers are prone to ‘experience resistance and animosity from colleagues’, which could consequently undermine their self-efficacy when applying educational games to their teaching activities (Stieler-Hunt & Jones, 2017 ). Numerous studies also suggest that school support can help teachers alleviate their technostress, which in turn facilitates the integration of emerging technologies into teaching (e.g., Joo et al., 2016 ; Özgür, 2020 ; Chou & Chou, 2021 ). We therefore hypothesise:
H 24 -H 25 : TPACK mediates the relations between school support (SS) and behavioural intention (BI) and actual behaviour (AB).
H 26 -H 27 : self-efficacy (SE) mediates the relations between school support (SS) and behavioural intention (BI) and actual behaviour (AB).
H 28 -H 29 : technostress (TS) mediates the relations between school support (SS) and behavioural intention (BI) and actual behaviour (AB).
Subjective norms have been recognised as a key factor in shaping teachers’ technology usage intention and behaviour (Jeong & Kim, 2017 ; Shin, 2015 ; Wijnen et al., 2021 ). Nevertheless, there is a scarcity of knowledge concerning whether and how teachers’ personal factors mediate the influences of subjective norms on their usage intention and behaviour towards educational technologies. Previous studies (e.g., Kwon et al., 2019 ; Wu et al., 2022 ; Zhang & Chen, 2022 ) demonstrated the significant influences of teachers’ TPACK, self-efficacy, and technostress on their behavioural intention and actual usage towards digital learning technologies. At the same time, subjective norms were found to be highly associated with TPACK, self-efficacy, and technostress (Dong et al., 2020 ; Jang et al., 2021 ; Scherer et al., 2019 ). Hence, we expect that subjective norms have significant indirect effects on behavioural intention and actual behaviours through TPACK, self-efficacy, and technostress, respectively. We therefore hypothesise:
H 30 -H 31 : TPACK mediates the relations between subjective norms (SN) and behavioural intention (BI) and actual behaviour (AB).
H 32 -H 33 : self-efficacy (SE) mediates the relations between subjective norms (SN) and behavioural intention (BI) and actual behaviour (AB).
H 34 -H 35 : technostress (TS) mediates the relations between subjective norms (SN) and behavioural intention (BI) and actual behaviour (AB).
3.1 Overview of the CPS game and the LAD
For facilitating the assessment of young students’ CPS skills, Digital City Fighter ( D-City Fighter ) was developed as part of a larger theme-based research project titled Learning and Assessment for Digital Citizenship. D-City Fighter (see Fig. 2 ) is a mobile online role-playing game focused on CPS with a 3D interface supporting multiple players. Based on the principles of evidence-centred design, three CPS quests have been designed and developed in the game (Tsang et al., 2020 ; Liu et al., 2024 ). As Fig. 2 shows, one of the quests requires a group of four student-players to locate puzzle pieces scattered throughout the digital city and assemble them within a 15-minute timeframe. Specifically, players are tasked with identifying puzzle pieces whose frames correspond to the colour of the circle at their feet and positioning these pieces correctly in their respective locations within the puzzle area. A hidden clue for completing this puzzle task becomes available to players upon entering the bush. Various tools (i.e., Virtual Joystick, Pickup/Putdown, Emoji, Chat, Scoreboard, Map, and Timer) are incorporated into the graphical user interface to support players’ CPS processes. To assess the players’ CPS skills, measures from their gameplay data (e.g., movement trajectories, clickstreams) were mapped to CPS skills, referencing a well-known framework for teachable CPS skills proposed by Hesse et al. ( 2015 ): participation, perspective taking, task regulation, social regulation, and knowledge building.
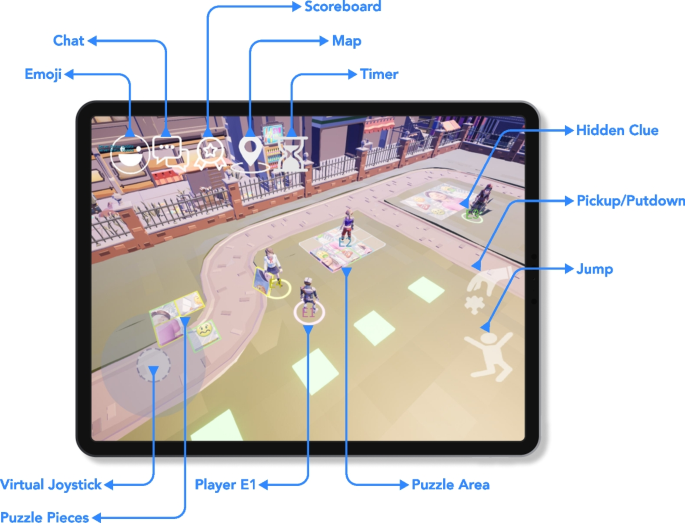
The interface of D-City Fighter (Payer E1’s point of view)
CPSLens (see Fig. 3 ) is a teacher-facing mobile LAD designed to analyse and visualise students’ interactions within virtual CPS environments, such as D-City Fighter . It aims to assist teachers in assessing students’ CPS skills and performance. The pipeline for game learning analytics of CPS is depicted in Fig. 4 . Specifically, student-players’ gameplay data are fed into CPSLens , which visualises their CPS skills and processes as well as quest performance and engagement to teachers. Teachers can then use the generated visualisations to evaluate students’ CPS skills and performance. In CPSLens , six visualisation panels are offered: Quest Performance , Player Movement , CPS Performance , Quest Playback , Quest Engagement , and CPS Dynamics . Moreover, CPSLens allows teachers to switch between the visualisation interfaces of different groups and group members.
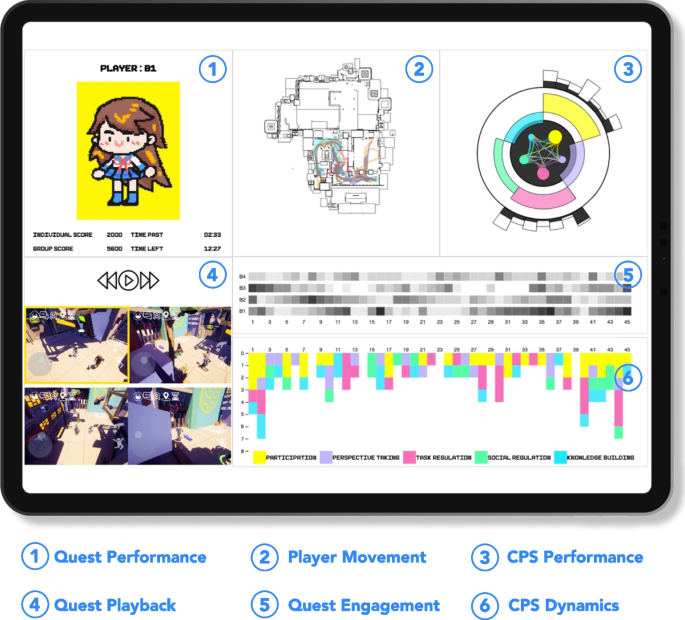
The interface of CPSLens (Group B and Player B1)
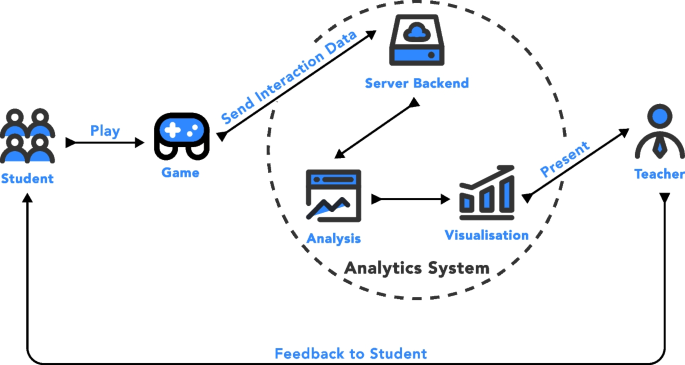
Game learning analytics pipeline adapted from Calvo-Morata et al. ( 2019 )
Students’ CPS skills and processes are visualised via the CPS Performance , CPS Dynamics , Player Movement , and Quest Playback panels. Through the CPS Performance panel, teachers can assess group members’ performance on five CPS skills identified in the literature (Hesse et al., 2015 ), represented by a circular bar chart. Teachers can also visually check the transition patterns among the CPS skills, depicted by nodes of varying sizes corresponding to skill performance levels. When clicking specific skill bars, such as participation (yellow) and task regulation (pink) (See Fig. 3 ), teachers will be presented with multiple black-and-white bars. Each of these black-and-white bars indicates the proportion of the selected skill performed by one player (black part) relative to the corresponding skill performance of the entire group at a given time point on the x-axis of the CPS Dynamics panel. The CPS Dynamics panel displays a stacked bar chart with coordinates; the x-axis represents time points (in minutes), and the y-axis signifies one group member’s performance scores on the CPS skills, providing teachers with insights into dynamic changes in individual CPS skills over time. The Player Movement panel visualises different group members’ movement trajectories within the digital city. The city’s geography is divided into quest zones containing quest-related elements (e.g., puzzle pieces) and non-quest zones without relevant problem-solving information. This feature enables teachers to track and monitor different group members’ task progress. For instance, teachers can leverage this visualisation panel to identify difficulties that group members encounter as well as unintended events (e.g., ‘gaming the system’, off-quest behaviours) in the CPS process by comparing their actual movement trajectories with the expected ones. Finally, the Quest Playback panel allows teachers to review the video recording of each group member’s CPS process individually.
The Quest Performance and Quest Engagement panels visualise the performance and engagement of the CPS quests, respectively. Teachers can use the Quest Performance panel to examine the quest scores at both individual and group levels, as well as check the remaining and expended time during group members engaging with the quests. The quest scores are computed using performance metrics derived from students’ gameplay data, such as the duration of quest completion and the count of incorrect attempts. Additionally, the Quest Engagement panel showcases the level of engagement of each group member, evaluated through the group member’s interactions with teammates, in-game support tools, quest elements, and both quest and non-quest zones. A higher level of engagement correlates with darker shades of the square colour. Quest Engagement is represented as coordinates, with each point on the x-axis marking a time point (in minutes) and each label (e.g., B1-B4 in Group B) on the y-axis representing a group member. Quest Engagement informs teachers about different group members’ temporal changes in engagement levels in the entire CPS process.
The use of CPSLens offers interactive, near real-time visualisations of students’ strengths or weaknesses regarding particular CPS skills. Such feedback can not only support teachers to foster students’ CPS skills in an adaptive and personalised way but also help teachers improve their design and implementation of collaborative learning activities, such as building an appropriate group composition referring to CPS assessment results from CPSLens .
3.2 Participants and procedure
A total of 300 in-service teachers from ten K-12 schools in China participated in this study. Among the participants, 59.3% were female, with an overall average age of 40.40 ( SD = 7.05). Table 1 shows their demographic details. None of the participating teachers had experience implementing LADs or educational games in their classrooms. Before data collection, informed consent was obtained from the participating teachers, principals, students, and their parents. The researchers emphasized to the participating teachers that they had the freedom to withdraw from the study at any time without any consequences.
Initially, the research team disseminated multimedia materials for the teachers to acquire knowledge about technology-enhanced CPS assessments, LADs, and their pedagogical affordances. Then, considering teachers’ lack of experience in implementing LADs, the researchers conducted a full-day onsite training session in each school to familiarise the teachers with the operations of D-City Fighter and CPSLens . This comprehensive training, guided by the researchers, included a live demonstration of D-City Fighter and CPSLens , followed by a hands-on trial allowing teachers to gain practical experience with these technology applications. Upon completion, the participating teachers were invited to integrate D-City Fighter and CPSLens into their teaching and implement them within classroom environments in the subsequent semester. Inherently functioning as a domain-general competence (Graesser et al., 2017 ; Greiff et al., 2014 ), CPS has been widely applied across various disciplines (e.g., mathematics, computer science) (Baucal et al., 2023 ; Tian & Zheng, 2023 ) and different active learning approaches (e.g., project-based learning, problem-based learning) (Song , 2018 ; Saleh et al., 2022 ). In this study, as shown in Table 1 , the participating teachers implemented the CPS game and the LAD in STEM (e.g., technology, mathematics) and non-STEM (e.g., language) subjects.
Throughout the implementation of D-City Fighter and CPSLens , researchers provided remote assistance via a social media group whenever participants encountered and reported technical difficulties. Excluding these sporadic cases of troubleshooting, all the participants received similar amounts of support from the researchers. One semester after the implementation (approximately four months), we distributed questionnaires to elicit teachers’ perceptions of constructs corresponding to environmental and personal factors (as detailed in the next section), as well as the behavioural intentions and actual use of CPSLens . Log files from CPSLens showed that the teachers, on average, accessed CPSLens 4.63 times, spending approximately 10.13 min during each interaction.
3.3 Measures
We measured subjective norms (SN), self-efficacy (SE), and behavioural intention (BI) using 12 items with a 7-point Likert scale (1 = strongly disagree ; 7 = strongly agree ). The items were adapted from Admiraal et al. ( 2017 ) and Teo and van Schaik ( 2009 ). Cronbach’s alpha for SN (five items), SE (four items), and BI (three items) ranged from 0.838 to 0.902.
Technological pedagogical content knowledge (TPACK), school support (SS), and technostress (TS) were measured using 11 items with the same 7-point Likert scale used above. The items were adapted from the highly cited literature (Archambault & Crippen, 2009 ; Ayyagari et al., 2011 ; Chou & Chou, 2021 ; Lam et al., 2010 ; Schmidt et al., 2009 ). Cronbach’s alpha for TPACK (four items), SS (four items), and TS (three items) ranged from 0.784 to 0.907.
We assessed actual behaviour (AB) using five items adapted from Davis et al. ( 1989 ), Schildkamp et al. ( 2017 ), and Siyam ( 2019 ). These five 7-point Likert-type items included the teachers’ frequency of utilising the LAD over the one-semester period (from 1 = not at all to 7 = more than 10 times ), their duration per LAD usage (from 1 = less than five minutes to 7 = more than half an hour ), and three other items measuring the extent to which the teachers used LAD for instructional purposes (from 1 = never to 7 = always ). Cronbach’s alpha for AB was 0.838.
3.4 Data Analysis
All data analyses were performed using R 4.3.2 and SmartPLS 4.0.7.8. For examining the relationships between personal and environmental factors and how they influenced teachers’ behavioural intention and actual usage of the LAD, we evaluated the proposed model (see Fig. 1 ) through partial least squares structural equation modelling (PLS-SEM). The PLS-SEM algorithm combines principal component analysis with ordinary least squares regressions to estimate model structures. Compared with covariance-based SEM commonly adopted in studying technology acceptance and adoption, PLS-SEM offers several advantages, including accommodating non-normal data distributions, achieving sufficient statistical power with small sample sizes, and managing complex models with multiple latent and observed variables and their interrelationships. PLS-SEM is particularly exceptional at assessing a model’s out-of-sample predictive power. These strengths align well with our study’s characteristics, such as the non-normality of our data (see item descriptive statistics in Appendix Table 4 ), complex model relationships within a relatively small sample size, and the requirement to assess the model’s predictive capacity. Despite this study’s small sample size, it still meets the minimum sample size as calculated by G*power 3.1.9.4 (Faul et al., 2009 ). With population effect size, power level, and significance level \(\alpha\) set to 0.15, 0.95, and 0.01 respectively, G*power suggests that 189 is the minimum sample size required for our model estimation.
Adopting a two-stage process as delineated by Anderson and Gerbing ( 1988 ), the PLS-SEM analysis comprised assessments of the measurement model and structural model. In the measurement model, we checked indicator reliability (indicator loading \(\ge\) 0.708 and statistically significant), internal consistency reliability (0.70 \(\le\) Cronbach’s alpha and composite reliability (CR) \(\le\) 0.95), convergent validity (average variance extracted (AVE) \(\ge\) 0.50), and discriminant validity (the heterotrait-monotrait ratio of correlations (HTMT) \(<\) 0.85; Square root of AVE for a construct higher than its correlation with other constructs). In the structural model, we examined the statistical significance of path coefficients, collinearity (variance inflation factor (VIF) \(<\) 3.3), and effect size of path coefficients and model explanatory power (i.e., \({R}^{2}\) ), ranging from weak (0 \(\le\) x \(\le\) 0.10), modest (0.10 \(<\) x \(\le\) 0.30), moderate (0.30 \(<\) x \(\le\) 0.50) to strong (x \(>\) 0.50). These assessment criteria are from Hair et al.’s ( 2021 ) guidelines for PLS-SEM model evaluation.
Following the recommended procedures in Shmueli et al. ( 2019 ), this study assessed the out-of-sample predictive power of the proposed model. Initially, a holdout sample-based procedure was executed involving three-fold cross-validation and 20 repetitions to derive training and holdout samples. Next, out-of-sample prediction metrics for the model indicators were computed and compared with the linear regression model (LM) benchmark. According to Shmueli et al. ( 2019 ), a PLS model’s Q predict 2 larger than zero and prediction errors (e.g., root-mean-squared error) lower than the LM benchmark indicate the sufficient predictive power of the model. Because Shmueli et al. ( 2019 ) emphasise that the assessment of a PLS model’s prediction performance should concentrate on its key endogenous constructs, our analysis primarily targeted the BI and AB constructs within the model. For mediation analyses, the multiple mediation effects were analysed with reference to the procedure in Zhao et al. ( 2010 ).
4.1 Assessing the measurement model
The results from the measurement model assessment can be found in Appendix Table 4 . All indicator loadings proved statistically significant, with all but two items (AB4 = 0.560; AB5 = 0.560) surpassing the threshold of 0.708. These two items were retained due to their role in measuring the frequency and duration of LAD use—crucial for the content validity of the measurement—and the fact that the CR and AVE of the construct (i.e., AB) exceeded recommended thresholds (Hair et al., 2021 ). The CR of all constructs ranged from 0.869 to 0.931, larger than the cut-off value of 0.70, and Cronbach’s alpha ranging from 0.784 to 0.907—considered ‘satisfactory to good’ according to Hair et al. ( 2021 ). Convergent validity was achieved, as denoted by AVE (ranging between 0.598 and 0.795) exceeding 0.50 (see Appendix Table 4 ). The discriminant validity of the measurement model was acceptable, with HTMT below 0.85 and the square root of each construct’s AVE greater than its correlations with other constructs within the model (see Table 2 ).
4.2 Assessing the structural model and mediation effects
Appendix Table 5 and Fig. 5 showcase the results of the hypothesis testing. The structural model did not have the problem of collinearity, as the VIF values ranged from 1.109 to 2.274, not exceeding the threshold of 3.3. TPACK (𝛽 = 0.596, CI [0.496; 0.681], strong effect size) and SE (𝛽 = 0.494, CI [0.389; 0.587], moderate effect size) were positively predicted by SN, supporting H 1 and H 2 . While SS was a positive predictor of SE (𝛽 = 0.270, CI [0.159; 0.370]), it occurred to be a negative predictor of TS (𝛽 = -0.291, CI [-0.413; -0.143]), with both paths bearing modest effect sizes and accepting H 7 and H 8 . SN (𝛽 = 0.346, CI [0.217; 0.473]) and SE (𝛽 = 0.366, CI [0.216; 0.499]) positively predicted BI respectively with moderate effect sizes, while TS (𝛽 = -0.093, CI [-0.187; -0.010]) negatively predicted BI with a weak effect size, confirming H 4 , H 15 , and H 17 . SS (𝛽 = 0.087, CI [0.011; 0.167]) and TPACK (𝛽 = 0.162, CI [0.056; 0.275]) were predictive of AB, showing a weak and modest effect size, respectively. Therefore, H 10 and H 13 were established. With a strong effect size, BI was found to be a predictor of AB (𝛽 = 0.524, CI [0.409; 0.636]), supporting H 19
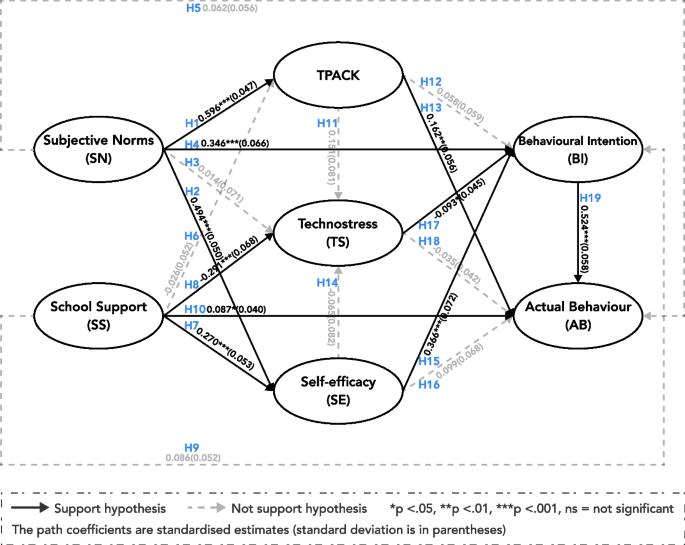
Results of structural model relationships
The model explained 54.5% of the variance for BI and 62.1% for AB, showing that our research model possessed a strong explanatory power (i.e., in-sample predictive power). According to the results of PLSpredict analysis (see Table 3 ), the Q predict 2 values for the BI and AB indicators exceeded zero, and the prediction errors in the PLS model were lower than the LM model, indicating that our model had high out-of-sample predictive power. Appendix Table 5 and Fig. 6 show the results of mediation analyses. TPACK (𝛽 = 0.097, CI [0.034; 0.167]) was a mediator of the relationship between SN and AB, supporting H 31 . SE mediated the relationships between SN and BI (𝛽 = 0.181, CI [0.101; 0.267]) and between SS and BI (𝛽 = 0.099, CI [0.053; 0.162]), confirming H 26 and H 32 .
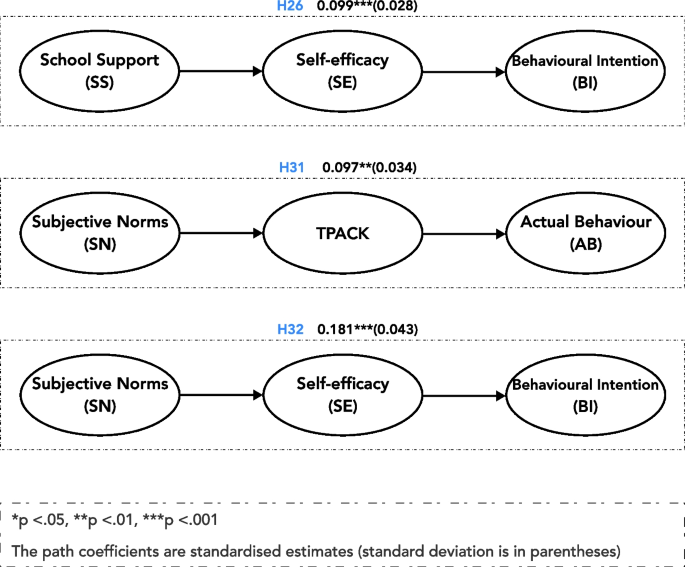
Statistically significant mediation effects
5 Discussion
The overarching objective of the current study was to formulate and validate a model that elucidates and predicts in-service K-12 teachers’ integration of LADs for assessing students’ CPS skills in an educational game. In particular, this study investigated the postulated relationships among environmental factors (i.e., SN and SS), personal factors (i.e., TPACK, TS, and SE), as well as intention (BI) and behaviour (AB) regarding LAD usage. Beyond a high explanatory power, our model demonstrated a strong out-of-sample predictive power, which provides supporting evidence for its predictive capability and external validity for similar research contexts of exploring teachers’ technology integration. It was found that SN positively predicted TPACK ( SN→TPACK ) and SE ( SN→SE ), which echoes the findings of previous studies, such as Jang et al. ( 2021 ), who examined in-service teachers’ integration of augmented reality and virtual reality techniques into teaching practices in elementary schools in South Korea, and Scherer et al. ( 2019 ), who identified the environmental and personal factors that determine the success of teacher technology integration using meta-analytic SEM. In line with previous studies (e.g., Dong et al., 2020 ; Joo et al., 2016 ), the impacts of SS on SE ( SS→SE ) and TS ( SS→TS ) were supported in the current study, indicating that backing and promotion of technology integration by school administrators could bolster teachers’ confidence and mitigate their technostress during the implementation of new technologies, such as LADs, in classrooms.
Results also showed that SS significantly predicted AB ( SS→AB ), which corroborates Atman Uslu and Usluel’s ( 2019 ) assertion that school support directly affected teachers’ utilisation of ICT in K-12 education. This was further substantiated by Hew and Syed A. Kadir ( 2017 ), who ascertained that school support is a fundamental prerequisite for the implementation of cloud computing and web 2.0 technologies for teaching purposes. Teachers’ SN was found to positively affect their BI ( SN→BI ) to employ the LAD, aligning with Wijnen et al.’s ( 2021 ) findings of systematic review, which highlighted the significance of the social acceptability of e-learning technologies within K-12 educational contexts. With respect to the personal factors, namely TPACK, TS, and SE, we found that SE ( SE→BI ) and TS ( TS→BI ) significantly predicted BI, which resonates with findings from various previous studies (e.g., Chou & Chou, 2021 ; Joo et al., 2018 ; Panisoara et al., 2020 ). To illustrate, Chou and Chou ( 2021 ) underscored the pivotal role of self-efficacy and technostress in shaping K-12 teachers’ intent to persistently employ online teaching technologies, even beyond the COVID-19 pandemic. Apart from SS, our study also identified both TPACK ( TPACK→AB ) and BI ( BI→AB ) as significant predictors of AB. Similar results were obtained in a variety of empirical studies (e.g., Anthony et al., 2021 ; Hsu et al., 2021 ; Zhang & Chen, 2022 ). For instance, Anthony et al. ( 2021 ) pinpointed lecturers’ TPACK and intention to adopt technologies for teaching as key determinants of their actual usage of e-learning systems.
Additionally, this study supported the mediating role of TAPCK in the linkage from SN to AB ( SN→TPACK→AB ). In other words, when teachers were exposed to social pressure (e.g., peers, societal trends) in using LADs, they would more likely incorporate LADs into their teaching with the consideration of their pedagogy and subject matter, which would subsequently lead to their increasing actual usage of LADs. This finding implies the importance of peer influence in teachers’ adoption of emerging educational technologies. The mediation analyses also revealed that SN ( SN→SE→BI ) and SS ( SS→SE→BI ) had indirect effects on BI through SE. That is, teachers experiencing social pressure to use LADs or receiving school support from administrators are more likely to feel confident in actual LAD use within their classrooms, thereby developing stronger intentions towards LAD integration into teaching. Upon reviewing existing literature on technology acceptance and adoption, we discovered numerous studies providing evidence of the direct influences of SN on AB (e.g., see review by Wijnen et al., 2021 ) and also those of SN and SS on BI (e.g., Jeong & Kim, 2017 ; Jung et al., 2019 ; Porter & Graham, 2016 ). However, no studies, to the best of our knowledge, have examined the mediation effects on these relationships.
A plausible explanation for such significant mediation effects could lie in the active environments we created—such as offering training sessions and establishing social media communities—where teachers could learn, discuss, and share ideas on incorporating LAD into their teaching activities, subsequently enhancing their TPACK and confidence in LAD integration. Our findings support the claim of Fishbein and Ajzen ( 2011 ) that actual behaviour is shaped by the confluence of personal competence and environmental support, a pattern particularly evident in our research context. Furthermore, in cultures characterised by collectivist traditions and Confucian values, which emphasise conformity and respect for authority, the decisions teachers make regarding technology integration into classroom settings may be directly or indirectly influenced by environmental factors (Huang et al., 2019 ; Huang & Teo, 2020 ; Teo et al., 2019 ). Specifically, the subjective opinions and tangible support from influential figures around them, such as school leaders, administrators, and colleagues, can play crucial roles (Huang & Teo, 2020 ).
6 Conclusion
To investigate in-service teachers’ integration of a mobile LAD for game-based CPS assessments in K-12 classrooms, the present study constructed and tested an integrated conceptual model based on the person-environment fit theory. This model was validated using PLS-SEM on survey data collected from 300 K to 12 in-service teachers from ten schools in China. It was found that teachers’ subjective norms significantly influenced TPACK and self-efficacy, while school support significantly influenced technostress and self-efficacy. More importantly, our proposed model exhibited both strong in-sample explanatory power and out-of-sample predictive capability. In particular, behavioural intention was predicted by subjective norms, technostress, and self-efficacy, while actual behaviour was predicted by school support, TPACK, and behavioural intention. Our analysis results also highlighted the mediating roles of TPACK and self-efficacy. Specifically, TPACK mediated the impact of subjective norms on actual behaviour, and self-efficacy mediated the impacts of subjective norms and school support on behavioural intention.
Our findings yield theoretical implications for studies concerning teacher integration of advanced learning technologies, empowered by artificial intelligence and big data (e.g., learning analytics tools), into teaching practices. Grounded in the person-environment fit theory, this study advances the theoretical understanding of the factors that determine the extent to which teachers incorporate learning analytics applications into their teaching. Our study also extends the literature on teacher technology integration by uncovering the mediation effects of personal factors on the linkages from environmental factors to technology acceptance and adoption. The methodological implication of this study is underscored by its demonstration of how to assess a model’s predictive capability and external validity through out-of-sample predictive power.
The novelty of this study resides in not only in the implementation of a LAD designed for CPS assessments in authentic classroom settings but also in the investigation of teachers’ acceptance and adoption of such emerging technologies within educational contexts. Given the interactive, interdependent, and temporal features that are inherent in CPS (Swiecki et al., 2020 ), it can be challenging for teachers to measure students’ CPS skills and performance at both individual and group levels during live instruction within physical classrooms. In this study, we introduced a solution leveraging a LAD, which provides teachers with immediate and actionable feedback on individual contributions and group performance. This equips teachers with the ability to implement an evidence-based, data-driven approach to teaching 21st century skills and delivering adaptive learning support. Consequently, teachers’ LAD-empowered teaching may improve student engagement and encourage better learning attainments. Technologically, besides game-based learning, due to the prevalence of CPS skills across educational contexts, our LAD holds the potential to be applied to supporting other active learning approaches (e.g., project-based learning, problem-based learning) across a diversity of STEM and non-STEM disciplines. Our study has also constructed a research model characterised by high explanatory capacity and external validity, which could be generalised to other contexts of educational technology integration. This model illuminates the intricate relationships among environmental factors, personal characteristics, and technology acceptance and adoption. In doing so, it encapsulates multiple critical elements that shape technology integration into teaching practices, which can inform the design, implementation, and evaluation of LADs for CPS assessments.
Our research findings lend themselves to practical recommendations for facilitating teachers’ usage of LADs in their teaching. Firstly, it is advisable for teachers to forge mutually beneficial virtual communities via social media. This would provide a constructive and relaxed atmosphere conducive to dialogues and problem solving, thereby fostering LAD integration into teaching practices. Secondly, schools can launch professional and technological training initiatives, inclusive of workshops, seminars, and certificate programs, with the objective of enhancing teachers’ TPACK, an essential prerequisite for the seamless and sustainable integration of LADs. These professional development programs should also develop teachers’ data literacy knowledge and skills, such as how to interpret data and formulate pedagogical responses (Liu et al., 2023 ; Khulbe & Tammets, 2023 ), particularly through capacity-building and reflective activities (Cui & Zhang, 2022 ). Thirdly, it is suggested that schools provide required software, hardware, and timely assistance both in-person and online under researchers’ support. These endeavours can mitigate teachers’ technostress, build their confidence, and even directly affect their actual utilisation of LAD. Lastly, given the significant mediating roles of TPACK and self-efficacy, school administrators should pay close attention to the needs of teachers displaying inadequate TPACK skills and low confidence in LAD usage. This is particularly applicable to those possessing traditional teaching conceptions (Tsai & Tsai, 2019 ) or limited information and digital literacy (Lim, 2023 ).
This study has several limitations that should be addressed in future research. Firstly, all the participating teachers are from China, which might impact the generalisation of our research findings. Future researchers are encouraged to leverage our proposed model to investigate teachers’ integration of other emerging technologies in other sociocultural contexts. In particular, the cultural norms in different educational systems (e.g., collectivist versus individualist tendencies, respectively in Chinese and Western systems) and the teachers’ cultural beliefs can also be considered influential factors on LAD adoption (Huang et al., 2019 , 2021b ; Teo & Huang, 2019 ). Secondly, although the proposed model has been validated in this study, the exclusive reliance on survey data might limit our understanding of the in-depth reasons behind teachers’ intentions and behaviours regarding LAD integration. Future investigations would benefit from gathering and analysing multimodal data (e.g., interviews and physical signals) to corroborate and enrich our research findings. For instance, qualitative data and methodologies (e.g., document analysis of institutional policies) can reveal the extent to which inter-twined policy-related and institutional factors (e.g., comprehensiveness of infrastructure) would affect LAD integration (Broos et al., 2020 ). Finally, despite the use of PLS-SEM in our study to examine relationships among variables, this variable-centred method may not fully account for the influences of teachers’ individual characteristics (e.g., digital literacy levels) on the actual use of LADs. Person-centred methods (e.g., clustering analysis and finite mixture modelling) could be adopted to further probe how distinct teacher profiles contribute to variability in integrating LADs into classrooms.
Data availability
The raw datasets used in the current study are not publicly available due to ethics requirements, but the anonymized data are available from the corresponding author upon reasonable request.
Admiraal, W., Louws, M., Lockhorst, D., Paas, T., Buynsters, M., Cviko, A., & van der Ven, F. (2017). Teachers in school-based technology innovations: A typology of their beliefs on teaching and technology. Computers & Education, 114 , 57–68. https://doi.org/10.1016/j.compedu.2017.06.013
Article Google Scholar
Ajzen, I., & Fishbein, M. (1980). Understanding attitudes and predicting social behaviour . Prentice-Hall.
Google Scholar
Al-Fudail, M., & Mellar, H. (2008). Investigating teacher stress when using technology. Computers & Education, 51 (3), 1103–1110. https://doi.org/10.1016/j.compedu.2007.11.004
Anderson, J. C., & Gerbing, D. W. (1988). Structural equation modeling in practice: A review and recommended two-step approach. Psychological Bulletin, 103 (3), 411–423.
Andrews-Todd, J., & Forsyth, C. M. (2020). Exploring social and cognitive dimensions of collaborative problem solving in an open online simulation-based task. Computers in Human Behavior, 104 , 105759. https://doi.org/10.1016/j.chb.2018.10.025
Anthony, B., Kamaludin, A., & Romli, A. (2021). Predicting academic staffs behaviour intention and actual use of blended learning in higher education: Model development and validation. Technology Knowledge and Learning, 28 , 1223–1269. https://doi.org/10.1007/s10758-021-09579-2
Archambault, L., & Crippen, K. (2009). Examining TPACK among K-12 online distance educators in the United States. Contemporary Issues in Technology and Teacher Education, 9 (1), 71–88. Retrieved from https://www.learntechlib.org/primary/p/29332/
Atman Uslu, N., & Usluel, Y. K. (2019). Predicting technology integration based on a conceptual framework for ICT use in education. Technology Pedagogy and Education, 28 (5), 517–531. https://doi.org/10.1080/1475939X.2019.1668293
Ayyagari, R., Grover, V., & Purvis, R. (2011). Technostress: Technological antecedents and implications. MIS Quarterly, 35 (4), 831–858. https://doi.org/10.2307/41409963
Azevedo, R., & Gašević, D. (2019). Analysing multimodal multichannel data about self-regulated learning with advanced learning technologies: Issues and challenges. Computers in Human Behavior, 96 , 207–210. https://doi.org/10.1016/j.chb.2019.03.025
Bandura, A. (1997). Self-efficacy: The exercise of control . W. H. Freeman and Company.
Bao, H., Li, Y., Su, Y., Xing, S., Chen, N. S., & Rosé, C. P. (2021). The effects of a learning analytics dashboard on teachers’ diagnosis and intervention in computer-supported collaborative learning. Technology Pedagogy and Education, 30 (2), 287–303. https://doi.org/10.1080/1475939X.2021.1902383
Baucal, A., Jošić, S., Ilić, I. S., Videnović, M., Ivanović, J., & Krstić, K. (2023). What makes peer collaborative problem solving productive or unproductive: A qualitative systematic review. Educational Research Review , 100567. https://doi.org/10.1016/j.edurev.2023.100567
Broos, T., Hilliger, I., Pérez-Sanagustín, M., Htun, N. N., Millecamp, M., Pesántez-Cabrera, P., & De Laet, T. (2020). Coordinating learning analytics policymaking and implementation at scale. British Journal of Educational Technology, 51 (4), 938–954. https://doi.org/10.1111/bjet.12934
Calvo-Morata, A., Alonso-Fernández, C., Pérez-Colado, I. J., Freire, M., Martínez-Ortiz, I., & Fernandez-Manjon, B. (2019). Improving teacher game learning analytics dashboards through ad-hoc development. Journal of Universal Computer Science, 25 (12), 1507–1530. https://doi.org/10.3217/jucs-025-12-1507
Care, E., & Kim, H. (2018). Assessment of 21st century skills: The issue of authenticity. In E. Care, P. Griffin, & M. Wilson (Eds.), Assessment and teaching of 21st century skills: Research and applications (pp. 21–39). Springer.
Care, E., Scoular, C., & Griffin, P. (2016). Assessment of collaborative problem solving in education environments. Applied Measurement in Education, 29 (4), 250–264. https://doi.org/10.1080/08957347.2016.1209204
Chen, Y., Hmelo-Silver, C. E., Lajoie, S. P., Zheng, J., Huang, L., & Bodnar, S. (2021). Using teacher dashboards to assess group collaboration in problem-based learning. Interdisciplinary Journal of Problem-Based Learning, 15 (2), 1–23. https://doi.org/10.14434/ijpbl.v15i2.28792
Chen, Y., Bae, H., Saleh, A., Uttamchandani, S., Hmelo-Silver, C. E., Glazewski, K., ... & Lester, J. (2022). A real-time teacher dashboard for a game-based collaborative inquiry learning environment. In J., Oshima, T., Mochizuki, & Hayashi, Y. (Eds.), General Proceedings of the 2nd Annual Meeting of the International Society of the Learning Sciences (pp. 32–35).
Chou, H. L., & Chou, C. (2021). A multigroup analysis of factors underlying teachers’ technostress and their continuance intention toward online teaching. Computers & Education, 175 , 104335. https://doi.org/10.1016/j.compedu.2021.104335
Cui, Y., & Zhang, H. (2022). Integrating teacher data literacy with TPACK: A self-report study based on a novel framework for teachers’ professional development. Frontiers in Psychology, 13 , 966575. https://doi.org/10.3389/fpsyg.2022.966575
Cukurova, M., Luckin, R., Millán, E., & Mavrikis, M. (2018). The NISPI framework: Analysing collaborative problem-solving from students’ physical interactions. Computers & Education, 116 , 93–109. https://doi.org/10.1016/j.compedu.2017.08.007
Davis, F. D., Bagozzi, R. P., & Warshaw, P. R. (1989). User acceptance of computer technology: A comparison of two theoretical models. Management Science, 35 (8), 982–1003. https://doi.org/10.1287/mnsc.35.8.982
Dong, Y., Xu, C., Chai, C. S., & Zhai, X. (2020). Exploring the structural relationship among teachers’ technostress, technological pedagogical content knowledge (TPACK), computer self-efficacy and school support. The Asia-Pacific Education Researcher, 29 , 147–157. https://doi.org/10.1007/s40299-019-00461-5
Edwards, J. R., Caplan, R. D., & Harrison, V. R. (1998). Person-environment fit theory: Conceptual foundations, empirical evidence, and directions for future research. In C. L. Cooper (Ed.), Theories of organisational stress (pp. 28–67). Oxford University Press.
Faul, F., Erdfelder, E., Buchner, A., & Lang, A. G. (2009). Statistical power analyses using G*Power 3.1: Tests for correlation and regression analyses. Behavior Research Methods, 41 (4), 1149–1160. https://doi.org/10.3758/BRM.41.4.1149
Fernández-Batanero, J. M., Román-Graván, P., Reyes-Rebollo, M. M., & Montenegro-Rueda, M. (2021). Impact of educational technology on teacher stress and anxiety: A literature review. International Journal of Environmental Research and Public Health, 18 (2), 548. https://doi.org/10.3390/ijerph18020548
Fiore, S. M., Graesser, A., Greiff, S., Griffin, P., Gong, B., Kyllonen, P., ... von Davier, A. A. (2017). Collaborative problem solving: Considerations for the National Assessment of Educational Progress . National Center for Education Statistics.
Fiore, S. M., Graesser, A., & Greiff, S. (2018). Collaborative problem-solving education for the twenty-first-century workforce. Nature Human Behaviour, 2 (6), 367–369. https://doi.org/10.1038/s41562-018-0363-y
Fishbein, M., & Ajzen, I. (2011). Predicting and changing behaviour: The reasoned action approach. Taylor and Francis . https://doi.org/10.4324/9780203838020
Gomez, M. J., Ruipérez-Valiente, J. A., & Clemente, F. J. G. (2022). A systematic literature review of game-based assessment studies: Trends and challenges. IEEE Transactions on Learning Technologies, 16 (4), 500–515. https://doi.org/10.1109/TLT.2022.3226661
Govender, R., & Mpungose, C. (2022). Lecturers’ technostress at a South African university in the context of coronavirus (COVID-19). Cogent Education, 9 (1), 2125205. https://doi.org/10.1080/2331186X.2022.2125205
Graesser, A., Kuo, B. C., & Liao, C. H. (2017). Complex problem solving in assessments of collaborative problem solving. Journal of Intelligence, 5 (2), 10. https://doi.org/10.3390/jintelligence5020010
Graesser, A. C., Fiore, S. M., Greiff, S., Andrews-Todd, J., Foltz, P. W., & Hesse, F. W. (2018). Advancing the science of collaborative problem solving. Psychological Science in the Public Interest, 19 (2), 59–92. https://doi.org/10.1177/1529100618808244
Greiff, S., Wüstenberg, S., Csapó, B., Demetriou, A., Hautamäki, J., Graesser, A. C., & Martin, R. (2014). Domain-general problem solving skills and education in the 21st century. Educational Research Review, 13 , 74–83. https://doi.org/10.1016/j.edurev.2014.10.002
Griffin, P. (2017). Assessing and teaching 21st century skills: Collaborative problem solving as a case study. In A. A. von Davier, M. Zhu, & P. C. Kyllonen (Eds.), Innovative assessment of collaboration (pp. 113–134). Springer.
Griffin, P., & Care, E. (2015). Assessment and Teaching of 21st Century skills: Methods and approach . Springer.
Book Google Scholar
Hair, J. F., Hult, G. T. M., Ringle, C. M., & Sarstedt, M. (2021). A primer on partial least squares structural equation modeling (PLS-SEM) . Sage Publications.
Hesse, F., Care, E., Buder, J., Sassenberg, K., & Griffin, P. (2015). A framework for teachable collaborative problem solving skills. In P. Griffin, and E. Care (Eds.), Assessment and teaching of 21st century skills: Methods and approach (pp. 37–56). Springer.
Hew, T. S., Syed, A., & Kadir, S. L. (2017). Applying channel expansion and self-determination theory in predicting use behaviour of cloud-based VLE. Behaviour & Information Technology, 36 (9), 875–896. https://doi.org/10.1080/0144929X.2017.1307450
Hsu, C. Y., Liang, J. C., Chuang, T. Y., Chai, C. S., & Tsai, C. C. (2021). Probing in-service elementary school teachers’ perceptions of TPACK for games, attitudes towards games, and actual teaching usage: A study of their structural models and teaching experiences. Educational Studies, 47 (6), 734–750. https://doi.org/10.1080/03055698.2020.1729099
Hu, X., Ng, J. T. D., & Chu, S. K. (2022). Implementing learning analytics in wiki-supported collaborative learning in secondary education: A framework-motivated empirical study. International Journal of Computer-Supported Collaborative Learning, 17 (3), 427–455. https://doi.org/10.1007/s11412-022-09377-7
Huang, F., & Teo, T. (2020). Influence of teacher-perceived organisational culture and school policy on Chinese teachers’ intention to use technology: An extension of technology acceptance model. Educational Technology Research and Development, 68 (3), 1547–1567. https://doi.org/10.1007/s11423-019-09722-y
Huang, F., Teo, T., Sánchez-Prieto, J. C., García-Peñalvo, F. J., & Olmos-Migueláñez, S. (2019). Cultural values and technology adoption: A model comparison with university teachers from China and Spain. Computers & Education, 133 , 69–81. https://doi.org/10.1016/j.compedu.2019.01.012
Huang, L., Li, S., Poitras, E. G., & Lajoie, S. P. (2021a). Latent profiles of self-regulated learning and their impacts on teachers’ technology integration. British Journal of Educational Technology , 52 (2), 695–713. https://doi.org/10.1111/bjet.13050 .
Huang, F., Sánchez-Prieto, J. C., Teo, T., García-Peñalvo, F. J., Olmos-Migueláñez, S., & Zhao, C. (2021b). A cross-cultural study on the influence of cultural values and teacher beliefs on university teachers’ information and communications technology acceptance. Educational Technology Research and Development, 69 , 1271–1297. https://doi.org/10.1007/s11423-021-09941-2
Huang, L., Zheng, J., Lajoie, S. P., Chen, Y., Hmelo-Silver, C. E., & Wang, M. (2023). Examining university teachers’ self-regulation in using a learning analytics dashboard for online collaboration. Education and Information Technologies , 1–25. https://doi.org/10.1007/s10639-023-12131-7
Ifinedo, E., & Kankaanranta, M. (2021). Understanding the influence of context in technology integration from teacher educators’ perspective. Technology Pedagogy and Education, 30 (2), 201–215. https://doi.org/10.1080/1475939X.2020.1867231
Jang, J., Ko, Y., Shin, W. S., & Han, I. (2021). Augmented reality and virtual reality for learning: An examination using an extended technology acceptance model. IEEE Access : Practical Innovations, Open Solutions, 9 , 6798–6809. https://doi.org/10.1109/ACCESS.2020.3048708
Jeong, H. I., & Kim, Y. (2017). The acceptance of computer technology by teachers in early childhood education. Interactive Learning Environments, 25 (4), 496–512. https://doi.org/10.1080/10494820.2016.1143376
Joo, Y. J., Lim, K. Y., & Kim, N. H. (2016). The effects of secondary teachers’ technostress on the intention to use technology in South Korea. Computers & Education, 95 , 114–122. https://doi.org/10.1016/j.compedu.2015.12.004
Joo, Y. J., Park, S., & Lim, E. (2018). Factors influencing preservice teachers’ intention to use technology: TPACK, teacher self-efficacy, and technology acceptance model. Journal of Educational Technology & Society, 21 (3), 48–59. Retrieved November 11, 2023, from https://www.jstor.org/stable/26458506
Jung, Y. J., Cho, K., & Shin, W. S. (2019). Revisiting critical factors on teachers’ technology integration: The differences between elementary and secondary teachers. Asia Pacific Journal of Education , 39 (4), 548–561. https://doi.org/10.1080/02188791.2019.1620683 .
Kaimara, P., Fokides, E., Oikonomou, A., & Deliyannis, I. (2021). Potential barriers to the implementation of digital game-based learning in the classroom: Preservice teachers’ views. Technology Knowledge and Learning, 26 (4), 825–844. https://doi.org/10.1007/s10758-021-09512-7
Kaliisa, R., & Dolonen, J. A. (2023). CADA: A teacher-facing learning analytics dashboard to foster teachers’ awareness of students’ participation and discourse patterns in online discussions. Technology Knowledge and Learning, 28 (3), 937–958. https://doi.org/10.1007/s10758-022-09598-7
Kaliisa, R., Gillespie, A., Herodotou, C., Kluge, A., & Rienties, B. (2021). Teachers’ perspectives on the promises, needs and challenges of learning analytics dashboards: Insights from institutions offering blended and distance learning. In M. Sahin & D. Ifenthaler (Eds.), Visualisations and dashboards for learning analytics (pp. 351–370). Springer. https://doi.org/10.1007/978-3-030-81222-5_16
Khulbe, M., & Tammets, K. (2023). Mediating teacher professional learning with a learning analytics dashboard and training intervention. Technology Knowledge and Learning, 28 (3), 981–998. https://doi.org/10.1007/s10758-023-09642-0
Koh, J. H. L., Chai, C. S., & Lim, W. Y. (2017). Teacher professional development for TPACK-21CL: Effects on teacher ICT integration and student outcomes. Journal of Educational Computing Research, 55 (2), 172–196. https://doi.org/10.1177/0735633116656848
Kristof-Brown, A., & Guay, R. P. (2011). Person-environment fit. In S. Zedeck (Ed.), Handbook of industrial/organisational psychology (Vol. 3, pp. 3–50). American Psychological Association.
Kristof-Brown, A. L., Zimmerman, R. D., & Johnson, E. C. (2005). Consequences of individuals’ fit at work: A meta‐analysis of person-job, person-organisation, person-group, and person-supervisor fit. Personnel Psychology , 58 (2), 281–342. https://doi.org/10.1111/j.1744-6570.2005.00672.x .
Kwon, K., Ottenbreit-Leftwich, A. T., Sari, A. R., Khlaif, Z., Zhu, M., Nadir, H., & Gok, F. (2019). Teachers’ self-efficacy matters: Exploring the integration of mobile computing device in middle schools. TechTrends, 63 , 682–692. https://doi.org/10.1007/s11528-019-00402-5
Lam, S. F., Cheng, R. W. Y., & Choy, H. C. (2010). School support and teacher motivation to implement project-based learning. Learning and Instruction, 20 (6), 487–497. https://doi.org/10.1016/j.learninstruc.2009.07.003
Lee-Cultura, S., Sharma, K., & Giannakos, M. (2023). Multimodal teacher dashboards: Challenges and opportunities of enhancing teacher insights through a case study. IEEE Transactions on Learning Technologies , 1–19. https://doi.org/10.1109/TLT.2023.3276848
Li, L., & Wang, X. (2021). Technostress inhibitors and creators and their impacts on university teachers’ work performance in higher education. Cognition Technology & Work, 23 , 315–330. https://doi.org/10.1007/s10111-020-00625-0
Li, Y., Zhang, M., Su, Y., Bao, H., & Xing, S. (2022). Examining teachers’ behavior patterns in and perceptions of using teacher dashboards for facilitating guidance in CSCL. Educational Technology Research and Development, 70 (3), 1035–1058. https://doi.org/10.1007/s11423-022-10102-2
Lim, E. M. (2023). The effects of pre-service early childhood teachers’ digital literacy and self-efficacy on their perception of AI education for young children. Education and Information Technologies, 28 , 12969–12995. https://doi.org/10.1007/s10639-023-11724-6
Liu, F., Ritzhaupt, A. D., Dawson, K., & Barron, A. E. (2017). Explaining technology integration in K-12 classrooms: A multilevel path analysis model. Educational Technology Research and Development, 65 , 795–813. https://doi.org/10.1007/s11423-016-9487-9
Liu, Y., Huang, L., & Doleck, T. (2023). How teachers’ self-regulation, emotions, perceptions, and experiences predict their capacities for learning analytics dashboard: A Bayesian approach. Education and Information Technologies, 1–36. https://doi.org/10.1007/s10639-023-12163-z
Liu, Y., Ng, J. T. D., Hu, X., Ma, Z., & Lai, X. (2024). Adopt or abandon: Facilitators and barriers of in-service teachers’ integration of game learning analytics in K–12 classrooms?. Computers & Education, 209, 104951. https://doi.org/10.1016/j.compedu.2023.104951
Martinez-Maldonado, R. (2019). A handheld classroom dashboard: Teachers’ perspectives on the use of real-time collaborative learning analytics. International Journal of Computer-Supported Collaborative Learning, 14 , 383–411. https://doi.org/10.1007/s11412-019-09308-z
OECD (2017b). PISA 2015 results (volume V): Collaborative problem solving . OECD. https://doi.org/10.1787/9789264285521-en
OECD (2017a). PISA 2015 assessment and analytical framework: Science, reading, mathematic, financial literacy and collaborative problem solving . OECD. https://doi.org/10.1787/9789264281820-en
Özgür, H. (2020). Relationships between teachers’ technostress, technological pedagogical content knowledge (TPACK), school support and demographic variables: A structural equation modeling. Computers in Human Behavior, 112 , 106468. https://doi.org/10.1016/j.chb.2020.106468
Panisoara, I. O., Lazar, I., Panisoara, G., Chirca, R., & Ursu, A. S. (2020). Motivation and continuance intention towards online instruction among teachers during the COVID-19 pandemic: The mediating effect of burnout and technostress. International Journal of Environmental Research and Public Health, 17 (21), 8002. https://doi.org/10.3390/ijerph17218002
Petko, D., Prasse, D., & Cantieni, A. (2018). The interplay of school readiness and teacher readiness for educational technology integration: A structural equation model. Computers in the Schools, 35 (1), 1–18. https://doi.org/10.1080/07380569.2018.1428007
Porter, W. W., & Graham, C. R. (2016). Institutional drivers and barriers to faculty adoption of blended learning in higher education. British Journal of Educational Technology, 47 (4), 748–762. https://doi.org/10.1111/bjet.12269
Qian, M., & Clark, K. R. (2016). Game-based learning and 21st century skills: A review of recent research. Computers in Human Behavior, 63 , 50–58. https://doi.org/10.1016/j.chb.2016.05.023
Rienties, B., Herodotou, C., Olney, T., Schencks, M., & Boroowa, A. (2018). Making sense of learning analytics dashboards: A technology acceptance perspective of 95 teachers. International Review of Research in Open and Distributed Learning, 19 (5), 187–202. https://doi.org/10.19173/irrodl.v19i5.3493
Ruipérez-Valiente, J. A., Gomez, M. J., Martínez, P. A., & Kim, Y. J. (2021). Ideating and developing a visualisation dashboard to support teachers using educational games in the classroom. IEEE Access : Practical Innovations, Open Solutions, 9 , 83467–83481. https://doi.org/10.1109/ACCESS.2021.3086703
Saleh, A., Phillips, T. M., Hmelo-Silver, C. E., Glazewski, K. D., Mott, B. W., & Lester, J. C. (2022). A learning analytics approach towards understanding collaborative inquiry in a problem‐based learning environment. British Journal of Educational Technology, 53 (5), 1321–1342. https://doi.org/10.1111/bjet.13198
Scherer, R., Siddiq, F., & Tondeur, J. (2019). The technology acceptance model (TAM): A meta-analytic structural equation modeling approach to explaining teachers’ adoption of digital technology in education. Computers & Education, 128 , 13–35. https://doi.org/10.1016/j.compedu.2018.09.009
Schildkamp, K., Poortman, C., Luyten, H., & Ebbeler, J. (2017). Factors promoting and hindering data-based decision making in schools. School Effectiveness and School Improvement, 28 (2), 242–258. https://doi.org/10.1080/09243453.2016.1256901
Schmid, M., Brianza, E., & Petko, D. (2021). Self-reported technological pedagogical content knowledge (TPACK) of preservice teachers in relation to digital technology use in lesson plans. Computers in Human Behavior, 115 , 106586. https://doi.org/10.1016/j.chb.2020.106586
Schmidt, D. A., Baran, E., Thompson, A. D., Mishra, P., Koehler, M. J., & Shin, T. S. (2009). Technological pedagogical content knowledge (TPACK): The development and validation of an assessment instrument for preservice teachers. Journal of Research on Technology in Education, 42 (2), 123–149. https://doi.org/10.1080/15391523.2009.10782544
Scoular, C., & Care, E. (2018). Teaching 21st century skills: Implications at system levels in Australia. In E. Care, P. Griffin, & M. Wilson (Eds.), Assessment and teaching of the 21st century skills: Research and applications (pp. 145–162). Springer.
Shin, W. S. (2015). Teachers’ use of technology and its influencing factors in Korean elementary schools. Technology Pedagogy and Education , 24 (4), 461–476. https://doi.org/10.1080/1475939X.2014.915229 .
Shmueli, G., Sarstedt, M., Hair, J. F., Cheah, J. H., Ting, H., Vaithilingam, S., & Ringle, C. M. (2019). Predictive model assessment in PLS-SEM: Guidelines for using PLSpredict. European Journal of Marketing, 53 (11), 2322–2347. https://doi.org/10.1108/EJM-02-2019-0189
Siyam, N. (2019). Factors impacting special education teachers’ acceptance and actual use of technology. Education and Information Technologies , 24 (3), 2035–2057. https://doi.org/10.1007/s10639-018-09859-y .
Song, Y. (2018). Improving primary students’ collaborative problem solving competency in project-based science learning with productive failure instructional design in a seamless learning environment. Educational Technology Research and Development , 66 , 979–1008. https://doi.org/10.1007/s11423-018-9600-3 .
Stieler-Hunt, C. J., & Jones, C. M. (2017). Feeling alienated–teachers using immersive digital games in classrooms. Technology Pedagogy and Education, 26 (4), 457–470. https://doi.org/10.1080/1475939X.2017.1334227
Sun, J. C. Y., & Liu, Y. (2022). The mediation effect of online self-regulated learning between engagement and cognitive load: A case of an online course with smart instant feedback. International Journal of Online Pedagogy and Course Design (IJOPCD), 12 (1), 1–17. https://doi.org/10.4018/IJOPCD.295953
Sun, C., Shute, V. J., Stewart, A. E. B., Beck-White, Q., Reinhardt, C. R., Zhou, G., ... & D’Mello, S. K. (2022). The relationship between collaborative problem solving behaviors and solution outcomes in a game-based learning environment. Computers in Human Behavior, 128 , 107120. https://doi.org/10.1016/j.chb.2021.107120
Swiecki, Z., Ruis, A. R., Farrell, C., & Shaffer, D. W. (2020). Assessing individual contributions to collaborative problem solving: A network analysis approach. Computers in Human Behavior, 104 , 105876. https://doi.org/10.1016/j.chb.2019.01.009
Teo, T., & Huang, F. (2019). Investigating the influence of individually espoused cultural values on teachers’ intentions to use educational technologies in Chinese universities. Interactive Learning Environments, 27 (5–6), 813–829. https://doi.org/10.1080/10494820.2018.1489856
Teo, T., & van Schaik, P. (2009). Understanding technology acceptance among preservice teachers: A structural-equation modeling approach. The Asia-Pacific Education Researcher, 18 (1), 47–66. https://doi.org/10.3860/taper.v18i1.1035
Teo, T., Sang, G., Mei, B., & Hoi, C. K. W. (2019). Investigating pre-service teachers’ acceptance of web 2.0 technologies in their future teaching: A Chinese perspective. Interactive Learning Environments, 27 (4), 530–546. https://doi.org/10.1080/10494820.2018.1489290
Tian, Q., & Zheng, X. (2023) Effectiveness of online collaborative problem-solving method on students’ learning performance: A meta‐analysis. Journal of Computer Assisted Learning , 1–16. https://doi.org/10.1111/jcal.12884
Tlili, A., Chang, M., Moon, J., Liu, Z., Burgos, D., Chen, N. S., & Kinshuk, K. (2021a). A systematic literature review of empirical studies on learning analytics in educational games. International Journal of Interactive Multimedia and Artificial Intelligence, 7 (2), 250–261. https://doi.org/10.9781/ijimai.2021.03.003
Tlili, A., Hattab, S., Essalmi, F., Chen, N. S., Huang, R., Martínez, K., ... & Burgos, D. (2021b). A smart collaborative educational game with learning analytics to support english vocabulary teaching. International Journal of Interactive Multimedia and Artificial Intelligence, 6 (6), 215–224. https://doi.org/10.9781/ijimai.2021.03.002
Tsai, P. S., & Tsai, C. C. (2019). Preservice teachers’ conceptions of teaching using mobile devices and the quality of technology integration in lesson plans. British Journal of Educational Technology, 50 (2), 614–625. https://doi.org/10.1111/bjet.12613
Tsang, H. W. C., Liu, Y., & Law, N. (2020). An in-depth study of assessment of collaborative problem solving (CPS) skills of students in both technological and authentic learning settings (pp. 1381–1388). International Society of the Learning Sciences. Retrieved November 11, 2023, from https://repository.isls.org//handle/1/6340
Van Leeuwen, A. (2019). Teachers’ perceptions of the usability of learning analytics reports in a flipped university course: When and how does information become actionable knowledge? Educational Technology Research and Development, 67 , 1043–1064. https://doi.org/10.1007/s11423-018-09639-y
Van Leeuwen, A., Rummel, N., & Van Gog, T. (2019). What information should CSCL teacher dashboards provide to help teachers interpret CSCL situations? International Journal of Computer-Supported Collaborative Learning, 14 , 261–289. https://doi.org/10.1007/s11412-019-09299-x
Van Leeuwen, A., Knoop-van Campen, C. A. N., Molenaar, I., & Rummel, N. (2021). How teacher characteristics relate to how teachers use dashboards: Results from two case studies in K-12. Journal of Learning Analytics, 8 (2), 6–21. https://doi.org/10.18608/jla.2021.7325
von Davier, A. A., & Halpin, P. F. (2013). Collaborative problem solving and the assessment of cognitive skills: Psychometric considerations. ETS Research Report Series, 2013 (2), i–36. https://doi.org/10.1002/j.2333-8504.2013.tb02348.x
Weil, M. M., & Rosen, L. D. (1997). Technostress: Coping with technology @Work @Home @Play . Wiley.
Wijnen, F., van der Walma, J., & Voogt, J. (2021). Primary school teachers’ attitudes toward technology use and stimulating higher-order thinking in students: A review of the literature. Journal of Research on Technology in Education , 55 (4), 545–567. https://doi.org/10.1080/15391523.2021.1991864 .
Wu, D., Yang, X., Yang, W., Lu, C., & Li, M. (2022). Effects of teacher- and school- level ICT training on teachers’ use of digital educational resources in rural schools in China: A multilevel moderation model. International Journal of Educational Research, 111 , 101910. https://doi.org/10.1016/j.ijer.2021.101910
Zhang, M., & Chen, S. (2022). Modeling dichotomous technology use among university EFL teachers in China: The roles of TPACK, affective and evaluative attitudes towards technology. Cogent Education, 9 (1), 2013396. https://doi.org/10.1080/2331186X.2021.2013396
Zhao, X., Lynch, J. G., & Chen, Q. (2010). Reconsidering Baron and Kenny: Myths and truths about mediation analysis. Journal of Consumer Research , 37 (2), 197–206. https://doi.org/10.1086/651257 .
Zheng, J., Huang, L., Li, S., Lajoie, S. P., Chen, Y., & Hmelo-Silver, C. E. (2021). Self-regulation and emotion matter: A case study of instructor interactions with a learning analytics dashboard. Computers & Education , 161 , 104061. https://doi.org/10.1016/j.compedu.2020.104061 .
Download references
Acknowledgements
The authors would like to extend the gratitude to the participating schools, teachers, and students.
This work was supported by the Research Grants Council of the HKSAR Government, #T44-707/16 N, under the Theme Based Research Scheme and Guangdong Planning Office of Philosophy and Social Science, China [Grant No. GD24YJY14].
Author information
Authors and affiliations.
Faculty of Education, The University of Hong Kong, Hong Kong, SAR, China
Yiming Liu, Xiao Hu & Jeremy Tzi Dong Ng
Computational Media and Arts Thrust, The Hong Kong University of Science and Technology (Guangzhou), Guangzhou, China
Zhengyang Ma
School of Educational Sciences, Guangdong Polytechnic Normal University, Guangzhou, China
Xiaoyan Lai
You can also search for this author in PubMed Google Scholar
Contributions
Yiming Liu : Conceptualization, Data curation, Formal analysis, Investigation, Methodology, Software, Visualization, Writing - original draft, Writing - review & editing. Xiao Hu : Conceptualization, Funding acquisition, Methodology, Resources, Supervision, Writing - review & editing. Jeremy T. D. Ng : Investigation, Validation, Writing - review & editing. Zhengyang Ma : Investigation, Validation, Software, Visualization, Writing - review & editing. Xiaoyan Lai : Investigation, Validation, Writing - review & editing.
Corresponding author
Correspondence to Yiming Liu .
Ethics declarations
Conflicts of interest.
There are no conflicts of interest to declare.
Additional information
Publisher’s note.
Springer Nature remains neutral with regard to jurisdictional claims in published maps and institutional affiliations.
Rights and permissions
Open Access This article is licensed under a Creative Commons Attribution 4.0 International License, which permits use, sharing, adaptation, distribution and reproduction in any medium or format, as long as you give appropriate credit to the original author(s) and the source, provide a link to the Creative Commons licence, and indicate if changes were made. The images or other third party material in this article are included in the article's Creative Commons licence, unless indicated otherwise in a credit line to the material. If material is not included in the article's Creative Commons licence and your intended use is not permitted by statutory regulation or exceeds the permitted use, you will need to obtain permission directly from the copyright holder. To view a copy of this licence, visit http://creativecommons.org/licenses/by/4.0/ .
Reprints and permissions
About this article
Liu, Y., Hu, X., Ng, J.T.D. et al. Ready or not? Investigating in-service teachers’ integration of learning analytics dashboard for assessing students’ collaborative problem solving in K–12 classrooms. Educ Inf Technol (2024). https://doi.org/10.1007/s10639-024-12842-5
Download citation
Received : 21 January 2024
Accepted : 04 June 2024
Published : 10 July 2024
DOI : https://doi.org/10.1007/s10639-024-12842-5
Share this article
Anyone you share the following link with will be able to read this content:
Sorry, a shareable link is not currently available for this article.
Provided by the Springer Nature SharedIt content-sharing initiative
- 21st century skills
- Collaborative problem solving
- Learning analytics dashboard
- Technology integration
- Person-environment fit
- K-12 teachers
- Find a journal
- Publish with us
- Track your research
More From Forbes
The dynamic learning model in manufacturing.
- Share to Facebook
- Share to Twitter
- Share to Linkedin
Raghunandan Gurumurthy is a global expert in industrial engineering and Director of North America Operations at Crossover Solutions.
Especially when it comes to manufacturing, problem-solving is an art. Every day, companies within this industry face challenges that test their processes, products and, ultimately, their bottom line.
Traditional problem-solving approaches, reliant on extensive data analysis and statistical methods, can often prove to be time-consuming and ineffective. Enter the dynamic learning model, which I find to be a revolutionary approach combining first principles thinking with a deterministic process , enabling teams to address complex issues swiftly and effectively.
The Wake-Up Call
It is 7 a.m. on a sunny morning. The air is crisp and full of promise as you drive to work, ready to tackle another day. Your phone rings, shattering the calm. It’s the president of the company you supply. Your stomach churns as you answer, bracing for the worst.
"We are rejecting 15% of what you send to us after sorting. Our customer is also scrapping the product in final assembly." The call ends abruptly, leaving you with a sinking feeling and a clear mandate: What you are doing now is not working and it’s time for a new approach.
Best High-Yield Savings Accounts Of 2024
Best 5% interest savings accounts of 2024.
Your organization, like many others, boasts a robust quality structure. Teams are trained, certified and equipped with the latest tools and techniques. Despite these resources, you find that problem-solving efforts often fall short. Teams spend countless hours in meetings, analyzing vast amounts of data and generating lists of potential causes.
These methods, while sometimes effective, are frequently slow and inefficient. The simplicity of the final solutions often prompts the question: "Why do we miss the inherent simplicity of causal explanations?"
Rediscovering Common Sense Engineering
Reflecting on past successes, it became clear to me that we used to solve problems faster with fewer resources; common-sense engineering problem-solving was the norm. Over time, we gravitated towards more complex, data-driven methods, losing sight of the basics. I believe it is time to return to those roots and embrace a framework that prioritizes simplicity and efficiency—the dynamic learning model.
The Dynamic Learning Model
The dynamic learning model eschews reliance on big data, large sample sizes and complex algorithms. Instead, it leverages first principles thinking and a deterministic problem-solving approach, reminiscent of effective strategies from years past.
Overall, the dynamic learning model distinguishes itself from traditional methods by focusing on an understanding of the underlying mechanisms rather than an exclusive reliance on statistics and probabilities.
Especially when confronted with a seemingly insurmountable problem, this approach can delve deeper than identifying root causes, aiming for a comprehensive causal explanation. By deconstructing complex problems functionally, I find that this process allows teams to develop targeted and effective solutions swiftly.
David Hartshorne, in his book Diagnosing Performance and Reliability , stresses that understanding the fundamental principles behind system performance is crucial for effective diagnosis and resolution of problems. I find that the dynamic learning model aligns perfectly with this philosophy, offering a structured yet flexible framework for tackling even the most challenging manufacturing problems.
For instance, I've seen a leading tier 1 automotive company grapple with a chronic problem for six months with no resolution in sight. Traditional methods failed to identify the root cause. However, when they adopted the dynamic learning model, they found the root cause within days.
The Power Of First Principles Thinking
As mentioned, this model focuses on the fundamental drivers of a problem through a first principles approach. When utilizing first principle thinking to problem solve in manufacturing, it's important to strip away assumptions and concentrate on fundamental truths.
Begin by clearly defining the issue at hand, then breaking it down into its most basic components. This approach challenges conventional wisdom and encourages questioning long-held beliefs about processes or systems.
Once problems are constructed into their basic elements, you can integrate your expert knowledge with scientific principles to can gain a comprehensive grasp of the system's inherent reality. Using this method, I think it can help to think of yourself more as a truth-seeker versus a problem solver, uncovering insights that traditional methods might miss.
By rebuilding understanding from first principles, practitioners can develop more targeted, effective solutions based on verified facts rather than historical practices. I've seen numerous times how this approach can prove particularly effective in solving chronic manufacturing issues that have resisted conventional problem-solving methods.
Unpacking The Dynamic Learning Model: First Steps
Putting into practice the first core principle/technique of this model involves a fundamental shift in questioning from "What's wrong?" to "What's happening?" This allows us to peel back layers of complexity. By focusing on what's actually occurring rather than presuming problems, you create a foundation for comprehensive understanding.
This deep dive into causality often leads to simpler, more effective solutions that might be overlooked by traditional problem-solving methods.
A New Era of Problem-Solving
I believe the dynamic learning model represents a significant shift in the way we approach problem-solving in manufacturing. By focusing on first principles and adopting a deterministic mindset, this approach enables teams to quickly identify and address root causes, leading to more effective and sustainable solutions. The real-world success stories I have witnessed demonstrate the power of this methodology.
In the upcoming articles, I plan to explain in more detail how you can become an integral part of this new era of problem-solving.
Forbes Business Council is the foremost growth and networking organization for business owners and leaders. Do I qualify?
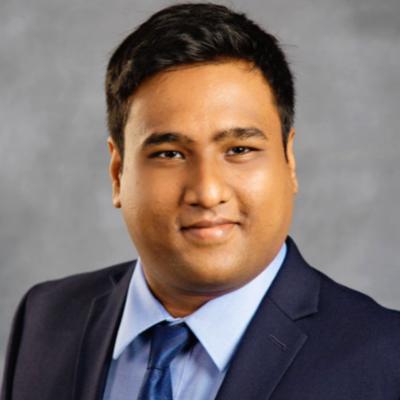
- Editorial Standards
- Reprints & Permissions
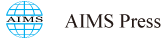
- {{subColumn.name}}
STEM Education

- {{newsColumn.name}}
- Share facebook twitter google linkedin

Solving word problems involving triangles and implications on training pre-service mathematics teachers
- William Guo ,
- School of Engineering and Technology, Central Queensland University, North Rockhampton, QLD 4702, Australia
- Academic Editor: Zlatko Jovanoski
- Received: 18 June 2024 Revised: 02 July 2024 Accepted: 05 July 2024 Published: 09 July 2024
- Full Text(HTML)
- Download PDF
Triangles and trigonometry are always difficult topics for both mathematics students and teachers. Hence, students' performance in solving mathematical word problems in these topics is not only a reflection of their learning outcomes but also an indication of teaching effectiveness. This case study drew from two examples of solving word problems involving triangles by pre-service mathematics teachers in a foundation mathematics course delivered by the author. The focus of this case study was on reasoning implications of students' performances on the effective training of pre-service mathematics teachers, from which a three-step interactive explicit teaching-learning approach, comprising teacher-led precise and inspiring teaching (or explicit teaching), student-driven engaged learning (or imitative learning), and student-led and teacher-guided problem-solving for real-world problems or projects (or active application), was summarized. Explicit teaching establishes a solid foundation for students to further their understanding of new mathematical concepts and to conceptualize the technical processes associated with these new concepts. Imitative learning helps students build technical abilities and enhance technical efficacy by engaging in learning activities. Once these first two steps have been completed, students should have a decent understanding of new mathematical concepts and technical efficacy to analyze, formulate, and finally solve real-world applications with assistance from teachers whenever required. Specially crafted professional development should also be considered for some in-service mathematics teachers to adopt this three-step interactive teaching-learning process.
- pre-service mathematics teacher ,
- word problem ,
- triangles ,
- problem-solving ,
- explicit teaching ,
- imitated learning ,
- active applications ,
- professional development
Citation: William Guo. Solving word problems involving triangles and implications on training pre-service mathematics teachers[J]. STEM Education, 2024, 4(3): 263-281. doi: 10.3934/steme.2024016
Related Papers:
[1] | , 2016, 47(7): 1028-1047. http://doi.org/10.1080/0020739X.2016.1155774 --> Koyunkaya, M.Y., Mathematics education graduate students' understanding of trigonometric ratios. , 2016, 47(7): 1028-1047. http://doi.org/10.1080/0020739X.2016.1155774 doi: |
[2] | , 2018, 6(1): 58-78. http://doi.org/10.18404/ijemst.328344 --> Koyunkaya, M.Y., An examination of a pre-service mathematics teacher's mental constructions of relationships in a right triangle. , 2018, 6(1): 58-78. http://doi.org/10.18404/ijemst.328344 doi: |
[3] | , 2019, 14(2): 72-91. https://doi.org/10.1016/j.teln.2018.11.004 --> Ngcobo, A.Z., Madonsela, S.P. and Brijlall, D., The teaching and learning of trigonometry. , 2019, 14(2): 72-91. https://doi.org/10.1016/j.teln.2018.11.004 doi: |
[4] | , 2022, 10(2): 208-224. https://doi.org/10.30935/scimath/11716 --> Durmaz, A.B. and Bostan, I.M., Pre-service teachers' knowledge regarding the area of triangle. , 2022, 10(2): 208-224. https://doi.org/10.30935/scimath/11716 doi: |
[5] | , 2018, 39: 171–196. https://doi.org/10.1007/s13138-017-0123-y --> Rellensmann, J. and Schukajlow, S., Do students enjoy computing a triangle's side? Enjoyment and boredom while solving problems with and without a connection to reality from students' and pre-service teachers' perspectives. , 2018, 39: 171–196. https://doi.org/10.1007/s13138-017-0123-y doi: |
[6] | , 2017, 5(1): 28-42. https://doi.org/10.30935/scimath/9495 --> Fyhn, A.B., What happens when a climber falls? Young climbers mathematise a climbing situation. , 2017, 5(1): 28-42. https://doi.org/10.30935/scimath/9495 doi: |
[7] | , 2023, 11(2): 249-258. https://doi.org/10.30935/scimath/12582 --> Guo, W., Solving word problems involving triangles by transitional engineering students: Learning outcomes and implications. , 2023, 11(2): 249-258. https://doi.org/10.30935/scimath/12582 doi: |
[8] | , 2015, 11(6): 1379-1397. https://doi.org/10.12973/eurasia.2015.1396a --> Dündar, S., Mathematics teacher-candidates' performance in solving problems with different representation styles: The trigonometry example. , 2015, 11(6): 1379-1397. https://doi.org/10.12973/eurasia.2015.1396a doi: |
[9] | , 2017, 36(3): 273-306. https://doi.org/10.1080/03323315.2017.1327361 --> Walsh, R., Fitzmaurice, O. and O'Donoghue, J., What subject matter knowledge do second-level teachers need to know to teach trigonometry? An exploration and case study. , 2017, 36(3): 273-306. https://doi.org/10.1080/03323315.2017.1327361 doi: |
[10] | , 2018, 9(1): 169-182. https://doi.org/10.22342/jme.9.2.5261.169-182 --> Nabie, M. J., Akayuure, P., Ibrahim-Bariham, U.A. and Sofo, S., Trigonometric concepts: Pre-service teachers' perceptions and knowledge. , 2018, 9(1): 169-182. https://doi.org/10.22342/jme.9.2.5261.169-182 doi: |
[11] | , 2021, 53(8): 2004–2025. https://doi.org/10.1080/0020739X.2020.1857858 --> Ubah, I., Pre-service mathematics teachers' semiotic transformation of similar triangles: Euclidean geometry. , 2021, 53(8): 2004–2025. https://doi.org/10.1080/0020739X.2020.1857858 doi: |
[12] | , 2022, 10(20): 3786. http://doi.org/10.3390/math10203786 --> Guo, W., Exploratory case study on solving word problems involving triangles by pre-service mathematics teachers in a regional university in Australia. , 2022, 10(20): 3786. http://doi.org/10.3390/math10203786 |
[13] | , 2024, 16(1): 58-68. https://doi.org/10.69721/TPS.J.2024.16.1.07 --> Pentang J.T., Andrade, L.J.T., Golben, J.C., Talua, J.P., Bautista, R.M., Sercenia, J.C., et al., Problem-solving difficulties, performance, and differences among preservice teachers in Western Philippines University. , 2024, 16(1): 58-68. https://doi.org/10.69721/TPS.J.2024.16.1.07 doi: |
[14] | , 2020, Pearson. --> Christensen, L.B., Johnson, R.B., Turner, L.A. and Christensen, L.B., , 2020, Pearson. |
[15] | , 2 ed. 2020, Melbourne, Australia: Pearson. --> Guo, W.W., , 2 ed. 2020, Melbourne, Australia: Pearson. |
[16] | , 2022, 10(16): 2862. https://doi.org/10.3390/math10162862 --> Rézio, S., Andrade, M.P. and Teodoro, M.F., Problem-based learning and applied mathematics. , 2022, 10(16): 2862. https://doi.org/10.3390/math10162862 doi: |
[17] | , 2023. https://doi.org/10.1007/s13394-023-00468-8 --> Gómez-Chacón, I.M., Bacelo, A., Marbán, J.M. and Palacios, A., Inquiry-based mathematics education and attitudes towards mathematics: tracking profiles for teaching. , 2023. https://doi.org/10.1007/s13394-023-00468-8 doi: |
[18] | , 1984, 77(6): 351-359. https://doi.org/10.1080/00220671.1984.10885555 --> Darch, C., Carnine D. and Gersten, R., Explicit instruction in mathematics problem solving. , 1984, 77(6): 351-359. https://doi.org/10.1080/00220671.1984.10885555 doi: |
[19] | , 2004,104(3): 233–251. https://doi.org/10.1086/499751 --> Kroesbergen, E.H., Van Luit, J.E.H. and Maas, C.J.M., Effectiveness of explicit and constructivist mathematics instruction for low-achieving students in The Netherlands. , 2004,104(3): 233–251. https://doi.org/10.1086/499751 doi: |
[20] | , 2015,115: 303–333. https://doi.org/10.1086/679969 --> Doabler, C.T., Baker, S.K., Kosty, D.B., Smolkowski, K., Clarke, B., Miller, S.J., et al., Examining the association between explicit mathematics instruction and student mathematics achievement. , 2015,115: 303–333. https://doi.org/10.1086/679969 doi: |
[21] | , 2021, 44(2-3): 267-283. https://doi.org/10.1007/s40614-021-00291-1 --> Gunn, B., Smolkowski, K., Strycker, L.A. and Dennis, C., Measuring Explicit Instruction Using Classroom Observations of Student-Teacher Interactions (COSTI). , 2021, 44(2-3): 267-283. https://doi.org/10.1007/s40614-021-00291-1 doi: |
[22] | , April 2024. Available from: . --> Evans, T., We need to go back to teacher-led explicit instruction: maths expert. , April 2024. Available from: . |
[23] | , 2018, 5(6): 2349–5219. --> Magbanua, M.U., Explicit instruction in problem-solving skills, creative and critical thinking skills of the elementary education students. , 2018, 5(6): 2349–5219. |
[24] | , 2022, 6(5): 7781–7787. --> Joaquin, C.J.A., A guided-discovery approach to problem solving: An explicit instruction. , 2022, 6(5): 7781–7787. |
[25] | , 2021, 9(14): 1623. https://doi.org/10.3390/math9141623 --> Guo, W., Li, W. and Tisdell, C.C., Effective pedagogy of guiding undergraduate engineering students solving first-order ordinary differential equations. , 2021, 9(14): 1623. https://doi.org/10.3390/math9141623 doi: |
[26] | , 2024, 12(1): 71-84. https://doi.org/10.30935/scimath/13831 --> Guo, W., Special tutorials to support pre-service mathematics teachers learning differential equations and mathematical modelling. , 2024, 12(1): 71-84. https://doi.org/10.30935/scimath/13831 doi: |
[27] | , 2022, 2(3): 221-244. https://doi.org/10.3934/steme.2022014 --> Evans, T. and Dietrich, H., Inquiry-based mathematics education: a call for reform in tertiary education seems unjustified. , 2022, 2(3): 221-244. https://doi.org/10.3934/steme.2022014 doi: |
- This work is licensed under a Creative Commons Attribution-NonCommercial-Share Alike 4.0 Unported License. To view a copy of this license, visit http://creativecommons.org/licenses/by-nc-sa/4.0/ -->
Supplements
Access History
- Corresponding author: Email: [email protected]
Reader Comments
- © 2024 the Author(s), licensee AIMS Press. This is an open access article distributed under the terms of the Creative Commons Attribution License ( http://creativecommons.org/licenses/by/4.0 )
通讯作者: 陈斌, [email protected]
沈阳化工大学材料科学与工程学院 沈阳 110142

Article views( 56 ) PDF downloads( 5 ) Cited by( 0 )
Figures and Tables

Figures( 5 ) / Tables( 3 )
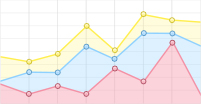
Associated material
Other articles by authors.
- William Guo
Related pages
- on Google Scholar
- Email to a friend
- Order reprints
Export File

- Figure 1. A sketch of isosceles triangle for the first word problem
- Figure 2. A reworked sketch for the second problem with derived angles (in red)
- Figure 3. The first reworked sketch for solving the second problem through right triangles
- Figure 4. The second reworked sketch for solving the second problem through right triangles
- Figure 5. The third reworked sketch for solving the second problem through right triangles
Designing STEM Learning Experiences for Youth with Lunar Trailblazer
- Miura, Jasper Kenzo
- Ehlmann, Bethany L.
- Wadhwa, Meenakshi
- Swann, Jessica L.
- Williams, David A.
- Day, Brian Hamilton
- Cass, Matthew
- Neff, Randi
NASA missions at the forefront of cutting-edge research and discovery have a unique opportunity to make mission content and resources available and accessible to inspire, engage, and educate the next generation of scientists and explorers. As a pioneering planetary science smallsat mission targeting lunar water, the Lunar Trailblazer mission is a prime subject for engaging young students in STEM. Water on the Moon is both a recent discovery and is also a readily comprehensible topic for young students. Furthermore, the Moon represents the first steps into the inspiring and largely unexplored frontier of space, yet is also visible from our own backyard. With support from the NASA SCoPE Mission Liaison grant and in collaboration with NASA Science Activation teams, we have developed mission-specific and mission-related methods and materials targeting K-8 students. Each of the first three multimedia projects was collaboratively designed over several months and addresses students in a variety of learning environments. Lunar Trailblazer Trek, an online, browser-based collaboration with NASA Solar System Treks, facilitates visualization of archetypal targets on the lunar surface that Lunar Trailblazer plans on imaging to better understand the origin and character of water on the Moon. This project lays the groundwork for future integration of Lunar Trailblazer data following launch. Hands-on activities about the lunar water cycle and how and why it is different from the water cycle on Earth were developed in collaboration with the Smoky Mountains STEM Collective to engage elementary school students in science and help develop foundational skills in critical thinking and problem solving. Finally, a digital learning curriculum was developed with Infiniscope to help students develop problem-solving skills involving models to describe, test, and predict abstract phenomena, a key Next Generation Science Standard. These projects are all accessible on an educational resource webpage specifically designed to be accessible and engaging for young students. By continuing to improve accessibility of the Lunar Trailblazer website and develop learning resources for K-8 students, we hope to engage and inspire the next generation of scientists, engineers, and problem solvers.
No Sources Found

IMAGES
VIDEO
COMMENTS
Problem-solving is a mental process that involves discovering, analyzing, and solving problems. The ultimate goal of problem-solving is to overcome obstacles and find a solution that best resolves the issue. The best strategy for solving a problem depends largely on the unique situation. In some cases, people are better off learning everything ...
Action Learning Sets put this theory into practice. When used in a business context, they bring together small groups of people to think about a problem, try out solutions, and discuss and question the results. These people repeat the Action Learning Cycle until they've developed a good solution.
Kolb's experiential learning theory works on two levels: a four-stage cycle of learning and four separate learning styles. Much of Kolb's theory is concerned with the learner's internal cognitive processes.
Problem-based learning (PBL) has been widely adopted in diverse fields and educational contexts to promote critical thinking and problem-solving in authentic learning situations.
A few months ago, I produced a video describing this the three stages of the problem-solving cycle: Understand, Strategize, and Implement. That is, we must first understand the problem, then we think of strategies that might help solve the problem, and finally we implement those strategies and see where they lead us. During two decades of observing myself and others in the teaching and ...
The study of problem solving begins with defining problem solving, problem, and problem types. This introduction to problem solving is rounded out with an examination of cognitive processes in problem solving, the role of knowledge in problem solving, and historical approaches to the study of problem solving.
Got a problem to solve? From school to relationships, we look at examples of problem-solving strategies and how to use them.
Problem-based learning (PEL) is. an instructional method in which students learn through facilitated problem. solving. In PBL, student learning centers on a complex problem that does not have a single correct answer. Students work in collaborative groups to identify what they need to learn in order to solve a problem.
Problem-solving is a vital skill for coping with various challenges in life. This webpage explains the different strategies and obstacles that can affect how you solve problems, and offers tips on how to improve your problem-solving skills. Learn how to identify, analyze, and overcome problems with Verywell Mind.
Action Learning involves a small group working on problem solving, taking action, and learning as individuals, as a team, and as an organization.
Learn how to solve problems effectively with this wide range of problem-solving tools and techniques from Mind Tools.
Problem solving is a skill that must be taught, and practiced in order to develop the skills necessary for success throughout grade school, into adulthood. We practice problem solving from a very young age. Learning how to problem solve applies to both academics, and real world situations. Students need problem solving skills to learn to ...
What Is Problem Solving? Definition and Importance Problem solving is the process of finding solutions to obstacles or challenges you encounter in your life or work. It is a crucial skill that allows you to tackle complex situations, adapt to changes, and overcome difficulties with ease. Mastering this ability will contribute to both your personal...
The learning cycle model is a constructivist instructional model in which student uses their initial understanding and skills to advance their thinking. The model offers steps that promote ...
Although problem-solving is something everyone does on a daily basis, many people lack confidence in their ability. Here we look at the basic problem-solving process to help keep you on the right track.
Make students articulate their problem solving process . In a one-on-one tutoring session, ask the student to work his/her problem out loud. This slows down the thinking process, making it more accurate and allowing you to access understanding. When working with larger groups you can ask students to provide a written "two-column solution.".
Become a better problem solver with insights and advice from leaders around the world on topics including developing a problem-solving mindset, solving problems in uncertain times, problem solving with AI, and much more.
A refined problem solving model that is easier to understand and apply. A new chapter that explores strategies for improving how schools and organizations can prepare people to think and solve problems.
Action Learning is an approach to problem solving that involves taking action and reflecting upon the results. This method is purported to help improve the problem-solving process and simplify the solutions developed as a result.
Problems often arise in organizational life. From understanding the root cause of a problem to using the power of empathy, here are five strategies for solving problems.
The cycle was based on a model of learning understood as a conceptual change and, therefore, on the constructivist theory of learning (Mayer, 2009 ). With this in mind, one could easily assume that several cognitive principles of learning had to underlie the design of the model.
The Problem-Solving Cycle (PSC) is a National Science Foundation funded project that has developed a research-based professional development (PD) model. This model is highly adaptable and can be specifically focused on problems of practice that are of interest to the participating teachers and administrators. Additionally, it can be tailored to ...
By consistently bringing fresh ideas to the table and demonstrating a proactive approach to problem-solving, they naturally attract the attention of higher-ups. ... engaging in continuous learning ...
Abstract and Figures This article focuses on the Problem-Solving Cycle (PSC), a model of professional development designed to assist teachers in supporting their students' mathematical reasoning.
The Problem-Solving Cycle (PSC) model presents one such opportunity. When teachers participate in this highly adaptable model, they become part of a professional learning community. This community engages in mathematical problem solving, examines video of classroom teaching, and shares ideas about teaching and learning mathematics. By electing ...
Collaborative problem solving (CPS) has emerged as a crucial 21st century competence that benefits students' studies, future careers, and general well-being, prevailing across disciplines and learning approaches. Given the complex and dynamic nature of CPS, teacher-facing learning analytics dashboards (LADs) have increasingly been adopted to support teachers' CPS assessments by analysing ...
Especially when it comes to manufacturing, problem-solving is an art. Every day, companies within this industry face challenges that test their processes, products and, ultimately, their bottom ...
Triangles and trigonometry are always difficult topics for both mathematics students and teachers. Hence, students' performance in solving mathematical word problems in these topics is not only a reflection of their learning outcomes but also an indication of teaching effectiveness. This case study drew from two examples of solving word problems involving triangles by pre-service mathematics ...
Hands-on activities about the lunar water cycle and how and why it is different from the water cycle on Earth were developed in collaboration with the Smoky Mountains STEM Collective to engage elementary school students in science and help develop foundational skills in critical thinking and problem solving. Finally, a digital learning ...
The impact of the urban environment on squirrels' problem-solving, learning and memory has been highlighted in new research from the UK's University of Chester and Hokkaido University in Japan.