Subscribe to the PwC Newsletter
Join the community, add a new evaluation result row, automated essay scoring.
26 papers with code • 1 benchmarks • 1 datasets
Essay scoring: Automated Essay Scoring is the task of assigning a score to an essay, usually in the context of assessing the language ability of a language learner. The quality of an essay is affected by the following four primary dimensions: topic relevance, organization and coherence, word usage and sentence complexity, and grammar and mechanics.
Source: A Joint Model for Multimodal Document Quality Assessment

Benchmarks Add a Result
Trend | Dataset | Best Model | -->Paper | Code | Compare |
---|---|---|---|---|---|
Tran-BERT-MS-ML-R | -->
Most implemented papers
Automated essay scoring based on two-stage learning.
Current state-of-art feature-engineered and end-to-end Automated Essay Score (AES) methods are proven to be unable to detect adversarial samples, e. g. the essays composed of permuted sentences and the prompt-irrelevant essays.
A Neural Approach to Automated Essay Scoring
nusnlp/nea • EMNLP 2016
SkipFlow: Incorporating Neural Coherence Features for End-to-End Automatic Text Scoring

Our new method proposes a new \textsc{SkipFlow} mechanism that models relationships between snapshots of the hidden representations of a long short-term memory (LSTM) network as it reads.
Neural Automated Essay Scoring and Coherence Modeling for Adversarially Crafted Input
Youmna-H/Coherence_AES • NAACL 2018
We demonstrate that current state-of-the-art approaches to Automated Essay Scoring (AES) are not well-suited to capturing adversarially crafted input of grammatical but incoherent sequences of sentences.
Co-Attention Based Neural Network for Source-Dependent Essay Scoring
This paper presents an investigation of using a co-attention based neural network for source-dependent essay scoring.
Language models and Automated Essay Scoring
In this paper, we present a new comparative study on automatic essay scoring (AES).
Evaluation Toolkit For Robustness Testing Of Automatic Essay Scoring Systems
midas-research/calling-out-bluff • 14 Jul 2020
This number is increasing further due to COVID-19 and the associated automation of education and testing.
Prompt Agnostic Essay Scorer: A Domain Generalization Approach to Cross-prompt Automated Essay Scoring
Cross-prompt automated essay scoring (AES) requires the system to use non target-prompt essays to award scores to a target-prompt essay.
Many Hands Make Light Work: Using Essay Traits to Automatically Score Essays
To find out which traits work best for different types of essays, we conduct ablation tests for each of the essay traits.
EXPATS: A Toolkit for Explainable Automated Text Scoring
octanove/expats • 7 Apr 2021
Automated text scoring (ATS) tasks, such as automated essay scoring and readability assessment, are important educational applications of natural language processing.

- Basics for GSIs
- Advancing Your Skills
Grading Essays
Grade for Learning Objectives Response to Writing Errors Commenting on Student Papers Plagiarism and Grading
Information about grading student writing also appears in the Grading Student Work section of the Teaching Guide. Here are some general guidelines to keep in mind when grading student writing.
Grade for Learning Objectives
Know what the objective of the assignment is and grade according to a standard (a rubric) that assesses precisely that. If the purpose of the assignment is to analyze a process, focus on the analysis in the essay. If the paper is unreadable, however, consult with the professor and other GSIs about how to proceed. It may be wise to have a shared policy about the level of readiness or comprehensibility expected and what is unacceptable.
Response to Writing Errors
The research is clear: do not even attempt to mark every error in students’ papers. There are several reasons for this. Teachers do not agree about what constitutes an error (so there is an unavoidable element of subjectivity); students do not learn when confronted by too many markings; and exhaustive marking takes way too much of the instructor’s time. Resist the urge to edit or proofread your students’ papers for superficial errors. At most, mark errors on one page or errors of only two or three types. One approach to avoid the temptation of marking every error is to read or skim the whole essay quickly once without marking anything on the page – or at least, with very minimal marks. Some instructors find this a useful method in order to get a general sense of the essay’s organization and argument, thus enabling them to better identify the major areas of concern. Your second pass can then focus more in-depth on a few select areas that require improvement.
Commenting on Student Papers
The scholarly literature in this area distinguishes formative from summative comments. Summative comments are the more traditional approach. They render judgment about an essay after it has been completed. They explain the instructor’s judgment of a student’s performance. If the instructor’s comments contain several critical statements, the student often becomes protective of his or her ego by filtering them out; learning from mistakes becomes more difficult. If the assignment is over with, the student may see no reason to revisit it to learn from the comments.
Formative comments, on the other hand, give the student feedback in an ongoing process of learning and skill building. Through formative comments, particularly in the draft stage of a writing assignment, instructors guide students on a strategic selection of the most important aspects of the essay. These include both what to keep because it is (at least relatively) well done and what requires revision. Formative comments let the student know clearly how to revise and why.
For the purposes of this guide, we have distinguished commenting on student writing (which is treated here) from grading student writing (which is treated in the Teaching Guide section on grading ). While it is true that instructors’ comments on student writing should give reasons for the grade assigned to it, we want to emphasize here that the comments on a student’s paper can function as instruction , not simply as justification. Here are ten tips.
- Use your comments on a student’s paper to highlight things the paper accomplishes well and a few major things that would most improve the paper.
- Always observe at least one or two strengths in the student’s paper, even if they seem to you to be low-level accomplishments — but avoid condescension. Writing is a complex activity, and students really do need to know they’re doing something right.
- Don’t make exhaustive comments. They take up too much of your time and leave the student with no sense of priority among them.
- Don’t proofread. If the paper is painfully replete with errors and you want to emphasize writing mechanics, count the first ten errors on the page, draw a line at that point, and ask the student to identify them and to show their corrections to you in office hours. Students do not learn much from instructors’ proofreading marks. Direct students to a writing reference guide such as the Random House Handbook.
- Notice patterns or repeated errors (in content or form). Choose the three or four most disabling ones and direct your comments toward helping the students understand what they need to learn to do differently to correct this kind of error.
- Use marginal notes to locate and comment on specific passages in the paper (for example “Interesting idea — develop it more” or “I lost the thread of the argument in this section” or “Very useful summary here before you transition to the next point”). Use final or end comments to discuss more global issues (e.g., “Work on paragraph structure” or “The argument from analogy is ineffective. A better way to make the point would be…”)
- Use questions to help the student unpack areas that are unclear or require more explanation and analysis. E.g.: “Can you explain more about what you mean by “x”?”; “What in the text shows this statement?”; “Is “y” consistent with what you’ve argued about “z”?” This approach can help the student recognize your comments less as a form of judgment than a form of dialogue with their work. As well, it can help you avoid “telling” the student how they should revise certain areas that remain undeveloped. Often, students just need a little more encouragement to focus on an area they haven’t considered in-depth or that they might have envisioned clearly in their head but did not translate to the page.
- Maintain a catalogue of positive end comments: “Good beginning for a 1B course.” “Very perceptive reading.” “Good engagement with the material.” “Gets at the most relevant material/issues/passages.” Anything that connects specific aspects of the student’s product with the grading rubric is useful. (For more on grading rubrics , see the Grading section of the Teaching Guide.)
- Diplomatic but firm suggestions for improvement: Here you must be specific and concrete. Global negative statements tend to enter students’ self-image (“I’m a bad writer”). This creates an attitudinal barrier to learning and makes your job harder and less satisfying. Instead, try “The most strategic improvement you could make is…” Again, don’t try to comment on everything. Select only the most essential areas for improvement, and watch the student’s progress on the next draft or paper.
- Typical in-text marks: Provide your students with a legend of your reading marks. Does a straight underline indicate “good stuff”? Does a wavy underline mean something different? Do you use abbreviations in the margins? You can find examples of standard editing marks in many writing guides, such as the Random House Handbook.
- The tone of your comments on student writing is important to students. Avoid sarcasm and jokes — students who take offense are less disposed to learn. Address the student by name before your end-comments, and sign your name after your remarks. Be professional, and bear in mind the sorts of comments that help you with your work.
Plagiarism and Grading
Students can be genuinely uninformed or misinformed about what constitutes plagiarism. In some instances students will knowingly resort to cutting and pasting from unacknowledged sources; a few may even pay for a paper written by someone else; more recently, students may attempt to pass off AI-generated essays as their own work. Your section syllabus should include a clear policy notice about plagiarism and AI so that students cannot miss it, and instructors should work with students to be sure they understand how to incorporate outside sources appropriately.
Plagiarism can be largely prevented by stipulating that larger writing assignments be completed in steps that the students must turn in for instructor review, or that students visit the instructor periodically for a brief but substantive chat about how their projects are developing, or that students turn in their research log and notes at intermediate points in the research process.
All of these strategies also deter students from using AI to substitute for their own critical thinking and writing. In addition, you may want to craft prompts that are specific to the course materials rather than overly-general ones; and you may also require students to provide detailed analysis about specific texts or cases. AI tools like ChatGPT tend to struggle significantly in both of these areas.
For further guidance on preventing academic misconduct, please see Academic Misconduct — Preventing Plagiarism .
You can also find more information and advice about AI technology like ChatGPT at the Berkeley Center for Teaching & Learning.
UC Berkeley has a campus license to use Turnitin to check the originality of students’ papers and to generate feedback to students about their integration of written sources into their papers. The tool is available in bCourses as an add-on to the Grading tool, and in the Assignments tool SpeedGrader. Even with the results of the originality check, instructors are obligated to exercise judgment in determining the degree to which a given use of source material was fair or unfair.
If a GSI does find a very likely instance of plagiarism, the faculty member in charge of the course must be notified and provided with the evidence. The faculty member is responsible for any sanctions against the student. Some faculty members give an automatic failing grade for the assignment or for the course, according to their own course policy. Instances of plagiarism should be reported to the Center for Student Conduct; please see If You Encounter Academic Misconduct .

- Industry News
- Beyond Multiple Choice
- 2024 Winners and Finalists
- 2023 Winners and Finalists
- 2022 Winners and Finalists
- 2021 Winners & Finalists
- Consultants Directory
- 2023 Conference Rewind Pass
- AI Special Interest Group
- Online Proctoring Special Interest Group
- Sponsorship Opportunities
Revolutionising essay grading with AI: future of assessment in education

A blog by Manjinder Kainth, PhD. CEO/CO-founder Graide
Gone are the days when teachers had to spend countless hours reading and evaluating stacks of essays. AI-powered essay grading systems are now capable of analysing and assessing a multitude of factors, such as grammar, structure, content, and more, with remarkable speed and precision. By leveraging machine learning algorithms, AI systems not only provide quick feedback to students but also enable educators to identify patterns and trends within the essays.
Furthermore, AI-based essay grading systems eliminate human biases and inconsistencies, levelling the playing field for students. These applications leverage advanced natural language processing techniques to analyse essays and provide constructive suggestions for improvement.
As technology continues to advance, AI is poised to shape the future of education, offering tremendous benefits to both educators and students. So, let’s explore how AI is revolutionising essay grading and opening up new possibilities for a more effective and personalised learning experience.
Traditional methods of essay grading
Every educator knows the drill: piles of essays waiting to be graded, hours spent poring over each one, and the constant challenge of providing meaningful feedback. Grading is an essential part of the educational process, ensuring students understand the material and receive valuable feedback to improve. However, the traditional grading system is fraught with challenges, from the sheer time it consumes to the inconsistency that can arise from human error.
The shortcomings of traditional essay grading
Traditional grading methods, while tried and tested, have inherent limitations. First and foremost, they are time-consuming. Educators often spend hours, if not days, grading a single batch of essays. This not only leads to fatigue but can also result in inconsistent grading as the teacher’s concentration wanes.
Moreover, no two educators grade identically. What one teacher might consider an ‘A’ essay, another might deem a ‘B+’. This lack of standardisation can be confusing for students and can even impact their academic trajectory.
Some might argue that the solution lies in fully automated grading systems. However, these systems often lack the nuance and understanding required to grade complex subjects, especially in subjects like literature or philosophy. They fail to capture the essence of an argument or the subtleties of a well-crafted essay. In short, while they might offer speed, they compromise on quality.
AI essay grading solution
With the traditional grading issues enters the AI essay grading system like Graide. Graide was born out of a need identified at the University of Birmingham, Graide sought to bridge the gap between speed and quality. Recognizing that fully automated solutions were falling short, the team at Graide embarked on a mission to create a system that combined the best of both worlds.
The result? An AI-driven grading system that learns from minimal data points. Instead of requiring vast amounts of data to understand and grade an essay, Graide’s system can quickly adapt and provide accurate, consistent feedback. It’s a game-changer, not just in terms of efficiency but also in the quality of feedback provided.
Case study of AI-powered essay grading
In collaboration with Oxbridge Ltd, the Graide AI essay tool was used to grade essays on complex subjects like Shakespeare and poetry. The results were nothing short of astounding. With minimal data input, the AI was able to understand and grade these intricate essays with remarkable accuracy.
For educators, this means a drastic reduction in the hours spent grading. But more than that, it promises consistent and precise feedback for students, ensuring they receive the guidance they need to improve.
For students, the benefits are manifold. With the potential for automated feedback on practice essays, they can receive feedback almost instantly, allowing for more touchpoints and opportunities to refine their skills.
Implementing AI-powered essay grading in educational institutions
To successfully implement AI-powered essay grading in educational institutions, a thoughtful and strategic approach is key. It is crucial to involve stakeholders, including teachers, students, and administrators, in the decision-making process. Their input can help identify specific needs and concerns, ensuring the successful integration of AI systems into existing educational frameworks.
Training and professional development programmes should be provided to educators to familiarise them with AI-powered grading systems. Educators need to understand the capabilities and limitations of the systems, enabling them to effectively leverage AI-generated feedback and tailor their instruction accordingly. This collaborative approach ensures that AI is used as a tool to enhance teaching and learning, rather than replace human interaction.
Additionally, ongoing monitoring and evaluation of AI systems should be conducted to ensure their effectiveness and address any unforeseen challenges. Regular feedback from educators and students can help refine and improve the algorithms, making them more accurate and reliable over time.
Final thoughts
AI is revolutionising higher education by transforming the learning experience. From personalised learning paths to intelligent tutoring systems to faster feedback, AI is reshaping traditional educational models and making education more accessible and effective. By leveraging AI, institutions can deliver personalised learning experiences, enhance student assessments and feedback, streamline administrative tasks, and gain valuable insights through learning analytics. However, as AI continues to advance, ethical considerations and challenges should be addressed to ensure fairness, privacy, and the preservation of human interaction in education.
Artificial intelligence will power education in the future. If you’re an educator or institution looking to revolutionise your grading system, to provide consistent, accurate feedback, and free up invaluable time, take a look at Graide’s AI essay grading system.

News categories
- Press Releases

Upcoming Events
The 11th world congress on education (wce-2024), european conference on educational research (ecer), eaa online proctoring user research findings.
- Privacy Policy
e-rater ® Scoring Engine
Evaluates students’ writing proficiency with automatic scoring and feedback
Selection an option below to learn more.
About the e-rater Scoring Engine
The e-rater automated scoring engine uses AI technology and Natural Language Processing (NLP) to evaluate the writing proficiency of student essays by providing automatic scoring and feedback. The engine provides descriptive feedback on the writer’s grammar, mechanics, word use and complexity, style, organization and more.
Who uses the e-rater engine and why?
Companies and institutions use this patented technology to power their custom applications.
The e-rater engine is used within the Criterion ® Online Writing Evaluation Service . Students use the e-rater engine's feedback to evaluate their essay-writing skills and to identify areas that need improvement. Teachers use the Criterion service to help their students develop their writing skills independently and receive automated, constructive feedback. The e-rater engine is also used in other low-stakes practice tests include TOEFL ® Practice Online and GRE ® ScoreItNow!™.
In high-stakes settings, the engine is used in conjunction with human ratings for both the Issue and Argument prompts of the GRE test's Analytical Writing section and the TOEFL iBT ® test's Independent and Integrated Writing prompts. ETS research has shown that combining automated and human essay scoring demonstrates assessment score reliability and measurement benefits.
For more information about the use of the e-rater engine, read E-rater as a Quality Control on Human Scores (PDF) .
How does the e-rater engine grade essays?
The e-rater engine provides a holistic score for an essay that has been entered into the computer electronically. It also provides real-time diagnostic feedback about grammar, usage, mechanics, style and organization, and development. This feedback is based on NLP research specifically tailored to the analysis of student responses and is detailed in ETS's research publications (PDF) .
How does the e-rater engine compare to human raters?
The e-rater engine uses NLP to identify features relevant to writing proficiency in training essays and their relationship with human scores. The resulting scoring model, which assigns weights to each observed feature, is stored offline in a database that can then be used to score new essays according to the same formula.
The e-rater engine doesn’t have the ability to read so it can’t evaluate essays the same way that human raters do. However, the features used in e-rater scoring have been developed to be as substantively meaningful as they can be, given the state of the art in NLP. They also have been developed to demonstrate strong reliability — often greater reliability than human raters themselves.
Learn more about how it works .
About Natural Language Processing
The e-rater engine is an artificial intelligence engine that uses Natural Language Processing (NLP), a field of computer science and linguistics that uses computational methods to analyze characteristics of a text. NLP methods support such burgeoning application areas as machine translation, speech recognition and information retrieval.
Ready to begin? Contact us to learn how the e-rater service can enhance your existing program.

Grading System Essays
Us and uk education system, popular essay topics.
- American Dream
- Artificial Intelligence
- Black Lives Matter
- Bullying Essay
- Career Goals Essay
- Causes of the Civil War
- Child Abusing
- Civil Rights Movement
- Community Service
- Cultural Identity
- Cyber Bullying
- Death Penalty
- Depression Essay
- Domestic Violence
- Freedom of Speech
- Global Warming
- Gun Control
- Human Trafficking
- I Believe Essay
- Immigration
- Importance of Education
- Israel and Palestine Conflict
- Leadership Essay
- Legalizing Marijuanas
- Mental Health
- National Honor Society
- Police Brutality
- Pollution Essay
- Racism Essay
- Romeo and Juliet
- Same Sex Marriages
- Social Media
- The Great Gatsby
- The Yellow Wallpaper
- Time Management
- To Kill a Mockingbird
- Violent Video Games
- What Makes You Unique
- Why I Want to Be a Nurse
- Send us an e-mail

An official website of the United States government
The .gov means it’s official. Federal government websites often end in .gov or .mil. Before sharing sensitive information, make sure you’re on a federal government site.
The site is secure. The https:// ensures that you are connecting to the official website and that any information you provide is encrypted and transmitted securely.
- Publications
- Account settings
Preview improvements coming to the PMC website in October 2024. Learn More or Try it out now .
- Advanced Search
- Journal List
- Springer Nature - PMC COVID-19 Collection

An automated essay scoring systems: a systematic literature review
Dadi ramesh.
1 School of Computer Science and Artificial Intelligence, SR University, Warangal, TS India
2 Research Scholar, JNTU, Hyderabad, India
Suresh Kumar Sanampudi
3 Department of Information Technology, JNTUH College of Engineering, Nachupally, Kondagattu, Jagtial, TS India
Associated Data
Assessment in the Education system plays a significant role in judging student performance. The present evaluation system is through human assessment. As the number of teachers' student ratio is gradually increasing, the manual evaluation process becomes complicated. The drawback of manual evaluation is that it is time-consuming, lacks reliability, and many more. This connection online examination system evolved as an alternative tool for pen and paper-based methods. Present Computer-based evaluation system works only for multiple-choice questions, but there is no proper evaluation system for grading essays and short answers. Many researchers are working on automated essay grading and short answer scoring for the last few decades, but assessing an essay by considering all parameters like the relevance of the content to the prompt, development of ideas, Cohesion, and Coherence is a big challenge till now. Few researchers focused on Content-based evaluation, while many of them addressed style-based assessment. This paper provides a systematic literature review on automated essay scoring systems. We studied the Artificial Intelligence and Machine Learning techniques used to evaluate automatic essay scoring and analyzed the limitations of the current studies and research trends. We observed that the essay evaluation is not done based on the relevance of the content and coherence.
Supplementary Information
The online version contains supplementary material available at 10.1007/s10462-021-10068-2.
Introduction
Due to COVID 19 outbreak, an online educational system has become inevitable. In the present scenario, almost all the educational institutions ranging from schools to colleges adapt the online education system. The assessment plays a significant role in measuring the learning ability of the student. Most automated evaluation is available for multiple-choice questions, but assessing short and essay answers remain a challenge. The education system is changing its shift to online-mode, like conducting computer-based exams and automatic evaluation. It is a crucial application related to the education domain, which uses natural language processing (NLP) and Machine Learning techniques. The evaluation of essays is impossible with simple programming languages and simple techniques like pattern matching and language processing. Here the problem is for a single question, we will get more responses from students with a different explanation. So, we need to evaluate all the answers concerning the question.
Automated essay scoring (AES) is a computer-based assessment system that automatically scores or grades the student responses by considering appropriate features. The AES research started in 1966 with the Project Essay Grader (PEG) by Ajay et al. ( 1973 ). PEG evaluates the writing characteristics such as grammar, diction, construction, etc., to grade the essay. A modified version of the PEG by Shermis et al. ( 2001 ) was released, which focuses on grammar checking with a correlation between human evaluators and the system. Foltz et al. ( 1999 ) introduced an Intelligent Essay Assessor (IEA) by evaluating content using latent semantic analysis to produce an overall score. Powers et al. ( 2002 ) proposed E-rater and Intellimetric by Rudner et al. ( 2006 ) and Bayesian Essay Test Scoring System (BESTY) by Rudner and Liang ( 2002 ), these systems use natural language processing (NLP) techniques that focus on style and content to obtain the score of an essay. The vast majority of the essay scoring systems in the 1990s followed traditional approaches like pattern matching and a statistical-based approach. Since the last decade, the essay grading systems started using regression-based and natural language processing techniques. AES systems like Dong et al. ( 2017 ) and others developed from 2014 used deep learning techniques, inducing syntactic and semantic features resulting in better results than earlier systems.
Ohio, Utah, and most US states are using AES systems in school education, like Utah compose tool, Ohio standardized test (an updated version of PEG), evaluating millions of student's responses every year. These systems work for both formative, summative assessments and give feedback to students on the essay. Utah provided basic essay evaluation rubrics (six characteristics of essay writing): Development of ideas, organization, style, word choice, sentence fluency, conventions. Educational Testing Service (ETS) has been conducting significant research on AES for more than a decade and designed an algorithm to evaluate essays on different domains and providing an opportunity for test-takers to improve their writing skills. In addition, they are current research content-based evaluation.
The evaluation of essay and short answer scoring should consider the relevance of the content to the prompt, development of ideas, Cohesion, Coherence, and domain knowledge. Proper assessment of the parameters mentioned above defines the accuracy of the evaluation system. But all these parameters cannot play an equal role in essay scoring and short answer scoring. In a short answer evaluation, domain knowledge is required, like the meaning of "cell" in physics and biology is different. And while evaluating essays, the implementation of ideas with respect to prompt is required. The system should also assess the completeness of the responses and provide feedback.
Several studies examined AES systems, from the initial to the latest AES systems. In which the following studies on AES systems are Blood ( 2011 ) provided a literature review from PEG 1984–2010. Which has covered only generalized parts of AES systems like ethical aspects, the performance of the systems. Still, they have not covered the implementation part, and it’s not a comparative study and has not discussed the actual challenges of AES systems.
Burrows et al. ( 2015 ) Reviewed AES systems on six dimensions like dataset, NLP techniques, model building, grading models, evaluation, and effectiveness of the model. They have not covered feature extraction techniques and challenges in features extractions. Covered only Machine Learning models but not in detail. This system not covered the comparative analysis of AES systems like feature extraction, model building, and level of relevance, cohesion, and coherence not covered in this review.
Ke et al. ( 2019 ) provided a state of the art of AES system but covered very few papers and not listed all challenges, and no comparative study of the AES model. On the other hand, Hussein et al. in ( 2019 ) studied two categories of AES systems, four papers from handcrafted features for AES systems, and four papers from the neural networks approach, discussed few challenges, and did not cover feature extraction techniques, the performance of AES models in detail.
Klebanov et al. ( 2020 ). Reviewed 50 years of AES systems, listed and categorized all essential features that need to be extracted from essays. But not provided a comparative analysis of all work and not discussed the challenges.
This paper aims to provide a systematic literature review (SLR) on automated essay grading systems. An SLR is an Evidence-based systematic review to summarize the existing research. It critically evaluates and integrates all relevant studies' findings and addresses the research domain's specific research questions. Our research methodology uses guidelines given by Kitchenham et al. ( 2009 ) for conducting the review process; provide a well-defined approach to identify gaps in current research and to suggest further investigation.
We addressed our research method, research questions, and the selection process in Sect. 2 , and the results of the research questions have discussed in Sect. 3 . And the synthesis of all the research questions addressed in Sect. 4 . Conclusion and possible future work discussed in Sect. 5 .
Research method
We framed the research questions with PICOC criteria.
Population (P) Student essays and answers evaluation systems.
Intervention (I) evaluation techniques, data sets, features extraction methods.
Comparison (C) Comparison of various approaches and results.
Outcomes (O) Estimate the accuracy of AES systems,
Context (C) NA.
Research questions
To collect and provide research evidence from the available studies in the domain of automated essay grading, we framed the following research questions (RQ):
RQ1 what are the datasets available for research on automated essay grading?
The answer to the question can provide a list of the available datasets, their domain, and access to the datasets. It also provides a number of essays and corresponding prompts.
RQ2 what are the features extracted for the assessment of essays?
The answer to the question can provide an insight into various features so far extracted, and the libraries used to extract those features.
RQ3, which are the evaluation metrics available for measuring the accuracy of algorithms?
The answer will provide different evaluation metrics for accurate measurement of each Machine Learning approach and commonly used measurement technique.
RQ4 What are the Machine Learning techniques used for automatic essay grading, and how are they implemented?
It can provide insights into various Machine Learning techniques like regression models, classification models, and neural networks for implementing essay grading systems. The response to the question can give us different assessment approaches for automated essay grading systems.
RQ5 What are the challenges/limitations in the current research?
The answer to the question provides limitations of existing research approaches like cohesion, coherence, completeness, and feedback.
Search process
We conducted an automated search on well-known computer science repositories like ACL, ACM, IEEE Explore, Springer, and Science Direct for an SLR. We referred to papers published from 2010 to 2020 as much of the work during these years focused on advanced technologies like deep learning and natural language processing for automated essay grading systems. Also, the availability of free data sets like Kaggle (2012), Cambridge Learner Corpus-First Certificate in English exam (CLC-FCE) by Yannakoudakis et al. ( 2011 ) led to research this domain.
Search Strings : We used search strings like “Automated essay grading” OR “Automated essay scoring” OR “short answer scoring systems” OR “essay scoring systems” OR “automatic essay evaluation” and searched on metadata.
Selection criteria
After collecting all relevant documents from the repositories, we prepared selection criteria for inclusion and exclusion of documents. With the inclusion and exclusion criteria, it becomes more feasible for the research to be accurate and specific.
Inclusion criteria 1 Our approach is to work with datasets comprise of essays written in English. We excluded the essays written in other languages.
Inclusion criteria 2 We included the papers implemented on the AI approach and excluded the traditional methods for the review.
Inclusion criteria 3 The study is on essay scoring systems, so we exclusively included the research carried out on only text data sets rather than other datasets like image or speech.
Exclusion criteria We removed the papers in the form of review papers, survey papers, and state of the art papers.
Quality assessment
In addition to the inclusion and exclusion criteria, we assessed each paper by quality assessment questions to ensure the article's quality. We included the documents that have clearly explained the approach they used, the result analysis and validation.
The quality checklist questions are framed based on the guidelines from Kitchenham et al. ( 2009 ). Each quality assessment question was graded as either 1 or 0. The final score of the study range from 0 to 3. A cut off score for excluding a study from the review is 2 points. Since the papers scored 2 or 3 points are included in the final evaluation. We framed the following quality assessment questions for the final study.
Quality Assessment 1: Internal validity.
Quality Assessment 2: External validity.
Quality Assessment 3: Bias.
The two reviewers review each paper to select the final list of documents. We used the Quadratic Weighted Kappa score to measure the final agreement between the two reviewers. The average resulted from the kappa score is 0.6942, a substantial agreement between the reviewers. The result of evolution criteria shown in Table Table1. 1 . After Quality Assessment, the final list of papers for review is shown in Table Table2. 2 . The complete selection process is shown in Fig. Fig.1. 1 . The total number of selected papers in year wise as shown in Fig. Fig.2. 2 .
Quality assessment analysis
Number of papers | Quality assessment score |
---|---|
50 | 3 |
12 | 2 |
59 | 1 |
23 | 0 |
Final list of papers
Data base | Paper count |
---|---|
ACL | 28 |
ACM | 5 |
IEEE Explore | 19 |
Springer | 5 |
Other | 5 |
Total | 62 |

Selection process

Year wise publications
What are the datasets available for research on automated essay grading?
To work with problem statement especially in Machine Learning and deep learning domain, we require considerable amount of data to train the models. To answer this question, we listed all the data sets used for training and testing for automated essay grading systems. The Cambridge Learner Corpus-First Certificate in English exam (CLC-FCE) Yannakoudakis et al. ( 2011 ) developed corpora that contain 1244 essays and ten prompts. This corpus evaluates whether a student can write the relevant English sentences without any grammatical and spelling mistakes. This type of corpus helps to test the models built for GRE and TOFEL type of exams. It gives scores between 1 and 40.
Bailey and Meurers ( 2008 ), Created a dataset (CREE reading comprehension) for language learners and automated short answer scoring systems. The corpus consists of 566 responses from intermediate students. Mohler and Mihalcea ( 2009 ). Created a dataset for the computer science domain consists of 630 responses for data structure assignment questions. The scores are range from 0 to 5 given by two human raters.
Dzikovska et al. ( 2012 ) created a Student Response Analysis (SRA) corpus. It consists of two sub-groups: the BEETLE corpus consists of 56 questions and approximately 3000 responses from students in the electrical and electronics domain. The second one is the SCIENTSBANK(SemEval-2013) (Dzikovska et al. 2013a ; b ) corpus consists of 10,000 responses on 197 prompts on various science domains. The student responses ladled with "correct, partially correct incomplete, Contradictory, Irrelevant, Non-domain."
In the Kaggle (2012) competition, released total 3 types of corpuses on an Automated Student Assessment Prize (ASAP1) (“ https://www.kaggle.com/c/asap-sas/ ” ) essays and short answers. It has nearly 17,450 essays, out of which it provides up to 3000 essays for each prompt. It has eight prompts that test 7th to 10th grade US students. It gives scores between the [0–3] and [0–60] range. The limitations of these corpora are: (1) it has a different score range for other prompts. (2) It uses statistical features such as named entities extraction and lexical features of words to evaluate essays. ASAP + + is one more dataset from Kaggle. It is with six prompts, and each prompt has more than 1000 responses total of 10,696 from 8th-grade students. Another corpus contains ten prompts from science, English domains and a total of 17,207 responses. Two human graders evaluated all these responses.
Correnti et al. ( 2013 ) created a Response-to-Text Assessment (RTA) dataset used to check student writing skills in all directions like style, mechanism, and organization. 4–8 grade students give the responses to RTA. Basu et al. ( 2013 ) created a power grading dataset with 700 responses for ten different prompts from US immigration exams. It contains all short answers for assessment.
The TOEFL11 corpus Blanchard et al. ( 2013 ) contains 1100 essays evenly distributed over eight prompts. It is used to test the English language skills of a candidate attending the TOFEL exam. It scores the language proficiency of a candidate as low, medium, and high.
International Corpus of Learner English (ICLE) Granger et al. ( 2009 ) built a corpus of 3663 essays covering different dimensions. It has 12 prompts with 1003 essays that test the organizational skill of essay writing, and13 prompts, each with 830 essays that examine the thesis clarity and prompt adherence.
Argument Annotated Essays (AAE) Stab and Gurevych ( 2014 ) developed a corpus that contains 102 essays with 101 prompts taken from the essayforum2 site. It tests the persuasive nature of the student essay. The SCIENTSBANK corpus used by Sakaguchi et al. ( 2015 ) available in git-hub, containing 9804 answers to 197 questions in 15 science domains. Table Table3 3 illustrates all datasets related to AES systems.
ALL types Datasets used in Automatic scoring systems
Data Set | Language | Total responses | Number of prompts |
---|---|---|---|
Cambridge Learner Corpus-First Certificate in English exam (CLC-FCE) | English | 1244 | |
CREE | English | 566 | |
CS | English | 630 | |
SRA | English | 3000 | 56 |
SCIENTSBANK(SemEval-2013) | English | 10,000 | 197 |
ASAP-AES | English | 17,450 | 8 |
ASAP-SAS | English | 17,207 | 10 |
ASAP + + | English | 10,696 | 6 |
power grading | English | 700 | |
TOEFL11 | English | 1100 | 8 |
International Corpus of Learner English (ICLE) | English | 3663 |
Features play a major role in the neural network and other supervised Machine Learning approaches. The automatic essay grading systems scores student essays based on different types of features, which play a prominent role in training the models. Based on their syntax and semantics and they are categorized into three groups. 1. statistical-based features Contreras et al. ( 2018 ); Kumar et al. ( 2019 ); Mathias and Bhattacharyya ( 2018a ; b ) 2. Style-based (Syntax) features Cummins et al. ( 2016 ); Darwish and Mohamed ( 2020 ); Ke et al. ( 2019 ). 3. Content-based features Dong et al. ( 2017 ). A good set of features appropriate models evolved better AES systems. The vast majority of the researchers are using regression models if features are statistical-based. For Neural Networks models, researches are using both style-based and content-based features. The following table shows the list of various features used in existing AES Systems. Table Table4 4 represents all set of features used for essay grading.
Types of features
Statistical features | Style based features | Content based features |
---|---|---|
Essay length with respect to the number of words | Sentence structure | Cohesion between sentences in a document |
Essay length with respect to sentence | POS | Overlapping (prompt) |
Average sentence length | Punctuation | Relevance of information |
Average word length | Grammatical | Semantic role of words |
N-gram | Logical operators | Correctness |
Vocabulary | Consistency | |
Sentence expressing key concepts |
We studied all the feature extracting NLP libraries as shown in Fig. Fig.3. that 3 . that are used in the papers. The NLTK is an NLP tool used to retrieve statistical features like POS, word count, sentence count, etc. With NLTK, we can miss the essay's semantic features. To find semantic features Word2Vec Mikolov et al. ( 2013 ), GloVe Jeffrey Pennington et al. ( 2014 ) is the most used libraries to retrieve the semantic text from the essays. And in some systems, they directly trained the model with word embeddings to find the score. From Fig. Fig.4 4 as observed that non-content-based feature extraction is higher than content-based.

Usages of tools

Number of papers on content based features
RQ3 which are the evaluation metrics available for measuring the accuracy of algorithms?
The majority of the AES systems are using three evaluation metrics. They are (1) quadrated weighted kappa (QWK) (2) Mean Absolute Error (MAE) (3) Pearson Correlation Coefficient (PCC) Shehab et al. ( 2016 ). The quadratic weighted kappa will find agreement between human evaluation score and system evaluation score and produces value ranging from 0 to 1. And the Mean Absolute Error is the actual difference between human-rated score to system-generated score. The mean square error (MSE) measures the average squares of the errors, i.e., the average squared difference between the human-rated and the system-generated scores. MSE will always give positive numbers only. Pearson's Correlation Coefficient (PCC) finds the correlation coefficient between two variables. It will provide three values (0, 1, − 1). "0" represents human-rated and system scores that are not related. "1" represents an increase in the two scores. "− 1" illustrates a negative relationship between the two scores.
RQ4 what are the Machine Learning techniques being used for automatic essay grading, and how are they implemented?
After scrutinizing all documents, we categorize the techniques used in automated essay grading systems into four baskets. 1. Regression techniques. 2. Classification model. 3. Neural networks. 4. Ontology-based approach.
All the existing AES systems developed in the last ten years employ supervised learning techniques. Researchers using supervised methods viewed the AES system as either regression or classification task. The goal of the regression task is to predict the score of an essay. The classification task is to classify the essays belonging to (low, medium, or highly) relevant to the question's topic. Since the last three years, most AES systems developed made use of the concept of the neural network.
Regression based models
Mohler and Mihalcea ( 2009 ). proposed text-to-text semantic similarity to assign a score to the student essays. There are two text similarity measures like Knowledge-based measures, corpus-based measures. There eight knowledge-based tests with all eight models. They found the similarity. The shortest path similarity determines based on the length, which shortest path between two contexts. Leacock & Chodorow find the similarity based on the shortest path's length between two concepts using node-counting. The Lesk similarity finds the overlap between the corresponding definitions, and Wu & Palmer algorithm finds similarities based on the depth of two given concepts in the wordnet taxonomy. Resnik, Lin, Jiang&Conrath, Hirst& St-Onge find the similarity based on different parameters like the concept, probability, normalization factor, lexical chains. In corpus-based likeness, there LSA BNC, LSA Wikipedia, and ESA Wikipedia, latent semantic analysis is trained on Wikipedia and has excellent domain knowledge. Among all similarity scores, correlation scores LSA Wikipedia scoring accuracy is more. But these similarity measure algorithms are not using NLP concepts. These models are before 2010 and basic concept models to continue the research automated essay grading with updated algorithms on neural networks with content-based features.
Adamson et al. ( 2014 ) proposed an automatic essay grading system which is a statistical-based approach in this they retrieved features like POS, Character count, Word count, Sentence count, Miss spelled words, n-gram representation of words to prepare essay vector. They formed a matrix with these all vectors in that they applied LSA to give a score to each essay. It is a statistical approach that doesn’t consider the semantics of the essay. The accuracy they got when compared to the human rater score with the system is 0.532.
Cummins et al. ( 2016 ). Proposed Timed Aggregate Perceptron vector model to give ranking to all the essays, and later they converted the rank algorithm to predict the score of the essay. The model trained with features like Word unigrams, bigrams, POS, Essay length, grammatical relation, Max word length, sentence length. It is multi-task learning, gives ranking to the essays, and predicts the score for the essay. The performance evaluated through QWK is 0.69, a substantial agreement between the human rater and the system.
Sultan et al. ( 2016 ). Proposed a Ridge regression model to find short answer scoring with Question Demoting. Question Demoting is the new concept included in the essay's final assessment to eliminate duplicate words from the essay. The extracted features are Text Similarity, which is the similarity between the student response and reference answer. Question Demoting is the number of repeats in a student response. With inverse document frequency, they assigned term weight. The sentence length Ratio is the number of words in the student response, is another feature. With these features, the Ridge regression model was used, and the accuracy they got 0.887.
Contreras et al. ( 2018 ). Proposed Ontology based on text mining in this model has given a score for essays in phases. In phase-I, they generated ontologies with ontoGen and SVM to find the concept and similarity in the essay. In phase II from ontologies, they retrieved features like essay length, word counts, correctness, vocabulary, and types of word used, domain information. After retrieving statistical data, they used a linear regression model to find the score of the essay. The accuracy score is the average of 0.5.
Darwish and Mohamed ( 2020 ) proposed the fusion of fuzzy Ontology with LSA. They retrieve two types of features, like syntax features and semantic features. In syntax features, they found Lexical Analysis with tokens, and they construct a parse tree. If the parse tree is broken, the essay is inconsistent—a separate grade assigned to the essay concerning syntax features. The semantic features are like similarity analysis, Spatial Data Analysis. Similarity analysis is to find duplicate sentences—Spatial Data Analysis for finding Euclid distance between the center and part. Later they combine syntax features and morphological features score for the final score. The accuracy they achieved with the multiple linear regression model is 0.77, mostly on statistical features.
Süzen Neslihan et al. ( 2020 ) proposed a text mining approach for short answer grading. First, their comparing model answers with student response by calculating the distance between two sentences. By comparing the model answer with student response, they find the essay's completeness and provide feedback. In this approach, model vocabulary plays a vital role in grading, and with this model vocabulary, the grade will be assigned to the student's response and provides feedback. The correlation between the student answer to model answer is 0.81.
Classification based Models
Persing and Ng ( 2013 ) used a support vector machine to score the essay. The features extracted are OS, N-gram, and semantic text to train the model and identified the keywords from the essay to give the final score.
Sakaguchi et al. ( 2015 ) proposed two methods: response-based and reference-based. In response-based scoring, the extracted features are response length, n-gram model, and syntactic elements to train the support vector regression model. In reference-based scoring, features such as sentence similarity using word2vec is used to find the cosine similarity of the sentences that is the final score of the response. First, the scores were discovered individually and later combined two features to find a final score. This system gave a remarkable increase in performance by combining the scores.
Mathias and Bhattacharyya ( 2018a ; b ) Proposed Automated Essay Grading Dataset with Essay Attribute Scores. The first concept features selection depends on the essay type. So the common attributes are Content, Organization, Word Choice, Sentence Fluency, Conventions. In this system, each attribute is scored individually, with the strength of each attribute identified. The model they used is a random forest classifier to assign scores to individual attributes. The accuracy they got with QWK is 0.74 for prompt 1 of the ASAS dataset ( https://www.kaggle.com/c/asap-sas/ ).
Ke et al. ( 2019 ) used a support vector machine to find the response score. In this method, features like Agreeability, Specificity, Clarity, Relevance to prompt, Conciseness, Eloquence, Confidence, Direction of development, Justification of opinion, and Justification of importance. First, the individual parameter score obtained was later combined with all scores to give a final response score. The features are used in the neural network to find whether the sentence is relevant to the topic or not.
Salim et al. ( 2019 ) proposed an XGBoost Machine Learning classifier to assess the essays. The algorithm trained on features like word count, POS, parse tree depth, and coherence in the articles with sentence similarity percentage; cohesion and coherence are considered for training. And they implemented K-fold cross-validation for a result the average accuracy after specific validations is 68.12.
Neural network models
Shehab et al. ( 2016 ) proposed a neural network method that used learning vector quantization to train human scored essays. After training, the network can provide a score to the ungraded essays. First, we should process the essay to remove Spell checking and then perform preprocessing steps like Document Tokenization, stop word removal, Stemming, and submit it to the neural network. Finally, the model will provide feedback on the essay, whether it is relevant to the topic. And the correlation coefficient between human rater and system score is 0.7665.
Kopparapu and De ( 2016 ) proposed the Automatic Ranking of Essays using Structural and Semantic Features. This approach constructed a super essay with all the responses. Next, ranking for a student essay is done based on the super-essay. The structural and semantic features derived helps to obtain the scores. In a paragraph, 15 Structural features like an average number of sentences, the average length of sentences, and the count of words, nouns, verbs, adjectives, etc., are used to obtain a syntactic score. A similarity score is used as semantic features to calculate the overall score.
Dong and Zhang ( 2016 ) proposed a hierarchical CNN model. The model builds two layers with word embedding to represents the words as the first layer. The second layer is a word convolution layer with max-pooling to find word vectors. The next layer is a sentence-level convolution layer with max-pooling to find the sentence's content and synonyms. A fully connected dense layer produces an output score for an essay. The accuracy with the hierarchical CNN model resulted in an average QWK of 0.754.
Taghipour and Ng ( 2016 ) proposed a first neural approach for essay scoring build in which convolution and recurrent neural network concepts help in scoring an essay. The network uses a lookup table with the one-hot representation of the word vector of an essay. The final efficiency of the network model with LSTM resulted in an average QWK of 0.708.
Dong et al. ( 2017 ). Proposed an Attention-based scoring system with CNN + LSTM to score an essay. For CNN, the input parameters were character embedding and word embedding, and it has attention pooling layers and used NLTK to obtain word and character embedding. The output gives a sentence vector, which provides sentence weight. After CNN, it will have an LSTM layer with an attention pooling layer, and this final layer results in the final score of the responses. The average QWK score is 0.764.
Riordan et al. ( 2017 ) proposed a neural network with CNN and LSTM layers. Word embedding, given as input to a neural network. An LSTM network layer will retrieve the window features and delivers them to the aggregation layer. The aggregation layer is a superficial layer that takes a correct window of words and gives successive layers to predict the answer's sore. The accuracy of the neural network resulted in a QWK of 0.90.
Zhao et al. ( 2017 ) proposed a new concept called Memory-Augmented Neural network with four layers, input representation layer, memory addressing layer, memory reading layer, and output layer. An input layer represents all essays in a vector form based on essay length. After converting the word vector, the memory addressing layer takes a sample of the essay and weighs all the terms. The memory reading layer takes the input from memory addressing segment and finds the content to finalize the score. Finally, the output layer will provide the final score of the essay. The accuracy of essay scores is 0.78, which is far better than the LSTM neural network.
Mathias and Bhattacharyya ( 2018a ; b ) proposed deep learning networks using LSTM with the CNN layer and GloVe pre-trained word embeddings. For this, they retrieved features like Sentence count essays, word count per sentence, Number of OOVs in the sentence, Language model score, and the text's perplexity. The network predicted the goodness scores of each essay. The higher the goodness scores, means higher the rank and vice versa.
Nguyen and Dery ( 2016 ). Proposed Neural Networks for Automated Essay Grading. In this method, a single layer bi-directional LSTM accepting word vector as input. Glove vectors used in this method resulted in an accuracy of 90%.
Ruseti et al. ( 2018 ) proposed a recurrent neural network that is capable of memorizing the text and generate a summary of an essay. The Bi-GRU network with the max-pooling layer molded on the word embedding of each document. It will provide scoring to the essay by comparing it with a summary of the essay from another Bi-GRU network. The result obtained an accuracy of 0.55.
Wang et al. ( 2018a ; b ) proposed an automatic scoring system with the bi-LSTM recurrent neural network model and retrieved the features using the word2vec technique. This method generated word embeddings from the essay words using the skip-gram model. And later, word embedding is used to train the neural network to find the final score. The softmax layer in LSTM obtains the importance of each word. This method used a QWK score of 0.83%.
Dasgupta et al. ( 2018 ) proposed a technique for essay scoring with augmenting textual qualitative Features. It extracted three types of linguistic, cognitive, and psychological features associated with a text document. The linguistic features are Part of Speech (POS), Universal Dependency relations, Structural Well-formedness, Lexical Diversity, Sentence Cohesion, Causality, and Informativeness of the text. The psychological features derived from the Linguistic Information and Word Count (LIWC) tool. They implemented a convolution recurrent neural network that takes input as word embedding and sentence vector, retrieved from the GloVe word vector. And the second layer is the Convolution Layer to find local features. The next layer is the recurrent neural network (LSTM) to find corresponding of the text. The accuracy of this method resulted in an average QWK of 0.764.
Liang et al. ( 2018 ) proposed a symmetrical neural network AES model with Bi-LSTM. They are extracting features from sample essays and student essays and preparing an embedding layer as input. The embedding layer output is transfer to the convolution layer from that LSTM will be trained. Hear the LSRM model has self-features extraction layer, which will find the essay's coherence. The average QWK score of SBLSTMA is 0.801.
Liu et al. ( 2019 ) proposed two-stage learning. In the first stage, they are assigning a score based on semantic data from the essay. The second stage scoring is based on some handcrafted features like grammar correction, essay length, number of sentences, etc. The average score of the two stages is 0.709.
Pedro Uria Rodriguez et al. ( 2019 ) proposed a sequence-to-sequence learning model for automatic essay scoring. They used BERT (Bidirectional Encoder Representations from Transformers), which extracts the semantics from a sentence from both directions. And XLnet sequence to sequence learning model to extract features like the next sentence in an essay. With this pre-trained model, they attained coherence from the essay to give the final score. The average QWK score of the model is 75.5.
Xia et al. ( 2019 ) proposed a two-layer Bi-directional LSTM neural network for the scoring of essays. The features extracted with word2vec to train the LSTM and accuracy of the model in an average of QWK is 0.870.
Kumar et al. ( 2019 ) Proposed an AutoSAS for short answer scoring. It used pre-trained Word2Vec and Doc2Vec models trained on Google News corpus and Wikipedia dump, respectively, to retrieve the features. First, they tagged every word POS and they found weighted words from the response. It also found prompt overlap to observe how the answer is relevant to the topic, and they defined lexical overlaps like noun overlap, argument overlap, and content overlap. This method used some statistical features like word frequency, difficulty, diversity, number of unique words in each response, type-token ratio, statistics of the sentence, word length, and logical operator-based features. This method uses a random forest model to train the dataset. The data set has sample responses with their associated score. The model will retrieve the features from both responses like graded and ungraded short answers with questions. The accuracy of AutoSAS with QWK is 0.78. It will work on any topics like Science, Arts, Biology, and English.
Jiaqi Lun et al. ( 2020 ) proposed an automatic short answer scoring with BERT. In this with a reference answer comparing student responses and assigning scores. The data augmentation is done with a neural network and with one correct answer from the dataset classifying reaming responses as correct or incorrect.
Zhu and Sun ( 2020 ) proposed a multimodal Machine Learning approach for automated essay scoring. First, they count the grammar score with the spaCy library and numerical count as the number of words and sentences with the same library. With this input, they trained a single and Bi LSTM neural network for finding the final score. For the LSTM model, they prepared sentence vectors with GloVe and word embedding with NLTK. Bi-LSTM will check each sentence in both directions to find semantic from the essay. The average QWK score with multiple models is 0.70.
Ontology based approach
Mohler et al. ( 2011 ) proposed a graph-based method to find semantic similarity in short answer scoring. For the ranking of answers, they used the support vector regression model. The bag of words is the main feature extracted in the system.
Ramachandran et al. ( 2015 ) also proposed a graph-based approach to find lexical based semantics. Identified phrase patterns and text patterns are the features to train a random forest regression model to score the essays. The accuracy of the model in a QWK is 0.78.
Zupanc et al. ( 2017 ) proposed sentence similarity networks to find the essay's score. Ajetunmobi and Daramola ( 2017 ) recommended an ontology-based information extraction approach and domain-based ontology to find the score.
Speech response scoring
Automatic scoring is in two ways one is text-based scoring, other is speech-based scoring. This paper discussed text-based scoring and its challenges, and now we cover speech scoring and common points between text and speech-based scoring. Evanini and Wang ( 2013 ), Worked on speech scoring of non-native school students, extracted features with speech ratter, and trained a linear regression model, concluding that accuracy varies based on voice pitching. Loukina et al. ( 2015 ) worked on feature selection from speech data and trained SVM. Malinin et al. ( 2016 ) used neural network models to train the data. Loukina et al. ( 2017 ). Proposed speech and text-based automatic scoring. Extracted text-based features, speech-based features and trained a deep neural network for speech-based scoring. They extracted 33 types of features based on acoustic signals. Malinin et al. ( 2017 ). Wu Xixin et al. ( 2020 ) Worked on deep neural networks for spoken language assessment. Incorporated different types of models and tested them. Ramanarayanan et al. ( 2017 ) worked on feature extraction methods and extracted punctuation, fluency, and stress and trained different Machine Learning models for scoring. Knill et al. ( 2018 ). Worked on Automatic speech recognizer and its errors how its impacts the speech assessment.

The state of the art
This section provides an overview of the existing AES systems with a comparative study w. r. t models, features applied, datasets, and evaluation metrics used for building the automated essay grading systems. We divided all 62 papers into two sets of the first set of review papers in Table Table5 5 with a comparative study of the AES systems.
State of the art
System | Approach | Dataset | Features applied | Evaluation metric and results |
---|---|---|---|---|
Mohler and Mihalcea in ( ) | shortest path similarity, LSA regression model | Word vector | Finds the shortest path | |
Niraj Kumar and Lipika Dey. In ( ) | Word-Graph | ASAP Kaggle | Content and style-based features | 63.81% accuracy |
Alex Adamson et al. in ( ) | LSA regression model | ASAP Kaggle | Statistical features | QWK 0.532 |
Nguyen and Dery ( ) | LSTM (single layer bidirectional) | ASAP Kaggle | Statistical features | 90% accuracy |
Keisuke Sakaguchi et al. in ( ) | Classification model | ETS (educational testing services) | Statistical, Style based features | QWK is 0.69 |
Ramachandran et al. in ( ) | regression model | ASAP Kaggle short Answer | Statistical and style-based features | QWK 0.77 |
Sultan et al. in ( ) | Ridge regression model | SciEntBank answers | Statistical features | RMSE 0.887 |
Dong and Zhang ( ) | CNN neural network | ASAP Kaggle | Statistical features | QWK 0.734 |
Taghipour and Ngl in ( ) | CNN + LSTM neural network | ASAP Kaggle | Lookup table (one hot representation of word vector) | QWK 0.761 |
Shehab et al. in ( ) | Learning vector quantization neural network | Mansoura University student's essays | Statistical features | correlation coefficient 0.7665 |
Cummins et al. in ( ) | Regression model | ASAP Kaggle | Statistical features, style-based features | QWK 0.69 |
Kopparapu and De ( ) | Neural network | ASAP Kaggle | Statistical features, Style based | |
Dong, et al. in ( ) | CNN + LSTM neural network | ASAP Kaggle | Word embedding, content based | QWK 0.764 |
Ajetunmobi and Daramola ( ) | WuPalmer algorithm | Statistical features | ||
Siyuan Zhao et al. in ( ) | LSTM (memory network) | ASAP Kaggle | Statistical features | QWK 0.78 |
Mathias and Bhattacharyya ( ) | Random Forest Classifier a classification model | ASAP Kaggle | Style and Content based features | Classified which feature set is required |
Brian Riordan et al. in ( ) | CNN + LSTM neural network | ASAP Kaggle short Answer | Word embeddings | QWK 0.90 |
Tirthankar Dasgupta et al. in ( ) | CNN -bidirectional LSTMs neural network | ASAP Kaggle | Content and physiological features | QWK 0.786 |
Wu and Shih ( ) | Classification model | SciEntBank answers | unigram_recall | Squared correlation coefficient 59.568 |
unigram_precision | ||||
unigram_F_measure | ||||
log_bleu_recall | ||||
log_bleu_precision | ||||
log_bleu_F_measure BLUE features | ||||
Yucheng Wang, etc.in ( ) | Bi-LSTM | ASAP Kaggle | Word embedding sequence | QWK 0.724 |
Anak Agung Putri Ratna et al. in ( ) | Winnowing ALGORITHM | 86.86 accuracy | ||
Sharma and Jayagopi ( ) | Glove, LSTM neural network | ASAP Kaggle | Hand written essay images | QWK 0.69 |
Jennifer O. Contreras et al. in ( ) | OntoGen (SVM) Linear Regression | University of Benghazi data set | Statistical, style-based features | |
Mathias, Bhattacharyya ( ) | GloVe,LSTM neural network | ASAP Kaggle | Statistical features, style features | Predicted Goodness score for essay |
Stefan Ruseti, et al. in ( ) | BiGRU Siamese architecture | Amazon Mechanical Turk online research service. Collected summaries | Word embedding | Accuracy 55.2 |
Zining wang, et al. in ( ) | LSTM (semantic) HAN (hierarchical attention network) neural network | ASAP Kaggle | Word embedding | QWK 0.83 |
Guoxi Liang et al. ( ) | Bi-LSTM | ASAP Kaggle | Word embedding, coherence of sentence | QWK 0.801 |
Ke et al. in ( ) | Classification model | ASAP Kaggle | Content based | Pearson’s Correlation Coefficient (PC)-0.39 ME-0.921 |
Tsegaye Misikir Tashu and Horváth in ( ) | Unsupervised learning–Locality Sensitivity Hashing | ASAP Kaggle | Statistical features | root mean squared error |
Kumar and Dey ( ) | Random Forest CNN, RNN neural network | ASAP Kaggle short Answer | Style and content-based features | QWK 0.82 |
Pedro Uria Rodriguez et al. ( ) | BERT, Xlnet | ASAP Kaggle | Error correction, sequence learning | QWK 0.755 |
Jiawei Liu et al. ( ) | CNN, LSTM, BERT | ASAP Kaggle | semantic data, handcrafted features like grammar correction, essay length, number of sentences, etc | QWK 0.709 |
Darwish and Mohamed ( ) | Multiple Linear Regression | ASAP Kaggle | Style and content-based features | QWK 0.77 |
Jiaqi Lun et al. ( ) | BERT | SemEval-2013 | Student Answer, Reference Answer | Accuracy 0.8277 (2-way) |
Süzen, Neslihan, et al. ( ) | Text mining | introductory computer science class in the University of North Texas, Student Assignments | Sentence similarity | Correlation score 0.81 |
Wilson Zhu and Yu Sun in ( ) | RNN (LSTM, Bi-LSTM) | ASAP Kaggle | Word embedding, grammar count, word count | QWK 0.70 |
Salim Yafet et al. ( ) | XGBoost machine learning classifier | ASAP Kaggle | Word count, POS, parse tree, coherence, cohesion, type token ration | Accuracy 68.12 |
Andrzej Cader ( ) | Deep Neural Network | University of Social Sciences in Lodz students’ answers | asynchronous feature | Accuracy 0.99 |
Tashu TM, Horváth T ( ) | Rule based algorithm, Similarity based algorithm | ASAP Kaggle | Similarity based | Accuracy 0.68 |
Masaki Uto(B) and Masashi Okano ( ) | Item Response Theory Models (CNN-LSTM,BERT) | ASAP Kaggle | QWK 0.749 |
Comparison of all approaches
In our study, we divided major AES approaches into three categories. Regression models, classification models, and neural network models. The regression models failed to find cohesion and coherence from the essay because it trained on BoW(Bag of Words) features. In processing data from input to output, the regression models are less complicated than neural networks. There are unable to find many intricate patterns from the essay and unable to find sentence connectivity. If we train the model with BoW features in the neural network approach, the model never considers the essay's coherence and coherence.
First, to train a Machine Learning algorithm with essays, all the essays are converted to vector form. We can form a vector with BoW and Word2vec, TF-IDF. The BoW and Word2vec vector representation of essays represented in Table Table6. 6 . The vector representation of BoW with TF-IDF is not incorporating the essays semantic, and it’s just statistical learning from a given vector. Word2vec vector comprises semantic of essay in a unidirectional way.
Vector representation of essays
Essay | BoW << vector >> | Word2vec << vector >> | |
---|---|---|---|
Student 1 response | I believe that using computers will benefit us in many ways like talking and becoming friends will others through websites like facebook and mysace | << 0.00000 0.00000 0.165746 0.280633 … 0.00000 0.280633 0.280633 0.280633 >> | << 3.9792988e-03 − 1.9810481e-03 1.9830784e-03 9.0381579e-04 − 2.9438005e-03 2.1778699e-03 4.4950014e-03 2.9508960e -03 − 2.2331756e-03 − 3.8774475e-03 3.5967759e- 03 − 4.0194849e-03 − 3.0412588e-03 − 2.4055617e-03 4.8296354e-03 2.4813593e-03… − 2.7158875e-03 − 1.4563646e-03 1.4072991e-03 − 5.2228488e-04 − 2.3597316e-03 6.2979700e-04 − 3.0249553e-03 4.4125126e-04 2.1633594e-03 − 4.9487003e-03 9.9755758e-05 − 2.4388896e-03 >> |
Student 2 response | More and more people use computers, but not everyone agrees that this benefits society. Those who support advances in technology believe that computers have a positive effect on people | << 0.26043 0.26043 0.153814 0.000000 … 0.26043 0.000000 0.000000 0.000000 > > | << 3.9792988e-03 − 1.9810481e- 03 1.9830784e-03 9.0381579e-04 − 2.9438005e-03 2.1778699e-03 4.4950014e-03 2.9508960e-03 − 2.2331756e-03 − 3.8774475e-03 3.5967759e-03 − 4.0194849e-03… − 2.7158875e-03 − 1.4563646e-03 1.4072991e-03 − 5.2228488e-04 − 2.3597316e-03 6.2979700e-04 − 3.0249553e-03 4.4125126e-04 3.7868773e-03 − 4.4193151e-03 3.0735810e-03 2.5546195e-03 2.1633594e-03 − 4.9487003e-03 9.9755758e-05 − 2.4388896e-03 >> |
In BoW, the vector contains the frequency of word occurrences in the essay. The vector represents 1 and more based on the happenings of words in the essay and 0 for not present. So, in BoW, the vector does not maintain the relationship with adjacent words; it’s just for single words. In word2vec, the vector represents the relationship between words with other words and sentences prompt in multiple dimensional ways. But word2vec prepares vectors in a unidirectional way, not in a bidirectional way; word2vec fails to find semantic vectors when a word has two meanings, and the meaning depends on adjacent words. Table Table7 7 represents a comparison of Machine Learning models and features extracting methods.
Comparison of models
BoW | Word2vec | |
---|---|---|
Regression models/classification models | The system implemented with Bow features and regression or classification algorithms will have low cohesion and coherence | The system implemented with Word2vec features and regression or classification algorithms will have low to medium cohesion and coherence |
Neural Networks (LSTM) | The system implemented with BoW features and neural network models will have low cohesion and coherence | The system implemented with Word2vec features and neural network model (LSTM) will have medium to high cohesion and coherence |
In AES, cohesion and coherence will check the content of the essay concerning the essay prompt these can be extracted from essay in the vector from. Two more parameters are there to access an essay is completeness and feedback. Completeness will check whether student’s response is sufficient or not though the student wrote correctly. Table Table8 8 represents all four parameters comparison for essay grading. Table Table9 9 illustrates comparison of all approaches based on various features like grammar, spelling, organization of essay, relevance.
Comparison of all models with respect to cohesion, coherence, completeness, feedback
Authors | Cohesion | Coherence | Completeness | Feed Back |
---|---|---|---|---|
Mohler and Mihalcea ( ) | Low | Low | Low | Low |
Mohler et al. ( ) | Medium | Low | Medium | Low |
Persing and Ng ( ) | Medium | Low | Low | Low |
Adamson et al. ( ) | Low | Low | Low | Low |
Ramachandran et al. ( ) | Medium | Medium | Low | Low |
Sakaguchi et al.. ( ), | Medium | Low | Low | Low |
Cummins et al. ( ) | Low | Low | Low | Low |
Sultan et al. ( ) | Medium | Medium | Low | Low |
Shehab et al. ( ) | Low | Low | Low | Low |
Kopparapu and De ( ) | Medium | Medium | Low | Low |
Dong an Zhang ( ) | Medium | Low | Low | Low |
Taghipour and Ng ( ) | Medium | Medium | Low | Low |
Zupanc et al. ( ) | Medium | Medium | Low | Low |
Dong et al. ( ) | Medium | Medium | Low | Low |
Riordan et al. ( ) | Medium | Medium | Medium | Low |
Zhao et al. ( ) | Medium | Medium | Low | Low |
Contreras et al. ( ) | Medium | Low | Low | Low |
Mathias and Bhattacharyya ( ; ) | Medium | Medium | Low | Low |
Mathias and Bhattacharyya ( ; ) | Medium | Medium | Low | Low |
Nguyen and Dery ( ) | Medium | Medium | Medium | Medium |
Ruseti et al. ( ) | Medium | Low | Low | Low |
Dasgupta et al. ( ) | Medium | Medium | Low | Low |
Liu et al.( ) | Low | Low | Low | Low |
Wang et al. ( ) | Medium | Low | Low | Low |
Guoxi Liang et al. ( ) | High | High | Low | Low |
Wang et al. ( ) | Medium | Medium | Low | Low |
Chen and Li ( ) | Medium | Medium | Low | Low |
Li et al. ( ) | Medium | Medium | Low | Low |
Alva-Manchego et al.( ) | Low | Low | Low | Low |
Jiawei Liu et al. ( ) | High | High | Medium | Low |
Pedro Uria Rodriguez et al. ( ) | Medium | Medium | Medium | Low |
Changzhi Cai( ) | Low | Low | Low | Low |
Xia et al. ( ) | Medium | Medium | Low | Low |
Chen and Zhou ( ) | Low | Low | Low | Low |
Kumar et al. ( ) | Medium | Medium | Medium | Low |
Ke et al. ( ) | Medium | Low | Medium | Low |
Andrzej Cader( ) | Low | Low | Low | Low |
Jiaqi Lun et al. ( ) | High | High | Low | Low |
Wilson Zhu and Yu Sun ( ) | Medium | Medium | Low | Low |
Süzen, Neslihan et al. ( ) | Medium | Low | Medium | Low |
Salim Yafet et al. ( ) | High | Medium | Low | Low |
Darwish and Mohamed ( ) | Medium | Low | Low | Low |
Tashu and Horváth ( ) | Medium | Medium | Low | Medium |
Tashu ( ) | Medium | Medium | Low | Low |
Masaki Uto(B) and Masashi Okano( ) | Medium | Medium | Medium | Medium |
Panitan Muangkammuen and Fumiyo Fukumoto( ) | Medium | Medium | Medium | Low |
comparison of all approaches on various features
Approaches | Grammar | Style (Word choice, sentence structure) | Mechanics (Spelling, punctuation, capitalization) | Development | BoW (tf-idf) | relevance |
---|---|---|---|---|---|---|
Mohler and Mihalcea ( ) | No | No | No | No | Yes | No |
Mohler et al. ( ) | Yes | No | No | No | Yes | No |
Persing and Ng ( ) | Yes | Yes | Yes | No | Yes | Yes |
Adamson et al. ( ) | Yes | No | Yes | No | Yes | No |
Ramachandran et al. ( ) | Yes | No | Yes | Yes | Yes | Yes |
Sakaguchi et al. ( ), | No | No | Yes | Yes | Yes | Yes |
Cummins et al. ( ) | Yes | No | Yes | No | Yes | No |
Sultan et al. ( ) | No | No | No | No | Yes | Yes |
Shehab et al. ( ) | Yes | Yes | Yes | No | Yes | No |
Kopparapu and De ( ) | No | No | No | No | Yes | No |
Dong and Zhang ( ) | Yes | No | Yes | No | Yes | Yes |
Taghipour and Ng ( ) | Yes | No | No | No | Yes | Yes |
Zupanc et al. ( ) | No | No | No | No | Yes | No |
Dong et al. ( ) | No | No | No | No | No | Yes |
Riordan et al. ( ) | No | No | No | No | No | Yes |
Zhao et al. ( ) | No | No | No | No | No | Yes |
Contreras et al. ( ) | Yes | No | No | No | Yes | Yes |
Mathias and Bhattacharyya ( , ) | No | Yes | Yes | No | No | Yes |
Mathias and Bhattacharyya ( , ) | Yes | No | Yes | No | Yes | Yes |
Nguyen and Dery ( ) | No | No | No | No | Yes | Yes |
Ruseti et al. ( ) | No | No | No | Yes | No | Yes |
Dasgupta et al. ( ) | Yes | Yes | Yes | Yes | No | Yes |
Liu et al.( ) | Yes | Yes | No | No | Yes | No |
Wang et al. ( ) | No | No | No | No | No | Yes |
Guoxi Liang et al. ( ) | No | No | No | No | No | Yes |
Wang et al. ( ) | No | No | No | No | No | Yes |
Chen and Li ( ) | No | No | No | No | No | Yes |
Li et al. ( ) | Yes | No | No | No | No | Yes |
Alva-Manchego et al. ( ) | Yes | No | No | Yes | No | Yes |
Jiawei Liu et al. ( ) | Yes | No | No | Yes | No | Yes |
Pedro Uria Rodriguez et al. ( ) | No | No | No | No | Yes | Yes |
Changzhi Cai( ) | No | No | No | No | No | Yes |
Xia et al. ( ) | No | No | No | No | No | Yes |
Chen and Zhou ( ) | No | No | No | No | No | Yes |
Kumar et al. ( ) | Yes | Yes | No | Yes | Yes | Yes |
Ke et al. ( ) | No | Yes | No | Yes | Yes | Yes |
Andrzej Cader( ) | No | No | No | No | No | Yes |
Jiaqi Lun et al. ( ) | No | No | No | No | No | Yes |
Wilson Zhu and Yu Sun ( ) | No | No | No | No | No | Yes |
Süzen, Neslihan, et al. ( ) | No | No | No | No | Yes | Yes |
Salim Yafet et al. ( ) | Yes | Yes | Yes | No | Yes | Yes |
Darwish and Mohamed ( ) | Yes | Yes | No | No | No | Yes |
What are the challenges/limitations in the current research?
From our study and results discussed in the previous sections, many researchers worked on automated essay scoring systems with numerous techniques. We have statistical methods, classification methods, and neural network approaches to evaluate the essay automatically. The main goal of the automated essay grading system is to reduce human effort and improve consistency.
The vast majority of essay scoring systems are dealing with the efficiency of the algorithm. But there are many challenges in automated essay grading systems. One should assess the essay by following parameters like the relevance of the content to the prompt, development of ideas, Cohesion, Coherence, and domain knowledge.
No model works on the relevance of content, which means whether student response or explanation is relevant to the given prompt or not if it is relevant to how much it is appropriate, and there is no discussion about the cohesion and coherence of the essays. All researches concentrated on extracting the features using some NLP libraries, trained their models, and testing the results. But there is no explanation in the essay evaluation system about consistency and completeness, But Palma and Atkinson ( 2018 ) explained coherence-based essay evaluation. And Zupanc and Bosnic ( 2014 ) also used the word coherence to evaluate essays. And they found consistency with latent semantic analysis (LSA) for finding coherence from essays, but the dictionary meaning of coherence is "The quality of being logical and consistent."
Another limitation is there is no domain knowledge-based evaluation of essays using Machine Learning models. For example, the meaning of a cell is different from biology to physics. Many Machine Learning models extract features with WordVec and GloVec; these NLP libraries cannot convert the words into vectors when they have two or more meanings.
Other challenges that influence the Automated Essay Scoring Systems.
All these approaches worked to improve the QWK score of their models. But QWK will not assess the model in terms of features extraction and constructed irrelevant answers. The QWK is not evaluating models whether the model is correctly assessing the answer or not. There are many challenges concerning students' responses to the Automatic scoring system. Like in evaluating approach, no model has examined how to evaluate the constructed irrelevant and adversarial answers. Especially the black box type of approaches like deep learning models provides more options to the students to bluff the automated scoring systems.
The Machine Learning models that work on statistical features are very vulnerable. Based on Powers et al. ( 2001 ) and Bejar Isaac et al. ( 2014 ), the E-rater was failed on Constructed Irrelevant Responses Strategy (CIRS). From the study of Bejar et al. ( 2013 ), Higgins and Heilman ( 2014 ), observed that when student response contain irrelevant content or shell language concurring to prompt will influence the final score of essays in an automated scoring system.
In deep learning approaches, most of the models automatically read the essay's features, and some methods work on word-based embedding and other character-based embedding features. From the study of Riordan Brain et al. ( 2019 ), The character-based embedding systems do not prioritize spelling correction. However, it is influencing the final score of the essay. From the study of Horbach and Zesch ( 2019 ), Various factors are influencing AES systems. For example, there are data set size, prompt type, answer length, training set, and human scorers for content-based scoring.
Ding et al. ( 2020 ) reviewed that the automated scoring system is vulnerable when a student response contains more words from prompt, like prompt vocabulary repeated in the response. Parekh et al. ( 2020 ) and Kumar et al. ( 2020 ) tested various neural network models of AES by iteratively adding important words, deleting unimportant words, shuffle the words, and repeating sentences in an essay and found that no change in the final score of essays. These neural network models failed to recognize common sense in adversaries' essays and give more options for the students to bluff the automated systems.
Other than NLP and ML techniques for AES. From Wresch ( 1993 ) to Madnani and Cahill ( 2018 ). discussed the complexity of AES systems, standards need to be followed. Like assessment rubrics to test subject knowledge, irrelevant responses, and ethical aspects of an algorithm like measuring the fairness of student response.
Fairness is an essential factor for automated systems. For example, in AES, fairness can be measure in an agreement between human score to machine score. Besides this, From Loukina et al. ( 2019 ), the fairness standards include overall score accuracy, overall score differences, and condition score differences between human and system scores. In addition, scoring different responses in the prospect of constructive relevant and irrelevant will improve fairness.
Madnani et al. ( 2017a ; b ). Discussed the fairness of AES systems for constructed responses and presented RMS open-source tool for detecting biases in the models. With this, one can change fairness standards according to their analysis of fairness.
From Berzak et al.'s ( 2018 ) approach, behavior factors are a significant challenge in automated scoring systems. That helps to find language proficiency, word characteristics (essential words from the text), predict the critical patterns from the text, find related sentences in an essay, and give a more accurate score.
Rupp ( 2018 ), has discussed the designing, evaluating, and deployment methodologies for AES systems. They provided notable characteristics of AES systems for deployment. They are like model performance, evaluation metrics for a model, threshold values, dynamically updated models, and framework.
First, we should check the model performance on different datasets and parameters for operational deployment. Selecting Evaluation metrics for AES models are like QWK, correlation coefficient, or sometimes both. Kelley and Preacher ( 2012 ) have discussed three categories of threshold values: marginal, borderline, and acceptable. The values can be varied based on data size, model performance, type of model (single scoring, multiple scoring models). Once a model is deployed and evaluates millions of responses every time for optimal responses, we need a dynamically updated model based on prompt and data. Finally, framework designing of AES model, hear a framework contains prompts where test-takers can write the responses. One can design two frameworks: a single scoring model for a single methodology and multiple scoring models for multiple concepts. When we deploy multiple scoring models, each prompt could be trained separately, or we can provide generalized models for all prompts with this accuracy may vary, and it is challenging.
Our Systematic literature review on the automated essay grading system first collected 542 papers with selected keywords from various databases. After inclusion and exclusion criteria, we left with 139 articles; on these selected papers, we applied Quality assessment criteria with two reviewers, and finally, we selected 62 writings for final review.
Our observations on automated essay grading systems from 2010 to 2020 are as followed:
- The implementation techniques of automated essay grading systems are classified into four buckets; there are 1. regression models 2. Classification models 3. Neural networks 4. Ontology-based methodology, but using neural networks, the researchers are more accurate than other techniques, and all the methods state of the art provided in Table Table3 3 .
- The majority of the regression and classification models on essay scoring used statistical features to find the final score. It means the systems or models trained on such parameters as word count, sentence count, etc. though the parameters extracted from the essay, the algorithm are not directly training on essays. The algorithms trained on some numbers obtained from the essay and hear if numbers matched the composition will get a good score; otherwise, the rating is less. In these models, the evaluation process is entirely on numbers, irrespective of the essay. So, there is a lot of chance to miss the coherence, relevance of the essay if we train our algorithm on statistical parameters.
- In the neural network approach, the models trained on Bag of Words (BoW) features. The BoW feature is missing the relationship between a word to word and the semantic meaning of the sentence. E.g., Sentence 1: John killed bob. Sentence 2: bob killed John. In these two sentences, the BoW is "John," "killed," "bob."
- In the Word2Vec library, if we are prepared a word vector from an essay in a unidirectional way, the vector will have a dependency with other words and finds the semantic relationship with other words. But if a word has two or more meanings like "Bank loan" and "River Bank," hear bank has two implications, and its adjacent words decide the sentence meaning; in this case, Word2Vec is not finding the real meaning of the word from the sentence.
- The features extracted from essays in the essay scoring system are classified into 3 type's features like statistical features, style-based features, and content-based features, which are explained in RQ2 and Table Table3. 3 . But statistical features, are playing a significant role in some systems and negligible in some systems. In Shehab et al. ( 2016 ); Cummins et al. ( 2016 ). Dong et al. ( 2017 ). Dong and Zhang ( 2016 ). Mathias and Bhattacharyya ( 2018a ; b ) Systems the assessment is entirely on statistical and style-based features they have not retrieved any content-based features. And in other systems that extract content from the essays, the role of statistical features is for only preprocessing essays but not included in the final grading.
- In AES systems, coherence is the main feature to be considered while evaluating essays. The actual meaning of coherence is to stick together. That is the logical connection of sentences (local level coherence) and paragraphs (global level coherence) in a story. Without coherence, all sentences in a paragraph are independent and meaningless. In an Essay, coherence is a significant feature that is explaining everything in a flow and its meaning. It is a powerful feature in AES system to find the semantics of essay. With coherence, one can assess whether all sentences are connected in a flow and all paragraphs are related to justify the prompt. Retrieving the coherence level from an essay is a critical task for all researchers in AES systems.
- In automatic essay grading systems, the assessment of essays concerning content is critical. That will give the actual score for the student. Most of the researches used statistical features like sentence length, word count, number of sentences, etc. But according to collected results, 32% of the systems used content-based features for the essay scoring. Example papers which are on content-based assessment are Taghipour and Ng ( 2016 ); Persing and Ng ( 2013 ); Wang et al. ( 2018a , 2018b ); Zhao et al. ( 2017 ); Kopparapu and De ( 2016 ), Kumar et al. ( 2019 ); Mathias and Bhattacharyya ( 2018a ; b ); Mohler and Mihalcea ( 2009 ) are used content and statistical-based features. The results are shown in Fig. Fig.3. 3 . And mainly the content-based features extracted with word2vec NLP library, but word2vec is capable of capturing the context of a word in a document, semantic and syntactic similarity, relation with other terms, but word2vec is capable of capturing the context word in a uni-direction either left or right. If a word has multiple meanings, there is a chance of missing the context in the essay. After analyzing all the papers, we found that content-based assessment is a qualitative assessment of essays.
- On the other hand, Horbach and Zesch ( 2019 ); Riordan Brain et al. ( 2019 ); Ding et al. ( 2020 ); Kumar et al. ( 2020 ) proved that neural network models are vulnerable when a student response contains constructed irrelevant, adversarial answers. And a student can easily bluff an automated scoring system by submitting different responses like repeating sentences and repeating prompt words in an essay. From Loukina et al. ( 2019 ), and Madnani et al. ( 2017b ). The fairness of an algorithm is an essential factor to be considered in AES systems.
- While talking about speech assessment, the data set contains audios of duration up to one minute. Feature extraction techniques are entirely different from text assessment, and accuracy varies based on speaking fluency, pitching, male to female voice and boy to adult voice. But the training algorithms are the same for text and speech assessment.
- Once an AES system evaluates essays and short answers accurately in all directions, there is a massive demand for automated systems in the educational and related world. Now AES systems are deployed in GRE, TOEFL exams; other than these, we can deploy AES systems in massive open online courses like Coursera(“ https://coursera.org/learn//machine-learning//exam ”), NPTEL ( https://swayam.gov.in/explorer ), etc. still they are assessing student performance with multiple-choice questions. In another perspective, AES systems can be deployed in information retrieval systems like Quora, stack overflow, etc., to check whether the retrieved response is appropriate to the question or not and can give ranking to the retrieved answers.
Conclusion and future work
As per our Systematic literature review, we studied 62 papers. There exist significant challenges for researchers in implementing automated essay grading systems. Several researchers are working rigorously on building a robust AES system despite its difficulty in solving this problem. All evaluating methods are not evaluated based on coherence, relevance, completeness, feedback, and knowledge-based. And 90% of essay grading systems are used Kaggle ASAP (2012) dataset, which has general essays from students and not required any domain knowledge, so there is a need for domain-specific essay datasets to train and test. Feature extraction is with NLTK, WordVec, and GloVec NLP libraries; these libraries have many limitations while converting a sentence into vector form. Apart from feature extraction and training Machine Learning models, no system is accessing the essay's completeness. No system provides feedback to the student response and not retrieving coherence vectors from the essay—another perspective the constructive irrelevant and adversarial student responses still questioning AES systems.
Our proposed research work will go on the content-based assessment of essays with domain knowledge and find a score for the essays with internal and external consistency. And we will create a new dataset concerning one domain. And another area in which we can improve is the feature extraction techniques.
This study includes only four digital databases for study selection may miss some functional studies on the topic. However, we hope that we covered most of the significant studies as we manually collected some papers published in useful journals.
Below is the link to the electronic supplementary material.
Not Applicable.
Publisher's Note
Springer Nature remains neutral with regard to jurisdictional claims in published maps and institutional affiliations.
Contributor Information
Dadi Ramesh, Email: moc.liamg@44hsemaridad .
Suresh Kumar Sanampudi, Email: ni.ca.hutnj@idupmanashserus .
- Adamson, A., Lamb, A., & December, R. M. (2014). Automated Essay Grading.
- Ajay HB, Tillett PI, Page EB (1973) Analysis of essays by computer (AEC-II) (No. 8-0102). Washington, DC: U.S. Department of Health, Education, and Welfare, Office of Education, National Center for Educational Research and Development
- Ajetunmobi SA, Daramola O (2017) Ontology-based information extraction for subject-focussed automatic essay evaluation. In: 2017 International Conference on Computing Networking and Informatics (ICCNI) p 1–6. IEEE
- Alva-Manchego F, et al. (2019) EASSE: Easier Automatic Sentence Simplification Evaluation.” ArXiv abs/1908.04567 (2019): n. pag
- Bailey S, Meurers D (2008) Diagnosing meaning errors in short answers to reading comprehension questions. In: Proceedings of the Third Workshop on Innovative Use of NLP for Building Educational Applications (Columbus), p 107–115
- Basu S, Jacobs C, Vanderwende L. Powergrading: a clustering approach to amplify human effort for short answer grading. Trans Assoc Comput Linguist (TACL) 2013; 1 :391–402. doi: 10.1162/tacl_a_00236. [ CrossRef ] [ Google Scholar ]
- Bejar, I. I., Flor, M., Futagi, Y., & Ramineni, C. (2014). On the vulnerability of automated scoring to construct-irrelevant response strategies (CIRS): An illustration. Assessing Writing, 22, 48-59.
- Bejar I, et al. (2013) Length of Textual Response as a Construct-Irrelevant Response Strategy: The Case of Shell Language. Research Report. ETS RR-13-07.” ETS Research Report Series (2013): n. pag
- Berzak Y, et al. (2018) “Assessing Language Proficiency from Eye Movements in Reading.” ArXiv abs/1804.07329 (2018): n. pag
- Blanchard D, Tetreault J, Higgins D, Cahill A, Chodorow M (2013) TOEFL11: A corpus of non-native English. ETS Research Report Series, 2013(2):i–15, 2013
- Blood, I. (2011). Automated essay scoring: a literature review. Studies in Applied Linguistics and TESOL, 11(2).
- Burrows S, Gurevych I, Stein B. The eras and trends of automatic short answer grading. Int J Artif Intell Educ. 2015; 25 :60–117. doi: 10.1007/s40593-014-0026-8. [ CrossRef ] [ Google Scholar ]
- Cader, A. (2020, July). The Potential for the Use of Deep Neural Networks in e-Learning Student Evaluation with New Data Augmentation Method. In International Conference on Artificial Intelligence in Education (pp. 37–42). Springer, Cham.
- Cai C (2019) Automatic essay scoring with recurrent neural network. In: Proceedings of the 3rd International Conference on High Performance Compilation, Computing and Communications (2019): n. pag.
- Chen M, Li X (2018) "Relevance-Based Automated Essay Scoring via Hierarchical Recurrent Model. In: 2018 International Conference on Asian Language Processing (IALP), Bandung, Indonesia, 2018, p 378–383, doi: 10.1109/IALP.2018.8629256
- Chen Z, Zhou Y (2019) "Research on Automatic Essay Scoring of Composition Based on CNN and OR. In: 2019 2nd International Conference on Artificial Intelligence and Big Data (ICAIBD), Chengdu, China, p 13–18, doi: 10.1109/ICAIBD.2019.8837007
- Contreras JO, Hilles SM, Abubakar ZB (2018) Automated essay scoring with ontology based on text mining and NLTK tools. In: 2018 International Conference on Smart Computing and Electronic Enterprise (ICSCEE), 1-6
- Correnti R, Matsumura LC, Hamilton L, Wang E. Assessing students’ skills at writing analytically in response to texts. Elem Sch J. 2013; 114 (2):142–177. doi: 10.1086/671936. [ CrossRef ] [ Google Scholar ]
- Cummins, R., Zhang, M., & Briscoe, E. (2016, August). Constrained multi-task learning for automated essay scoring. Association for Computational Linguistics.
- Darwish SM, Mohamed SK (2020) Automated essay evaluation based on fusion of fuzzy ontology and latent semantic analysis. In: Hassanien A, Azar A, Gaber T, Bhatnagar RF, Tolba M (eds) The International Conference on Advanced Machine Learning Technologies and Applications
- Dasgupta T, Naskar A, Dey L, Saha R (2018) Augmenting textual qualitative features in deep convolution recurrent neural network for automatic essay scoring. In: Proceedings of the 5th Workshop on Natural Language Processing Techniques for Educational Applications p 93–102
- Ding Y, et al. (2020) "Don’t take “nswvtnvakgxpm” for an answer–The surprising vulnerability of automatic content scoring systems to adversarial input." In: Proceedings of the 28th International Conference on Computational Linguistics
- Dong F, Zhang Y (2016) Automatic features for essay scoring–an empirical study. In: Proceedings of the 2016 Conference on Empirical Methods in Natural Language Processing p 1072–1077
- Dong F, Zhang Y, Yang J (2017) Attention-based recurrent convolutional neural network for automatic essay scoring. In: Proceedings of the 21st Conference on Computational Natural Language Learning (CoNLL 2017) p 153–162
- Dzikovska M, Nielsen R, Brew C, Leacock C, Gi ampiccolo D, Bentivogli L, Clark P, Dagan I, Dang HT (2013a) Semeval-2013 task 7: The joint student response analysis and 8th recognizing textual entailment challenge
- Dzikovska MO, Nielsen R, Brew C, Leacock C, Giampiccolo D, Bentivogli L, Clark P, Dagan I, Trang Dang H (2013b) SemEval-2013 Task 7: The Joint Student Response Analysis and 8th Recognizing Textual Entailment Challenge. *SEM 2013: The First Joint Conference on Lexical and Computational Semantics
- Educational Testing Service (2008) CriterionSM online writing evaluation service. Retrieved from http://www.ets.org/s/criterion/pdf/9286_CriterionBrochure.pdf .
- Evanini, K., & Wang, X. (2013, August). Automated speech scoring for non-native middle school students with multiple task types. In INTERSPEECH (pp. 2435–2439).
- Foltz PW, Laham D, Landauer TK (1999) The Intelligent Essay Assessor: Applications to Educational Technology. Interactive Multimedia Electronic Journal of Computer-Enhanced Learning, 1, 2, http://imej.wfu.edu/articles/1999/2/04/ index.asp
- Granger, S., Dagneaux, E., Meunier, F., & Paquot, M. (Eds.). (2009). International corpus of learner English. Louvain-la-Neuve: Presses universitaires de Louvain.
- Higgins D, Heilman M. Managing what we can measure: quantifying the susceptibility of automated scoring systems to gaming behavior” Educ Meas Issues Pract. 2014; 33 :36–46. doi: 10.1111/emip.12036. [ CrossRef ] [ Google Scholar ]
- Horbach A, Zesch T. The influence of variance in learner answers on automatic content scoring. Front Educ. 2019; 4 :28. doi: 10.3389/feduc.2019.00028. [ CrossRef ] [ Google Scholar ]
- https://www.coursera.org/learn/machine-learning/exam/7pytE/linear-regression-with-multiple-variables/attempt
- Hussein, M. A., Hassan, H., & Nassef, M. (2019). Automated language essay scoring systems: A literature review. PeerJ Computer Science, 5, e208. [ PMC free article ] [ PubMed ]
- Ke Z, Ng V (2019) “Automated essay scoring: a survey of the state of the art.” IJCAI
- Ke, Z., Inamdar, H., Lin, H., & Ng, V. (2019, July). Give me more feedback II: Annotating thesis strength and related attributes in student essays. In Proceedings of the 57th Annual Meeting of the Association for Computational Linguistics (pp. 3994-4004).
- Kelley K, Preacher KJ. On effect size. Psychol Methods. 2012; 17 (2):137–152. doi: 10.1037/a0028086. [ PubMed ] [ CrossRef ] [ Google Scholar ]
- Kitchenham B, Brereton OP, Budgen D, Turner M, Bailey J, Linkman S. Systematic literature reviews in software engineering–a systematic literature review. Inf Softw Technol. 2009; 51 (1):7–15. doi: 10.1016/j.infsof.2008.09.009. [ CrossRef ] [ Google Scholar ]
- Klebanov, B. B., & Madnani, N. (2020, July). Automated evaluation of writing–50 years and counting. In Proceedings of the 58th Annual Meeting of the Association for Computational Linguistics (pp. 7796–7810).
- Knill K, Gales M, Kyriakopoulos K, et al. (4 more authors) (2018) Impact of ASR performance on free speaking language assessment. In: Interspeech 2018.02–06 Sep 2018, Hyderabad, India. International Speech Communication Association (ISCA)
- Kopparapu SK, De A (2016) Automatic ranking of essays using structural and semantic features. In: 2016 International Conference on Advances in Computing, Communications and Informatics (ICACCI), p 519–523
- Kumar, Y., Aggarwal, S., Mahata, D., Shah, R. R., Kumaraguru, P., & Zimmermann, R. (2019, July). Get it scored using autosas—an automated system for scoring short answers. In Proceedings of the AAAI Conference on Artificial Intelligence (Vol. 33, No. 01, pp. 9662–9669).
- Kumar Y, et al. (2020) “Calling out bluff: attacking the robustness of automatic scoring systems with simple adversarial testing.” ArXiv abs/2007.06796
- Li X, Chen M, Nie J, Liu Z, Feng Z, Cai Y (2018) Coherence-Based Automated Essay Scoring Using Self-attention. In: Sun M, Liu T, Wang X, Liu Z, Liu Y (eds) Chinese Computational Linguistics and Natural Language Processing Based on Naturally Annotated Big Data. CCL 2018, NLP-NABD 2018. Lecture Notes in Computer Science, vol 11221. Springer, Cham. 10.1007/978-3-030-01716-3_32
- Liang G, On B, Jeong D, Kim H, Choi G. Automated essay scoring: a siamese bidirectional LSTM neural network architecture. Symmetry. 2018; 10 :682. doi: 10.3390/sym10120682. [ CrossRef ] [ Google Scholar ]
- Liua, H., Yeb, Y., & Wu, M. (2018, April). Ensemble Learning on Scoring Student Essay. In 2018 International Conference on Management and Education, Humanities and Social Sciences (MEHSS 2018). Atlantis Press.
- Liu J, Xu Y, Zhao L (2019) Automated Essay Scoring based on Two-Stage Learning. ArXiv, abs/1901.07744
- Loukina A, et al. (2015) Feature selection for automated speech scoring.” BEA@NAACL-HLT
- Loukina A, et al. (2017) “Speech- and Text-driven Features for Automated Scoring of English-Speaking Tasks.” SCNLP@EMNLP 2017
- Loukina A, et al. (2019) The many dimensions of algorithmic fairness in educational applications. BEA@ACL
- Lun J, Zhu J, Tang Y, Yang M (2020) Multiple data augmentation strategies for improving performance on automatic short answer scoring. In: Proceedings of the AAAI Conference on Artificial Intelligence, 34(09): 13389-13396
- Madnani, N., & Cahill, A. (2018, August). Automated scoring: Beyond natural language processing. In Proceedings of the 27th International Conference on Computational Linguistics (pp. 1099–1109).
- Madnani N, et al. (2017b) “Building better open-source tools to support fairness in automated scoring.” EthNLP@EACL
- Malinin A, et al. (2016) “Off-topic response detection for spontaneous spoken english assessment.” ACL
- Malinin A, et al. (2017) “Incorporating uncertainty into deep learning for spoken language assessment.” ACL
- Mathias S, Bhattacharyya P (2018a) Thank “Goodness”! A Way to Measure Style in Student Essays. In: Proceedings of the 5th Workshop on Natural Language Processing Techniques for Educational Applications p 35–41
- Mathias S, Bhattacharyya P (2018b) ASAP++: Enriching the ASAP automated essay grading dataset with essay attribute scores. In: Proceedings of the Eleventh International Conference on Language Resources and Evaluation (LREC 2018).
- Mikolov T, et al. (2013) “Efficient Estimation of Word Representations in Vector Space.” ICLR
- Mohler M, Mihalcea R (2009) Text-to-text semantic similarity for automatic short answer grading. In: Proceedings of the 12th Conference of the European Chapter of the ACL (EACL 2009) p 567–575
- Mohler M, Bunescu R, Mihalcea R (2011) Learning to grade short answer questions using semantic similarity measures and dependency graph alignments. In: Proceedings of the 49th annual meeting of the association for computational linguistics: Human language technologies p 752–762
- Muangkammuen P, Fukumoto F (2020) Multi-task Learning for Automated Essay Scoring with Sentiment Analysis. In: Proceedings of the 1st Conference of the Asia-Pacific Chapter of the Association for Computational Linguistics and the 10th International Joint Conference on Natural Language Processing: Student Research Workshop p 116–123
- Nguyen, H., & Dery, L. (2016). Neural networks for automated essay grading. CS224d Stanford Reports, 1–11.
- Palma D, Atkinson J. Coherence-based automatic essay assessment. IEEE Intell Syst. 2018; 33 (5):26–36. doi: 10.1109/MIS.2018.2877278. [ CrossRef ] [ Google Scholar ]
- Parekh S, et al (2020) My Teacher Thinks the World Is Flat! Interpreting Automatic Essay Scoring Mechanism.” ArXiv abs/2012.13872 (2020): n. pag
- Pennington, J., Socher, R., & Manning, C. D. (2014, October). Glove: Global vectors for word representation. In Proceedings of the 2014 conference on empirical methods in natural language processing (EMNLP) (pp. 1532–1543).
- Persing I, Ng V (2013) Modeling thesis clarity in student essays. In:Proceedings of the 51st Annual Meeting of the Association for Computational Linguistics (Volume 1: Long Papers) p 260–269
- Powers DE, Burstein JC, Chodorow M, Fowles ME, Kukich K. Stumping E-Rater: challenging the validity of automated essay scoring. ETS Res Rep Ser. 2001; 2001 (1):i–44. [ Google Scholar ]
- Powers DE, Burstein JC, Chodorow M, Fowles ME, Kukich K. Stumping e-rater: challenging the validity of automated essay scoring. Comput Hum Behav. 2002; 18 (2):103–134. doi: 10.1016/S0747-5632(01)00052-8. [ CrossRef ] [ Google Scholar ]
- Ramachandran L, Cheng J, Foltz P (2015) Identifying patterns for short answer scoring using graph-based lexico-semantic text matching. In: Proceedings of the Tenth Workshop on Innovative Use of NLP for Building Educational Applications p 97–106
- Ramanarayanan V, et al. (2017) “Human and Automated Scoring of Fluency, Pronunciation and Intonation During Human-Machine Spoken Dialog Interactions.” INTERSPEECH
- Riordan B, Horbach A, Cahill A, Zesch T, Lee C (2017) Investigating neural architectures for short answer scoring. In: Proceedings of the 12th Workshop on Innovative Use of NLP for Building Educational Applications p 159–168
- Riordan B, Flor M, Pugh R (2019) "How to account for misspellings: Quantifying the benefit of character representations in neural content scoring models."In: Proceedings of the Fourteenth Workshop on Innovative Use of NLP for Building Educational Applications
- Rodriguez P, Jafari A, Ormerod CM (2019) Language models and Automated Essay Scoring. ArXiv, abs/1909.09482
- Rudner, L. M., & Liang, T. (2002). Automated essay scoring using Bayes' theorem. The Journal of Technology, Learning and Assessment, 1(2).
- Rudner, L. M., Garcia, V., & Welch, C. (2006). An evaluation of IntelliMetric™ essay scoring system. The Journal of Technology, Learning and Assessment, 4(4).
- Rupp A. Designing, evaluating, and deploying automated scoring systems with validity in mind: methodological design decisions. Appl Meas Educ. 2018; 31 :191–214. doi: 10.1080/08957347.2018.1464448. [ CrossRef ] [ Google Scholar ]
- Ruseti S, Dascalu M, Johnson AM, McNamara DS, Balyan R, McCarthy KS, Trausan-Matu S (2018) Scoring summaries using recurrent neural networks. In: International Conference on Intelligent Tutoring Systems p 191–201. Springer, Cham
- Sakaguchi K, Heilman M, Madnani N (2015) Effective feature integration for automated short answer scoring. In: Proceedings of the 2015 conference of the North American Chapter of the association for computational linguistics: Human language technologies p 1049–1054
- Salim, Y., Stevanus, V., Barlian, E., Sari, A. C., & Suhartono, D. (2019, December). Automated English Digital Essay Grader Using Machine Learning. In 2019 IEEE International Conference on Engineering, Technology and Education (TALE) (pp. 1–6). IEEE.
- Shehab A, Elhoseny M, Hassanien AE (2016) A hybrid scheme for Automated Essay Grading based on LVQ and NLP techniques. In: 12th International Computer Engineering Conference (ICENCO), Cairo, 2016, p 65-70
- Shermis MD, Mzumara HR, Olson J, Harrington S. On-line grading of student essays: PEG goes on the World Wide Web. Assess Eval High Educ. 2001; 26 (3):247–259. doi: 10.1080/02602930120052404. [ CrossRef ] [ Google Scholar ]
- Stab C, Gurevych I (2014) Identifying argumentative discourse structures in persuasive essays. In: Proceedings of the 2014 Conference on Empirical Methods in Natural Language Processing (EMNLP) p 46–56
- Sultan MA, Salazar C, Sumner T (2016) Fast and easy short answer grading with high accuracy. In: Proceedings of the 2016 Conference of the North American Chapter of the Association for Computational Linguistics: Human Language Technologies p 1070–1075
- Süzen, N., Gorban, A. N., Levesley, J., & Mirkes, E. M. (2020). Automatic short answer grading and feedback using text mining methods. Procedia Computer Science, 169, 726–743.
- Taghipour K, Ng HT (2016) A neural approach to automated essay scoring. In: Proceedings of the 2016 conference on empirical methods in natural language processing p 1882–1891
- Tashu TM (2020) "Off-Topic Essay Detection Using C-BGRU Siamese. In: 2020 IEEE 14th International Conference on Semantic Computing (ICSC), San Diego, CA, USA, p 221–225, doi: 10.1109/ICSC.2020.00046
- Tashu TM, Horváth T (2019) A layered approach to automatic essay evaluation using word-embedding. In: McLaren B, Reilly R, Zvacek S, Uhomoibhi J (eds) Computer Supported Education. CSEDU 2018. Communications in Computer and Information Science, vol 1022. Springer, Cham
- Tashu TM, Horváth T (2020) Semantic-Based Feedback Recommendation for Automatic Essay Evaluation. In: Bi Y, Bhatia R, Kapoor S (eds) Intelligent Systems and Applications. IntelliSys 2019. Advances in Intelligent Systems and Computing, vol 1038. Springer, Cham
- Uto M, Okano M (2020) Robust Neural Automated Essay Scoring Using Item Response Theory. In: Bittencourt I, Cukurova M, Muldner K, Luckin R, Millán E (eds) Artificial Intelligence in Education. AIED 2020. Lecture Notes in Computer Science, vol 12163. Springer, Cham
- Wang Z, Liu J, Dong R (2018a) Intelligent Auto-grading System. In: 2018 5th IEEE International Conference on Cloud Computing and Intelligence Systems (CCIS) p 430–435. IEEE.
- Wang Y, et al. (2018b) “Automatic Essay Scoring Incorporating Rating Schema via Reinforcement Learning.” EMNLP
- Zhu W, Sun Y (2020) Automated essay scoring system using multi-model Machine Learning, david c. wyld et al. (eds): mlnlp, bdiot, itccma, csity, dtmn, aifz, sigpro
- Wresch W. The Imminence of Grading Essays by Computer-25 Years Later. Comput Compos. 1993; 10 :45–58. doi: 10.1016/S8755-4615(05)80058-1. [ CrossRef ] [ Google Scholar ]
- Wu, X., Knill, K., Gales, M., & Malinin, A. (2020). Ensemble approaches for uncertainty in spoken language assessment.
- Xia L, Liu J, Zhang Z (2019) Automatic Essay Scoring Model Based on Two-Layer Bi-directional Long-Short Term Memory Network. In: Proceedings of the 2019 3rd International Conference on Computer Science and Artificial Intelligence p 133–137
- Yannakoudakis H, Briscoe T, Medlock B (2011) A new dataset and method for automatically grading ESOL texts. In: Proceedings of the 49th annual meeting of the association for computational linguistics: human language technologies p 180–189
- Zhao S, Zhang Y, Xiong X, Botelho A, Heffernan N (2017) A memory-augmented neural model for automated grading. In: Proceedings of the Fourth (2017) ACM Conference on Learning@ Scale p 189–192
- Zupanc K, Bosnic Z (2014) Automated essay evaluation augmented with semantic coherence measures. In: 2014 IEEE International Conference on Data Mining p 1133–1138. IEEE.
- Zupanc K, Savić M, Bosnić Z, Ivanović M (2017) Evaluating coherence of essays using sentence-similarity networks. In: Proceedings of the 18th International Conference on Computer Systems and Technologies p 65–72
- Dzikovska, M. O., Nielsen, R., & Brew, C. (2012, June). Towards effective tutorial feedback for explanation questions: A dataset and baselines. In Proceedings of the 2012 Conference of the North American Chapter of the Association for Computational Linguistics: Human Language Technologies (pp. 200-210).
- Kumar, N., & Dey, L. (2013, November). Automatic Quality Assessment of documents with application to essay grading. In 2013 12th Mexican International Conference on Artificial Intelligence (pp. 216–222). IEEE.
- Wu, S. H., & Shih, W. F. (2018, July). A short answer grading system in chinese by support vector approach. In Proceedings of the 5th Workshop on Natural Language Processing Techniques for Educational Applications (pp. 125-129).
- Agung Putri Ratna, A., Lalita Luhurkinanti, D., Ibrahim I., Husna D., Dewi Purnamasari P. (2018). Automatic Essay Grading System for Japanese Language Examination Using Winnowing Algorithm, 2018 International Seminar on Application for Technology of Information and Communication, 2018, pp. 565–569. 10.1109/ISEMANTIC.2018.8549789.
- Sharma A., & Jayagopi D. B. (2018). Automated Grading of Handwritten Essays 2018 16th International Conference on Frontiers in Handwriting Recognition (ICFHR), 2018, pp 279–284. 10.1109/ICFHR-2018.2018.00056
Teaching Topic
Grading Student Work
From a student’s perspective, grading can seem like a mysterious and even sometimes arbitrary process, and yet it has the potential to be an important, productive, and educational part of a course. For ideas on low-stakes (ungraded) evaluation of students, see our page on Classroom Assessment Techniques .
It helps to recognize that grading serves multiple purposes beyond the obvious one of giving the registrar information for their calculations of credits and GPAs. Assessing students:
- can tell you a lot about what students are understanding;
- can give you insight into your teaching effectiveness;
- and can be educational for the student as well.
For all these benefits to accrue, however, you have to devise a consistent and fair system of grading that aligns with your teaching goals, and you have to communicate that system to your students.
In their book Effective Grading: A Tool for Learning and Assessment , professors Barbara Walvoord and Virginia Johnson Anderson remind us that “a model for calculating course grades is not just a mathematical formula; it is an expression of your values and goals.” In other words, when devising a system for grading student work, you need to keep in mind your learning goals for those students, so that you (a) evaluate students on dimensions that you care about, dimensions that are relevant to those goals, and (b) so that your feedback can help them pursue those goals.
Grading across the semester
Your first decision is going to be about how much weight you give to each graded student activity (tests, papers, projects, participation), and this should certainly map on to your values and goals for the course.
In a unit-based approach , you weight a variety of activities equally across the length of the semester. You might do this in a course that covers a number of topics of similar importance. Giving equal weight means that a test or paper for the first section of the course, say, has no more or less impact on a student’s overall grade than a test or paper for the final section of the course. (In a maximal version of this, there might be four exams, each of which was worth 25% of the final grade.) One advantage of this structure is that it encourages a relatively steady level of student effort throughout the course.
In a developmental approach , you give more weight to activities that come later in the semester; a final exam or project or essay contributes more to the final grade than earlier work. (Perhaps the first paper of the semester is worth 15% of the grade, and the final one is worth, say, 35%, the rest of the points being associated with other activities.) This is a good choice for classes where students are going to be in a better position to show their learning at the end of the semester than at the beginning—if, for example, it’s a class where students have to amass a number of skills before they can experience a qualitative shift in their aptitude.
Grading assignments
Grading efficiently.
Grading does take time and energy, but there are ways to do it more efficiently:
- Bear in mind that grading efficiently is partly a matter of practice. When you’re just starting out teaching, you’re working to determine what your priorities and standards are, which makes it harder to come to decisions about grades; once you’ve become more certain of those (at least provisionally), the grading will go more quickly.
- As the first point suggests, it helps to know, in advance, what you’re looking for in the student work, instead of trying to figure it out once you’re hip-deep in the grading process for a particular assignment. The development of rubrics (see the Grading Fairly section below) will focus you on the elements that matter most to you and allow you to ignore things that don’t matter as much.
- Relatedly, if your grading includes written feedback for the students, don’t comment on everything—just comment on the things that are central to the assignment, the things students really need to master. (See our Responding to Student Writing page for more thoughts on this.)
- Successful work is easier to grade than unsuccessful work. You can speed up your grading process by doing more ahead of the assignment—providing opportunities for practice and review sessions, using a wide range of teaching techniques, checking in with students in class to see how much they understand—to make sure students are ready to perform well.
- Finally, to state the obvious, we live in a world of distractions. Research shows that interruptions make us much less efficient; a helpful practice when sitting down to grade is to turn off the email, phone, social media, and anything else that might break your concentration.
Grading fairly
There are a few easy things you can do to ensure you’re approaching grading in a fair and consistent way: you can remove names from assignments before grading (which can be easier if assignments are submitted electronically), can make sure you grade assignments in a different order each time (e.g., don’t always go through the stack alphabetically), and, most importantly, you can define clearly (for yourself and for students) exactly what you want students to do.
One way to nail this down and communicate it to students is through the use of rubrics that describe what you’re looking for and explain the basis for your grades. The two main types of rubrics, Holistic and Analytic, approach the matter in different ways. Analytic rubrics delineate a variety of dimensions you’re interested in and, for each dimension, indicate what distinguishes different levels of performance (A, B, C, D, F; Excellent, Successful, Fair, Failing; etc.). Here’s a hypothetical example:

And here’s an example of how an analytic rubric could be applied to a presentation:
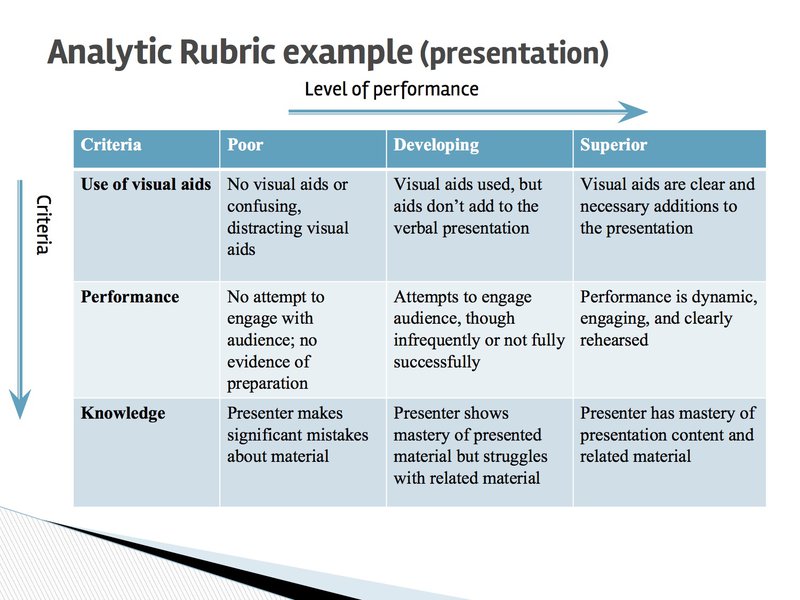
These various dimensions could be weighted equally in the calculation of the grade, or you could give more weight to some and less to others. Either way, the key is to communicate the system and your reasons for adopting it.
Holistic rubrics , on the other hand, are concerned with the overall quality of the work, considering all the dimensions of success at once. For example, here’s a rubric for the participation grade in Georgetown University Professor Betsy Sigman’s Developing and Managing Business Databases course:
- 90-100%—Almost always very well-prepared and has something relevant to say.
- 80-89%—Well-prepared and contributes significantly during the majority of class sessions.
- 70-79%—Adequately prepared and contributes on an occasional basis.
- 60-69%—Adequately prepared but seldom volunteers to speak.
- Below 60%—Inadequately prepared and never voluntarily contributes.
Rather than separate out elements like preparation and frequency and relevance of contributions, these concerns are combined into one overall judgment. This can, of course, be applied to work handed in, such as this excerpt from Georgetown University Professor David Ebenbach’s paper-grading rubric:
- A “B” paper is a good paper, one with strong ideas and a thesis to guide it. All the basic requirements of the assignment are met, and the facts are correct. In many cases, however, the way the ideas are presented makes them somewhat less effective. For example, the ideas may be organized in such a way that the paragraphs could be rearranged easily without changing the quality of the paper notably. There may be some issues with the clarity of the thesis, or some contradictions as well. Overall, however, a “B” paper is a good one, in which the writer basically got her/his point across.
Choosing between holistic and analytic rubrics is a matter of matching the rubric to the situation. Analytic rubrics are particularly useful when you have multiple graders who need to come together to agree on grades, or when you want to focus students’ attention on particular concerns about the work, or where you want to weight different concerns differently. Holistic rubrics, on the other hand, tend to be a good fit for experienced graders, or any situation where you want to emphasize overall quality rather than specific elements.
Find out more about rubrics at our Assessment Portal . You may also want to consider alternative modes of grading .
Also, keep an eye open for the possibility of bias. The human mind depends on unconscious mental shortcuts and generalizations just to get through the day, so we all regularly do this kind of heuristic thinking. When these shortcuts intersect with identity groups, they can be dangerous. For example, maybe, without even realizing it, you have a picture in your mind of what a “good student in the major” looks like, and maybe that picture has a very particular demographic profile. Or maybe you see a particular kind of name on your roster and think, completely unintentionally and perhaps even unconsciously, “That student is going to have trouble writing in the English language.” Those are examples of implicit bias, and they can attach to race, nationality, class, religion, gender, sexuality, or any of a number of other identity-related categories, and they can lead to an approach to grading that’s not equally fair for all of your students. Again, we’re all equipped with brains that work this way, so we all have bias of one form or another (or, likely, multiple forms). The appropriate question isn’t Who’s biased? but What are my biases, and what am I going to do about them? See our inclusive pedagogy page for ideas on how to counter bias. Or check out our inclusive pedagogy toolkit for ideas and tips on bias, assessment, and a variety of other areas you can address to make your class more accessible to all students.
Additional resources
- Center for New Designs in Learning and Scholarship, Alternative Modes of Grading .
- University of Michigan Center for Research on Learning and Teaching. Testing and Grading . Includes comments on the grading of exams and lab reports.
- Vanderbilt University Center for Teaching. Grading Student Work .
- Walvoord, Barbara and Anderson, Virginia Johnson. (2009). Effective Grading: A Tool for Learning and Assessment in College .
Please reach out to us at [email protected] if you’d like to have a conversation with someone at CNDLS about these or other teaching issues.
An automated essay scoring systems: a systematic literature review
New citation alert added.
This alert has been successfully added and will be sent to:
You will be notified whenever a record that you have chosen has been cited.
To manage your alert preferences, click on the button below.
New Citation Alert!
Please log in to your account
Information & Contributors
Bibliometrics & citations, view options.
- Takaki P Dutra M (2024) Text mining applied to distance higher education: A systematic literature review Education and Information Technologies 10.1007/s10639-023-12235-0 29 :9 (10851-10878) Online publication date: 1-Jun-2024 https://dl.acm.org/doi/10.1007/s10639-023-12235-0
- Zafievsky D Lagutina N Melnikova O Poletaev A (2023) Text Model for the Automatic Scoring of Business Letter Writing Automatic Control and Computer Sciences 10.3103/S0146411623070167 57 :7 (828-840) Online publication date: 1-Dec-2023 https://dl.acm.org/doi/10.3103/S0146411623070167
- Masikisiki B Marivate V Hlophe Y (2023) Investigating the Efficacy of Large Language Models in Reflective Assessment Methods through Chain of Thought Prompting Proceedings of the 4th African Human Computer Interaction Conference 10.1145/3628096.3628747 (44-49) Online publication date: 27-Nov-2023 https://dl.acm.org/doi/10.1145/3628096.3628747
- Show More Cited By
Recommendations
Essay scoring tool by employing roberta architecture.
The automated essay scoring (AES) has significant importance in machine grading of student essays particularly in standardized exams like the Graduate Record Examination (GRE). However, some issues in AES have remained unsolved over the past several ...
Fully Automated Short Answer Scoring of the Trial Tests for Common Entrance Examinations for Japanese University
Studies on automated short-answer scoring (SAS) have been conducted to apply natural language processing to education. Short-answer scoring is a task to grade the responses from linguistic information. Most answer sheets for short-answer questions ...
Automated Essay Scoring via Example-Based Learning
Automated essay scoring (AES) is the task of assigning grades to essays. It can be applied for quality assessment as well as pricing on User Generated Content. Previous works mainly consider using the prompt information for scoring. However, some ...
Information
Published in.
Kluwer Academic Publishers
United States
Publication History
Author tags.
- Short answer scoring
- Essay grading
- Natural language processing
- Deep learning
- Research-article
Contributors
Other metrics, bibliometrics, article metrics.
- 14 Total Citations View Citations
- 0 Total Downloads
- Downloads (Last 12 months) 0
- Downloads (Last 6 weeks) 0
- Messer M Brown N Kölling M Shi M Laakso M Monga M Simon Sheard J (2023) Machine Learning-Based Automated Grading and Feedback Tools for Programming: A Meta-Analysis Proceedings of the 2023 Conference on Innovation and Technology in Computer Science Education V. 1 10.1145/3587102.3588822 (491-497) Online publication date: 29-Jun-2023 https://dl.acm.org/doi/10.1145/3587102.3588822
- Uto M Aomi I Tsutsumi E Ueno M (2023) Integration of Prediction Scores From Various Automated Essay Scoring Models Using Item Response Theory IEEE Transactions on Learning Technologies 10.1109/TLT.2023.3253215 16 :6 (983-1000) Online publication date: 1-Dec-2023 https://dl.acm.org/doi/10.1109/TLT.2023.3253215
- Lin J Song J Zhou Z Chen Y Shi X (2023) Automated scholarly paper review Information Fusion 10.1016/j.inffus.2023.101830 98 :C Online publication date: 26-Jul-2023 https://dl.acm.org/doi/10.1016/j.inffus.2023.101830
- Cai Y Mao S Wang C Ge T Wu W Xia Y Zheng C Guan Q (2023) Enhancing Detailed Feedback to Chinese Writing Learners Using a Soft-Label Driven Approach and Tag-Aware Ranking Model Natural Language Processing and Chinese Computing 10.1007/978-3-031-44693-1_45 (576-587) Online publication date: 12-Oct-2023 https://dl.acm.org/doi/10.1007/978-3-031-44693-1_45
- Seßler K Xiang T Bogenrieder L Kasneci E (2023) PEER: Empowering Writing with Large Language Models Responsive and Sustainable Educational Futures 10.1007/978-3-031-42682-7_73 (755-761) Online publication date: 4-Sep-2023 https://dl.acm.org/doi/10.1007/978-3-031-42682-7_73
- Nguyen H Stec H Hou X Di S McLaren B (2023) Evaluating ChatGPT’s Decimal Skills and Feedback Generation in a Digital Learning Game Responsive and Sustainable Educational Futures 10.1007/978-3-031-42682-7_19 (278-293) Online publication date: 4-Sep-2023 https://dl.acm.org/doi/10.1007/978-3-031-42682-7_19
- Pande J Min W Spain R Saville J Lester J (2023) Robust Team Communication Analytics with Transformer-Based Dialogue Modeling Artificial Intelligence in Education 10.1007/978-3-031-36272-9_52 (639-650) Online publication date: 3-Jul-2023 https://dl.acm.org/doi/10.1007/978-3-031-36272-9_52
View options
Login options.
Check if you have access through your login credentials or your institution to get full access on this article.
Full Access
Share this publication link.
Copying failed.
Share on social media
Affiliations, export citations.
- Please download or close your previous search result export first before starting a new bulk export. Preview is not available. By clicking download, a status dialog will open to start the export process. The process may take a few minutes but once it finishes a file will be downloadable from your browser. You may continue to browse the DL while the export process is in progress. Download
- Download citation
- Copy citation
We are preparing your search results for download ...
We will inform you here when the file is ready.
Your file of search results citations is now ready.
Your search export query has expired. Please try again.
Navigation Menu
Search code, repositories, users, issues, pull requests..., provide feedback.
We read every piece of feedback, and take your input very seriously.
Saved searches
Use saved searches to filter your results more quickly.
To see all available qualifiers, see our documentation .
- Notifications You must be signed in to change notification settings
This project is a machine learning application that predicts the score of an essay based on its content. It utilizes a Long Short-Term Memory (LSTM) neural network along with Word2Vec embeddings for text processing.
Soumedhik/Essay-Grading-System
Folders and files.
Name | Name | |||
---|---|---|---|---|
65 Commits | ||||
Repository files navigation
Essay Grading System
This project, titled "Essay Grading with LSTM Model," is a machine learning application that predicts the grade of an essay using a Long Short-Term Memory (LSTM) model trained on Word2Vec embeddings. Users input an essay, which undergoes preprocessing to remove stopwords, lemmatize words, and convert them to vectors. These vectors are then fed into the LSTM model, which predicts the essay grade.
Streamlit Demo
Check out the live demo of the Essay Grading System using Streamlit:
This project was developed by Archisman Ray, Soumedhik Bharati, and Sohoom Lal Banerjee for the SIT Hackathon. It was created as part of an effort to automate the grading process of essays using machine learning techniques.
Model Links
Installation.
To run the application locally, follow these steps:
Clone the repository:
Install the required dependencies:
Download the pre-trained models from the provided links and place them in the project directory.
Run the application:
Pre-trained Models
Download the pre-trained LSTM models from the following links:

This project is licensed under the MIT License. See the LICENSE file for details.
Contributors 3
- Jupyter Notebook 99.3%
- Python 0.7%
IEEE Account
- Change Username/Password
- Update Address
Purchase Details
- Payment Options
- Order History
- View Purchased Documents
Profile Information
- Communications Preferences
- Profession and Education
- Technical Interests
- US & Canada: +1 800 678 4333
- Worldwide: +1 732 981 0060
- Contact & Support
- About IEEE Xplore
- Accessibility
- Terms of Use
- Nondiscrimination Policy
- Privacy & Opting Out of Cookies
A not-for-profit organization, IEEE is the world's largest technical professional organization dedicated to advancing technology for the benefit of humanity. © Copyright 2024 IEEE - All rights reserved. Use of this web site signifies your agreement to the terms and conditions.
Advertisement
Supported by
Fact-Checking Claims About Tim Walz’s Record
Republicans have leveled inaccurate or misleading attacks on Mr. Walz’s response to protests in the summer of 2020, his positions on immigration and his role in the redesign of Minnesota’s flag.
- Share full article

By Linda Qiu
Since Gov. Tim Walz of Minnesota was announced as the Democratic nominee for vice president, the Trump campaign and its allies have gone on the attack.
Mr. Walz, a former teacher and football coach from Nebraska who served in the National Guard, was elected to the U.S. House of Representatives in 2006 and then as Minnesota’s governor in 2018. His branding of former President Donald J. Trump as “weird” this year caught on among Democrats and helped catapult him into the national spotlight and to the top of Vice President Kamala Harris’s list of potential running mates.
The Republican accusations, which include questions over his military service , seem intended at undercutting a re-energized campaign after President Biden stepped aside and Ms. Harris emerged as his replacement at the top of the ticket. Mr. Trump and his allies have criticized, sometimes inaccurately, Mr. Walz’s handling of protests in his state, his immigration policies, his comments about a ladder factory and the redesign of his state’s flag.
Here’s a fact check of some claims.
What Was Said
“Because if we remember the rioting in the summer of 2020, Tim Walz was the guy who let rioters burn down Minneapolis.” — Senator JD Vance of Ohio, the Republican nominee for vice president, during a rally on Wednesday in Philadelphia
This is exaggerated. Mr. Walz has faced criticism for not quickly activating the National Guard to quell civil unrest in Minneapolis in the summer of 2020 after the murder of George Floyd by a police officer. But claims that he did not respond at all, or that the city burned down, are hyperbolic.
Mr. Floyd was murdered on May 25, 2020, and demonstrators took to the streets the next day . The protests intensified, with some vandalizing vehicles and setting fires. More than 700 state troopers and officers with the Minnesota Department of Natural Resources’ mobile response team were deployed on May 26 to help the city’s police officers, according to a 2022 independent assessment by the state’s Department of Public Safety of the response to the unrest.
We are having trouble retrieving the article content.
Please enable JavaScript in your browser settings.
Thank you for your patience while we verify access. If you are in Reader mode please exit and log into your Times account, or subscribe for all of The Times.
Thank you for your patience while we verify access.
Already a subscriber? Log in .
Want all of The Times? Subscribe .
Automatic Essay Grading System Using Deep Neural Network
- Conference paper
- First Online: 02 October 2023
- Cite this conference paper
- Vikkurty Sireesha 6 ,
- Nagaratna P. Hegde 6 ,
- Sriperambuduri Vinay Kumar 6 ,
- Alekhya Naravajhula 7 &
- Dulugunti Sai Haritha 8
Part of the book series: Cognitive Science and Technology ((CSAT))
Included in the following conference series:
- International Conference on Information and Management Engineering
250 Accesses
Essays are important for testing students’ academic scores, creativity, and being able to remember what they studied, but grading them manually is really expensive and time-consuming for a large number of essays. This project aims to implement and train neural networks to assess and grade essays automatically. The human grades given to the essays should be matched with grades generated from our automatic essay grading system consistently with minimum error. Automated essay grading can be used for evaluating essays written according to specific prompts or specific topics. It is the process of automating scoring system of essays without any human intervention and using computer programs. This system is most beneficial for educators since it helps reducing manual work and saves a lot of time. It not only saves a lot of time but also speeds up the process of learning feedback. We used deep neural networks in our system instead of traditional machine learning models.
This is a preview of subscription content, log in via an institution to check access.
Access this chapter
Subscribe and save.
- Get 10 units per month
- Download Article/Chapter or eBook
- 1 Unit = 1 Article or 1 Chapter
- Cancel anytime
- Available as PDF
- Read on any device
- Instant download
- Own it forever
- Available as EPUB and PDF
- Durable hardcover edition
- Dispatched in 3 to 5 business days
- Free shipping worldwide - see info
Tax calculation will be finalised at checkout
Purchases are for personal use only
Institutional subscriptions
Similar content being viewed by others
Smart Grading System Using Bi LSTM with Attention Mechanism
Automatically Grading Brazilian Student Essays
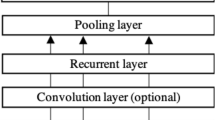
A review of deep-neural automated essay scoring models
Dong F, Zhang Y, Yang J (2017) Attention-based recurrent convolutional neural network for automatic essay scoring. In: Proceedings of the 21st conference on computational natural language learning (CoNLL 2017), pp153–162.
Google Scholar
dos Santos CN, Gatti M. Deep convolutional neural networks for sentiment analysis of short texts. In: Proceedings of the 25th international conference on computational linguistics (COLING), Dublin, Ireland
Alikaniotis D, Yannakoudakis H, Rei M (2016) Automatic text scoring using neural networks. ArXiv:1606.04289
Boulanger D, Kumar V (2019) Shedding light on the automated essay scoring process. In Proceedings of the 12th international conference on educational data mining (EDM)
Liang G, On B-W, Jeong D, Kim H-C, Choi G (2018) Automated essay scoring: a Siamese bidirectional LSTM neural network architecture. Symmetry 10(12):682
Article Google Scholar
Cozma M, Butnaru AM, Ionescu RT (2018) Automated essay scoring with string kernels and word embeddings. ArXiv:1804.07954
Download references
Acknowledgements
We thank Vasavi College of Engineering (Autonomous), Hyderabad for the support extended toward this work.
Author information
Authors and affiliations.
Vasavi College of Engineering, Hyderabad, India
Vikkurty Sireesha, Nagaratna P. Hegde & Sriperambuduri Vinay Kumar
Accolite Digital, Hyderabad, India
Alekhya Naravajhula
Providence, Hyderabad, India
Dulugunti Sai Haritha
You can also search for this author in PubMed Google Scholar
Corresponding author
Correspondence to Vikkurty Sireesha .
Editor information
Editors and affiliations.
BioAxis DNA Research Centre Private Limited, Hyderabad, Andhra Pradesh, India
Department of Computer Science, Brunel University, Uxbridge, UK
Gheorghita Ghinea
CMR College of Engineering and Technology, Hyderabad, India
Suresh Merugu
Rights and permissions
Reprints and permissions
Copyright information
© 2023 The Author(s), under exclusive license to Springer Nature Singapore Pte Ltd.
About this paper
Cite this paper.
Sireesha, V., Hegde, N.P., Kumar, S.V., Naravajhula, A., Haritha, D.S. (2023). Automatic Essay Grading System Using Deep Neural Network. In: Kumar, A., Ghinea, G., Merugu, S. (eds) Proceedings of the 2nd International Conference on Cognitive and Intelligent Computing. ICCIC 2022. Cognitive Science and Technology. Springer, Singapore. https://doi.org/10.1007/978-981-99-2746-3_53
Download citation
DOI : https://doi.org/10.1007/978-981-99-2746-3_53
Published : 02 October 2023
Publisher Name : Springer, Singapore
Print ISBN : 978-981-99-2745-6
Online ISBN : 978-981-99-2746-3
eBook Packages : Intelligent Technologies and Robotics Intelligent Technologies and Robotics (R0)
Share this paper
Anyone you share the following link with will be able to read this content:
Sorry, a shareable link is not currently available for this article.
Provided by the Springer Nature SharedIt content-sharing initiative
- Publish with us
Policies and ethics
- Find a journal
- Track your research

IMAGES
COMMENTS
This paper aims to provide a systematic literature review (SLR) on automated essay grading systems. An SLR is an Evidence-based systematic review to summarize the existing research. It critically evaluates and integrates all relevant studies' findings and addresses the research domain's specific research questions.
In the new grading system, students will have a clearer picture of where they stand in their academic progress thanks to standards-based rubrics and feedback. They will also have additional opportunities to show mastery as opposed to one make-or-break test, including student projects and presentations.
The Grading System: Completely Necessary Grades are an important part of the school system. Grades set the extraordinary students apart from the ordinary ones. In Jerry Farber's essay, "A Young Person's Guide to the Grading System," he argues that grades are the only motivation students have in school. Farber even calls it "phony ...
The grading system can be expressed in four primary ways. It can either be in terms of letters whereby different letters represent one's performance and these letters are assigned numeric values. For example, the best letter grade is an A with a numeric value of 4 while the worst grade is F with a numeric value of 0. It.
As a result, automated essay scoring systems generate a single score or detailed evaluation of predefined assessment features. This chapter describes the evolution and features of automated scoring systems, discusses their limitations, and concludes with future directions for research and practice.
Essay on Grading System Reform Good Essays 984 Words 4 Pages 1 Works Cited Open Document Grading System Reform Teachers have always used grades to measure the amount a student has learned. This practice is becoming ineffective. Many students have a wide range of grades, which show that grades may not show what a student really knows.
Essay scoring: Automated Essay Scoring is the task of assigning a score to an essay, usually in the context of assessing the language ability of a language learner. The quality of an essay is affected by the following four primary dimensions: topic relevance, organization and coherence, word usage and sentence complexity, and grammar and mechanics.
Grading Essays Grade for Learning Objectives Response to Writing Errors Commenting on Student Papers Plagiarism and Grading Information about grading student writing also appears in the Grading Student Work section of the Teaching Guide. Here are some general guidelines to keep in mind when grading student writing.
Furthermore, AI-based essay grading systems eliminate human biases and inconsistencies, levelling the playing field for students. These applications leverage advanced natural language processing techniques to analyse essays and provide constructive suggestions for improvement.
How does the e-rater engine grade essays? The e-rater engine provides a holistic score for an essay that has been entered into the computer electronically. It also provides real-time diagnostic feedback about grammar, usage, mechanics, style and organization, and development.
The automated grading of essay finds the syntactic and semantic features from student answers and reference answers. Then construct a machine learning model that relates these features to the final scores assigned by evaluators. This trained model is used to find score of unseen essays.
Automated grading, if proven to match or exceed the reliability of human graders, will signi cantly reduce costs. The purpose of this project is to implement and train machine learning algorithms to automatically assess and grade essay responses. These grades from the automatic grading system should match the human grades consistently.
Many researchers are working on automated essay grading and short answer scoring for the last few decades, but assessing an essay by considering all parameters like the relevance of the content to ...
developed an automated essay-grading sys-tem called Project Essay Grader. He started with a set of student essays that teachers had already graded. He then experimented with a variety of automatically extractable textual features and applied multiple linear regres-sion to determine an optimal combination of weighted features that best predicted the teachers'grades. His system could then ...
Us and UK Education System. The education systems differ depending on different countries' cultures, priorities and values. This essay points out the differences between the education systems in the United Kingdom and the United States. Despite having shared similarities in the funding structures, the two methods differ in evaluating and ...
This paper aims to provide a systematic literature review (SLR) on automated essay grading systems. An SLR is an Evidence-based systematic review to summarize the existing research. It critically evaluates and integrates all relevant studies' findings and addresses the research domain's specific research questions.
In other words, when devising a system for grading student work, you need to keep in mind your learning goals for those students, so that you (a) evaluate students on dimensions that you care about, dimensions that are relevant to those goals, and (b) so that your feedback can help them pursue those goals.
Many researchers are working on automated essay grading and short answer scoring for the last few decades, but assessing an essay by considering all parameters like the relevance of the content to the prompt, development of ideas, Cohesion, and Coherence is a big challenge till now.
Accordingly, automated essay grading (AEG) systems, or automated essay scoring (AES systems, are de fined as a computer-based process. of applying standardized measurements on open-ended or ...
Automated essay grading has become an important area of research in natural language processing. In this paper, we present a new approach for essay grading using BERT language model with Convolutional Neural Networks and Long Short-Term Memory networks on the Automated Student Prize Assessment dataset. The proposed essay grader evaluates essays ...
Summary This project, titled "Essay Grading with LSTM Model," is a machine learning application that predicts the grade of an essay using a Long Short-Term Memory (LSTM) model trained on Word2Vec embeddings. Users input an essay, which undergoes preprocessing to remove stopwords, lemmatize words, and convert them to vectors.
The system can be able to integrate with existing learning management systems. The goal is to provide a more efficient and accurate essay grading process, so the teachers can provide valuable feedback to students. The proposed work aims to develop an automated essay grading system using AI technology.
A judge on Monday blocked the Texas Education Agency from releasing statewide A-F accountability school rankings this week as planned. The order, issued by Travis County District Court Judge Karin ...
Abstract Automated essay grading has become an important area of research in natural language processing. In this paper, we present a new approach for essay grading using BERT language model with Convolutional Neural Networks and Long Short-Term Memory networks on the Automated Student Prize Assessment dataset. The proposed essay grader evaluates essays based on several writing traits such as ...
Republicans have leveled inaccurate or misleading attacks on Mr. Walz's response to protests in the summer of 2020, his positions on immigration and his role in the redesign of Minnesota's flag.
Essays are important for testing students' academic scores, creativity, and being able to remember what they studied, but grading them manually is really expensive and time-consuming for a large number of essays. This project aims to implement and train neural...