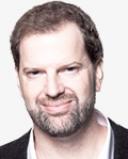

Critical Thinking: What It Is and Why It Matters
Defining critical thinking dispositions and why they’re crucial..
Posted September 23, 2024 | Reviewed by Devon Frye
- Another way to think about and measure critical thinking is to include aspects of motivational dispositions.
- Dispositions include open-mindedness and a willingness to be reflective when evaluating information.
- People scoring low in critical thinking dispositions tend to “keep it simple” when something is complex.
- Critical thinking dispositions help individuals avoid oversimplification and can facilitate awareness of bias.
Critical thinking springs from the notion of reflective thought proposed by Dewey (1933), who borrowed from the work of philosophers such as William James and Charles Peirce. Reflective thought was defined as the process of suspending judgment, remaining open-minded, maintaining a healthy skepticism, and taking responsibility for one’s own development (Gerber et al., 2005; Stoyanov & Kirshner, 2007).
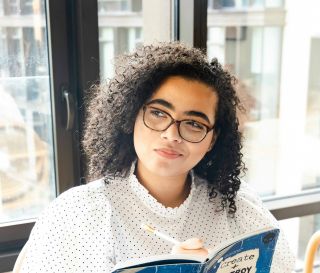
Kurland (1995) suggested, “Critical thinking is concerned with reason, intellectual honesty, and open-mindedness, as opposed to emotionalism, intellectual laziness, and closed-mindedness. Thus, critical thinking involves… considering all possibilities… being precise; considering a variety of possible viewpoints and explanations; weighing the effects of motives and biases; being concerned more with finding the truth than with being right…being aware of one’s own prejudices and biases” (p. 3). Thus, being able to perspective-take and becoming conscious of one’s own biases are potential benefits of critical thinking capacities.
Reviews of the critical thinking literature (e.g., Bensley, 2023) suggest that the assessment of this construct ought to include aspects of motivational dispositions. Numerous frameworks of critical thinking dispositions have been proposed (e.g., Bensley, 2018; Butler & Halpern, 2019; Dwyer, 2017); some commonly identified dispositions are open-mindedness, intellectual engagement, and a proclivity to take a reflective stance or approach to evaluating information and the views and beliefs of both oneself and others. Demir (2022) posited that critical thinking dispositions reflect persons’ attitudes toward and routine ways of responding to new information and diverging ideas, willingness to engage in nuanced and complex rather than either/or reductionistic thinking, and perseverance in attempts to understand and resolve complex problems.
Other examples of dispositions are inquisitiveness, open-mindedness, tolerance for ambiguity, thinking about thinking, honesty in assessing or evaluating biases, and willingness to reconsider one’s own views and ways of doing things (Facione et al., 2001). Individual personality attributes associated with these proclivities include a need for cognition (a desire for intellectual stimulation), which is positively associated with critical thinking, and the need for closure (a motivated cognitive style in which individuals prefer predictability, firm answers, and rapid decision making ) and anti-intellectualism (a resentment of “the life of the mind” and those who represent it), both negatively associated with critical thinking.
Further, an ideological component that can impede critical thinking is dogmatism . In addition, rigid, dichotomous thinking impedes critical thinking in that it oversimplifies the complexity of social life in a pluralistic society (Bensley, 2023; Cheung et al., 2002; Halpern & Dunn, 2021) and tries to reduce complicated phenomena and resolve complex problems via “either/or” formulations and simplistic solutions.
In other words, folks with low critical thinking dispositions would tend to “keep it simple” when something is really quite complicated, and think it absolute terms and categories rather than seeing “the gray” in between the black and white extremes.
In sum, critical thinking dispositions are vitally important because they may help individuals avoid oversimplifying reality; they also permit perspective-taking and can facilitate their awareness of diversity and systematic biases, such as racial or gender bias . Some research has indicated that critical thinking dispositions uniquely contribute to academic performance beyond general cognition (Ren et al., 2020), and may help to reduce unsubstantiated claims and conspiracy beliefs (Bensley, 2023; Lantian et al., 2021).
But before we can study the potential impact of critical thinking dispositions, it is necessary to have a reliable, valid, and hopefully brief measure for this construct. I will discuss the development and validation of a measure of critical thinking dispositions in another post.
Bensley, D.A. ( 2023.) Critical thinking, intelligence, and unsubstantiated beliefs: An integrative review. Journal of Intelligence, 1 , 207. https://doi.org/10.3390/jintelligence11110207
Bensley, D.A. (2018). Critical thinking in psychology and everyday life: A guide to effective thinking . New York: Worth Publishers.
Butler, H.A., & Halpern, D.F. (2019). Is critical thinking a better model of intelligence? In Robert J. Sternberg (Ed.) The Nature of Intelligence (pp. 183–96). Cambridge: Cambridge University Press.
Cheung, C.-K, Rudowicz. E., Kwan, A., & Yue, X.. (2002). Assessing university students’ general and specific criticalthinking. College Student Journal, 36 , 504 – 25.
Demir, E. (2022). An examination of high school students’ critical thinking dispositions and analytical thinking skills. Journal of Pedagogical Research, 6 , 190–200. https://doi.org/10.33902/JPR.202217357
Dewey, J. (1933). How we think: A restatement of the relation of reflective thinking to the educative process . Lexington: Heath and Company.
Dwyer, C. P. (2017). Critical thinking: Conceptual perspectives and practical guidelines . Cambridge: CambridgeUniversity Press.
Facione, P., Facione, N,C,, & Giancarlo, C.A.F. (2001(. California Critical Disposition Inventory . Millbrae: California Academic Press.
Gerber, S., Scott, L., Clements, D.H., & Sarama, J. (2005). Instructor influence on reasoned argument in discussion boards. Educational Technology, Research & Development, 53 , 25–39. https://doi.org/10.1007/BF02504864
Halpern, D. F., & Dunn, D.S. (2021). Critical thinking: A model of intelligence for solving real-world problems. Journal of Intelligence, 9 , 22. https://doi.org/10.3390/jintelligence9020022
Kurland, D. (1995). I know what it says… What does it mean? Critical skills for critical reading . Belmont: Wadsworth.
Lantian, A., Bagneux, V., Delouvee, S., & Gauvrit, N. (2021). Maybe a free thinker but not a critical one: High conspiracybelief is associated with low critical thinking ability. Applied Cognitive Psychology, 35 , 674 – 84. https://doi.org/10.1002/acp.3790
Ren, X., Tong, Y., Peng, P. & Wang, T. (2020). Critical thinking predicts academic performance beyond general cognitiveability: Evidence from adults and children. Intelligence, 82 , 101487. https://doi.org/10.1016/j.intell.2020.101487
Stoyanov, S., & Kirschner, P. ( 2007). Effect of problem solving support and cognitive styles on idea generation:Implications for technology-enhanced learning. Journal of Research on Technology in Education, 40 , 49–63. https://doi.org/10.1080/15391523.2007.10782496
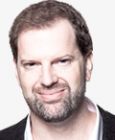
Kyle D. Killian, Ph.D., LMFT is the author of Interracial Couples, Intimacy and Therapy: Crossing Racial Borders.
- Find a Therapist
- Find a Treatment Center
- Find a Psychiatrist
- Find a Support Group
- Find Online Therapy
- United States
- Brooklyn, NY
- Chicago, IL
- Houston, TX
- Los Angeles, CA
- New York, NY
- Portland, OR
- San Diego, CA
- San Francisco, CA
- Seattle, WA
- Washington, DC
- Asperger's
- Bipolar Disorder
- Chronic Pain
- Eating Disorders
- Passive Aggression
- Personality
- Goal Setting
- Positive Psychology
- Stopping Smoking
- Low Sexual Desire
- Relationships
- Child Development
- Self Tests NEW
- Therapy Center
- Diagnosis Dictionary
- Types of Therapy
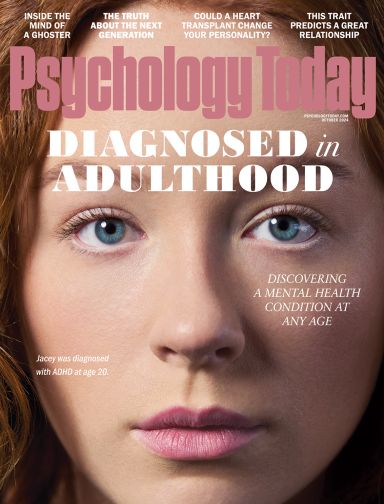
It’s increasingly common for someone to be diagnosed with a condition such as ADHD or autism as an adult. A diagnosis often brings relief, but it can also come with as many questions as answers.
- Emotional Intelligence
- Gaslighting
- Affective Forecasting
- Neuroscience

Is Intelligence Genetic? What the Science Says
Published: 30/11/2023
Written by: Mike Battista, Director of Science & Research
As our population ages, brain health is becoming an increasing concern in patient care. Questions about brain health may concern cognitive performance, memory, verbal ability, or even general intelligence.
But what we call “intelligence” is actually a complex system of brain structures, cognitive functions, and expressions of skill. Research shows that multiple neural systems actually contribute together to what we traditionally think of as expressions of intelligence .
While neural systems are partially shaped by genetic factors, there’s still plenty that patients can do to keep cognitive abilities at their best. Other factors that go into the development of intelligence can include physical fitness, learning new skills, healthy habits, and social connectedness.
When it comes to communicating the complex traits and nature of intelligence to your patients, it may help to be clear about what’s within their control—and what might be in their makeup. Read on for more about the role genetics play in the development of intelligence, and how best to support your patients who want to maintain good cognitive function.
How Much of Intelligence is Genetic?
In a 1994 issue of the Wall Street Journal , Linda S. Gottfredson summarized views of intelligence researchers that have since proved to be highly controversial and inaccurate. However, the statement also provided a useful definition of general intelligence: "a very general mental capability that, among other things, involves the ability to reason, plan, solve problems, think abstractly, comprehend complex ideas, learn quickly and learn from experience."
Many of these skills are acquired through the course of a person’s life. But it’s also true that they come more easily to some people than others.
The reasons for that could be partially explained by genetic predisposition. Genome-wide association studies (GWAS) have been conducted on a wide scale in recent decades trying to get to the bottom of how heritable intelligence is, and which genes are involved.
“The genetic contribution to intelligence differences is highly polygenic,” wrote Ian J. Deary, Simon R. Cox, and W. David Hill in an aggregate study of genetically-linked intelligence data in 2022 . In other words, “there are large numbers of independent genetic variants, each of which accounts for a tiny proportion of intelligence variation.”
The overall degree to which genes account for intelligence is usually estimated to be at least half, or as high as 80%, with the rest accounted for by environment and life experience. However, a strong genetic influence on intelligence does not mean that environment is not important, or that intelligence cannot be changed. As Bruno Sauce and Louis D. Matzel (2018) summarize, there is plenty of evidence that intelligence is both heritable and malleable.
The interplay between genetic and environmental influences is not yet fully understood yet, but acknowledging the importance of both is key to removing barriers to improved brain health, particularly in under-privileged patient populations.
So is there such thing as an “IQ gene”?
While there may well be biological reasons for why one patient’s brain works a certain way, there’s no such thing as a single “IQ gene”—instead, it is likely hundreds of genes working together and interacting with environmental factors to determine cognitive abilities. How much genetic influence plays a part in learning or planning may even vary from person to person.
This said, research is ongoing and growing rapidly in this field. Practitioners may benefit from keeping up to date on the ongoing debates and emerging information when it comes to figuring out how to talk to their patients about brain health.
How Is Intelligence Measured?
We’re all familiar with the concept of IQ tests, which test cognitive abilities such as spatial reasoning, memory, verbal ability, and reaction time.
A full neuropsychological examination that includes intelligence testing can take 4 hours more. Then, after all that, the focus is often on an aggregate IQ number. What happened to the details?
Quicker computerized cognitive tests might be more appropriate in many healthcare contexts, and can measure domains like reasoning , short-term memory , and verbal ability . Getting higher scores in some domains or subdomains than others is normal, and provides more detail than an aggregate number representing an individual’s overall intelligence.
Knowing where an individual’s cognitive strengths and weaknesses are might give practitioners more information on how best to advise their patients. Cognitive tests like these, as well as assessments tailored more narrowly to measure specific areas of cognitive function , can also help to catch early signs of cognitive dysfunction in older demographics .
Use Objective Cognitive Performance Tests in Your Clinical Practice
Backed by over 30 years of research, Creyos provides objective measures of cognitive performance such as memory, attention, verbal reasoning, reaction time, and more. Provide meaningful brain health data for your patients.
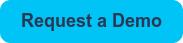
Can a Person Increase Their Intelligence?
The brain is an active, elastic, ever-changing organ. It’s never stagnant—and neither are intelligence skills!
We’ve covered how a number of biological factors, from brain structure to genetics, can affect how a person thinks. The good news is that social and environmental factors, as well as lifestyle factors, can also play a significant role.
People’s always-changing environments and ability to alter their habits means that no one’s intelligence is a fixed trait. Here are just a few ways patients worried about their cognitive abilities can make a difference in their thinking skills.
Read more: Does Brain Training Work?
Engage in Appropriate Exercise
Physical activity can enhance brain function in people of any age. It’s well known that exercise is shown to help with mental health , but it can also help improve performance in nearly every domain of cognition , suggesting that level of physical activity is one non-genetic factor that determines an individual's overall intelligence.
Some studies even show that older adults who exercise regularly are up to 17% less likely to report subjective cognitive decline. Exercise may make the difference in staying sharper for longer.
Intense, aerobic exercise may not be the right fit for every patient. When advising patients, noting that any amount of exercise —whatever is within their ability—may produce health benefits may help them feel empowered to make a beneficial lifestyle change.
Read more: Is Exercise Good for the Brain? Here's What the Science Says.
Learn a New Skill
Every time we learn something new, our brain lights up in a flurry of activity, which may lead to better brain health.
According to Dr. John N. Morris at the Institute of Aging Research , the three skill-building factors that make the biggest difference in cognitive ability are:
- Challenge. Even challenging a patient to reach a new bar in an activity they already enjoy has benefits!
- Complexity . Problem-solving is an important skill in robust cognitive function.
- Ease of practice. Among other things, practicing a skill is a great way to exercise memory. According to Dr. Morris, returning to a skill again and again may be even more important than mastering it.
What about easier activities, like online brain training? We’ve found that simple games have no association with overall cognitive performance . There’s still no quick fix for the brain; improving intelligence requires dedication to complex skills.
Build New—or Stronger—Habits
It’s no secret that cutting down on tobacco and alcohol can help improve health outcomes . But building new habits can be just as beneficial.
Regularly reading a book can create all kinds of positive reactions in the brain . Adults who regularly do puzzles also reflect higher levels of cognitive functions .
Get Quality Sleep
Sleep might be the most important habit of all to build. With strong connections to cognitive and mental health, getting the right amount of sleep is a significant determinant of health. The amount of sleep everyone needs is different, but some studies suggest 7 hours per night is the needed baseline for robust cognitive function.
Talk to Family and Friends
A study of Japanese adults showed that regular social exposure was linked to healthy brain structure and volume. Strengthening thinking skills can be as easy as hanging out, catching up, or even striking up a conversation while walking the dog.
Living with someone, small talk with new people, or weekly classes are all great ways to keep brains healthy and active. Patients could even mix social time with another activity and gain multiple benefits at once. A regular walking date with a neighbor offers a great double-whammy of chatting while exercising.
Read more: How To Prevent Dementia: 12 Modifiable Risk Factors
Final Thoughts: DNA Is Not Destiny
Though our genes and physical bodies have a lot of say on how we move through the world, there’s still plenty patients can do to help improve their thinking skills. Framing intelligence as merely shorthand for a whole network of pathways, structures, chemicals, and skills may help patients feel more at ease with variations in their cognitive performance.
To help guide patients concerned about their brain health, focus on the factors they can control, like skills-building, appropriate exercise, and other relevant lifestyle factors. Another approach may be to look into cognitive tests that measure performance in a number of different areas, instead of reducing intelligence to a single number.
Not only does more comprehensive cognitive information put patients at ease, but it improves your ability to provide quality patient care by pinpointing where actual deficits may exist—and where they don’t.
Empower Patients with Meaningful Brain Health Data
Backed by over 30 years of research, Creyos provides objective measures of cognitive performance that help healthcare providers make more confident decisions about how best to support their cognitive and mental wellbeing.
Join thousands of healthcare professionals who receive a monthly update on the latest research and news in brain health.
An official website of the United States government
Official websites use .gov A .gov website belongs to an official government organization in the United States.
Secure .gov websites use HTTPS A lock ( Lock Locked padlock icon ) or https:// means you've safely connected to the .gov website. Share sensitive information only on official, secure websites.
- Publications
- Account settings
- Advanced Search
- Journal List

Critical Thinking: A Model of Intelligence for Solving Real-World Problems
Diane f halpern, dana s dunn.
- Author information
- Article notes
- Copyright and License information
Correspondence: [email protected]
Received 2021 Jan 13; Accepted 2021 Mar 25; Collection date 2021 Jun.
Licensee MDPI, Basel, Switzerland. This article is an open access article distributed under the terms and conditions of the Creative Commons Attribution (CC BY) license ( https://creativecommons.org/licenses/by/4.0/ ).
Most theories of intelligence do not directly address the question of whether people with high intelligence can successfully solve real world problems. A high IQ is correlated with many important outcomes (e.g., academic prominence, reduced crime), but it does not protect against cognitive biases, partisan thinking, reactance, or confirmation bias, among others. There are several newer theories that directly address the question about solving real-world problems. Prominent among them is Sternberg’s adaptive intelligence with “adaptation to the environment” as the central premise, a construct that does not exist on standardized IQ tests. Similarly, some scholars argue that standardized tests of intelligence are not measures of rational thought—the sort of skill/ability that would be needed to address complex real-world problems. Other investigators advocate for critical thinking as a model of intelligence specifically designed for addressing real-world problems. Yes, intelligence (i.e., critical thinking) can be enhanced and used for solving a real-world problem such as COVID-19, which we use as an example of contemporary problems that need a new approach.
Keywords: critical thinking, intelligence, real-world problems
1. Introduction
The editors of this Special Issue asked authors to respond to a deceptively simple statement: “How Intelligence Can Be a Solution to Consequential World Problems.” This statement holds many complexities, including how intelligence is defined and which theories are designed to address real-world problems.
2. The Problem with Using Standardized IQ Measures for Real-World Problems
For the most part, we identify high intelligence as having a high score on a standardized test of intelligence. Like any test score, IQ can only reflect what is on the given test. Most contemporary standardized measures of intelligence include vocabulary, working memory, spatial skills, analogies, processing speed, and puzzle-like elements (e.g., Wechsler Adult Intelligence Scale Fourth Edition; see ( Drozdick et al. 2012 )). Measures of IQ correlate with many important outcomes, including academic performance ( Kretzschmar et al. 2016 ), job-related skills ( Hunter and Schmidt 1996 ), reduced likelihood of criminal behavior ( Burhan et al. 2014 ), and for those with exceptionally high IQs, obtaining a doctorate and publishing scholarly articles ( McCabe et al. 2020 ). Gottfredson ( 1997, p. 81 ) summarized these effects when she said the “predictive validity of g is ubiquitous.” More recent research using longitudinal data, found that general mental abilities and specific abilities are good predictors of several work variables including job prestige, and income ( Lang and Kell 2020 ). Although assessments of IQ are useful in many contexts, having a high IQ does not protect against falling for common cognitive fallacies (e.g., blind spot bias, reactance, anecdotal reasoning), relying on biased and blatantly one-sided information sources, failing to consider information that does not conform to one’s preferred view of reality (confirmation bias), resisting pressure to think and act in a certain way, among others. This point was clearly articulated by Stanovich ( 2009, p. 3 ) when he stated that,” IQ tests measure only a small set of the thinking abilities that people need.”
3. Which Theories of Intelligence Are Relevant to the Question?
Most theories of intelligence do not directly address the question of whether people with high intelligence can successfully solve real world problems. For example, Grossmann et al. ( 2013 ) cite many studies in which IQ scores have not predicted well-being, including life satisfaction and longevity. Using a stratified random sample of Americans, these investigators found that wise reasoning is associated with life satisfaction, and that “there was no association between intelligence and well-being” (p. 944). (critical thinking [CT] is often referred to as “wise reasoning” or “rational thinking,”). Similar results were reported by Wirthwein and Rost ( 2011 ) who compared life satisfaction in several domains for gifted adults and adults of average intelligence. There were no differences in any of the measures of subjective well-being, except for leisure, which was significantly lower for the gifted adults. Additional research in a series of experiments by Stanovich and West ( 2008 ) found that participants with high cognitive ability were as likely as others to endorse positions that are consistent with their biases, and they were equally likely to prefer one-sided arguments over those that provided a balanced argument. There are several newer theories that directly address the question about solving real-world problems. Prominent among them is Sternberg’s adaptive intelligence with “adaptation to the environment” as the central premise, a construct that does not exist on standardized IQ tests (e.g., Sternberg 2019 ). Similarly, Stanovich and West ( 2014 ) argue that standardized tests of intelligence are not measures of rational thought—the sort of skill/ability that would be needed to address complex real-world problems. Halpern and Butler ( 2020 ) advocate for CT as a useful model of intelligence for addressing real-world problems because it was designed for this purpose. Although there is much overlap among these more recent theories, often using different terms for similar concepts, we use Halpern and Butler’s conceptualization to make our point: Yes, intelligence (i.e., CT) can be enhanced and used for solving a real-world problem like COVID-19.
4. Critical Thinking as an Applied Model for Intelligence
One definition of intelligence that directly addresses the question about intelligence and real-world problem solving comes from Nickerson ( 2020, p. 205 ): “the ability to learn, to reason well, to solve novel problems, and to deal effectively with novel problems—often unpredictable—that confront one in daily life.” Using this definition, the question of whether intelligent thinking can solve a world problem like the novel coronavirus is a resounding “yes” because solutions to real-world novel problems are part of his definition. This is a popular idea in the general public. For example, over 1000 business managers and hiring executives said that they want employees who can think critically based on the belief that CT skills will help them solve work-related problems ( Hart Research Associates 2018 ).
We define CT as the use of those cognitive skills or strategies that increase the probability of a desirable outcome. It is used to describe thinking that is purposeful, reasoned, and goal directed--the kind of thinking involved in solving problems, formulating inferences, calculating likelihoods, and making decisions, when the thinker is using skills that are thoughtful and effective for the particular context and type of thinking task. International surveys conducted by the OECD ( 2019, p. 16 ) established “key information-processing competencies” that are “highly transferable, in that they are relevant to many social contexts and work situations; and ‘learnable’ and therefore subject to the influence of policy.” One of these skills is problem solving, which is one subset of CT skills.
The CT model of intelligence is comprised of two components: (1) understanding information at a deep, meaningful level and (2) appropriate use of CT skills. The underlying idea is that CT skills can be identified, taught, and learned, and when they are recognized and applied in novel settings, the individual is demonstrating intelligent thought. CT skills include judging the credibility of an information source, making cost–benefit calculations, recognizing regression to the mean, understanding the limits of extrapolation, muting reactance responses, using analogical reasoning, rating the strength of reasons that support and fail to support a conclusion, and recognizing hindsight bias or confirmation bias, among others. Critical thinkers use these skills appropriately, without prompting, and usually with conscious intent in a variety of settings.
One of the key concepts in this model is that CT skills transfer in appropriate situations. Thus, assessments using situational judgments are needed to assess whether particular skills have transferred to a novel situation where it is appropriate. In an assessment created by the first author ( Halpern 2018 ), short paragraphs provide information about 20 different everyday scenarios (e.g., A speaker at the meeting of your local school board reported that when drug use rises, grades decline; so schools need to enforce a “war on drugs” to improve student grades); participants provide two response formats for every scenario: (a) constructed responses where they respond with short written responses, followed by (b) forced choice responses (e.g., multiple choice, rating or ranking of alternatives) for the same situations.
There is a large and growing empirical literature to support the assertion that CT skills can be learned and will transfer (when taught for transfer). See for example, Holmes et al. ( 2015 ), who wrote in the prestigious Proceedings of the National Academy of Sciences , that there was “significant and sustained improvement in students’ critical thinking behavior” (p. 11,199) for students who received CT instruction. Abrami et al. ( 2015, para. 1 ) concluded from a meta-analysis that “there are effective strategies for teaching CT skills, both generic and content specific, and CT dispositions, at all educational levels and across all disciplinary areas.” Abrami et al. ( 2008, para. 1 ), included 341 effect sizes in a meta-analysis. They wrote: “findings make it clear that improvement in students’ CT skills and dispositions cannot be a matter of implicit expectation.” A strong test of whether CT skills can be used for real-word problems comes from research by Butler et al. ( 2017 ). Community adults and college students (N = 244) completed several scales including an assessment of CT, an intelligence test, and an inventory of real-life events. Both CT scores and intelligence scores predicted individual outcomes on the inventory of real-life events, but CT was a stronger predictor.
Heijltjes et al. ( 2015, p. 487 ) randomly assigned participants to either a CT instruction group or one of six other control conditions. They found that “only participants assigned to CT instruction improved their reasoning skills.” Similarly, when Halpern et al. ( 2012 ) used random assignment of participants to either a learning group where they were taught scientific reasoning skills using a game format or a control condition (which also used computerized learning and was similar in length), participants in the scientific skills learning group showed higher proportional learning gains than students who did not play the game. As the body of additional supportive research is too large to report here, interested readers can find additional lists of CT skills and support for the assertion that these skills can be learned and will transfer in Halpern and Dunn ( Forthcoming ). There is a clear need for more high-quality research on the application and transfer of CT and its relationship to IQ.
5. Pandemics: COVID-19 as a Consequential Real-World Problem
A pandemic occurs when a disease runs rampant over an entire country or even the world. Pandemics have occurred throughout history: At the time of writing this article, COVID-19 is a world-wide pandemic whose actual death rate is unknown but estimated with projections of several million over the course of 2021 and beyond ( Mega 2020 ). Although vaccines are available, it will take some time to inoculate most or much of the world’s population. Since March 2020, national and international health agencies have created a list of actions that can slow and hopefully stop the spread of COVID (e.g., wearing face masks, practicing social distancing, avoiding group gatherings), yet many people in the United States and other countries have resisted their advice.
Could instruction in CT encourage more people to accept and comply with simple life-saving measures? There are many possible reasons to believe that by increasing citizens’ CT abilities, this problematic trend can be reversed for, at least, some unknown percentage of the population. We recognize the long history of social and cognitive research showing that changing attitudes and behaviors is difficult, and it would be unrealistic to expect that individuals with extreme beliefs supported by their social group and consistent with their political ideologies are likely to change. For example, an Iranian cleric and an orthodox rabbi both claimed (separately) that the COVID-19 vaccine can make people gay ( Marr 2021 ). These unfounded opinions are based on deeply held prejudicial beliefs that we expect to be resistant to CT. We are targeting those individuals who beliefs are less extreme and may be based on reasonable reservations, such as concern about the hasty development of the vaccine and the lack of long-term data on its effects. There should be some unknown proportion of individuals who can change their COVID-19-related beliefs and actions with appropriate instruction in CT. CT can be a (partial) antidote for the chaos of the modern world with armies of bots creating content on social media, political and other forces deliberately attempting to confuse issues, and almost all media labeled “fake news” by social influencers (i.e., people with followers that sometimes run to millions on various social media). Here, are some CT skills that could be helpful in getting more people to think more critically about pandemic-related issues.
Reasoning by Analogy and Judging the Credibility of the Source of Information
Early communications about the ability of masks to prevent the spread of COVID from national health agencies were not consistent. In many regions of the world, the benefits of wearing masks incited prolonged and acrimonious debates ( Tang 2020 ). However, after the initial confusion, virtually all of the global and national health organizations (e.g., WHO, National Health Service in the U. K., U. S. Centers for Disease Control and Prevention) endorse masks as a way to slow the spread of COVID ( Cheng et al. 2020 ; Chu et al. 2020 ). However, as we know, some people do not trust governmental agencies and often cite the conflicting information that was originally given as a reason for not wearing a mask. There are varied reasons for refusing to wear a mask, but the one most often cited is that it is against civil liberties ( Smith 2020 ). Reasoning by analogy is an appropriate CT skill for evaluating this belief (and a key skill in legal thinking). It might be useful to cite some of the many laws that already regulate our behavior such as, requiring health inspections for restaurants, setting speed limits, mandating seat belts when riding in a car, and establishing the age at which someone can consume alcohol. Individuals would be asked to consider how the mandate to wear a mask compares to these and other regulatory laws.
Another reason why some people resist the measures suggested by virtually every health agency concerns questions about whom to believe. Could training in CT change the beliefs and actions of even a small percentage of those opposed to wearing masks? Such training would include considering the following questions with practice across a wide domain of knowledge: (a) Does the source have sufficient expertise? (b) Is the expertise recent and relevant? (c) Is there a potential for gain by the information source, such as financial gain? (d) What would the ideal information source be and how close is the current source to the ideal? (e) Does the information source offer evidence that what they are recommending is likely to be correct? (f) Have you traced URLs to determine if the information in front of you really came from the alleged source?, etc. Of course, not everyone will respond in the same way to each question, so there is little likelihood that we would all think alike, but these questions provide a framework for evaluating credibility. Donovan et al. ( 2015 ) were successful using a similar approach to improve dynamic decision-making by asking participants to reflect on questions that relate to the decision. Imagine the effect of rigorous large-scale education in CT from elementary through secondary schools, as well as at the university-level. As stated above, empirical evidence has shown that people can become better thinkers with appropriate instruction in CT. With training, could we encourage some portion of the population to become more astute at judging the credibility of a source of information? It is an experiment worth trying.
6. Making Cost—Benefit Assessments for Actions That Would Slow the Spread of COVID-19
Historical records show that refusal to wear a mask during a pandemic is not a new reaction. The epidemic of 1918 also included mandates to wear masks, which drew public backlash. Then, as now, many people refused, even when they were told that it was a symbol of “wartime patriotism” because the 1918 pandemic occurred during World War I ( Lovelace 2020 ). CT instruction would include instruction in why and how to compute cost–benefit analyses. Estimates of “lives saved” by wearing a mask can be made meaningful with graphical displays that allow more people to understand large numbers. Gigerenzer ( 2020 ) found that people can understand risk ratios in medicine when the numbers are presented as frequencies instead of probabilities. If this information were used when presenting the likelihood of illness and death from COVID-19, could we increase the numbers of people who understand the severity of this disease? Small scale studies by Gigerenzer have shown that it is possible.
Analyzing Arguments to Determine Degree of Support for a Conclusion
The process of analyzing arguments requires that individuals rate the strength of support for and against a conclusion. By engaging in this practice, they must consider evidence and reasoning that may run counter to a preferred outcome. Kozyreva et al. ( 2020 ) call the deliberate failure to consider both supporting and conflicting data “deliberate ignorance”—avoiding or failing to consider information that could be useful in decision-making because it may collide with an existing belief. When applied to COVID-19, people would have to decide if the evidence for and against wearing a face mask is a reasonable way to stop the spread of this disease, and if they conclude that it is not, what are the costs and benefits of not wearing masks at a time when governmental health organizations are making them mandatory in public spaces? Again, we wonder if rigorous and systematic instruction in argument analysis would result in more positive attitudes and behaviors that relate to wearing a mask or other real-world problems. We believe that it is an experiment worth doing.
7. Conclusions
We believe that teaching CT is a worthwhile approach for educating the general public in order to improve reasoning and motivate actions to address, avert, or ameliorate real-world problems like the COVID-19 pandemic. Evidence suggests that CT can guide intelligent responses to societal and global problems. We are NOT claiming that CT skills will be a universal solution for the many real-world problems that we confront in contemporary society, or that everyone will substitute CT for other decision-making practices, but we do believe that systematic education in CT can help many people become better thinkers, and we believe that this is an important step toward creating a society that values and practices routine CT. The challenges are great, but the tools to tackle them are available, if we are willing to use them.
Author Contributions
Conceptualization, D.F.H. and D.S.D.; resources, D.F.H.; data curation, writing—original draft preparation, D.F.H.; writing—review and editing, D.F.H. and D.S.D. All authors have read and agreed to the published version of the manuscript.
This research received no external funding.
Institutional Review Board Statement
No IRB Review.
Informed Consent Statement
No Informed Consent.
Conflicts of Interest
The authors declare no conflict of interest.
Publisher’s Note: MDPI stays neutral with regard to jurisdictional claims in published maps and institutional affiliations.
- Abrami Philip C., Bernard Robert M., Borokhovski Evgueni, Wade C. Anne, Surkes Michael A., Tamim Rana, Zhang Dai. Instructional interventions affecting critical thinking skills and dispositions: A Stage 1 meta-analysis. Review of Educational Research. 2008;78:1102–34. doi: 10.3102/0034654308326084. [ DOI ] [ Google Scholar ]
- Abrami Philip C., Bernard Robert M., Borokhovski Evgueni, Waddington David I., Wade C. Anne. Strategies for teaching students to think critically: A meta-analysis. Review of Educational Research. 2015;85:275–341. doi: 10.3102/0034654314551063. [ DOI ] [ Google Scholar ]
- Burhan Nik Ahmad Sufian, Kurniawan Yohan, Sidek Abdul Halim, Mohamad Mohd Rosli. Crimes and the Bell curve: Th e role of people with high, average, and low intelligence. Intelligence. 2014;47:12–22. doi: 10.1016/j.intell.2014.08.005. [ DOI ] [ Google Scholar ]
- Butler Heather A., Pentoney Christopher, Bong Maebelle P. Predicting real-world outcomes: Critical thinking ability is a better predictor of life decisions than intelligence. Thinking Skills and Creativity. 2017;25:38–46. doi: 10.1016/j.tsc.2017.06.005. [ DOI ] [ Google Scholar ]
- Cheng Vincent Chi-Chung, Wong Shuk-Ching, Chuang Vivien Wai-Man, So Simon Yung-Chun, Chen Jonathan Hon-Kwan, Sridhar Sidharth, To Kelvin Kai-Wwang, Chan Jasper Fuk-Wu, Hung Ivan Fan-Ngai, Ho Pak-Leung, et al. The role of community-wide wearing of face mask for control of coronavirus disease 2019 (COVID-19) epidemic due to SARS-CoV-2. Journal of Infectious Disease. 2020;81:107–14. doi: 10.1016/j.jinf.2020.04.024. [ DOI ] [ PMC free article ] [ PubMed ] [ Google Scholar ]
- Chu Derek K., Aki Elie A., Duda Stephanie, Solo Karla, Yaacoub Sally, Schunemann Holger J. Physical distancing, face masks, and eye protection to prevent person-to-person transmission of SARS-CoV-2 and COVID-19: A system atic review and meta-analysis. Lancet. 2020;395:1973–87. doi: 10.1016/S0140-6736(20)31142-9. [ DOI ] [ PMC free article ] [ PubMed ] [ Google Scholar ]
- Donovan Sarah J., Guss C. Dominick, Naslund Dag. Improving dynamic decision-making through training and self-re flection. Judgment and Decision Making. 2015;10:284–95. [ Google Scholar ]
- Drozdick Lisa Whipple, Wahlstrom Dustin, Zhu Jianjun, Weiss Lawrence G. The Wechsler Adult Intelligence Scale—Fourth Edition and the Wechsler Memory Scale—Fourth Edition. In: Flanagan Dawn P., Harrison Patti L., editors. Contemporary Intellectual as Sessment: Theories, Tests, and Issues. The Guilford Press; New York: 2012. pp. 197–223. [ Google Scholar ]
- Gigerenzer Gerd. When all is just a click away: Is critical thinking obsolete in the digital age? In: Sternberg Robert J., Halpern Diane F., editors. Critical Thinking IN Psychology. 2nd ed. Cambridge University Press; Cambridge: 2020. pp. 197–223. [ Google Scholar ]
- Gottfredson Linda S. Why g matters: The complexity of everyday life. Intelligence. 1997;24:79–132. doi: 10.1016/S0160-2896(97)90014-3. [ DOI ] [ Google Scholar ]
- Grossmann Igor, Varnum Michael E. W., Na Jinkyung, Kitayama Shinobu, Nisbett Richard E. A route to well-being: Intelligence ver sus wise reasoning. Journal of Experimental Psychology: General. 2013;142:944–53. doi: 10.1037/a0029560. [ DOI ] [ PMC free article ] [ PubMed ] [ Google Scholar ]
- Halpern Diane F. Halpern Critical Thinking Assessment. Schuhfried Test Publishers; Modling: 2018. [(accessed on 30 March 2021)]. Available online: www.schuhfried.com . [ Google Scholar ]
- Halpern Diane F., Butler Heather A. Is critical thinking a better model of intelligence? In: Sternberg Robert J., editor. The nature of Intelligence. 2nd ed. Cambridge University Press; Cambridge: 2020. pp. 183–96. [ Google Scholar ]
- Halpern Diane F., Dunn Dana S. Thought and Knowledge: An Introduction to Critical Thinking. 6th ed. Taylor & Francis; New York: Forthcoming. in press. [ Google Scholar ]
- Halpern Diane F., Millis Keith, Graesser Arthur, Butler Heather, Forsyth Carol, Cai Zhiqiang. Operation ARA: A computerized learn ing game that teaches critical thinking and scientific reasoning. Thinking Skills and Creativity. 2012;7:93–100. doi: 10.1016/j.tsc.2012.03.006. [ DOI ] [ Google Scholar ]
- Hart Research Associates [(accessed on 30 March 2021)];Employers Express Confidence in Colleges and Universities: See College as Worth the Investment, New Research Finds. 2018 Aug 29; Available online: https://hartresearch.com/employers-express-confidence-in-colleges-and-universities-see-college-as-worth-the-investment-new-research-finds/
- Heijltjes Anita, Gog Tamara van, Lippink Jimmie, Paas Fred. Unraveling the effects of critical thinking instructions, practice, and self-explanation on students’ reasoning performance. Instructional Science. 2015;43:487–506. doi: 10.1007/s11251-015-9347-8. [ DOI ] [ Google Scholar ]
- Holmes Natasha G., Wieman Carl E., Bonn DougA. Teaching critical thinking. Proceedings of the National Academy of Sciences. 2015;112:11199–204. doi: 10.1073/pnas.1505329112. [ DOI ] [ PMC free article ] [ PubMed ] [ Google Scholar ]
- Hunter John E., Schmidt Frank L. Intelligence and job performance: Economic and social implications. Psychology, Public Policy, and Law. 1996;2:447–72. doi: 10.1037/1076-8971.2.3-4.447. [ DOI ] [ Google Scholar ]
- Kozyreva Anastasia, Lewandowsky Stephan, Hertwig Ralph. Citizens versus the internet: Confronting digital challenges with cognitive tools. [(accessed on 30 March 2021)];Psychological Science in the Public Interest. 2020 21 doi: 10.1177/1529100620946707. Available online: https://www.psychologi calscience.org/publications/confronting-digital-challenges-with-cognitive-tools.html . [ DOI ] [ PMC free article ] [ PubMed ] [ Google Scholar ]
- Kretzschmar Andre, Neubert Jonas C., Wusternberg Sascha, Greiff Samuel. Construct validity of complex problem- solv ing: A comprehensive view on different facts of intelligence and school grades. Intelligence. 2016;54:55–69. doi: 10.1016/j.intell.2015.11.004. [ DOI ] [ Google Scholar ]
- Lang Jonas W.B., Kell Harrison J. General mental ability and specific abilities: Their relative importance for extrinsic career success. Journal of Applied Psychology. 2020;105:1047–61. doi: 10.1037/apl0000472. [ DOI ] [ PubMed ] [ Google Scholar ]
- Lovelace Berkeley., Jr. Medical Historians Compare the Coronavirus to the 1918 Flu Pandemic: Both Were Highly Political. [(accessed on 30 March 2021)];CNBC. 2020 Available online: https://www.cnbc.com/2020/09/28/comparing-1918-flu-vs-corona virus.html?fbclid=IwAR1RAVRUOIdN9qqvNnMPimf5Q4XfV-pn_qdC3DwcfnPu9kavwumDI2zq9Xs .
- Marr Rhuaridh. Iranian Cleric Claims COVID-19 Vaccine Can Make People Gay. [(accessed on 30 March 2021)];Metro Weekly. 2021 Available online: https://www.metroweekly.com/2021/02/iranian-cleric-claims-covid-19-vaccine-can-make-people-gay/
- McCabe Kira O., Lubinski David, Benbow Camilla P. Who shines most among the brightest?: A 25-year longitudinal study of elite STEM graduate students. Journal of Personality and Social Psychology. 2020;119:390–416. doi: 10.1037/pspp0000239. [ DOI ] [ PMC free article ] [ PubMed ] [ Google Scholar ]
- Mega Emiliano R. COVID Has Killed more than One Million People. How Many more will Die? [(accessed on 30 March 2021)];Nature. 2020 doi: 10.1038/d41586-020-02762-y. Available online: https://www.nature.com/articles/d41586-020-02762-y . [ DOI ] [ PubMed ]
- Nickerson Raymond S. Developing intelligence through instruction. In: Sternberg Robert J., editor. The Cambridge Handbook of Intelligence. 2nd ed. Cambridge University Press; Cambridge: 2020. pp. 205–37. [ Google Scholar ]
- OECD . The Survey of Adult Skills: Reader’s Companion. 3rd ed. OECD Publishing; Paris: 2019. OECD Skills Studies. [ DOI ] [ Google Scholar ]
- Smith Matthew. Why won’t Britons Wear Face Masks? [(accessed on 30 March 2021)];YouGov. 2020 Available online: https://yougov.co.uk/topics/health/articles-reports/2020/07/15/why-wont-britons-wear-face-masks .
- Stanovich Keith E. What Intelligence Tests Miss: The Psychology of Rational Thought. Yale University Press; New Haven: 2009. [ Google Scholar ]
- Stanovich Keith E., West Richard F. On the failure of cognitive ability to predict my-side bias and one-sided thinking biases. Thinking & Reasoning. 2008;14:129–67. doi: 10.1080/13546780701679764. [ DOI ] [ Google Scholar ]
- Stanovich Keith E., West Richard F. What intelligence tests miss. The Psychologist. 2014;27:80–83. doi: 10.5840/inquiryctnews201126216. [ DOI ] [ Google Scholar ]
- Sternberg Robert J. A theory of adaptive intelligence and its relation to general intelligence. Journal of Intelligence. 2019;7:23. doi: 10.3390/jintelligence7040023. [ DOI ] [ PMC free article ] [ PubMed ] [ Google Scholar ]
- Tang Julian W. COVID-19: Interpreting scientific evidence—Uncertainty, confusion, and delays. BMC Infectious Diseases. 2020;20:653. doi: 10.1186/s12879-020-05387-8. [ DOI ] [ PMC free article ] [ PubMed ] [ Google Scholar ]
- Wirthwein Linda, Rost Detlef H. Giftedness and subjective well-being: A study with adults. Learning and Individuals Differences. 2011;21:182–86. doi: 10.1016/j.lindif.2011.01.001. [ DOI ] [ Google Scholar ]
- View on publisher site
- PDF (245.1 KB)
- Collections
Similar articles
Cited by other articles, links to ncbi databases.
- Download .nbib .nbib
- Format: AMA APA MLA NLM
Add to Collections
Thank you for visiting nature.com. You are using a browser version with limited support for CSS. To obtain the best experience, we recommend you use a more up to date browser (or turn off compatibility mode in Internet Explorer). In the meantime, to ensure continued support, we are displaying the site without styles and JavaScript.
- View all journals
- Explore content
- About the journal
- Publish with us
- Sign up for alerts
- Open access
- Published: 15 October 2024
Neural, genetic, and cognitive signatures of creativity
- Cheng Liu 1 ,
- Kaixiang Zhuang 2 ,
- Daniel C. Zeitlen ORCID: orcid.org/0000-0003-3585-7699 3 ,
- Qunlin Chen 1 ,
- Xueyang Wang 1 ,
- Qiuyang Feng 1 ,
- Roger E. Beaty ORCID: orcid.org/0000-0001-6114-5973 3 &
- Jiang Qiu ORCID: orcid.org/0000-0003-0269-5910 1
Communications Biology volume 7 , Article number: 1324 ( 2024 ) Cite this article
323 Accesses
2 Altmetric
Metrics details
- Cognitive control
- Cognitive neuroscience
Creativity is typically operationalized as divergent thinking (DT) ability, a form of higher-order cognition which relies on memory, attention, and other component processes. Despite recent advances, creativity neuroscience lacks a unified framework to model its complexity across neural, genetic, and cognitive scales. Using task-based fMRI from two independent samples and MVPA, we identified a neural pattern that predicts DT, validated through cognitive decoding, genetic data, and large-scale resting-state fMRI. Our findings reveal that DT neural patterns span brain regions associated with diverse cognitive functions, with positive weights in the default mode and frontoparietal control networks and negative weights in the visual network. The high correlation with the primary gradient of functional connectivity suggests that DT involves extensive integration from concrete sensory information to abstract, higher-level cognition, distinguishing it from other advanced cognitive functions. Moreover, neurobiological analyses show that the DT pattern is positively correlated with dopamine-related neurotransmitters and genes influencing neurotransmitter release, advancing the neurobiological understanding of creativity.
Similar content being viewed by others
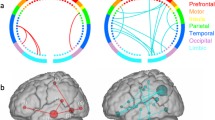
Predicting creative behavior using resting-state electroencephalography
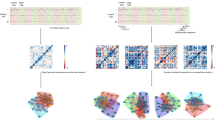
Static and dynamic functional connectivity supports the configuration of brain networks associated with creative cognition
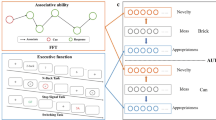
Semantic associative abilities and executive control functions predict novelty and appropriateness of idea generation
Introduction.
Creativity aids in the development of individual problem-solving skills and is critical to the cultural and economic advancement of society. Divergent thinking (DT), the ability to produce multiple ideas on an open-ended task, plays an essential role in creativity; indeed, creative ability is typically operationalized by performance on DT tasks 1 . DT may also help people to develop an understanding of differences and an appreciation of different perspectives 2 , have a positive impact on mood 3 , and can serve as an indicator of representative potential 4 , 5 , 6 , 7 .
There are both consistent and inconsistent conclusions in task-related functional magnetic resonance imaging (fMRI) studies of DT. The most active brain regions are centered on the bilateral dorsolateral prefrontal cortex (DLPFC), the ventrolateral prefrontal cortex (VLPFC) and right anterior cingulate cortex (ACC) 8 , 9 , 10 , 11 , 12 , 13 . However, significant activation of other brain regions has also been found in different studies, such as the right angular gyrus (AG) 10 , the left fusiform gyrus (FG) 8 , 10 and the left middle temporal gyrus (MTG) 9 , 13 , 14 . This variability may be attributed to the fact that creativity represents the highest level of advanced cognition, requiring the coordination of multiple cognitive processes and the involvement of corresponding brain regions during creative thinking. Benedek et al. 15 , 16 , 17 , 18 , Functional connectivity, in conjunction with the dual-process theory of creativity, explains DT at the network level. The dual-process theory of creativity posits that creative thinking involves both spontaneous and unconscious processes of idea generation and connection supported by the default model network (DMN), such as mind-wandering and free association 19 , 20 , as well as the conscious evaluation and selection of generated answers supported by the frontoparietal control network (FPCN) 15 , 21 . The close collaboration between the DMN and FPCN networks facilitates the emergence of creative thought and constitutes a core mechanism of this complex cognitive process 21 , 22 , 23 . However, in addition to discretely dividing brain regions based on function, morphology, and other attributes 24 , a contemporary direction in neuroimaging research suggests that the mature adult cerebral cortex is organized as a series of smoothly varying continuous gradients. Notably, the primary gradient represents an organizational framework that transitions from sensory processing to abstract cognition 25 . As a complex cognitive process involving multiple brain regions, the neural representation of creative thinking is well-suited to be explained using a continuous gradient approach, which we refer to in this study as the organizing principle of neural representation.
Cognitive decoding offers a powerful approach for elucidating the complexity of DT. By leveraging quantitative reverse inference, cognitive decoding maps activation patterns in neuroimaging data onto psychological concepts that characterize the higher-level mental processes engaged 26 . In addition, human cognition is strongly influenced by genetics and neurotransmitters 27 , 28 , 29 . Integrating this understanding with neuroimaging techniques can provide deeper insights into the neurobiological mechanisms that underlie complex behavioral traits 30 . However, systematic applications of these approaches to creativity research are still limited, as few studies have attempted to integrate neuroimaging and genetic approaches 31 .
In the present study, we used MVPA-based neural decoding techniques to construct the neural representation of DT in two samples (sample 1 [ n = 55] and sample 2 [ n = 30]) and attempt to decipher the principles of its neural organizations. MVPA allows for capturing information at a more refined spatial scale 32 and has been shown to yield larger effect sizes in brain-outcome associations compared to univariate analysis 33 . Next, we explained the neural representations of DT from a cognitive decoding as well as a biogenetic perspective, by examining spatial correlations between the DT neural pattern and previously collected, open-source brain data on cognition, gene expression profiles, and neurotransmitter receptors and transporters. Finally, to extend the analyses from the group-level, we predicted individual DT scores from the DT brain pattern in the two task-based samples, and also from functional connectivity between the regions of the DT pattern across three large resting-state fMRI samples (SLIM = 410, GBB = 304, BBP = 600) (Fig. 1b ). This systematic evaluation collectively contributes to our understanding of the neurobiological foundations of creativity.
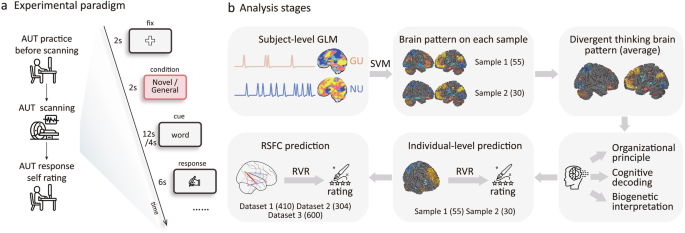
a Sample 1 and sample 2 underwent two similar paradigms during fMRI acquisition. The task-based fMRI contained two conditions, the control condition and the DT condition, requiring subjects to generate a common or original use, respectively, for a given object. This procedure consisted of 4 runs (6 runs in sample 2), each run included 20 trials, comprised of 12 novel use prompts and 8 general use prompts. After the fMRI scan, subjects were asked to recall and refine their responses, and then rate the originality of their responses. b The analytic stages and datasets used in the present study. Specifically, a whole-brain multivariate pattern predictive of novel use vs general use was trained on the sample 1 ( N = 55) and sample 2 ( N = 30) using support vector machine and further evaluated in the two samples (via cross-validation and mutual use as a validation sample for each other). After identifying the DT brain activity pattern, we parsed the cognitive processes related to the brain pattern using meta-analytic decoding on Neurosynth. Then, we explored the neural organizational principle of the DT brain pattern by examining which brain networks the DT regions belonged to, as well as the spatial correlation with functional connectivity gradient maps. Next, to interpret the brain pattern from a biogenetic perspective, we explored spatial correlations between the DT brain pattern and gene expression as well as neurotransmitters. Finally, to extend from group-level findings to individual-level predictions, we used the DT brain pattern to predict individual DT scores in the two task-based fMRI samples and generalized the identified effects to resting-state modalities (across three large resting-state fMRI samples [dataset 1, N = 410; dataset 2, N = 304; dataset 3, N = 600]). Figure 1 was created entirely by the authors for this publication, without the use of any pre-existing elements or content from external sources.
Decoding the neural basis of divergent thinking
To identify the multivariate patterns of fMRI activation for DT, we applied linear support vector machines (SVMs) to discriminate the novel use (NU) condition (i.e., DT) versus the general use (GU) control condition with a 10 × 10-fold cross-validation in the sample1 ( n = 55) and sample2 ( n = 30) respectively (see ‘Methods’ for details). In the classification of model1, the accuracy was 80% ± 3.8% (mean ± SE, p < 0.00001) and area under the receiver operating characteristic curve (AUC) was 0.90 with a sensitivity of 80.0% (95% CI: 70%–90%) and specificity of 80.0% (95% CI: 69%–89%) in the test participants (Fig. 2a, b ). In the classification of model2, the accuracy was 85% ± 4.6% (mean ± SE, p < 0.00001) and the AUC was 0.94 with a sensitivity of 96% (95% CI: 89%–100%) and specificity of 73% (95% CI: 56%–89%) in the test participants (Fig. 2d, e ).
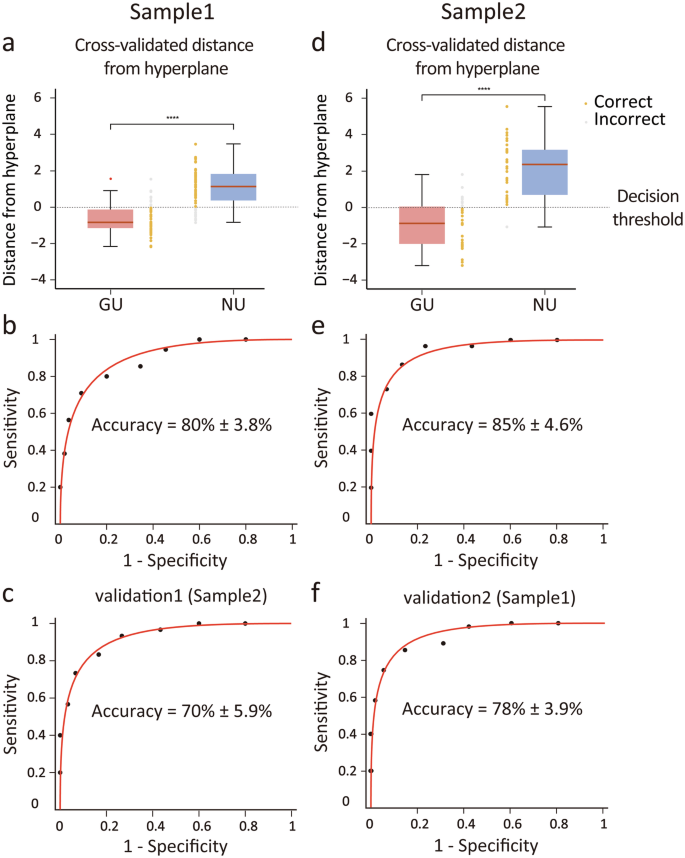
a The cross-validated distance from hyperplane and the decision threshold of model1, the yellow dots indicate correct classification, and the gray dots indicate misclassification. b The receiver operating characteristic (ROC) plot of model1. The accuracy of the model1 was 80% ± 3.8%. c The ROC plot of model1 when sample2 as validation dataset. The accuracy of the model1 was 70% ± 5.9%. d The cross-validated distance from hyperplane and the decision threshold of model2. e The ROC plot of model2. The accuracy of the model2 was 85% ± 4.6%. f The ROC plot of model2 when sample1 as validation dataset. The accuracy of the model2 was 70% ± 5.9%. Source data are provided as a Supplementary Data file.
To test whether a classification score was significant, we performed a permutation analysis: we first randomly reassigned subject labels and then performed tenfold cross-validation classification. Then, two samples were used to as validation dataset for each other to further test the generalization performance of two models, with no further model fitting (see ‘Methods’ for details). The results showed the accuracy of model1 when used sample2 as the validation dataset was 70% ± 5.9% (mean ± SE, p = 0.0027) (Fig. 2c ). The accuracy of model2 when used sample1 as the validation dataset was 78% ± 3.9% (mean ± SE, p < 0.00001) (Fig. 2f ). Altogether, the machine learning models achieved good accuracy across both samples, indicating reliable prediction of the DT (novel use) condition from multivariate patterns of fMRI activation.
To identify significant features that reliably contribute to the classification of NU and GU in model1 and model2, we conducted bootstrap tests with 10,000 iterations respectively (see ‘Methods’ for details). Then we obtain the thresholded maps of the two models. To statistically compare the two thresholded maps, we calculated Pearson correlation across voxel weights. The two pattern classifiers were high correlated with each other (Fig. 3a , r = 0.838, p < 0.001). Thus, we averaged the thresholded weight maps of the two models as the DT brain pattern (Fig. 3b , thresholded at q < 0.05 with a false discovery rate [FDR] correction). We found that NU versus GU was predicted by increased activity in bilateral DLPFC, bilateral dorsomedial prefrontal cortex (DMPFC), left VLPFC, bilateral ACC, bilateral orbitofrontal cortex (OFC), left AG, left MTG, bilateral thalamus, and right cerebellum (warm colors in Fig. 3b ), and was predicted by decreased activity in right Superior Parietal Lobule, right Precuneus, and right inferior Lateral Occipital Cortex (cool colors in Fig. 3b ).
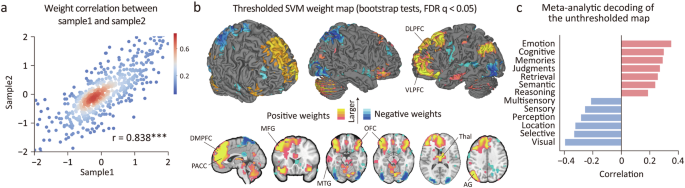
a Pearson correlation between thresholded weights of two models. b The weight map shows the final predictive features after average the thresholded maps (significant feature weights of SVM models by the bootstrap tests and thresholded at an FDR of q < 0.05) of two models. c Decoding analysis based on a meta-analytic database. The bar plot shows the functional terms obtained from the Neurosynth decoder applied to the thresholded map. The pink bars represent the decoding results for positive weights, and the blue bars represent the decoding results for negative weights. The significance level is FDR-corrected p < 0.05. Source data are provided as a Supplementary Data file.
To parse the cognitive processes involved in the DT brain pattern from a cognitive decoding perspective, we examined the spatial correlation between this pattern and meta-analytic fMRI activation maps (NeuroSynth datasets, see ‘Methods’). For positive weights, the decoding results focus on Emotion, Cognitive, Memories, Judgments, Retrieval, and Reasoning; the results for negative weights focus on Visual, Selective, Location, Perception and Sensory (Fig. 3c ) (see Supplementary Table 1 ). Thus, the DT brain pattern was positively associated with the neural bases of higher-order cognitive functions (e.g., memory and reasoning).
Mapping the neural signature of divergent thinking to functional gradients
In order to better integrate the results of the neural representations, we interpreted the distribution of brain regions for the DT brain pattern at the network level. Specifically, we examined which resting-state functional networks play important roles in the SVM model by calculating the proportions of overlap between the DT brain pattern (predictive weights) and each of the brain networks (see ‘Methods’ for details). The overlaps with the positive predictive weights of the model were focused on Default Model, Frontoparietal Control, and Limbic networks (Fig. 4a ). The overlaps with the negative predictive weights of the model were focused on dorsal Attention, Visual and Somatomotor networks. These overlap results with functional brain networks suggest that the positive brain activity pattern for DT (i.e., the regions more involved in creative thinking) is concentrated in the default and frontal-parietal control networks.
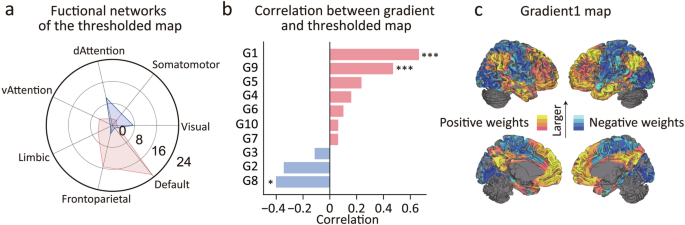
a Overlap with large-scale resting-state functional networks. The radar chart depicts the posterior probability of observing overlaps between the thresholded SVM model and the resting-state functional networks. The pink chart represents the overlaps with the positive predictive weights of the model, and the blue chart represents the overlaps with the negative predictive weights of the model. b Correlation between gradient and the thresholded map. c Principle gradient map of functional connectivity. *** indicates FDR-corrected p < 0.001, * indicates FDR-corrected p < 0.05. Source data are provided as a Supplementary Data file.
Next, we tested if these brain activity patterns for DT map to an underlying organizing principle. Given the importance of gradients in understanding organizing principles of the brain, we examined the spatial correlation between the DT brain pattern and the first 10 gradients in the brain (see ‘Methods’ for details). We found significant positive correlations between the DT brain pattern and the principal gradient map ( r = 0.66, p fdr = 0.0005), the 9th gradient map ( r = 0.47, p fdr = 0.0005), and a significant negative correlation with the 8th gradient map ( r = –0.40, p fdr = 0.0007) (Fig. 4b , thresholded at q < 0.05 with FDR correction; for the full list, see Supplementary table 2 ). Thus, by mapping the neural signature of DT onto macroscale gradients, we situate creativity within the brain’s primary system supporting complex psychological processes.
Linking the neural signature of divergent thinking to gene expression and neurotransmission
We next explored potential gene expression profiles (from Allen Human Brain Atlas datasets) that are associated with the DT brain pattern. First, we preprocessed the micro-array data according to a recommended pipeline 34 and obtained group-level gene expression maps that were normalized across samples and donors (15,633 genes). Second, we matched the gene expression maps to the DT brain pattern covering 574 regions (Fig. 5a ). Then, we conducted a Pearson’s correlation analysis to estimate the relationship between the DT brain pattern and each gene expression map (Fig. 5b ). The significance of these correlations was obtained by permutation tests (10,000 times) in which spatial autocorrelations were preserved in the surrogate maps 35 . Finally, we performed Gene Ontology enrichment analysis for assessing the overrepresentation of gene sets in biological experiments to derive meaningful insights. The significance of the GO enrichment terms was further tested using a null model, which was generated by performing an enrichment analysis using surrogate maps which preserved the empirically observed spatial autocorrelations 35 .
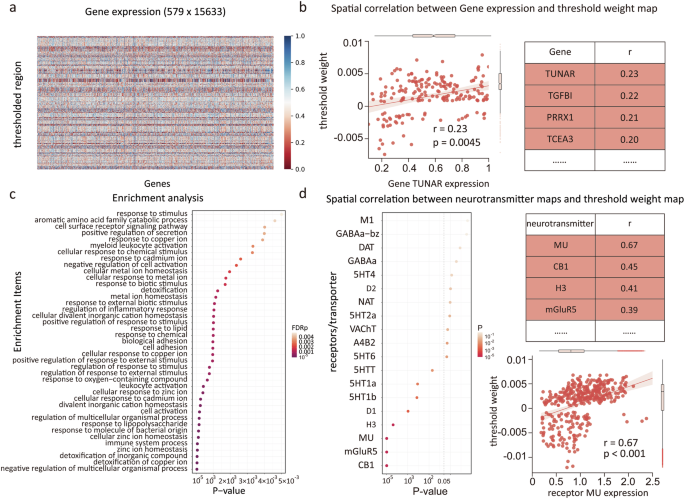
a Normalized gene expression levels in 579 regions. b The correlation between the expression profile of each gene and the thresholded map. One representative scatter plot was illustrated with positive correlations. c Enrichment analysis. d The correlation between each neurotransmitter map and thresholded map. One representative scatter plot was illustrated with positive correlations. Source data are provided as a Supplementary Data file.
We found that genes within the gene set that showed significant enrichment were those involved in the negative regulation of multicellular organismal process, detoxification of copper ion and detoxification of inorganic compound (FDR-corrected, all q < 0.05, Fig. 5c ; for the full list, see Supplementary Table 3 ). We also performed Gene Ontology enrichment analysis on a gene set made up of the genes ranked in reverse order (i.e., starting with the most significant negative correlation and ending with the most significant positive correlation), but did not find significant enrichment of any genes in this set. Taken together, these results indicate that the DT brain pattern is associated with the expression levels of genes that affect the overall functioning of the organism by influencing the synthesis and release of neurotransmitters and the transmission of information.
Furthermore, we assessed potential neurotransmitter receptors and transporters that are associated with the DT brain pattern. In this analysis, we calculated the spatial Pearson’s correlation between the DT brain pattern and each receptors/transporters map (Fig. 5d ), which were collected in previous studies via PET (see “Methods” for details). The significance of these correlations was obtained by permutation tests (10,000 times) in which spatial autocorrelations were preserved in the surrogate maps. We found that the neurotransmitter receptors/transporters that showed the most significant positive correlations were MOR ( r = 0.67, p spin < 0.001), CB 1 (omar) ( r = 0.45, p spin < 0.001), H 3 ( r = 0.41, p spin < 0.001), mGluR 5 ( r = 0.39, p spin < 0.001) (for the full list, see Supplementary Table 4 ). Thus, the DT brain pattern is related to neurotransmitters capable of promoting dopamine release.
Using the neural signature of DT to predict individual creativity during task performance
To validate the effectiveness of the brain pattern as biomarker for predicting DT we applied relevance vector regression (RVR) with single-trial beta maps (only NU condition for each subject) as feature to predict originality ratings for DT responses with a 10 × 10-fold cross-validation in the sample1 ( n = 55) and sample2 ( n = 30) (see ‘Methods’ for details). The prediction results of sample 1 are shown in Fig. 6a ; the distribution of the correlation(r) between the predicted value and the true value in 10 × 10-fold cross-validations ranged from 0.21 to 0.39 (average r = 0.35, p pt < 0.001, mean absolute error = 0.82). Figure 6b shows the prediction results of sample 2; the distribution of the correlation( r ) between the predicted value and the true value in 10 × 10-fold cross-validations ranged from 0.09 to 0.17 (average r = 0.14, p pt < 0.001, mean absolute error = 1.82). Thus, the DT brain pattern significantly and positively predicted individual DT ability across both task-based fMRI samples.
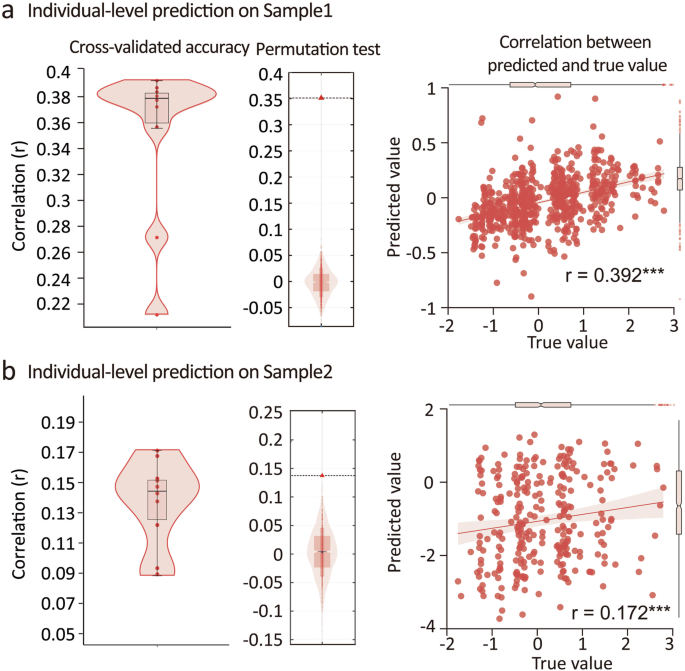
a The distribution of the correlation( r ) between the predicted value and the true value in 10 × 10-fold cross-validations on sample 1. One representative scatter plot was illustrated. b The distribution of the correlation(r) between the predicted value and the true value in 10 × 10-fold cross-validations on sample 2. One representative scatter plot was illustrated. Source data are provided as a Supplementary Data file.
Using the neural signature of DT to predict individual creativity from resting-state fMRI
To extend the value of the brain pattern and further generalize its predictive power at the individual level, we use data from resting-state modalities to further extend this work. Resting-state functional connectivity is considered a consistent message that measures the organization of intrinsic function within an individual and varies over time and across individuals. Therefore, we used three large-scale resting-state fMRI samples (SLIM, GBB, and BBP) to predict individuals’ DT (i.e., AUT) scores. First, we divided the DT brain pattern in 6 clusters including left AG, right IFG, left MTG, left Precuneus, right Thalamus, right cerebellum as 6 ROIs. Second, we constructed 6 × 6 symmetric Functional connectivity (FC) matrix with 15 unique edges per participant (see ‘Methods’ for details). Then, we applied RVR with 15 unique edges as feature to predict creative ratings for each subject on Southwest University Longitudinal Imaging Multimodal (SLIM) with a 10 × 10-fold cross-validation. The prediction results were shown in Fig. 7b . The distribution of the correlation( r ) between the predicted value and the true value in 10 × 10-fold cross-validations ranged from 0.05 to 0.19 (average r = 0.15, p pt = 0.002, mean absolute error = 0.76). To identify significant features that reliably contribute to the DT, we conducted bootstrap tests with 10,000 iterations. The functional connectivity between the left AG and left MTG, left AG and left Precuneus, left MTG and right cerebellum significantly predicted individual DT scores (Fig. 7a ).
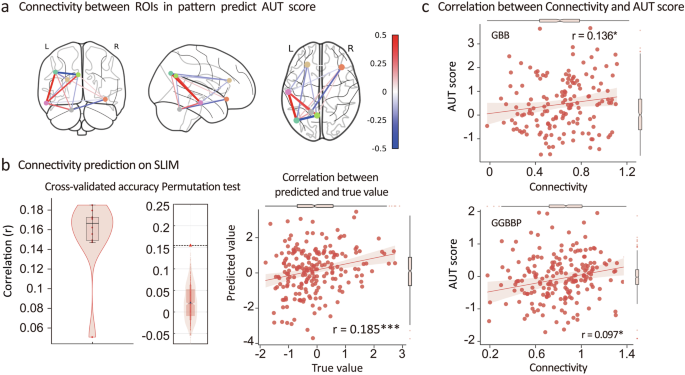
a The connectivity map shows the feature weight for predicting DT (i.e., AUT score). b The distribution of the correlation(r) between the predicted value and the true value in 10 × 10-fold cross-validations on SLIM. One representative scatter plot was illustrated. c Correlation between resting-state functional connectivity and DT ability in GBB and BBP. Source data are provided as a Supplementary Data file.
Then, we conducted a validation analysis on Gene-Brain-Behavior (GBB) and BBP samples. Specifically, we calculated the Pearson correlation between functional connectivity (between left AG and left MTG, left AG and left Precuneus, left MTG and right cerebellum) and individual DT scores in GBB and BBP. In the GBB sample, functional connectivity between left AG and left MTG was positively correlated with DT ( r = 0.14, p = 0.018) (Fig. 7c top), whereas functional connectivity between left AG and left Precuneus ( r = 0.08, p = 0.16), as well as left MTG and right cerebellum ( r = 0.02, p = 0.74) were both not significantly correlated with DT. In the BBP sample, we found that functional connectivity between left AG and left MTG was positively correlated with DT ( r = 0.10, p = 0.017) (Fig. 7c bottom), whereas functional connectivity between left AG and left Precuneus ( r = 0.04, p = 0.38), as well as left MTG and right cerebellum ( r = –0.01, p = 0.73) were both not significantly correlated with DT. Taken together, resting-state functional connectivity of some, but not all, regions in the task-based DT brain activity pattern was correlated with individual DT ability.
The current study aimed to develop and validate a comprehensive brain profile that captures the essence of DT. This profile demonstrates the potential to predict individual scores of DT, offering practical applications in understanding individual creative thinking. Through the utilization of MVPA, we examined the neural representation of DT across the entire brain, comparing it to control conditions. Notably, these brain features were found to be predominantly distributed within the default, frontoparietal, and limbic networks. Further, through spatial correlation analysis with resting-state functional connectivity gradients, we uncovered the organizing principles of the DT brain pattern. We also explained this brain pattern from cognitive and biogenetic perspectives, respectively. The DT brain pattern was associated with higher-order cognitive functions (such as memory, judgment, and reasoning), increased expression levels of genes that influence neurotransmitter release, as well as increased release of neurotransmitters related to mood and reward such as dopamine, MOR, CB 1 . Additionally, the features of the DT brain activity pattern were able to predict DT scores at the individual level and demonstrate generalizability across different modalities—task-based activity pattern and resting-state functional connectivity. Collectively, these findings shed light on the neural expression of creative thinking in the human brain, offering insights into its cognitive functioning and biological underpinnings.
We observed that the DT brain pattern was distributed across a broad range of regions, primarily including bilateral DLPFC, bilateral DMPFC, left VLPFC, bilateral ACC, bilateral OFC, left AG, and bilateral thalamus regions. These brain regions are distributed across a wide range of brain networks involved in different cognitive functions, and we first parsed such representational patterns from a cognitive decoding perspective. Our results suggest that DT brain patterns share partial commonalities with many representations of cognitive functions. For instance, free association refers to the process by which an individual spontaneously links one thought, memory, or concept to another related or unrelated thought, memory, or concept without external constraints or control 36 , primarily involving the activation of brain regions within DMN 37 . Working memory, as a form of higher-order cognition, is a cognitive system responsible for the sustained attention, monitoring, temporary storage, and manipulation of information 38 , and it primarily involves widespread and consistent activation of regions such as the DLPFC, VLPFC, and parietal cortex 39 . Inhibitory control refers to the ability of an individual to suppress or regulate responses when faced with inappropriate or irrelevant distractions, which is a key component of executive functions 40 . This function is mainly associated with the DLPFC, inferior frontal cortex or OFC 40 , 41 , 42 , as well as the ACC and posterior parietal cortex 42 . These cognitive abilities partially overlap with the brain patterns associated with DT. The spontaneous cognitive process (free association) is related to enhanced internal connectivity within the DMN, which facilitates associative fluency in creative processes, thereby improving performance on creativity tasks 43 . Working memory contributes to DT through attentional control mechanisms that manage and guide the complex search processes 44 . The inhibitory control system of the prefrontal cortex suppresses spontaneously generated solutions that are rapid/unconscious, ultimately preserving only original ideas 45 , 46 , 47 .
When overlapping with large resting-state brain networks, we found that these regions mainly reside within the DMN, the FPCN, and a small portion of the limbic network. The DMN consisting of the MPFC, precuneus, and AG is typically associated with spontaneous thinking, daydreaming, or associative processes 48 , 49 , 50 . During the generation of ideas in DT, the DMN facilitates the production of ideas by supporting a series of associative processes in semantic memory through spontaneous and unconscious recall 51 , 52 , 53 , 54 . The FPCN, involving the DLPFC, VLPFC, and dorsomedial thalamus, is typically associated with executive control processes 55 , 56 , 57 . During DT, the FPCN is responsible for evaluating and selecting the generated ideas, filtering out those that are insufficiently novel, and ultimately producing innovative thought 18 , 58 , 59 , 60 . Additionally, our results indicate that the primary sensory and motor networks play an inhibitory role in the brain’s production of DT. Therefore, both the DMN and FPCN are crucial for DT, and their activity is accompanied by a reallocation of resources within the visual network, reducing reliance on external visual information while enhancing internal thinking and imagery 61 , 62 .
However, beyond observing the representation of DT from the perspective of discrete networks, the emergence of resting-state functional connectivity gradients provides a new perspective for understanding it from a continuous standpoint. The principal gradient is generally considered to reflect the global hierarchical structure of brain function, with the DMN that represents high-level/abstract cognitive processes, positioned at one end, and the primary sensory and motor networks at the other 25 . Our findings reveal a strong positive correlation between the distribution of brain features associated with DT and the principal gradient, which first highlights the critical role of the DMN in DT. Moreover, this suggests that the neural representation of DT is not distributed in a discrete and unstructured manner across various brain regions but rather organized in a continuous gradient that hierarchically structures brain regions with different functions, forming a unique neural organizational principle for DT. DT likely depends on the dynamic integration of functional regions 20 , 63 , from basic sensory input to high-level cognition. Additionally, DT requires flexible integration and cooperation among these different networks, aligning with research that emphasizes the need for high cognitive flexibility 64 , 65 , 66 , which involves rapidly shifting from one idea to another. In summary, we propose that creative thinking involves a broad integration from concrete sensory information to abstract, high-level cognition. The core of creative thinking lies in the distinctive coordination and organizational framework that spans across brain networks, distinguishing it from other high-level cognitive functions.
Next, we explained the DT brain pattern from a biogenetic perspective. We established links between the DT brain pattern and enriched expression of genes involved in the release of the neurotransmitters such as dopamine, MOR, CB 1 , H 3 , and mGluR 5 , as well as the negative regulation of multicellular organismal processes, and detoxification of copper ions and inorganic compounds. Negative regulation of multicellular organismal processes ( https://www.yeastgenome.org/go/GO:0051241 ) refers to the process of inhibiting/preventing or reducing the frequency/rate or extent of various activities in multicellular organisms. Excessive accumulation of copper ions with other inorganic compounds can lead to neurological damage, affecting neurotransmitter synthesis/release and messaging processes 67 , 68 , 69 . We, therefore, suggest that negative regulation of multicellular organismal processes and the detoxification of copper ions and other inorganic compounds may affect the overall functioning of the organism, potentially influencing its ability to engage in DT activities or problem-solving. Neurotransmitter release has been linked to creative cognition, and creative drives such as motivation, emotional states, and rewards can influence creative thinking through related neuromodulatory systems 70 . It has been suggested that positive-activating moods with an approach motivation (e.g., happiness) promote creativity 71 , 72 . In addition to this, many researchers have emphasized the close link between rewards and creativity, as the promise of rewards may facilitate creative thinking and original solutions 73 , 74 .
The dopamine system is involved in various aspects of cognitive function related to reward, addiction, attention, and compulsion, and studies have shown that interactions between the frontal and striatal dopamine pathways can contribute to creative cognition by facilitating flexible processing and sustained-driven creativity 70 , 75 . Kranz, Kasper, and Lanzenberger 76 made the argument that serotonin serves as an important mediator of the affective, motivational, and cognitive elements of reward representations, and thus the neurotransmitter serotonin has similar value to dopamine in reward processing 70 . Similarly, opioids such as MOR are distributed in the reward centers of the brain and are associated with the regulation of pain, reward, and addictive behaviors 77 , 78 , 79 . Moreover, other studies have mentioned that highly potent cannabis which affects CB 1 receptors may impair DT 80 ; H 3 receptors are involved in various cognitive and motor processes 81 , play a role in the central nervous system and have been explored as a potential target for cognitive symptoms and impairments 82 ; mGluR 5 plays an important role in memory and learning processes in the brain 83 . Thus, our findings are consistent with previous studies and provide evidence supporting the link between dopamine release and DT brain patterns from a novel perspective. In addition to this, evidence was also obtained that other neurotransmitters and related gene expression profiles are associated with DT, which may provide a neurobiological explanation of the DT brain pattern.
Finally, the DT brain pattern also predicted individual-level creativity scores well. Meanwhile, the predictive power of brain patterns was further generalized across three large resting-state samples, in addition to the two task-based samples. This suggests that brain pattern not only represents a common basis for neural representations of DT but also captures individual differences in generating creative ideas and problem-solving. Understanding individual differences in DT can help identify individuals who excel in this area, and these assessments, used in the fields of psychology and education, can also be used to develop effective interventions for students to improve their learning experiences and outcomes.
A limitation of the present study was the use of the AUT, although this task is typically used to measure DT (thus better aligning our work with prior DT studies). The traditional AUT suffers from poor reliability, influenced by various factors. This study mitigated individual differences associated with the task itself by making adaptations. With respect to solution duration, participants were instructed to generate a response based on the items provided, without the need to generate as many responses as possible, thus addressing potential changes resulting from a longer solution duration. Additionally, compared to previous AUT administrations, the present study expanded the pool of stimulus words by selecting 80 specific object words (e.g., “umbrella”) from an entity noun library as experimental stimuli (K 84 ). To ensure the suitability of each object word for idea generation, five trained raters evaluated their appropriateness 85 , thus minimizing individual differences arising from the choice of stimulus words.
Although the present study integrated neural, cognitive, and genetic approaches to comprehensively study verbal DT, future studies should apply such integrated fMRI-based approaches to investigate other forms of DT (e.g., visual), as well as convergent thinking which is also important for creativity. Similarly, future studies should utilize such integrated methods to assess creativity across domains (e.g., music, art, science). Indeed, approaches which integrate neuroimaging and biogenetic methods are needed for holistic creativity neuroscience, yet such approaches remain rare in the literature. Additionally, the current study’s sample was predominantly female, we encourage future research to include a more balanced sample to enhance generalizability.
In conclusion, the present study has identified a comprehensive neural representation of DT and its organizational principle, which has been validated and generalized across independent samples and various data modalities. Additionally, the DT brain pattern was explained from both cognitive decoding and neurobiological perspectives, as this pattern was positively linked to cognitive abilities (e.g., memory and reasoning), and neurotransmitter release (e.g., dopamine). Taken together, these findings offer substantial evidence for the neural representation and deciphering of DT, enhancing our comprehension of its cognitive significance and underlying biological foundations.
Task-based fMRI samples
Participant.
The task-based fMRI data employed in the current study comes from two independent samples. A total of 57 subjects were recruited in Sample 1 (43 females, mean age = 19.23 years, SD = 0.96, range = 17–22 years). Two subjects were excluded from subsequent analyses due to image abnormalities when normalized to standard space. Thus, 55 subjects within sample 1 were include in the following analysis. Sample 2 has a total 31 participants (20 females, mean age = 19.30 years, SD = 2.49, range = 17–28 years). One subject was excluded from subsequent analyses due to falling asleep during scanning. Thus, 30 subjects were included in the following analyses. All subjects were college students at Southwest University, all were healthy, met MRI safety criteria (e.g., they had no stents or metal implants), and had no history of neurological or psychiatric problems. In accordance with the Declaration of Helsinki, all subjects provided written informed consent prior to participation in the study and were paid for their participation.
Stimuli and paradigm
Subjects performed an adjusted version of the AUT during the fMRI scan (Fig. 1a ). The task contains two conditions, the control condition is the general use (GU) condition, which requires subjects to answer what is commonly used for the provided object (an entity), e.g., a map is commonly used for navigation (map->navigation). The DT condition is the novel use (NU) condition, which requires subjects to generate original and unique uses for a given object, e.g., a map can be used to make a necklace (map -> necklace). The procedure consists of 4 sessions, each session includes 20 trials, with 12 NU conditions and 8 GU conditions. Each trial starts with a fixation screen for 2 s. Then the cue for the experimental condition - “novel use” (or “general use”) appears on the screen for 4 s, followed by the object (e.g., ring -> ?) and remains on the screen for 12 s, (the “general use” condition has the object on the screen for 4 s), which is called the idea generation period. In this phase, subjects must think about possible responses to a given stimulus (original usage of an everyday object or general use of a given noun). If response comes to mind, the subject is asked to quickly press “1” with the left hand and then write down the response on the paper as quickly as possible (within 6 s), summarizing the response in one phrase (2–3 words). If no response comes to mind, the subject cannot press a key and draws an “X” on the paper when the writing prompt appears. Finally, the “rest” appeared on the screen for 8 s and the subject was asked not to write, to look at the screen and to remain calm. The experimental conditions are presented in random order and total time for the task is about 40 min. After the MRI scan, subjects were asked to recall and refine their responses, and then rate the originality of their responses using a 5-point scale that ranged from 1 (‘not original’) to 5 (‘highly original’). Collecting individuals’ ratings of the originality of their answers based on personal meaning can reflect their own creative processes and internal reward mechanisms, revealing their self-perception of creative performance (K 86 , 87 ).
Sample 2 was obtained from 85 , in which two conditions NU and GU, consistent with sample 1, were selected for subsequent analysis in this study. The procedure consists of 6 sessions, total 40 NU conditions and 20 GU conditions. As with sample 1, after the MRI scan, subjects were asked to recall and refine their responses, and then rate the originality of their responses using a 5-point scale that ranged from 1 (‘not original’) to 5 (‘highly original’).
MRI data acquisition and preprocessing
Mri data acquisition.
Whole-brain imaging was acquired on a Siemens 3T Trio scanner (Siemens Medical Systems, Erlangen, Germany) at the Brain Imaging Center, Southwest University. Task fMRI images were acquired using Gradient Echo Type Echo Planar Imaging (GRE-EPI) sequence (TR/TE = 2000 ms/30 ms, FA = 90°, resolution matrix = 64 × 64, FOV = 220 × 220 mm 2 , thickness = 3 mm, slices = 32, interslice gap = 1 mm, acquisition voxel size = 3.4 × 3.4 × 4 mm 3 ). High-resolution three-dimensional T1-weighted structure images were obtained using a Magnetization Prepared Rapid Acquisition Gradient-echo (MPRAGE) sequence (TR/TE = 1900 ms/2.52 ms, FA = 9°, FOV = 256 × 256 mm 2 , slices = 176, thickness = 1.0 mm, voxel size = 1 × 1 × 1 mm 3 ).
MRI data preprocessing
For each of the 4 (6 in sample 2) BOLD (Blood Oxygen Level-Dependent) runs found per subject (across all tasks and sessions), the following preprocessing was performed. First, a reference volume and its skull-stripped version were generated using a custom methodology of fMRIPrep. Head-motion parameters with respect to the BOLD reference (transformation matrices, and six corresponding rotation and translation parameters) are estimated before any spatiotemporal filtering using mcflirt (FSL 6.0.5.1:57b01774, Jenkinson et al., 2002). BOLD runs were slice-time corrected to 0.962 s (0.5 of slice acquisition range 0–1.93 s) using 3dTshift from AFNI (Cox and Hyde 1997, RRID:SCR_005927). The BOLD time-series (including slice-timing correction when applied) were resampled onto their original, native space by applying the transforms to correct for head-motion. These resampled BOLD time-series will be referred to as preprocessed BOLD in original space, or just preprocessed BOLD. The BOLD reference was then co-registered to the T1w reference using mri_coreg (FreeSurfer) followed by flirt (FSL 6.0.5.1:57b01774, Jenkinson and Smith 2001) with the boundary-based registration (Greve and Fischl 2009) cost-function. Co-registration was configured with six degrees of freedom. Several confounding time-series were calculated based on the preprocessed BOLD: framewise displacement (FD), DVARS, and three region-wise global signals. FD was computed using two formulations following Power (absolute sum of relative motions, Power et al. (2014)) and Jenkinson (relative root mean square displacement between affines, Jenkinson et al. (2002)). FD and DVARS are calculated for each functional run, both using their implementations in Nipype (following the definitions by Power et al., 2014). The three global signals are extracted within the CSF, the WM, and the whole-brain masks. Additionally, a set of physiological regressors were extracted to allow for component-based noise correction (CompCor, Behzadi et al. 2007). Principal components are estimated after high-pass filtering the preprocessed BOLD time-series (using a discrete cosine filter with 128 s cut-off) for the two CompCor variants: temporal (tCompCor) and anatomical (aCompCor). tCompCor components are then calculated from the top 2% variable voxels within the brain mask. For aCompCor, three probabilistic masks (CSF, WM and combined CSF + WM) are generated in anatomical space. The implementation differs from that of Behzadi et al. in that instead of eroding the masks by 2 pixels on BOLD space, the aCompCor masks are subtracted a mask of pixels that likely contain a volume fraction of GM. This mask is obtained by thresholding the corresponding partial volume map at 0.05, and it ensures components are not extracted from voxels containing a minimal fraction of GM. Finally, these masks are resampled into BOLD space and binarized by thresholding at 0.99 (as in the original implementation). Components are also calculated separately within the WM and CSF masks. For each CompCor decomposition, the k components with the largest singular values are retained, such that the retained components’ time series are sufficient to explain 50 percent of variance across the nuisance mask (CSF, WM, combined, or temporal). The remaining components are dropped from consideration. The head-motion estimates calculated in the correction step were also placed within the corresponding confounds file. The confound time series derived from head motion estimates and global signals were expanded with the inclusion of temporal derivatives and quadratic terms for each (Satterthwaite et al., 2013). Frames that exceeded a threshold of 0.5 mm FD or 1.5 standardised DVARS were annotated as motion outliers. The BOLD time-series were resampled into standard space, generating a preprocessed BOLD run in MNI152NLin2009cAsym space. First, a reference volume and its skull-stripped version were generated using a custom methodology of fMRIPrep. All resamplings can be performed with a single interpolation step by composing all the pertinent transformations (i.e., head-motion transform matrices, susceptibility distortion correction when available, and co-registrations to anatomical and output spaces). Gridded (volumetric) resamplings were performed using antsApplyTransforms (ANTs), configured with Lanczos interpolation to minimize the smoothing effects of other kernels (Lanczos 1964). Non-gridded (surface) resamplings were performed using mri_vol2surf (FreeSurfer).
First-level fMRI analysis
We conducted two separate GLM (general linear model) analysis in SPM12: subject-level analysis and single-trial analysis in two samples respectively. Subject-level GLM was used to obtain beta images for the Multivariate voxel pattern analysis. The four runs of the stimulation task were concatenated for each subject. The onset of the two conditions (NU and GU) was modeled using a canonical hemodynamic response function. Single-trial analysis was used to obtain beta images for the RVR analysis. To estimate single-trial responses, we conducted a GLM design matrix with separate regressors for each trial with respective onset time. Six head motion parameters ( x , y , z , roll, pitch and yaw) are included in the matrix as confounding variables and a high pass filter of 128 s was applied to remove low-frequency drifts.
Multivariate voxel pattern analysis
We applied whole-brain (restricted to a gray matter mask) multivariate machine-learning pattern analysis to obtain a brain activation pattern that best-classified subjects in NU and GU. We used the SVM algorithm using a linear kernel ( C = 1) implemented in the Interpreting machine learning models in neuroimaging toolbox ( https://github.com/cocoanlab/interpret_ml_neuroimaging ) with individual beta maps (one condition for each subject) as feature to classify subjects in NU and GU while undergoing fMRI. We performed the classification on sample 1 and sample 2, respectively. Neuroimaging studies are usually based on small number of subjects. Therefore, we used cross-validation (CV) strategies to overcome the loss of generalization due to the small training and testing sample size in the neuroimaging applications. To evaluate the performance, 10 × 10-fold CV procedure on the sample1 and sample2 was conducted respectively. All subjects were randomly assigned to 10 subsamples of 5 or 6 subjects (3 subjects in sample 2). The optimal hyperplane was computed based on the multivariate pattern about 50 participants (27 subjects in sample 2) (training set) and evaluated by the excluded 5 or 6 participants (3 subjects in sample 2) (test set). This procedure was repeated ten times, once for each subsample of the test set. To avoid potential bias in the training-test split, the CV procedure was repeated ten times throughout the study, with each repetition producing a different split, resulting in the prediction performance being averaged to produce a convergent estimate. We evaluated the performance of the SVM models from the following indices: accuracy, sensitivity, specificity, ROC, AUC and the definitions is as follows. Accuracy was defined as the ratio of the correct predictions to the total number of classifications: accuracy = (TP + TN)/(TP + FP + TN + FN). The ROC was also calculated by the one-versus-rest approach, with the parameter sensitivity and specificity denoted by: sensitivity = TP/(TP + FN) and specificity = TN/(TN + FP). Here, TP is the true positive, FP is the false positive, TN is the true negative and FN is the false negative for each label. We also performed a permutation test to estimate the empirical distribution of the classifier accuracy under the null hypothesis. To this end, we tested the SVM classifier with the random labeling and calculated the accuracy of SVM classifier in sample 1 and sample 2 respectively. The permutation test was repeated 5000 times. Classifier performance greater than 95% of the random permutations indicates above-chance accuracy (given an alpha of p < 0.05). To test the generalization performance of the two classification models, we performed validation using sample 2 (sample 1) as the validation set. We calculated the dot-product of vectorized activation images in sample 2 (sample 1) with the threshold weights of the model in sample 1 (sample 2). Then the average accuracy, sensitivity, specificity, positive predictively, and the AUC across this repetition were calculated. Bootstrap tests were conducted to provide P -values for voxel weights to threshold the classifier weights for display and interpretation. We constructed 10,000 bootstrap sample sets (with replacement) and ran SVMs on each bootstrap sample. Each bootstrap sample serves as training data for a new model. The hyper-parameters of the model were the same as the original model. Then, two-tailed, 5000 permutation tests were conducted to calculation P -values for each voxel based on the proportion of weights above or below zero. Then we performed FDR multiple comparison correction for the results of permutation test.

DT brain pattern obtained by classifier weights of two samples
Since the model weights obtained on the two samples had a high positive correlation, we combined the weights map of the two samples to obtain the final brain activation pattern that could be classified as specific to novel idea generation. First, we overlap the weights of the classification model trained by two samples separately to gain the common region. Then, we averaged the weight values of the voxels in the common region to obtain the DT brain pattern.
Evaluate the neurobiological validity of the model
To evaluate the neurobiological plausibility and validity of SVM model, we conducted biology-level assessments. The first assessment is evaluating overlaps of the MVPA-based DT brain pattern with large-scale resting-state functional networks. We used the mask that had unique values for seven resting-state functional networks 88 . Then, we calculated the posterior probability of observing the DT brain pattern given each network. Specifically, we calculated the overlap-based similarity separately for positive and negative predictive weights. The second assessment is using a large-scale meta-analytic decoding framework provided by Neurosynth, we can identify the psychological terms associated with DT brain pattern of predictive weights values. We used meta-analytic maps previously generated for various psychological terms and assessed their similarity to the DT brain pattern, implemented in the Neurosynth Python package ( https://github.com/neurosynth/neurosynth/blob/master/neurosynth/analysis/decode.py ). Then, it returns a list of the terms with correlation coefficients between the DT brain pattern and the meta-analytic maps. Then, to explore the neural organizational principle of the DT brain pattern, we calculated the Pearson’s correlation coefficient between the weights in DT brain pattern (i.e., the β-map) between previously-identified gradients. The group-level (averaged across participants) first ten gradients maps was accessed via the neuromaps ( https://netneurolab.github.io/neuromaps/ ) 89 . Firstly, we assigned the DT brain pattern to the gradient map. Then, the Pearson’s correlation between the β values and the gradient values across the overlapping regions was calculated. The statistical significance of association was assessed using “spin tests” (as detailed below in the “null model” section).
Spatial correlation between gene expression and DT brain pattern
Microarray expression data were obtained from six post-mortem brains provided by the Allen Human Brain Atlas (AHBA; http://human.brain-map.org/ 90 ). Preprocessing was performed using the abagen toolbox ( https://github.com/netneurolab/abagen 91 ). Each human brain tissue sample was preprocessed according to a recommended pipeline with default settings. We assigned all tissue samples from Allen Human Brain Atlas to DT brain pattern regions, resulting in expression level maps for 15,633 gene covering 574 regions within DT brain pattern. Samples were assigned to brain regions in the provided DT brain pattern region if their MNI coordinates were within 2 mm of a given parcel (the DT brain pattern native space is 2 mm). Then, we normalized gene expression levels across probes within the sample and across samples within a subject. This preprocessing produced a group-level (averaged across donors) gene expression profile of the 574 regions within DT brain pattern. To explore whether DT brain pattern is associated with gene transcriptional profiles, we calculated the Pearson’s correlation coefficient between the weights in DT brain pattern (i.e., the β-map) and the preprocessed expression profile of each gene in the microarray. Firstly, the DT brain pattern β-map was aligned to the 574 regions as described above. Then, the Pearson’s correlation between the β-map values and the gene expression values across the 574 overlapping regions was calculated. The statistical significance of association was assessed using “spin tests” (as detailed in the next section). We then arranged all genes in descending order according to the significance level of the correlation coefficients, starting with the genes with the most significant positive correlation to those with the most significant negative correlation. Subsequently, we uploaded the sequenced gene sets to the Gene Ontology enrichment analysis and visualization tool (GOrilla, http://cbl-gorilla.cs.technion.ac.il/ ) 92 : (i) a threshold of P < 10 –5 in the advanced parameter settings; and (ii) q -value < 0.05 in Benjamini–Hochberg FDR corrections.
Spatial correlation between neurotransmitter expression and DT brain pattern
We used neuromaps ( https://netneurolab.github.io/neuromaps/ 89 ); to access volumetric PET images previously collected for 19 different neurotransmitter receptors and transporters across 9 different neurotransmitter systems (Bedard et al. 93 , 94 , 95 , Ding et al. 96 , 97 , 98 , 99 , 100 , 101 , 102 , 103 , 104 , 105 , 106 , 107 , 108 , 109 ). To protect patient privacy, individual subject maps were averaged across studies prior to sharing. Each study, associated receptor/transporter, tracer, number of healthy subjects, age and references, and complete methodological details can be found in ref. 110 . The Pearson’s correlation between the DT brain pattern β-map and the receptors/transporters map was calculated. Then, the statistical significance of the association is assessed using a “spin test” (as detailed in the next section).
Spatial autocorrelation-preserving permutation tests were used to assess the statistical significance of associations across brain regions, called “spin tests”. Specifically, we choose for each parcel the vertex closest to its center of mass on the spherical projection of the fsaverage surface. We then rotate the coordinates of these centers of mass and reassign the values of the closest rotated parcels to each parcel. This procedure is repeated 10,000 times. These processes were implemented in neuromaps ( https://netneurolab.github.io/neuromaps/ 89 ).
Relevance vector regression analysis within DT brain pattern
We applied RVR to examine the effectiveness of the DT brain pattern as a brain activation pattern that is specific to original idea generation, implemented in the Pattern_Regression_Matlab ( https://github.com/ZaixuCui/Pattern_Regression_Matlab ). First, we use the positive activation region within the DT brain pattern as ROI. Then, the single-trial beta maps (only NU condition for each subject) as feature to predict originality ratings for DT responses. RVR is a sparse kernel multivariate regression method that uses Bayesian inference to obtain analytic solutions that generalize well and provide inference at low computational cost. RVR has no specific algorithm parameters and does not require additional computational resources to estimate the best specific algorithm parameters. Sample 1 had a total of 2047 NU trials and sample 2 had a total of 846 NU trials. To evaluate the performance, 10 × 10-fold CV procedure on the sapmle1 and sample2 was conducted respectively. All trials were randomly assigned to 10 subsamples, ninefolds were used as the training set, and the remaining fold was used as the testing set. We included gender, age, and head movement as control variables in the analysis. This procedure was repeated ten times, once for each subsample of the test set. Pearson correlation coefficients and mean absolute errors between predicted and actual scores were calculated to provide a final estimate of prediction performance. Prediction performance greater than 95% of the random permutations indicates above-chance accuracy (given an alpha of p < 0.05).
Large-scale resting-state fMRI samples
Participants.
The resting-state fMRI data employed in current study comes from three independent datasets. The main dataset comes from the SLIM project. The validation datasets included data from the GBB project and Behavioral Brain Research Project of Chinese Personality (BBP). All projects were approved by the Southwest University Brain Imaging Center Institutional Review Board. In accordance with the Declaration of Helsinki, all subjects provided written informed consent prior to participation in the study. The recruiting program and exclusion procedures of participants in SLIM and GBB have been described in detail elsewhere 111 , 112 . After matching with behavioral data, the present study included 410 undergraduates from SLIM (233 females, mean age = 20.01 years, SD = 1.24, range = 17–27 years), 304 undergraduates from GBB (218 females, mean age = 19.70 years, SD = 1.73, range = 17–26 years). A total of 804 undergraduates from the BBP were recruited, after excluding 31 participants with excessive head motion (mean FD (Jenkinson) greater than ±0.2 mm) and matching with behavioral data, the present study included 600 undergraduates from BBP (412 females, mean age = 20.7 years, SD = 0.99, range = 17–27 years).
The resting-state fMRI data sets were collected using the same scanner at the Brain Imaging Center of Southwest University. The SLIM and GBB datasets used the same scanning sequence as task-based fMRI data. The BBP images were acquired using Gradient Echo Type Echo Planar Imaging (GRE-EPI) sequence (TR/TE = 2000 ms/30 ms, FA = 90°, resolution matrix = 112 × 112, FOV = 224 × 224 mm 2 , thickness = 2 mm, slices = 62, interslice gap = 0.3 mm, acquisition voxel size = 2 × 2 × 2 mm 3 ). High-resolution three-dimensional T1-weighted structure images were obtained using a MPRAGE sequence (TR/TE = 2530 ms/2.98 ms, FA = 7°, FOV = 224 × 256 mm 2 , resolution matrix = 448 × 512, slices = 192, thickness = 1.0 mm, inversion time = 1100 ms, voxel size = 0.5 × 0.5 × 1 mm 3 ).
Resting-state fMRI data from the different data sets were preprocessed independently. The preprocessing performed for SLIM and GBB datasets using the fMRIPrep default pipeline that is same as task-based data preprocessing. BBP datasets preprocessing using SPM8 ( http://www.fil.ion.ucl.ac.uk/spm ) and the Data Processing & Analysis of Brain Imaging toolbox (DPABI) 113 . We firstly removed the first 10 EPI scans to suppress the equilibration effect, and performed slice timing correction and realignment of the remaining scans. The functional images were then normalized to a standard template (Montreal Neurological Institute) and resampled to 3 × 3 × 3 mm 3 . A Friston 24-parameter model was used to regress nuisance signals including white matter, cerebrospinal fluid, head motion parameters, and their derivatives to control for the potential influence of physiological artifacts. We further implemented data scrubbing to better address head motion concerns. Bad time points were regressed, defined as volumes with frame displacement (FD) power > 0.2 mm, as well as the two succeeding volumes and the one preceding volume to reduce the spillover effect of head motion. In addition, since the BOLD signal exhibits low-frequency drift, linear trends were also used as regressors. Next, we performed spatial smoothing with a 4 mm full-width half-maximum Gaussian kernel and band-pass temporal filtering (0.01–0.1 Hz). Finally, we did not perform global signal regression (GSR) considering several issues: the use of GSR has been controversial because the global signal also includes BOLD fluctuations associated with neurons, especially when these fluctuations are strong and widespread throughout the brain. If the global signal is removed, these neuron-related fluctuations will be removed. GSR introduces a negative bias in the estimated BOLD response, reducing the positive BOLD response and artificially creating a negative response or “deactivation”. Again, the overall effect of GSR in measuring correlation is to force the average correlation across the brain to be zero. GSR may artificially introduce anti-correlations between brain regions that would otherwise not exhibit significant correlations.
Behavioral AUT
To be as consistent as possible with the task-based fMRI, we used behavioral AUT to assess participants’ creativity. The AUT 114 is a commonly used measure of DT ability used to assess participants’ creativity. In this study, two items commonly used or encountered by Chinese people were provided, namely a can and a brick. Participants were asked to imagine and write down a novel use for each item. The instructions were as follows: “Cans (or bricks) have many unusual USES. In the blanks below, write down the interesting and unusual USES you can imagine. You can think of other people’s unexpected uses; the more your ideas, the better; the more novel, the better.” AUT scores measure three components: fluency, flexibility, and originality. Fluency refers to the number of meaningful and relevant responses, related to the ability to generate and consider other possibilities. Flexibility refers to the number of responses in different categories, reflecting an individual’s ability to switch between conceptual domains. Originality refers to the originality of the answer, thereby providing an index of the response’s creative quality, using a 1–5 scale (1 = not creative at all; 5 = very creative) 115 , 116 . The assessment process was conducted separately by different raters in SLIM, GBB and BBP. In this study, we transformed the scores in every dimension into Z scores within each sample, and the total score for creativity (sum of fluency, flexibility, and originality scores) 117 , 118 was used as the final creativity score, for each dimension was highly correlated with the total score for creativity, as well as with each other.
Functional network construction on SLIM
The DT brain pattern can be divided into six clusters by the peak values, namely 1. left AG, 2. bilateral OFC, 3. left MTG/ITG, 4. left Precuneus, 5. bilateral PFC/ACC/Thalamus, 6. right cerebellum. These six clusters were treated as nodes in the analysis. A representative time series of each node for everyone was obtained by averaging the time series of all voxels in it. The Pearson correlation of the time courses between each node pair was calculated and then a Fisher Z-transform was performed to improve normality, resulting in a 6 × 6 symmetric FC matrix with 15 unique edges per participant.
Relevance vector regression analysis on SLIM
We applied RVR to examine the effectiveness of the MVPA-based DT brain pattern as a brain activation pattern that is specific to original idea generation, implemented in the Pattern_Regression_Matlab ( https://github.com/ZaixuCui/Pattern_Regression_Matlab ) with functional connectivity within biomarker (15 unique edges) as feature to predict individual creativity ratings for each subject on SLIM. To evaluate the performance, 10 × 10-fold CV procedure was conducted. All subjects were randomly assigned to 10 subsamples, ninefolds were used as the training set, and the remaining fold was used as the testing set. We included gender, age, and head movement as control variables in the analysis. This procedure was repeated ten times, once for each subsample of the test set. Pearson correlation coefficients and mean absolute errors between predicted and actual scores were calculated to provide a final estimate of prediction performance. Prediction performance greater than 95% of the random permutations indicates above-chance accuracy (given an alpha of p < 0.05). Bootstrap tests were conducted to provide P -values for edge weights to threshold the predictive weights for display and interpretation. We constructed 10,000 bootstrap sample sets (with replacement). Two-tailed, 1000 permutation test were conducted to calculation P -values for each voxel based on the proportion of weights above or below zero. Then we performed FDR multiple comparison correction for the results of permutation test.
Correlation analysis on GBB and BBP
We applied Pearson correlation to examine the effectiveness of the functional connectivity within the DT brain pattern as feature that significantly predicts individual creative ability. Specifically, we conducted the Pearson correlation between functional connectivity (between left AG and left MTG/ITG, left AG and left Precuneus, left MTG/ITG and right cerebellum) and individual DT scores on GBB and BBP.
Statistics and reproducibility
All statistical tests used, sample sizes, and the number of replicates are described in the corresponding methods.
Reporting summary
Further information on research design is available in the Nature Portfolio Reporting Summary linked to this article.
Data availability
The data and material used in this study are available from the corresponding author upon reasonable request. The source data behind the figures in the paper can be found in Supplementary Data 1 .
Barbot, B., Hass, R. W. & Reiter-Palmon, R. Creativity assessment in psychological research:(Re) setting the standards. Psychol. Aesthet. Creat. Arts 13 , 233 (2019).
Article Google Scholar
Sun, M., Wang, M. & Wegerif, R. Effects of divergent thinking training on students’ scientific creativity: the impact of individual creative potential and domain knowledge. Think. Skills Creat. 37 , 100682 (2020).
Akbari Chermahini, S. & Hommel, B. Creative mood swings: divergent and convergent thinking affect mood in opposite ways. Psychol. Res. 76 , 634–640 (2012).
Article PubMed Google Scholar
Guilford, J. P. Measurement and creativity. Theory Pract. 5 , 185–189 (1966).
Kim, K. H. Meta‐analyses of the relationship of creative achievement to both IQ and divergent thinking test scores. J. Creat. Behav. 42 , 106–130 (2008).
Plucker, J. A. Is the proof in the pudding? Reanalyses of Torrance’s (1958 to present) longitudinal data. Creat. Res. J. 12 , 103–114 (1999).
Runco, M. A. & Acar, S. Do tests of divergent thinking have an experiential bias? Psychol. Aesthet. Creat. Arts 4 , 144 (2010).
Abraham, A. et al. Creativity and the brain: uncovering the neural signature of conceptual expansion. Neuropsychologia 50 , 1906–1917 (2012).
Fink, A. et al. The creative brain: Investigation of brain activity during creative problem solving by means of EEG and fMRI. Hum. Brain Mapp. 30 , 734–748 (2009).
Fink, A. et al. Enhancing creativity by means of cognitive stimulation: evidence from an fMRI study. NeuroImage 52 , 1687–1695 (2010).
Howard-Jones, P. A., Blakemore, S.-J., Samuel, E. A., Summers, I. R. & Claxton, G. Semantic divergence and creative story generation: an fMRI investigation. Cogn. Brain Res. 25 , 240–250 (2005).
Kleibeuker, S. W., Koolschijn, P. C. M., Jolles, D. D., De Dreu, C. K. & Crone, E. A. The neural coding of creative idea generation across adolescence and early adulthood. Front. Hum. Neurosci. 7 , 905 (2013).
Article PubMed PubMed Central Google Scholar
Mashal, N., Faust, M., Hendler, T. & Jung-Beeman, M. An fMRI investigation of the neural correlates underlying the processing of novel metaphoric expressions. Brain Lang. 100 , 115–126 (2007).
Article PubMed CAS Google Scholar
Bekhtereva, N. et al. Study of the brain organization of creativity: II. Positron-emission tomography data. Hum. Physiol. 26 , 516–522 (2000).
Benedek, M., Jauk, E., Sommer, M., Arendasy, M. & Neubauer, A. C. Intelligence, creativity, and cognitive control: The common and differential involvement of executive functions in intelligence and creativity. Intelligence 46 , 73–83 (2014).
Green, A. E., Kraemer, D. J., Fugelsang, J. A., Gray, J. R. & Dunbar, K. N. Neural correlates of creativity in analogical reasoning. J. Exp. Psychol. Learn. Mem. Cogn. 38 , 264 (2012).
Kenett, Y. N. et al. Flexibility of thought in high creative individuals represented by percolation analysis. Proc. Natl Acad. Sci. 115 , 867–872 (2018).
Article PubMed PubMed Central CAS Google Scholar
Nusbaum, E. C. & Silvia, P. J. Are intelligence and creativity really so different?: Fluid intelligence, executive processes, and strategy use in divergent thinking. Intelligence 39 , 36–45 (2011).
Sowden, P. T., Pringle, A. & Gabora, L. The shifting sands of creative thinking: connections to dual-process theory. Think. Reason. 21 , 40–60 (2015).
Beaty, R. E., Silvia, P. J., Nusbaum, E. C., Jauk, E. & Benedek, M. The roles of associative and executive processes in creative cognition. Mem. Cogn. 42 , 1186–1197 (2014).
Beaty, R. E., Benedek, M., Barry Kaufman, S. & Silvia, P. J. Default and executive network coupling supports creative idea production. Sci. Rep. 5 , 10964 (2015).
Beaty, R. E., Benedek, M., Silvia, P. J. & Schacter, D. L. Creative cognition and brain network dynamics. Trends Cogn. Sci. 20 , 87–95 (2016).
Christensen, A. P., Benedek, M., Silvia, P., & Beaty, R. Executive and Default Network Connectivity Reflects Conceptual Interference during Creative Imagery Generation PsyArXiv. https://doi.org/10.31234/osf.io/n438d (2019).
Van Essen, D. C. & Glasser, M. F. Parcellating cerebral cortex: how invasive animal studies inform noninvasive mapmaking in humans. Neuron 99 , 640–663 (2018).
Margulies, D. S. et al. Situating the default-mode network along a principal gradient of macroscale cortical organization. Proc. Natl Acad. Sci. 113 , 12574–12579 (2016).
Poldrack, R. A. Inferring mental states from neuroimaging data: from reverse inference to large-scale decoding. Neuron 72 , 692–697 (2011).
Colantuoni, C. et al. Temporal dynamics and genetic control of transcription in the human prefrontal cortex. Nature 478 , 519–523 (2011).
Douet, V., Chang, L., Cloak, C. & Ernst, T. Genetic influences on brain developmental trajectories on neuroimaging studies: from infancy to young adulthood. Brain Imaging Behav. 8 , 234–250 (2014).
Levitt, P., Harvey, J. A., Friedman, E., Simansky, K. & Murphy, E. H. New evidence for neurotransmitter influences on brain development. Trends Neurosci. 20 , 269–274 (1997).
Bogdan, R. et al. Imaging genetics and genomics in psychiatry: a critical review of progress and potential. Biol. Psychiatry 82 , 165–175 (2017).
Orwig, W. et al. Cortical networks of creative ability trace gene expression profiles of synaptic plasticity in the human brain. Front. Hum. Neurosci. 15 , 694274 (2021).
Kamitani, Y. & Tong, F. Decoding the visual and subjective contents of the human brain. Nat. Neurosci. 8 , 679–685 (2005).
Kragel, P. A., Koban, L., Barrett, L. F. & Wager, T. D. Representation, pattern information, and brain signatures: from neurons to neuroimaging. Neuron 99 , 257–273 (2018).
Arnatkevic̆iūtė, A., Fulcher, B. D. & Fornito, A. A practical guide to linking brain-wide gene expression and neuroimaging data. NeuroImage 189 , 353–367 (2019).
Burt, J. B., Helmer, M., Shinn, M., Anticevic, A. & Murray, J. D. Generative modeling of brain maps with spatial autocorrelation. NeuroImage 220 , 117038 (2020).
Nelson, D. L., McEvoy, C. L. & Dennis, S. What is free association and what does it measure? Mem. Cogn. 28 , 887–899 (2000).
Article CAS Google Scholar
Smallwood, J. et al. The default mode network in cognition: a topographical perspective. Nat. Rev. Neurosci. 22 , 503–513 (2021).
Baddeley, A. Working memory. Science 255 , 556–559 (1992).
Owen, A. M., McMillan, K. M., Laird, A. R. & Bullmore, E. N‐back working memory paradigm: a meta‐analysis of normative functional neuroimaging studies. Hum. Brain Mapp. 25 , 46–59 (2005).
Munakata, Y. et al. A unified framework for inhibitory control. Trends Cogn. Sci. 15 , 453–459 (2011).
Aron, A. R., Robbins, T. W. & Poldrack, R. A. Inhibition and the right inferior frontal cortex. Trends Cogn. Sci. 8 , 170–177 (2004).
Nee, D. E., Wager, T. D. & Jonides, J. Interference resolution: insights from a meta-analysis of neuroimaging tasks. Cogn. Affect. Behav. Neurosci. 7 , 1–17 (2007).
Marron, T. R., Berant, E., Axelrod, V. & Faust, M. Spontaneous cognition and its relationship to human creativity: a functional connectivity study involving a chain free association task. NeuroImage 220 , 117064 (2020).
Gerver, C. R., Griffin, J. W., Dennis, N. A., & Beaty, R. E. Memory and creativity: a meta-analytic examination of the relationship between memory systems and creative cognition. Psychon. Bull. Rev. 30 , 2116–2154 (2023).
Camarda, A. et al. Do we need inhibitory control to be creative? Evidence from a dual-task paradigm. Psychol. Aesthet. Creat. Arts 12 , 351 (2018).
Cassotti, M., Agogué, M., Camarda, A., Houdé, O. & Borst, G. Inhibitory control as a core process of creative problem solving and idea generation from childhood to adulthood. New Dir. Child Adolesc. Dev. 2016 , 61–72 (2016).
Chrysikou, E. G. Creativity in and out of (cognitive) control. Curr. Opin. Behav. Sci. 27 , 94–99 (2019).
Buckner, R. L., Andrews‐Hanna, J. R. & Schacter, D. L. The brain’s default network: anatomy, function, and relevance to disease. Ann. N. Y. Acad. Sci. 1124 , 1–38 (2008).
Fox, K. C. & Beaty, R. E. Mind-wandering as creative thinking: neural, psychological, and theoretical considerations. Curr. Opin. Behav. Sci. 27 , 123–130 (2019).
Andrews‐Hanna, J. R., Smallwood, J. & Spreng, R. N. The default network and self‐generated thought: Component processes, dynamic control, and clinical relevance. Ann. N. Y. Acad. Sci. 1316 , 29–52 (2014).
Shi, L. et al. Large-scale brain network connectivity underlying creativity in resting-state and task fMRI: cooperation between default network and frontal-parietal network. Biol. Psychol. 135 , 102–111 (2018).
Bashwiner, D. M., Wertz, C. J., Flores, R. A. & Jung, R. E. Musical creativity “revealed” in brain structure: interplay between motor, default mode and limbic networks. Sci. Rep. 6 , 1–8 (2016).
Beaty, R. E. et al. Default network contributions to episodic and semantic processing during divergent creative thinking: a representational similarity analysis. NeuroImage 209 , 116499 (2020).
Marron, T. R. et al. Chain free association, creativity, and the default mode network. Neuropsychologia 118 , 40–58 (2018).
Shen, K. K. et al. Structural core of the executive control network: a high angular resolution diffusion MRI study. Hum. Brain Mapp. 41 , 1226–1236 (2020).
Niendam, T. A. et al. Meta-analytic evidence for a superordinate cognitive control network subserving diverse executive functions. Cogn. Affect. Behav. Neurosci. 12 , 241–268 (2012).
Seeley, W. W. et al. Dissociable intrinsic connectivity networks for salience processing and executive control. J. Neurosci. 27 , 2349–2356 (2007).
Beaty, R. E. & Silvia, P. J. Why do ideas get more creative across time? An executive interpretation of the serial order effect in divergent thinking tasks. Psychol. Aesthet. Creat. Arts 6 , 309 (2012).
Beaty, R. E. & Silvia, P. J. Metaphorically speaking: cognitive abilities and the production of figurative language. Mem. Cogn. 41 , 255–267 (2013).
Gilhooly, K. J., Fioratou, E., Anthony, S. H. & Wynn, V. Divergent thinking: strategies and executive involvement in generating novel uses for familiar objects. Br. J. Psychol. 98 , 611–625 (2007).
Shi, L. et al. Brain entropy is associated with divergent thinking. Cereb. Cortex 30 , 708–717 (2020).
PubMed Google Scholar
Heinonen, J. et al. Default mode and executive networks areas: association with the serial order in divergent thinking. PLoS ONE 11 , e0162234 (2016).
Silvia, P. J., Beaty, R. E. & Nusbaum, E. C. Verbal fluency and creativity: general and specific contributions of broad retrieval ability (Gr) factors to divergent thinking. Intelligence 41 , 328–340 (2013).
Wu, Y. & Koutstaal, W. Charting the contributions of cognitive flexibility to creativity: Self-guided transitions as a process-based index of creativity-related adaptivity. PLoS ONE 15 , e0234473 (2020).
Zabelina, D. L. & Robinson, M. D. Creativity as flexible cognitive control. Psychol. Aesthet. Creat. Arts 4 , 136 (2010).
Preiss, D. D. Metacognition, mind wandering, and cognitive flexibility: understanding creativity. J. Intell. 10 , 69 (2022).
Wang, M. et al. Specific recognition, intracellular assay and detoxification of fluorescent curcumin derivative for copper ions. J. Hazard. Mater. 420 , 126490 (2021).
Algov, I., Feiertag, A., Shikler, R. & Alfonta, L. Sensitive enzymatic determination of neurotransmitters in artificial sweat. Biosens. Bioelectron. 210 , 114264 (2022).
Nam, E., Nam, G., & Lim, M. H. Synaptic Copper, Amyloid-β, and Neurotransmitters in Alzheimer’s Disease (ACS Publications, 2019).
Boot, N., Baas, M., van Gaal, S., Cools, R. & De Dreu, C. K. Creative cognition and dopaminergic modulation of fronto-striatal networks: Integrative review and research agenda. Neurosci. Biobehav. Rev. 78 , 13–23 (2017).
Lin, W.-L., Tsai, P.-H., Lin, H.-Y. & Chen, H.-C. How does emotion influence different creative performances? The mediating role of cognitive flexibility. Cogn. Emot. 28 , 834–844 (2014).
Baas, M., De Dreu, C. K. & Nijstad, B. A. A meta-analysis of 25 years of mood-creativity research: Hedonic tone, activation, or regulatory focus? Psychol. Bull. 134 , 779 (2008).
Volf, N. & Tarasova, I. The influence of reward on the performance of verbal creative tasks: behavioral and EEG effects. Hum. Physiol. 39 , 302–308 (2013).
Muhle-Karbe, P. S. & Krebs, R. M. On the influence of reward on action-effect binding. Front. Psychol. 3 , 450 (2012).
Zabelina, D. L., Colzato, L., Beeman, M. & Hommel, B. Dopamine and the creative mind: Individual differences in creativity are predicted by interactions between dopamine genes DAT and COMT. PLoS ONE 11 , e0146768 (2016).
Kranz, G., Kasper, S. & Lanzenberger, R. Reward and the serotonergic system. Neuroscience 166 , 1023–1035 (2010).
Le Merrer, J., Becker, J. A., Befort, K. & Kieffer, B. L. Reward processing by the opioid system in the brain. Physiol. Rev. 89 , 1379–1412 (2009).
Nummenmaa, L. et al. μ-opioid receptor system mediates reward processing in humans. Nat. Commun. 9 , 1500 (2018).
Severino, A. L. et al. μ-Opioid receptors on distinct neuronal populations mediate different aspects of opioid reward-related behaviors. eNeuro 7 , ENEURO.0146–20.2020 (2020).
Kowal, M. A. et al. Cannabis and creativity: highly potent cannabis impairs divergent thinking in regular cannabis users. Psychopharmacology 232 , 1123–1134 (2015).
Vohora, D. & Bhowmik, M. Histamine H3 receptor antagonists/inverse agonists on cognitive and motor processes: relevance to Alzheimer’s disease, ADHD, schizophrenia, and drug abuse. Front. Syst. Neurosci. 6 , 72 (2012).
Sadek, B., Saad, A., Sadeq, A., Jalal, F. & Stark, H. Histamine H3 receptor as a potential target for cognitive symptoms in neuropsychiatric diseases. Behav. Brain Res. 312 , 415–430 (2016).
Abd-Elrahman, K. S. & Ferguson, S. S. Noncanonical metabotropic glutamate receptor 5 signaling in Alzheimer’s disease. Annu. Rev. Pharmacol. Toxicol. 62 , 235–254 (2022).
Ding, K. et al. Recognizing ideas generated in a creative thinking task: effect of the subjective novelty. Curr. Psychol. 42 , 529–541 (2021).
Chen, Q. et al. Common brain activation and connectivity patterns supporting the generation of creative uses and creative metaphors. Neuropsychologia 181 , 108487 (2023).
Ding, K., He, R., Wang, X., Chen, Q. & Kenett, Y. N. Recognizing ideas generated in a creative task: the roles of the hippocampus and medial prefrontal cortex in facilitating self-generated learning. Cereb. Cortex 34 , bhae219 (2024).
Madore, K. P., Thakral, P. P., Beaty, R. E., Addis, D. R. & Schacter, D. L. Neural mechanisms of episodic retrieval support divergent creative thinking. Cereb. Cortex 29 , 150–166 (2019).
Yeo, B. T. et al. The organization of the human cerebral cortex estimated by intrinsic functional connectivity. J. Neurophysiol. 106 , 1125–1165 (2011).
Markello, R. D. et al. Neuromaps: structural and functional interpretation of brain maps. Nat. Methods 19 , 1472–1479 (2022).
Hawrylycz, M. J. et al. An anatomically comprehensive atlas of the adult human brain transcriptome. Nature 489 , 391–399 (2012).
Markello, R. D. et al. Standardizing workflows in imaging transcriptomics with the abagen toolbox. eLife 10 , e72129 (2021).
Eden, E., Navon, R., Steinfeld, I., Lipson, D. & Yakhini, Z. GOrilla: a tool for discovery and visualization of enriched GO terms in ranked gene lists. BMC Bioinform. 10 , 48 (2009).
Bedard, M.-A. et al. Brain cholinergic alterations in idiopathic REM sleep behaviour disorder: a PET imaging study with 18F-FEOBV. Sleep. Med. 58 , 35–41 (2019).
Beliveau, V. et al. A high-resolution in vivo atlas of the human brain’s serotonin system. J. Neurosci. 37 , 120–128 (2017).
PubMed PubMed Central CAS Google Scholar
Aghourian, M. et al. Quantification of brain cholinergic denervation in Alzheimer’s disease using PET imaging with [18F]-FEOBV. Mol. Psychiatry 22 , 1531–1538 (2017).
Ding, Y. S. et al. PET imaging of the effects of age and cocaine on the norepinephrine transporter in the human brain using (S, S)‐[11C] O‐methylreboxetine and HRRT. Synapse 64 , 30–38 (2010).
DuBois, J. M. et al. Characterization of age/sex and the regional distribution of mglur5 availability in the healthy human brain measured by high-resolution [11 c] abp688 pet. Eur. J. Nucl. Med. Mol. Imaging 43 , 152–162 (2016).
Dukart, J. et al. Cerebral blood flow predicts differential neurotransmitter activity. Sci. Rep. 8 , 4074 (2018).
Gallezot, J.-D. et al. Kinetic modeling of the serotonin 5-HT1B receptor radioligand [11C] P943 in humans. J. Cereb. Blood Flow. Metab. 30 , 196–210 (2010).
Hillmer, A. T. et al. Imaging of cerebral α4β2* nicotinic acetylcholine receptors with (−)-[18F] Flubatine PET: Implementation of bolus plus constant infusion and sensitivity to acetylcholine in human brain. NeuroImage 141 , 71–80 (2016).
Kaller, S. et al. Test–retest measurements of dopamine d 1-type receptors using simultaneous pet/mri imaging. Eur. J. Nucl. Med. Mol. Imaging 44 , 1025–1032 (2017).
Kantonen, T. et al. Interindividual variability and lateralization of μ-opioid receptors in the human brain. NeuroImage 217 , 116922 (2020).
Naganawa, M. et al. First-in-human assessment of 11c-lsn3172176, an m1 muscarinic acetylcholine receptor pet radiotracer. J. Nucl. Med. 62 , 553–560 (2021).
Nørgaard, M. et al. A high-resolution in vivo atlas of the human brain’s benzodiazepine binding site of GABAA receptors. NeuroImage 232 , 117878 (2021).
Normandin, M. D. et al. Imaging the cannabinoid cb1 receptor in humans with [11c] omar: assessment of kinetic analysis methods, test–retest reproducibility, and gender differences. J. Cereb. Blood Flow Metab. 35 , 1313–1322 (2015).
Parker, C. A. et al. Human kinetic modeling of the 5HT6 PET radioligand 11C-GSK215083 and its utility for determining occupancy at both 5HT6 and 5HT2A receptors by SB742457 as a potential therapeutic mechanism of action in Alzheimer disease. J. Nucl. Med. 56 , 1901–1909 (2015).
Sandiego, C. M. et al. Reference region modeling approaches for amphetamine challenge studies with [11C] FLB 457 and PET. J. Cereb. Blood Flow. Metab. 35 , 623–629 (2015).
Savli, M. et al. Normative database of the serotonergic system in healthy subjects using multi-tracer PET. NeuroImage 63 , 447–459 (2012).
Smart, K. et al. Sex differences in [11 c] abp688 binding: a positron emission tomography study of mglu5 receptors. Eur. J. Nucl. Med. Mol. Imaging 46 , 1179–1183 (2019).
Hansen, J. Y. et al. Mapping neurotransmitter systems to the structural and functional organization of the human neocortex. Nat. Neurosci. 25 , 1569–1581 (2022).
Chen, Q. et al. Longitudinal alterations of frontoparietal and frontotemporal networks predict future creative cognitive ability. Cereb. Cortex 28 , 103–115 (2018).
Liu, W. et al. Longitudinal test-retest neuroimaging data from healthy young adults in southwest China. Sci. Data 4 , 1–9 (2017).
Yan, C.-G., Wang, X.-D., Zuo, X.-N. & Zang, Y.-F. DPABI: data processing & analysis for (resting-state) brain imaging. Neuroinformatics 14 , 339–351 (2016).
Guilford, J. P. The Nature of Human Intelligence (1967).
Silvia, P. J. et al. Assessing creativity with divergent thinking tasks: exploring the reliability and validity of new subjective scoring methods. Psychol. Aesthet. Creat. Arts 2 , 68 (2008).
Guo, J., Ge, Y. & Pang, W. The underlying cognitive mechanisms of the rater effect in creativity assessment: The mediating role of perceived semantic distance. Think. Skills Creat. 33 , 100572 (2019).
Wei, D. et al. Increased resting functional connectivity of the medial prefrontal cortex in creativity by means of cognitive stimulation. Cortex 51 , 92–102 (2014).
de Souza, L. C. et al. Poor creativity in frontotemporal dementia: a window into the neural bases of the creative mind. Neuropsychologia 48 , 3733–3742 (2010).
Download references
Acknowledgements
This research was supported by the Major Research Plan of National Social Science Foundation of China (21&ZD312), National Natural Science Foundation of China (32071070), Natural Science Foundation of Chongqing (cstc2019jcyj-msxmX0520, cstc2020jcyj-msxmX0299), the Research Program Funds of the Collaborative Innovation Center of Assessment toward Basic Education Quality at Beijing Normal University, the planned project of Chongqing humanities and Social Sciences (2018PY80, 2019PY51), 111 program (B21036), Fundamental Research Funds for the Central Universities (SWU119007), Chang Jiang Scholars Program, National Outstanding Young People Plan and Chongqing Talent Program, Innovation Research 2035 Pilot Plan of Southwest University (SWUPilotPlan005).
Author information
Authors and affiliations.
Key Laboratory of Cognition and Personality of Ministry of Education, Faculty of Psychology, Southwest University, Chongqing, China
Cheng Liu, Qunlin Chen, Xueyang Wang, Qiuyang Feng & Jiang Qiu
Institute of Science and Technology for Brain-Inspired Intelligence, Fudan University, Shanghai, China
Kaixiang Zhuang
Department of Psychology, Pennsylvania State University, Pennsylvania, USA
Daniel C. Zeitlen & Roger E. Beaty
You can also search for this author in PubMed Google Scholar
Contributions
C. Liu, Q.L. Chen and J. Qiu designed the study. C. Liu analyzed the data. C. Liu drafted the manuscript. C. Liu, K.X. Zhuang, D.C. Zeitlen, X.Y. W, Q.Y. Feng, R.E. Beaty contributed to revisions and approved the final version of the manuscript for submission.
Corresponding author
Correspondence to Jiang Qiu .
Ethics declarations
Competing interests.
The authors declare no competing interests.
Peer review
Peer review information.
Communications Biology thanks Bernhard Hommel and the other, anonymous, reviewers for their contribution to the peer review of this work. Primary Handling Editor: Benjamin Bessieres.
Additional information
Publisher’s note Springer Nature remains neutral with regard to jurisdictional claims in published maps and institutional affiliations.
Supplementary information
Supplementary information, description of additional supplementary files, supplementary data 1, rights and permissions.
Open Access This article is licensed under a Creative Commons Attribution-NonCommercial-NoDerivatives 4.0 International License, which permits any non-commercial use, sharing, distribution and reproduction in any medium or format, as long as you give appropriate credit to the original author(s) and the source, provide a link to the Creative Commons licence, and indicate if you modified the licensed material. You do not have permission under this licence to share adapted material derived from this article or parts of it. The images or other third party material in this article are included in the article’s Creative Commons licence, unless indicated otherwise in a credit line to the material. If material is not included in the article’s Creative Commons licence and your intended use is not permitted by statutory regulation or exceeds the permitted use, you will need to obtain permission directly from the copyright holder. To view a copy of this licence, visit http://creativecommons.org/licenses/by-nc-nd/4.0/ .
Reprints and permissions
About this article
Cite this article.
Liu, C., Zhuang, K., Zeitlen, D.C. et al. Neural, genetic, and cognitive signatures of creativity. Commun Biol 7 , 1324 (2024). https://doi.org/10.1038/s42003-024-07007-6
Download citation
Received : 07 April 2024
Accepted : 02 October 2024
Published : 15 October 2024
DOI : https://doi.org/10.1038/s42003-024-07007-6
Share this article
Anyone you share the following link with will be able to read this content:
Sorry, a shareable link is not currently available for this article.
Provided by the Springer Nature SharedIt content-sharing initiative
Quick links
- Explore articles by subject
- Guide to authors
- Editorial policies
Sign up for the Nature Briefing newsletter — what matters in science, free to your inbox daily.

Our systems are now restored following recent technical disruption, and we’re working hard to catch up on publishing. We apologise for the inconvenience caused. Find out more: https://www.cambridge.org/universitypress/about-us/news-and-blogs/cambridge-university-press-publishing-update-following-technical-disruption
We use cookies to distinguish you from other users and to provide you with a better experience on our websites. Close this message to accept cookies or find out how to manage your cookie settings .
Login Alert
- > Critical Thinking in Psychology
- > The Nature and Nurture of Critical Thinking
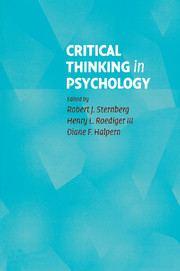
Book contents
- Frontmatter
- List of Illustrations and Tables
- List of Contributors
- 1 The Nature and Nurture of Critical Thinking
- 2 Evaluating Experimental Research
- 3 Critical Thinking in Quasi-Experimentation
- 4 Evaluating Surveys and Questionnaires
- 5 Critical Thinking in Designing and Analyzing Research
- 6 The Case Study Perspective on Psychological Research
- 7 Informal Logical Fallacies
- 8 Designing Studies to Avoid Confounds
- 9 Evaluating Theories
- 10 Not All Experiments Are Created Equal
- 11 Making Claims in Papers and Talks
- 12 Critical Thinking in Clinical Inference
- 13 Evaluating Parapsychological Claims
- 14 Why Would Anyone Do or Believe Such a Thing?
- 15 The Belief Machine
- 16 Critical Thinking and Ethics in Psychology
- 17 Critical Thinking in Psychology
- Author Index
- Subject Index
1 - The Nature and Nurture of Critical Thinking
Published online by Cambridge University Press: 05 June 2012
It was during a presentation on ways to enhance critical thinking in college classes that a jaded faculty member shot back at me, “What kind of thinking do you think I teach – noncritical thinking?” I assured this faculty member that no offense had been intended, although certainly it had been taken. In fact, often there is noncritical, or more appropriately labeled, rote memorization or lower level thinking that is taught and tested in many classrooms at all levels of education at the expense of higher order or critical thinking.
NONCRITICAL THINKING
Consider, for example, typical questions that might be found on tests given in developmental psychology classes. There is the ubiquitous question that asks students to list each stage of Piaget's theory of cognitive development, along with the age range for each stage, and an example of a cognitive task that can be accomplished at each stage. This is a basic recall question, even though there is an opportunity to provide an example, which allows for the application of the knowledge of what cognitive abilities become possible at each stage of development. The example given is almost always the same as an example that was presented in class or in the text. If this is the extent of students' knowledge, they are unlikely to be able to use Piaget's conceptualization of cognitive development in any applied setting (such as designing an age-appropriate toy or activity for a preschool) or in a novel or useful way.
Access options
Save book to kindle.
To save this book to your Kindle, first ensure [email protected] is added to your Approved Personal Document E-mail List under your Personal Document Settings on the Manage Your Content and Devices page of your Amazon account. Then enter the ‘name’ part of your Kindle email address below. Find out more about saving to your Kindle .
Note you can select to save to either the @free.kindle.com or @kindle.com variations. ‘@free.kindle.com’ emails are free but can only be saved to your device when it is connected to wi-fi. ‘@kindle.com’ emails can be delivered even when you are not connected to wi-fi, but note that service fees apply.
Find out more about the Kindle Personal Document Service .
- The Nature and Nurture of Critical Thinking
- By Diane F. Halpern , Claremont McKenna College
- Edited by Robert J. Sternberg , Yale University, Connecticut , Henry L. Roediger III , Washington University, St Louis , Diane F. Halpern , Claremont McKenna College, California
- Book: Critical Thinking in Psychology
- Online publication: 05 June 2012
- Chapter DOI: https://doi.org/10.1017/CBO9780511804632.002
Save book to Dropbox
To save content items to your account, please confirm that you agree to abide by our usage policies. If this is the first time you use this feature, you will be asked to authorise Cambridge Core to connect with your account. Find out more about saving content to Dropbox .
Save book to Google Drive
To save content items to your account, please confirm that you agree to abide by our usage policies. If this is the first time you use this feature, you will be asked to authorise Cambridge Core to connect with your account. Find out more about saving content to Google Drive .
- Find your local Age UK
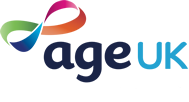
- Site search Search term
- Back Information and advice
- Back Money & legal
- Back Benefits and Entitlements
- Attendance Allowance
- Benefit rates 2024-25
- Benefits Calculator – what are you entitled to?
- Bereavement Support Payment
- Carer's Allowance
- Benefits for mixed age couples
- Changes to the benefits system
- Cold Weather Payment
- Council Tax Reduction
- Disability Living Allowance
- Employment and Support Allowance
- Free bus pass and transport concessions
- Free prescriptions for over 60s
- Housing Benefit
- How to apply for a Blue Badge
- How to challenge a benefits decision through mandatory reconsideration
- How to get help with urgent or one-off expenses
- How your benefits are means tested
- Income Support
- Jobseeker's Allowance
- Pension Credit
- Personal Independence Payment
- Returning to the UK after living abroad
- Support for Mortgage Interest
- TV licence concessions
- Universal Credit
- Warm Home Discount
- Winter Fuel Payment
- More money in your pocket information guide
- Back Debt and savings
- Debt help and advice
- Energy advice for the home
- Financial jargon buster: financial terms explained
- How to reduce your water bills
- How to trace old bank accounts & lost money
- Back Income and tax
- What is equity release and how does it work?
- Ways to reduce Council Tax
- How does income tax work?
- How does Inheritance Tax work?
- How to boost your income in retirement
- Equity release information guide
- Back End of life issues
- Back Legal issues
- Finding legal advice
- How to be an executor information guide
- Making a will
- Power of attorney
- Power of attorney information guide
- Thinking about end of life information guide
- What is a living will (advance decision)?
- What is an executor?
- What to do when someone dies
- Wills and estate planning information guide
- What is probate?
- Back Pensions advice
- Annuities explained
- Finding old pensions
- Pension calculator
- Pensions scams and fraud
- State Pension
- What are workplace pensions?
- What pension options do I have?
- Back Scams and fraud
- How to spot a catfish
- Spotting TV Licence scams
- Doorstep scams
- Postal scams
- Phone scams
- What to do if you're the victim of a scam
- Investment scams and fraud
- Avoiding scams information guide
- Help with the cost of living
- Back Consumer advice
- Are landlines being phased out?
- Back Health & Wellbeing
- Back Conditions and illnesses
- Bladder and bowel problems information guide
- Bowel problems
- Coronavirus
- Depression and anxiety
- Hearing loss
- High blood pressure
- Influenza (flu) prevention
- Malnutrition
- Osteoporosis
- Seasonal affective disorder (SAD)
- Urinary incontinence
- Urinary tract infections (UTIs)
- Back Health services
- Dental services for older people
- 9 health tests that could save your life
- Getting the most from your medicines
- Getting help when feeling unwell
- NHS continuing healthcare
- Your hospital stay
- GP services for older people
- Leaving hospital
- Complaining about NHS services and hospitals
- What standards you should expect from NHS services
- Your hospital stay information guide
- Back Being active
- Getting active when you find exercise difficult
- Getting active but not sure where to start
- Helping someone to get active
- Preventing falls
- How to look after your feet
- Walking tips
- Physical activity videos to use at home
- Staying steady information guide
- Back Mind and body
- 10 tips for ageing better
- 5 tips to boost your mood
- Getting a good night's sleep
- Your mind matters
- Mindfulness
- Staying cool in a heatwave
- Staying sharp
- Preventing and treating burns
- Back Loneliness
- A Life Less Lonely
- Caring for someone who's lonely
- Feeling lonely
- Back Looking after your health and money this winter
- Keeping warm and well in winter
- Keep your spirits up
- Managing money in winter
- Winter wrapped up information guide
- Winter illnesses
- Back Relationships and family
- Financial and legal tips before remarrying
- Planning for end of life
- Protection from abuse and neglect
- Dating in later life
- Sex in later life
- How to deal with grief after a bereavement
- How to adjust to living alone
- Top tips for grandparents
- Worried about someone?
- Back Care & support
- Back Finding and arranging social care
- Care needs assessment
- Homecare: how to find the care you need at home
- Back Paying for care
- Financial assessment explained
- Paying for residential care
- Paying for homecare
- How much does care cost?
- Back Help & advice for carers
- What is a carer's assessment?
- Financial support for carers
- Balancing working and caring responsibilities
- Caring for someone with dementia
- Looking after yourself as a carer
- What is respite care?
- What to do when your caring role changes or ends
- Carer's checklist
- Back Housing options in later life
- Assisted living and extra-care housing
- Safety in the home
- How to make your home safer and more comfortable
- Specialist housing options
- Renting and social housing
- Homelessness
- Moving in with children and family
- Park homes (mobile homes)
- Sheltered housing
- Back Problems with care
- How to complain about care to your local council
- Problems with homecare
- Back Work & learning
- Back Making the most of the internet
- Computer training courses
- Glossary of online terms
- Getting started on the internet
- Staying safe online
- Keeping in touch using a video call
- Making your device easier to use
- How to download apps
- Email essentials
- What's WhatsApp?
- Using social media
- Is online banking safe?
- How to transfer money and make payments
- Shopping safely online
- Online password security
- Age UK's digital instruction guides
- Back Discrimination and rights
- Changes to immigration status – what is an eVisa?
- EU citizens and settled status after Brexit
- Help for Ukrainian nationals in the UK
- Human rights
- The Equality Act
- Windrush Compensation Scheme information guide
- Windrush Scheme
- Your rights at work
- Back Education and training
- Build up your skills
- Classes and courses
- Higher education and university
- u3a – Later life learning
- Back Looking for work
- Be your own boss
- CVs and job applications
- Job interview tips
- Back Retirement
- Preparing emotionally for retirement
- Paul Lewis: Retirement planning advice
- Living abroad after retirement
- Retirement age
- Back For professionals
- Benefits hub for professionals
- Mental health hub for professionals
- Winter hub for professionals
- Information guides and factsheets
Age UK Advice Line 0800 678 1602
Free to call 8am – 7pm 365 days a year Find out more
Telephone friendship
Get a free weekly friendship call. We'll match you with one of our volunteers. Our service is flexible to suit the different needs of everyone who takes part.
- Sign up for telephone befriending
- Find services near you
- Find charity shops near you
Popular services
- Age UK Advice Line
- Befriending services
- The Silver Line Helpline
- Day Centres
- Exercise and physical activity
- Handyperson services
- IT Training
- Social activities
- Back Services
- Back Get involved
- Back Donate
- Make a donation
- Back Leave a legacy donation in your will
- How to leave a gift in your will
- Get your free gifts in wills guide
- Request your free will writing guide
- Making and amending your will to include a gift to Age UK
- Information for executors
- The difference a gift in your will could make
- Ways to write your will
- Free Wills Month 2024
- Will Aid November 2024
- Back Donate to our shops
- Sustainability
- Shop online
- Back Donate in memory
- Make a donation in memory
- Fundraise for Age UK in memory of your loved one
- Make a one-off donation
- Funeral donations and collections
- Set up a Tribute Fund
- Meaningful ways to remember your loved one
- Make a major gift
- The difference your money makes
- Our professional fundraisers
- Back Fundraise
- Back The innocent Big Knit – get knitting for Age UK
- innocent Big Knit updates
- Run for charity
- Back Fundraising ideas
- Most popular ideas
- Sporty ideas
- Fundraise at work
- Fundraise at school
- Fundraise while you celebrate
- Fundraise as a community
- A-Z of fundraising ideas
- Get your free fundraising guide
- Pay in your fundraising
- Fundraising resources
- Leeds Abbey Dash
- Back Stream for Ages
- Your free downloads
- Back Lottery and raffle
- Play the Age UK lottery
- Tuesday Treat Draw
- Play the Age UK raffle
- Gift the Lottery
- Player Zone
- How every entry helps
- Are you a winner?
- Lottery and Raffle rules
- Back Volunteering
- Telephone Friendship Service volunteering
- The Silver Line Helpline volunteering
- Connect and Support Service volunteering
- Age UK shops volunteering
- Local Age UK volunteering
- Support our campaigns
- Back Corporate partnerships
- Why partner with Age UK
- Back Ways we could work together
- Can your company support the Age UK Telephone Friendship Service?
- Help Age UK become Morrisons’ next charity partner
- Latest jobs with Age UK
Age UK. Know what to do.
Last year, over 10 million people trusted us to help them with some of the biggest issues in their lives. Learn more about how we help.
How your support helps
Here's how, with your support, Age UK is supporting older people through the cost of living crisis.
- See the difference your donations make
Making older people's voices heard
- Age UK know what to do
- Campaigning with older people
- Discover - news, stories and features
- Media Centre
- Policy and research
- Wellbeing programmes
- Politics and government
- Back Our impact
- Back Buy products
- Back Over-50s Insurance
- Car insurance
- Home insurance
- Motor breakdown cover
- Travel insurance
- Travel insurance with medical conditions
- Back Independent living
- Bathing Solutions
- Stairlifts and Homelifts
- Personal Alarms
- Back Financial services
- Funeral Plans
- Legal services
- Back Healthcare
- Incontinence products
How and why your thinking skills change
- Cognitive reserve
Genes and thinking skills
- How are thinking skills tested?
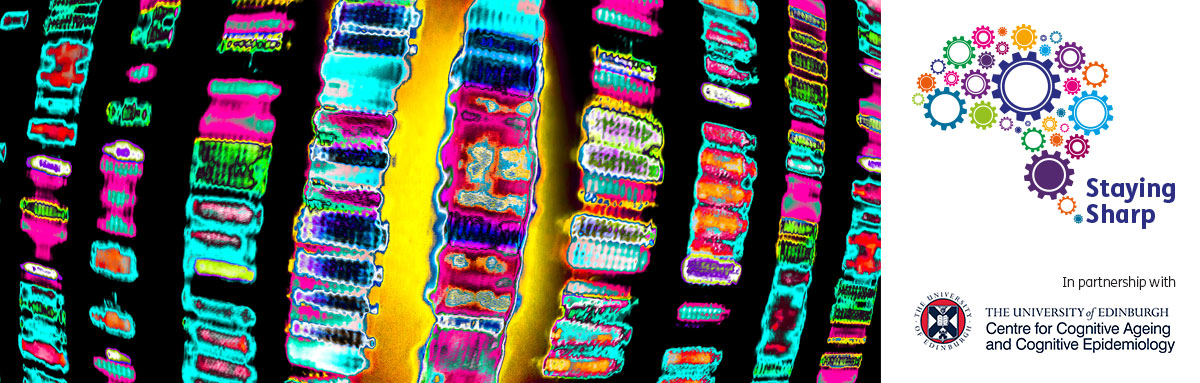
What are genes?
Do genes influence thinking skills, genes and ageing of thinking skills, how do environmental factors affect genes, epigenetics, ageing and the future.
Our bodies are made up of trillions of cells, each with a nucleus. The nucleus houses chromosomes, which are made up of DNA. In turn, DNA contains sequences of genetic code. Each sequence is known as a ‘gene’. Genes provide vital instructions to cells to make proteins that carry out particular functions in the body, e.g. building or repairing bone or muscle cells.
We inherit our genes from our parents. This is the way characteristics and conditions are passed down the generations in families. We have two copies of each gene, one inherited from each parent.
Most genes are the same in everyone. A small number ( about 0.1% of the total ) are different between people. This explains the differences between us that make each of us unique.
Did you know that people have approximately 24,000 genes, slightly more than mice and chimpanzees? [Source: Science Museum ]
As individuals, we vary widely in the level of our thinking skills, or ‘ cognitive function ’.
We inherit cognitive function from our parents, in the same way that physical characteristics are passed down. Scientists have discovered that, unlike eye colour, cognitive function is not influenced by a few genes but by many. Individually, each gene has a small influence but together they account for more than half (or 50%) of the differences in cognitive function between us.
In addition, The Disconnected Mind research project team at the University of Edinburgh has shown that the majority of genetic effects that act on cognitive ability in old age are those that act on cognitive ability in childhood. This means that people with a higher level of cognitive ability in youth are more likely to have a higher level of ability throughout their lives and in older age.
Identifying genes
Scientists are still working to identify genes which contribute to our capacity to think and learn, as so far only a small number have been identified. One gene that has reliably been associated with cognitive function, particularly in older people, is APOE. This gene is involved in cholesterol transport and is likely to influence brain function.
It’s normal for some of our thinking skills to decline slightly as we grow older – including speed of thinking, some types of memory and manipulating new information – while others stay stable or increase, for example wisdom and vocabulary.
But either side of the ‘norm’, we vary widely in the extent and rate of change. In other words, some people may do better in their thinking skills than the norm and others may decline more or faster.
The extent and rate of decline as we age is affected by both genetic influences (what we were born with) and ‘environmental’ influences, i.e. what we encounter or are exposed to during our lives. This includes life experiences such as education, health, lifestyle choices, attitude and emotions, and a range of other factors.
The Disconnected Mind project team studies the relative contribution of genetic and environmental influences to change in cognitive function as we age.
They discovered that approximately 24% of the extent of change between childhood and older age (age 70) is due to genetic influences. This means that environmental influences make a larger contribution, at approximately 76%.
Where these are things we can control or alter (e.g. the choice to smoke or not; decisions we make about diet), we can make changes to help protect our cognitive health as we age.
The genes we are born with never change but they don’t all need to produce the proteins they are responsible for all the time. This means that they can be switched ‘on’ and ‘off’ during our lives. This is known as gene regulation.
Much of the switching that takes place is natural – a normal part of our everyday biology – and is vital over the life course, for instance to build and repair different body cells at the right times. Switching can also happen in response to environmental factors and disease. This enables cells to react quickly to changes in their environment.
Researchers are increasingly interested in studying what switches genes on and off. This relatively new branch of biomedical science is known as ‘epigenetics’. We don’t fully understand it yet, but we do know that the switching is done by chemical modifications to the DNA.
One of the types of modification that has been identified so far is known as ‘methylation’, a process in which methyl groups are added to genes. This changes the activity of that gene without changing the gene itself. In other words, DNA methylation helps control how active or inactive our genes are.
The Disconnected Mind project team is studying what environmental factors trigger DNA methylation of genes that are associated with ageing. They have found, for example, that smoking is an environmental influence that has a large effect – it’s possible to spot current smokers by looking at methylation levels in a single gene.
Links between DNA methylation and schizophrenia and Alzheimer’s disease have also emerged, but further research is needed to find out whether this offers potential for prevention or treatment of these conditions.
The study of epigenetics and epigenetic differences between people is also providing findings on the rate of ageing and how long we live, information about the mechanisms behind diseases and the biological implications of our lifestyle choices.
In time, it will help us learn more about ageing of our brains and thinking skills, providing more understanding of how we can protect their health as we grow older.
Want to read more?
- Looking after your thinking skills
- How your thinking skills change with age
Suggested reading
- Relton, C.L., Hartwig, F.P., Davey Smith, G., 2015. From stem cells to the law courts: DNA methylation, the forensic epigenome and the possibility of a biosocial archive . Int J Epidemiol, 44:4, pp.1083-93.
With thanks for this page to scientists in the University of Edinburgh’s Centre for Cognitive Ageing and Cognitive Epidemiology.
For more information call the Age UK Advice Line on 0800 678 1602 . We’re open 8am to 7pm, every day of the year.
Share this page
- Share on Facebook
Last updated: Sep 20 2022
Become part of our story
- Please enter a valid email address
Back to top
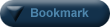
Translate this page from English...
*Machine translated pages not guaranteed for accuracy. Click Here for our professional translations.
Diversity: Making Sense of It Through Critical Thinking
; you will find this article in the Libraries there.
| ||
- DOI: 10.21009/biosferjpb.v12n2.135-143
- Corpus ID: 210123196
Critical thinking, metacognitive skills, and cognitive learning outcomes: a correlation study in genetic studies
- F. J. Miharja , I. Hindun , Ahmad Fauzi
- Published 17 November 2019
- Education, Biology, Psychology
35 Citations
The contribution of metacognitive skills and creative thinking skills in 21st century learning, relationship between critical thinking skills and metacognition awareness with learning outcomes in science learning, analysis of the correlation between critical thinking and metacognitive skills of senior high school students in biology jeneponto regency, south sulawesi, indonesia, guided discovery in genetic learning: implementation and impact on higher-level thinking skills, improving students’ metacognitive abilities and creative thinking skills through stem-based in online learning, international journal of instruction, enhancing students' biology-critical thinking skill through circ-based scientific approach (cirsa), computational thinking and scientific modules: effectiveness on students' cognitive learning outcomes and critical thinking skills, improving critical thinking skills students through problem based learning e-module, demonstration and experiment on archaebacteria and eubacteria: effectiveness for cognitive learning outcomes (clo) based on critical thinking skill, 44 references, the correlation between metacognitive skills and critical thinking skills toward students’ process skills in biology learning, the interplay between reflective thinking, critical thinking, self-monitoring, and academic achievement in higher education, critical thinking skills as related to university students gender and academic discipline., students’ understanding of genetics concepts: the effect of reasoning ability and learning approaches.
- Highly Influential
Investigating Predictive role of Critical Thinking on Metacognition with Structural Equation Modeling
Development of the critical thinking toolkit (critt): a measure of student attitudes and beliefs about critical thinking, metacognitive knowledge: a tool for academic success, lecturers’ perception on students’ critical thinking skills development and problems faced by students in developing their critical thinking skills, teacher perceptions of critical thinking among students and its influence on higher education, purposely teaching for the promotion of higher-order thinking skills: a case of critical thinking, related papers.
Showing 1 through 3 of 0 Related Papers

IMAGES
VIDEO
COMMENTS
Cognition. Critical Thinking: What It Is and Why It Matters. Defining critical thinking dispositions and why they’re crucial. Posted September 23, 2024 | Reviewed by Devon Frye. Key points....
While there may well be biological reasons for why one patient’s brain works a certain way, there’s no such thing as a single “IQ gene”—instead, it is likely hundreds of genes working together and interacting with environmental factors to determine cognitive abilities.
Yes, intelligence (i.e., critical thinking) can be enhanced and used for solving a real-world problem such as COVID-19, which we use as an example of contemporary problems that need a new approach. Keywords: critical thinking, intelligence, real-world problems
Creativity aids in the development of individual problem-solving skills and is critical to the cultural and economic advancement of society. Divergent thinking (DT), the ability to produce ...
Explore the underlying structures of everyday arguments and develop the tools to communicate effectively.
There is the ubiquitous question that asks students to list each stage of Piaget's theory of cognitive development, along with the age range for each stage, and an example of a cognitive task that can be accomplished at each stage.
Scientists have discovered that, unlike eye colour, cognitive function is not influenced by a few genes but by many. Individually, each gene has a small influence but together they account for more than half (or 50%) of the differences in cognitive function between us.
Diversity: Making Sense of It Through Critical Thinking. Within any given group of students, one can expect to find differences along all, or most, of the following parameters: preferred learning styles (including concrete vs. abstract, sequential vs. random, introverted versus extroverted, etc.), race, gender, ethnicity, intellectual skill ...
By developing critical thinking skills, students develop the reasoning tools that can reorient their beliefs and values. Therefore, critical thinking can result in a transformative experience and, in turn, transformative learning.
This study aims to determine the correlation between critical thinking, metacognitive skills, and cognitive learning outcomes in genetic. This study is correlational that measures the relationship between variables of students’ critical thinking skills (X1), metacognitive skills (X2), and students’ cognitive learning outcomes (Y).