

Case Study vs. Experiment: What's the Difference?

Key Differences
Comparison chart, generalization, preferred fields, case study and experiment definitions, when is a case study used, what is an experiment, how do case studies gather data, can case studies be generalized, what fields commonly use case studies, what type of data do experiments produce, can a case study include quantitative data, what is a case study, what is the main purpose of an experiment, is bias a concern in case studies, are experiments replicable, in which fields are experiments predominant, how do experiments impact scientific knowledge, what is a longitudinal case study, can experiments be conducted in natural settings, what is the role of control in experiments, how long can a case study last, what ethical considerations are involved in experiments, can case studies be used to test hypotheses, how is replicability ensured in experiments.

Trending Comparisons

Popular Comparisons
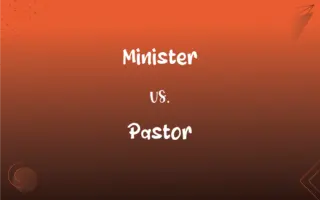
New Comparisons

- En español – ExME
- Em português – EME
An introduction to different types of study design
Posted on 6th April 2021 by Hadi Abbas
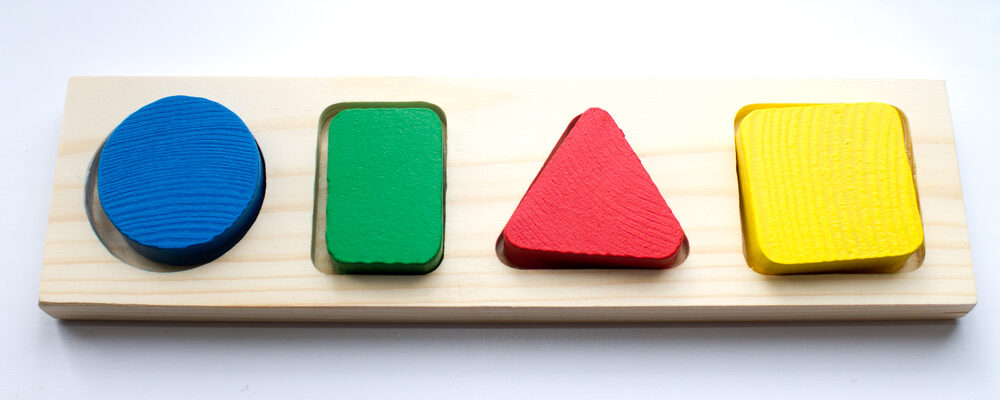
Study designs are the set of methods and procedures used to collect and analyze data in a study.
Broadly speaking, there are 2 types of study designs: descriptive studies and analytical studies.
Descriptive studies
- Describes specific characteristics in a population of interest
- The most common forms are case reports and case series
- In a case report, we discuss our experience with the patient’s symptoms, signs, diagnosis, and treatment
- In a case series, several patients with similar experiences are grouped.
Analytical Studies
Analytical studies are of 2 types: observational and experimental.
Observational studies are studies that we conduct without any intervention or experiment. In those studies, we purely observe the outcomes. On the other hand, in experimental studies, we conduct experiments and interventions.
Observational studies
Observational studies include many subtypes. Below, I will discuss the most common designs.
Cross-sectional study:
- This design is transverse where we take a specific sample at a specific time without any follow-up
- It allows us to calculate the frequency of disease ( p revalence ) or the frequency of a risk factor
- This design is easy to conduct
- For example – if we want to know the prevalence of migraine in a population, we can conduct a cross-sectional study whereby we take a sample from the population and calculate the number of patients with migraine headaches.
Cohort study:
- We conduct this study by comparing two samples from the population: one sample with a risk factor while the other lacks this risk factor
- It shows us the risk of developing the disease in individuals with the risk factor compared to those without the risk factor ( RR = relative risk )
- Prospective : we follow the individuals in the future to know who will develop the disease
- Retrospective : we look to the past to know who developed the disease (e.g. using medical records)
- This design is the strongest among the observational studies
- For example – to find out the relative risk of developing chronic obstructive pulmonary disease (COPD) among smokers, we take a sample including smokers and non-smokers. Then, we calculate the number of individuals with COPD among both.
Case-Control Study:
- We conduct this study by comparing 2 groups: one group with the disease (cases) and another group without the disease (controls)
- This design is always retrospective
- We aim to find out the odds of having a risk factor or an exposure if an individual has a specific disease (Odds ratio)
- Relatively easy to conduct
- For example – we want to study the odds of being a smoker among hypertensive patients compared to normotensive ones. To do so, we choose a group of patients diagnosed with hypertension and another group that serves as the control (normal blood pressure). Then we study their smoking history to find out if there is a correlation.
Experimental Studies
- Also known as interventional studies
- Can involve animals and humans
- Pre-clinical trials involve animals
- Clinical trials are experimental studies involving humans
- In clinical trials, we study the effect of an intervention compared to another intervention or placebo. As an example, I have listed the four phases of a drug trial:
I: We aim to assess the safety of the drug ( is it safe ? )
II: We aim to assess the efficacy of the drug ( does it work ? )
III: We want to know if this drug is better than the old treatment ( is it better ? )
IV: We follow-up to detect long-term side effects ( can it stay in the market ? )
- In randomized controlled trials, one group of participants receives the control, while the other receives the tested drug/intervention. Those studies are the best way to evaluate the efficacy of a treatment.
Finally, the figure below will help you with your understanding of different types of study designs.
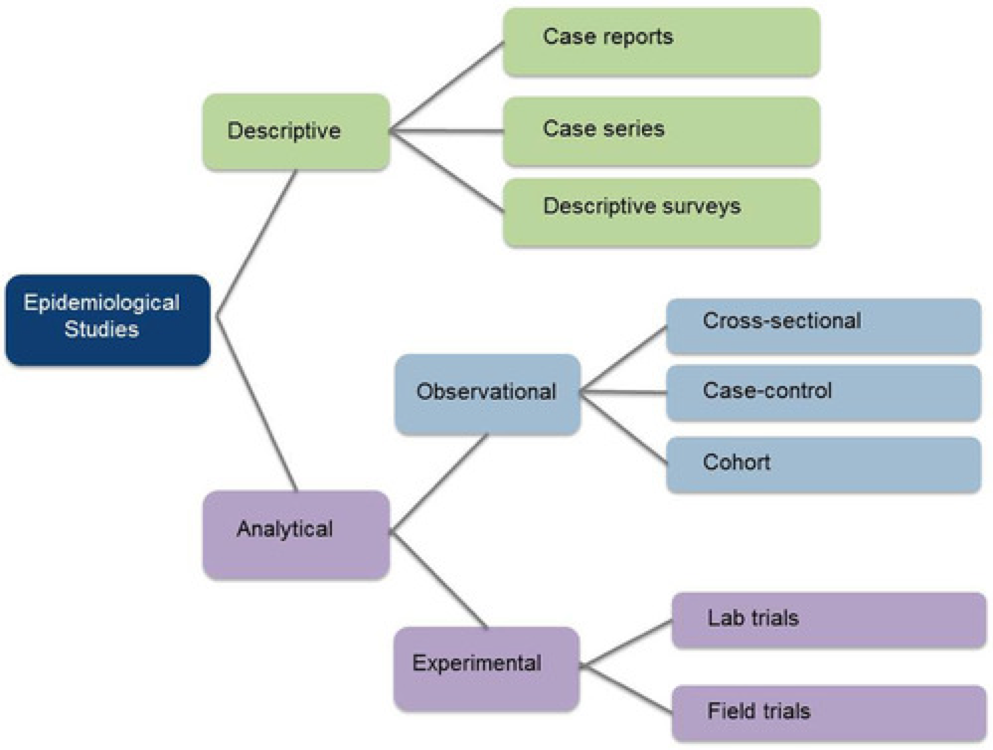
References (pdf)
You may also be interested in the following blogs for further reading:
An introduction to randomized controlled trials
Case-control and cohort studies: a brief overview
Cohort studies: prospective and retrospective designs
Prevalence vs Incidence: what is the difference?
Leave a Reply Cancel reply
Your email address will not be published. Required fields are marked *
Save my name, email, and website in this browser for the next time I comment.
No Comments on An introduction to different types of study design
you are amazing one!! if I get you I’m working with you! I’m student from Ethiopian higher education. health sciences student
Very informative and easy understandable
You are my kind of doctor. Do not lose sight of your objective.
Wow very erll explained and easy to understand
I’m Khamisu Habibu community health officer student from Abubakar Tafawa Balewa university teaching hospital Bauchi, Nigeria, I really appreciate your write up and you have make it clear for the learner. thank you
well understood,thank you so much
Well understood…thanks
Simply explained. Thank You.
Thanks a lot for this nice informative article which help me to understand different study designs that I felt difficult before
That’s lovely to hear, Mona, thank you for letting the author know how useful this was. If there are any other particular topics you think would be useful to you, and are not already on the website, please do let us know.
it is very informative and useful.
thank you statistician
Fabulous to hear, thank you John.
Thanks for this information
Thanks so much for this information….I have clearly known the types of study design Thanks
That’s so good to hear, Mirembe, thank you for letting the author know.
Very helpful article!! U have simplified everything for easy understanding
I’m a health science major currently taking statistics for health care workers…this is a challenging class…thanks for the simified feedback.
That’s good to hear this has helped you. Hopefully you will find some of the other blogs useful too. If you see any topics that are missing from the website, please do let us know!
Hello. I liked your presentation, the fact that you ranked them clearly is very helpful to understand for people like me who is a novelist researcher. However, I was expecting to read much more about the Experimental studies. So please direct me if you already have or will one day. Thank you
Dear Ay. My sincere apologies for not responding to your comment sooner. You may find it useful to filter the blogs by the topic of ‘Study design and research methods’ – here is a link to that filter: https://s4be.cochrane.org/blog/topic/study-design/ This will cover more detail about experimental studies. Or have a look on our library page for further resources there – you’ll find that on the ‘Resources’ drop down from the home page.
However, if there are specific things you feel you would like to learn about experimental studies, that are missing from the website, it would be great if you could let me know too. Thank you, and best of luck. Emma
Great job Mr Hadi. I advise you to prepare and study for the Australian Medical Board Exams as soon as you finish your undergrad study in Lebanon. Good luck and hope we can meet sometime in the future. Regards ;)
You have give a good explaination of what am looking for. However, references am not sure of where to get them from.
Subscribe to our newsletter
You will receive our monthly newsletter and free access to Trip Premium.
Related Articles
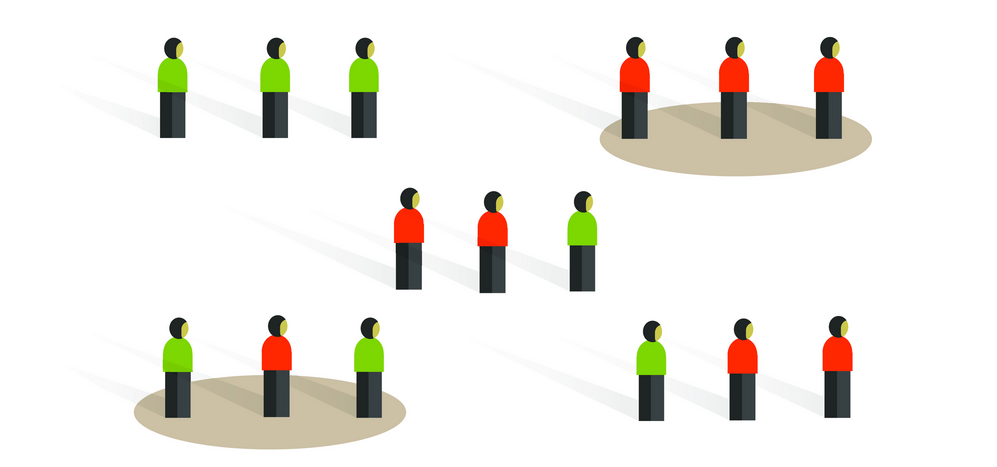
Cluster Randomized Trials: Concepts
This blog summarizes the concepts of cluster randomization, and the logistical and statistical considerations while designing a cluster randomized controlled trial.
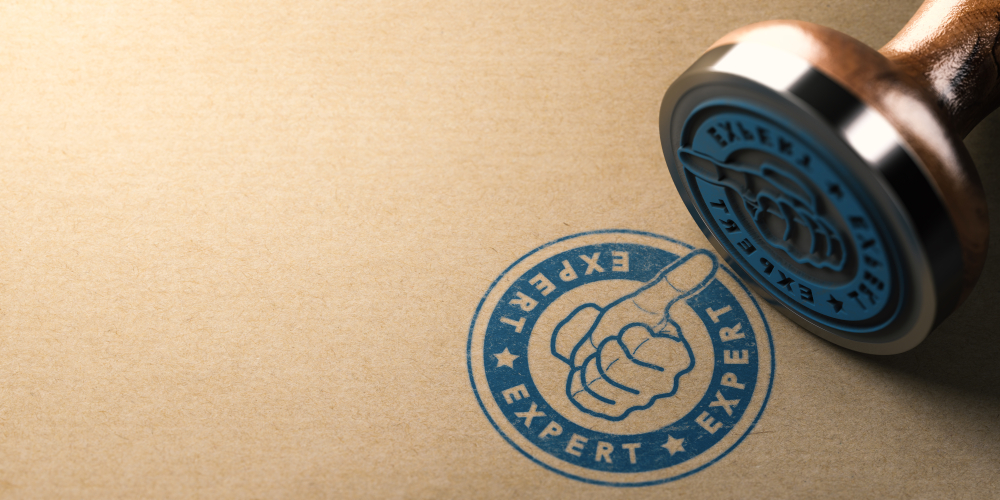
Expertise-based Randomized Controlled Trials
This blog summarizes the concepts of Expertise-based randomized controlled trials with a focus on the advantages and challenges associated with this type of study.
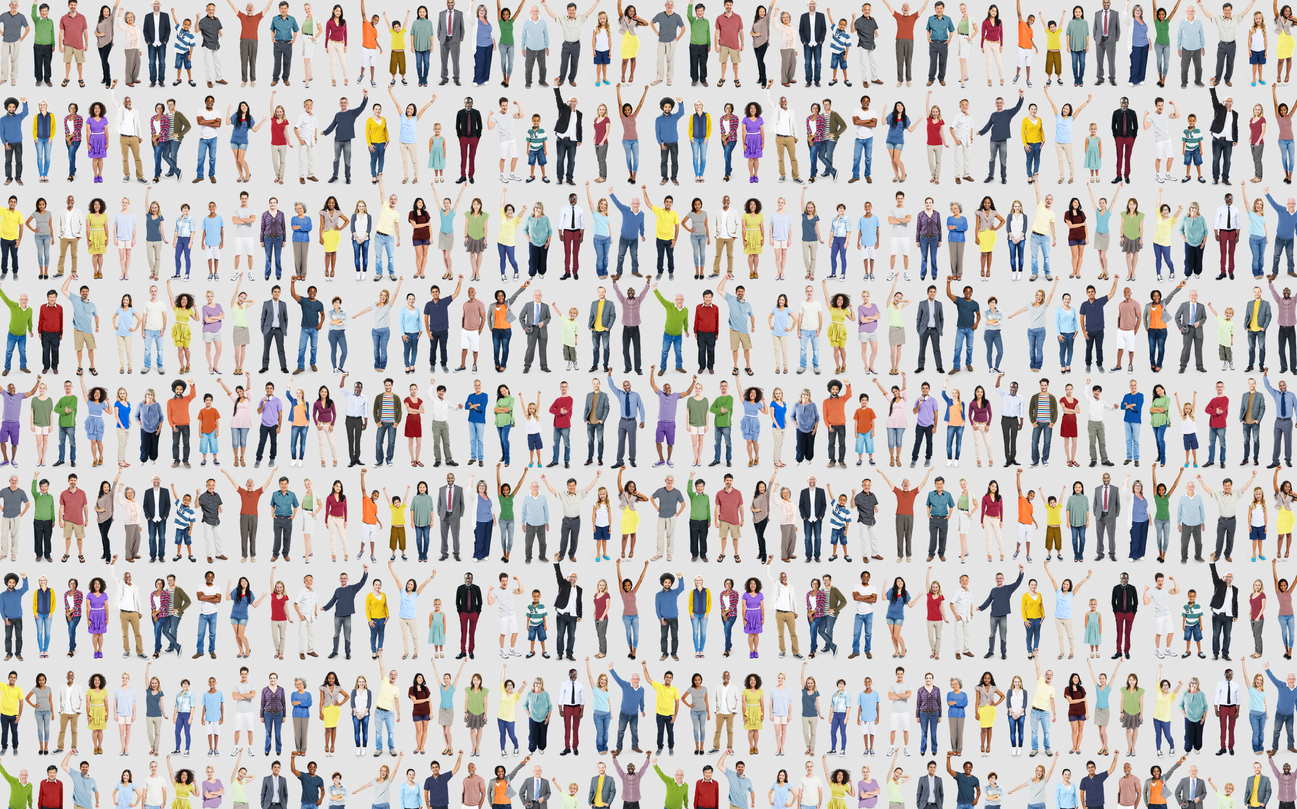
A well-designed cohort study can provide powerful results. This blog introduces prospective and retrospective cohort studies, discussing the advantages, disadvantages and use of these type of study designs.
Experimental and Quasi-Experimental Research
Guide Title: Experimental and Quasi-Experimental Research Guide ID: 64
You approach a stainless-steel wall, separated vertically along its middle where two halves meet. After looking to the left, you see two buttons on the wall to the right. You press the top button and it lights up. A soft tone sounds and the two halves of the wall slide apart to reveal a small room. You step into the room. Looking to the left, then to the right, you see a panel of more buttons. You know that you seek a room marked with the numbers 1-0-1-2, so you press the button marked "10." The halves slide shut and enclose you within the cubicle, which jolts upward. Soon, the soft tone sounds again. The door opens again. On the far wall, a sign silently proclaims, "10th floor."
You have engaged in a series of experiments. A ride in an elevator may not seem like an experiment, but it, and each step taken towards its ultimate outcome, are common examples of a search for a causal relationship-which is what experimentation is all about.
You started with the hypothesis that this is in fact an elevator. You proved that you were correct. You then hypothesized that the button to summon the elevator was on the left, which was incorrect, so then you hypothesized it was on the right, and you were correct. You hypothesized that pressing the button marked with the up arrow would not only bring an elevator to you, but that it would be an elevator heading in the up direction. You were right.
As this guide explains, the deliberate process of testing hypotheses and reaching conclusions is an extension of commonplace testing of cause and effect relationships.
Basic Concepts of Experimental and Quasi-Experimental Research
Discovering causal relationships is the key to experimental research. In abstract terms, this means the relationship between a certain action, X, which alone creates the effect Y. For example, turning the volume knob on your stereo clockwise causes the sound to get louder. In addition, you could observe that turning the knob clockwise alone, and nothing else, caused the sound level to increase. You could further conclude that a causal relationship exists between turning the knob clockwise and an increase in volume; not simply because one caused the other, but because you are certain that nothing else caused the effect.
Independent and Dependent Variables
Beyond discovering causal relationships, experimental research further seeks out how much cause will produce how much effect; in technical terms, how the independent variable will affect the dependent variable. You know that turning the knob clockwise will produce a louder noise, but by varying how much you turn it, you see how much sound is produced. On the other hand, you might find that although you turn the knob a great deal, sound doesn't increase dramatically. Or, you might find that turning the knob just a little adds more sound than expected. The amount that you turned the knob is the independent variable, the variable that the researcher controls, and the amount of sound that resulted from turning it is the dependent variable, the change that is caused by the independent variable.
Experimental research also looks into the effects of removing something. For example, if you remove a loud noise from the room, will the person next to you be able to hear you? Or how much noise needs to be removed before that person can hear you?
Treatment and Hypothesis
The term treatment refers to either removing or adding a stimulus in order to measure an effect (such as turning the knob a little or a lot, or reducing the noise level a little or a lot). Experimental researchers want to know how varying levels of treatment will affect what they are studying. As such, researchers often have an idea, or hypothesis, about what effect will occur when they cause something. Few experiments are performed where there is no idea of what will happen. From past experiences in life or from the knowledge we possess in our specific field of study, we know how some actions cause other reactions. Experiments confirm or reconfirm this fact.
Experimentation becomes more complex when the causal relationships they seek aren't as clear as in the stereo knob-turning examples. Questions like "Will olestra cause cancer?" or "Will this new fertilizer help this plant grow better?" present more to consider. For example, any number of things could affect the growth rate of a plant-the temperature, how much water or sun it receives, or how much carbon dioxide is in the air. These variables can affect an experiment's results. An experimenter who wants to show that adding a certain fertilizer will help a plant grow better must ensure that it is the fertilizer, and nothing else, affecting the growth patterns of the plant. To do this, as many of these variables as possible must be controlled.
Matching and Randomization
In the example used in this guide (you'll find the example below), we discuss an experiment that focuses on three groups of plants -- one that is treated with a fertilizer named MegaGro, another group treated with a fertilizer named Plant!, and yet another that is not treated with fetilizer (this latter group serves as a "control" group). In this example, even though the designers of the experiment have tried to remove all extraneous variables, results may appear merely coincidental. Since the goal of the experiment is to prove a causal relationship in which a single variable is responsible for the effect produced, the experiment would produce stronger proof if the results were replicated in larger treatment and control groups.
Selecting groups entails assigning subjects in the groups of an experiment in such a way that treatment and control groups are comparable in all respects except the application of the treatment. Groups can be created in two ways: matching and randomization. In the MegaGro experiment discussed below, the plants might be matched according to characteristics such as age, weight and whether they are blooming. This involves distributing these plants so that each plant in one group exactly matches characteristics of plants in the other groups. Matching may be problematic, though, because it "can promote a false sense of security by leading [the experimenter] to believe that [the] experimental and control groups were really equated at the outset, when in fact they were not equated on a host of variables" (Jones, 291). In other words, you may have flowers for your MegaGro experiment that you matched and distributed among groups, but other variables are unaccounted for. It would be difficult to have equal groupings.
Randomization, then, is preferred to matching. This method is based on the statistical principle of normal distribution. Theoretically, any arbitrarily selected group of adequate size will reflect normal distribution. Differences between groups will average out and become more comparable. The principle of normal distribution states that in a population most individuals will fall within the middle range of values for a given characteristic, with increasingly fewer toward either extreme (graphically represented as the ubiquitous "bell curve").
Differences between Quasi-Experimental and Experimental Research
Thus far, we have explained that for experimental research we need:
- a hypothesis for a causal relationship;
- a control group and a treatment group;
- to eliminate confounding variables that might mess up the experiment and prevent displaying the causal relationship; and
- to have larger groups with a carefully sorted constituency; preferably randomized, in order to keep accidental differences from fouling things up.
But what if we don't have all of those? Do we still have an experiment? Not a true experiment in the strictest scientific sense of the term, but we can have a quasi-experiment, an attempt to uncover a causal relationship, even though the researcher cannot control all the factors that might affect the outcome.
A quasi-experimenter treats a given situation as an experiment even though it is not wholly by design. The independent variable may not be manipulated by the researcher, treatment and control groups may not be randomized or matched, or there may be no control group. The researcher is limited in what he or she can say conclusively.
The significant element of both experiments and quasi-experiments is the measure of the dependent variable, which it allows for comparison. Some data is quite straightforward, but other measures, such as level of self-confidence in writing ability, increase in creativity or in reading comprehension are inescapably subjective. In such cases, quasi-experimentation often involves a number of strategies to compare subjectivity, such as rating data, testing, surveying, and content analysis.
Rating essentially is developing a rating scale to evaluate data. In testing, experimenters and quasi-experimenters use ANOVA (Analysis of Variance) and ANCOVA (Analysis of Co-Variance) tests to measure differences between control and experimental groups, as well as different correlations between groups.
Since we're mentioning the subject of statistics, note that experimental or quasi-experimental research cannot state beyond a shadow of a doubt that a single cause will always produce any one effect. They can do no more than show a probability that one thing causes another. The probability that a result is the due to random chance is an important measure of statistical analysis and in experimental research.
Example: Causality
Let's say you want to determine that your new fertilizer, MegaGro, will increase the growth rate of plants. You begin by getting a plant to go with your fertilizer. Since the experiment is concerned with proving that MegaGro works, you need another plant, using no fertilizer at all on it, to compare how much change your fertilized plant displays. This is what is known as a control group.
Set up with a control group, which will receive no treatment, and an experimental group, which will get MegaGro, you must then address those variables that could invalidate your experiment. This can be an extensive and exhaustive process. You must ensure that you use the same plant; that both groups are put in the same kind of soil; that they receive equal amounts of water and sun; that they receive the same amount of exposure to carbon-dioxide-exhaling researchers, and so on. In short, any other variable that might affect the growth of those plants, other than the fertilizer, must be the same for both plants. Otherwise, you can't prove absolutely that MegaGro is the only explanation for the increased growth of one of those plants.
Such an experiment can be done on more than two groups. You may not only want to show that MegaGro is an effective fertilizer, but that it is better than its competitor brand of fertilizer, Plant! All you need to do, then, is have one experimental group receiving MegaGro, one receiving Plant! and the other (the control group) receiving no fertilizer. Those are the only variables that can be different between the three groups; all other variables must be the same for the experiment to be valid.
Controlling variables allows the researcher to identify conditions that may affect the experiment's outcome. This may lead to alternative explanations that the researcher is willing to entertain in order to isolate only variables judged significant. In the MegaGro experiment, you may be concerned with how fertile the soil is, but not with the plants'; relative position in the window, as you don't think that the amount of shade they get will affect their growth rate. But what if it did? You would have to go about eliminating variables in order to determine which is the key factor. What if one receives more shade than the other and the MegaGro plant, which received more shade, died? This might prompt you to formulate a plausible alternative explanation, which is a way of accounting for a result that differs from what you expected. You would then want to redo the study with equal amounts of sunlight.
Methods: Five Steps
Experimental research can be roughly divided into five phases:
Identifying a research problem
The process starts by clearly identifying the problem you want to study and considering what possible methods will affect a solution. Then you choose the method you want to test, and formulate a hypothesis to predict the outcome of the test.
For example, you may want to improve student essays, but you don't believe that teacher feedback is enough. You hypothesize that some possible methods for writing improvement include peer workshopping, or reading more example essays. Favoring the former, your experiment would try to determine if peer workshopping improves writing in high school seniors. You state your hypothesis: peer workshopping prior to turning in a final draft will improve the quality of the student's essay.
Planning an experimental research study
The next step is to devise an experiment to test your hypothesis. In doing so, you must consider several factors. For example, how generalizable do you want your end results to be? Do you want to generalize about the entire population of high school seniors everywhere, or just the particular population of seniors at your specific school? This will determine how simple or complex the experiment will be. The amount of time funding you have will also determine the size of your experiment.
Continuing the example from step one, you may want a small study at one school involving three teachers, each teaching two sections of the same course. The treatment in this experiment is peer workshopping. Each of the three teachers will assign the same essay assignment to both classes; the treatment group will participate in peer workshopping, while the control group will receive only teacher comments on their drafts.
Conducting the experiment
At the start of an experiment, the control and treatment groups must be selected. Whereas the "hard" sciences have the luxury of attempting to create truly equal groups, educators often find themselves forced to conduct their experiments based on self-selected groups, rather than on randomization. As was highlighted in the Basic Concepts section, this makes the study a quasi-experiment, since the researchers cannot control all of the variables.
For the peer workshopping experiment, let's say that it involves six classes and three teachers with a sample of students randomly selected from all the classes. Each teacher will have a class for a control group and a class for a treatment group. The essay assignment is given and the teachers are briefed not to change any of their teaching methods other than the use of peer workshopping. You may see here that this is an effort to control a possible variable: teaching style variance.
Analyzing the data
The fourth step is to collect and analyze the data. This is not solely a step where you collect the papers, read them, and say your methods were a success. You must show how successful. You must devise a scale by which you will evaluate the data you receive, therefore you must decide what indicators will be, and will not be, important.
Continuing our example, the teachers' grades are first recorded, then the essays are evaluated for a change in sentence complexity, syntactical and grammatical errors, and overall length. Any statistical analysis is done at this time if you choose to do any. Notice here that the researcher has made judgments on what signals improved writing. It is not simply a matter of improved teacher grades, but a matter of what the researcher believes constitutes improved use of the language.
Writing the paper/presentation describing the findings
Once you have completed the experiment, you will want to share findings by publishing academic paper (or presentations). These papers usually have the following format, but it is not necessary to follow it strictly. Sections can be combined or not included, depending on the structure of the experiment, and the journal to which you submit your paper.
- Abstract : Summarize the project: its aims, participants, basic methodology, results, and a brief interpretation.
- Introduction : Set the context of the experiment.
- Review of Literature : Provide a review of the literature in the specific area of study to show what work has been done. Should lead directly to the author's purpose for the study.
- Statement of Purpose : Present the problem to be studied.
- Participants : Describe in detail participants involved in the study; e.g., how many, etc. Provide as much information as possible.
- Materials and Procedures : Clearly describe materials and procedures. Provide enough information so that the experiment can be replicated, but not so much information that it becomes unreadable. Include how participants were chosen, the tasks assigned them, how they were conducted, how data were evaluated, etc.
- Results : Present the data in an organized fashion. If it is quantifiable, it is analyzed through statistical means. Avoid interpretation at this time.
- Discussion : After presenting the results, interpret what has happened in the experiment. Base the discussion only on the data collected and as objective an interpretation as possible. Hypothesizing is possible here.
- Limitations : Discuss factors that affect the results. Here, you can speculate how much generalization, or more likely, transferability, is possible based on results. This section is important for quasi-experimentation, since a quasi-experiment cannot control all of the variables that might affect the outcome of a study. You would discuss what variables you could not control.
- Conclusion : Synthesize all of the above sections.
- References : Document works cited in the correct format for the field.
Experimental and Quasi-Experimental Research: Issues and Commentary
Several issues are addressed in this section, including the use of experimental and quasi-experimental research in educational settings, the relevance of the methods to English studies, and ethical concerns regarding the methods.
Using Experimental and Quasi-Experimental Research in Educational Settings
Charting causal relationships in human settings.
Any time a human population is involved, prediction of casual relationships becomes cloudy and, some say, impossible. Many reasons exist for this; for example,
- researchers in classrooms add a disturbing presence, causing students to act abnormally, consciously or unconsciously;
- subjects try to please the researcher, just because of an apparent interest in them (known as the Hawthorne Effect); or, perhaps
- the teacher as researcher is restricted by bias and time pressures.
But such confounding variables don't stop researchers from trying to identify causal relationships in education. Educators naturally experiment anyway, comparing groups, assessing the attributes of each, and making predictions based on an evaluation of alternatives. They look to research to support their intuitive practices, experimenting whenever they try to decide which instruction method will best encourage student improvement.
Combining Theory, Research, and Practice
The goal of educational research lies in combining theory, research, and practice. Educational researchers attempt to establish models of teaching practice, learning styles, curriculum development, and countless other educational issues. The aim is to "try to improve our understanding of education and to strive to find ways to have understanding contribute to the improvement of practice," one writer asserts (Floden 1996, p. 197).
In quasi-experimentation, researchers try to develop models by involving teachers as researchers, employing observational research techniques. Although results of this kind of research are context-dependent and difficult to generalize, they can act as a starting point for further study. The "educational researcher . . . provides guidelines and interpretive material intended to liberate the teacher's intelligence so that whatever artistry in teaching the teacher can achieve will be employed" (Eisner 1992, p. 8).
Bias and Rigor
Critics contend that the educational researcher is inherently biased, sample selection is arbitrary, and replication is impossible. The key to combating such criticism has to do with rigor. Rigor is established through close, proper attention to randomizing groups, time spent on a study, and questioning techniques. This allows more effective application of standards of quantitative research to qualitative research.
Often, teachers cannot wait to for piles of experimentation data to be analyzed before using the teaching methods (Lauer and Asher 1988). They ultimately must assess whether the results of a study in a distant classroom are applicable in their own classrooms. And they must continuously test the effectiveness of their methods by using experimental and qualitative research simultaneously. In addition to statistics (quantitative), researchers may perform case studies or observational research (qualitative) in conjunction with, or prior to, experimentation.
Relevance to English Studies
Situations in english studies that might encourage use of experimental methods.
Whenever a researcher would like to see if a causal relationship exists between groups, experimental and quasi-experimental research can be a viable research tool. Researchers in English Studies might use experimentation when they believe a relationship exists between two variables, and they want to show that these two variables have a significant correlation (or causal relationship).
A benefit of experimentation is the ability to control variables, such as the amount of treatment, when it is given, to whom and so forth. Controlling variables allows researchers to gain insight into the relationships they believe exist. For example, a researcher has an idea that writing under pseudonyms encourages student participation in newsgroups. Researchers can control which students write under pseudonyms and which do not, then measure the outcomes. Researchers can then analyze results and determine if this particular variable alone causes increased participation.
Transferability-Applying Results
Experimentation and quasi-experimentation allow for generating transferable results and accepting those results as being dependent upon experimental rigor. It is an effective alternative to generalizability, which is difficult to rely upon in educational research. English scholars, reading results of experiments with a critical eye, ultimately decide if results will be implemented and how. They may even extend that existing research by replicating experiments in the interest of generating new results and benefiting from multiple perspectives. These results will strengthen the study or discredit findings.
Concerns English Scholars Express about Experiments
Researchers should carefully consider if a particular method is feasible in humanities studies, and whether it will yield the desired information. Some researchers recommend addressing pertinent issues combining several research methods, such as survey, interview, ethnography, case study, content analysis, and experimentation (Lauer and Asher, 1988).
Advantages and Disadvantages of Experimental Research: Discussion
In educational research, experimentation is a way to gain insight into methods of instruction. Although teaching is context specific, results can provide a starting point for further study. Often, a teacher/researcher will have a "gut" feeling about an issue which can be explored through experimentation and looking at causal relationships. Through research intuition can shape practice .
A preconception exists that information obtained through scientific method is free of human inconsistencies. But, since scientific method is a matter of human construction, it is subject to human error . The researcher's personal bias may intrude upon the experiment , as well. For example, certain preconceptions may dictate the course of the research and affect the behavior of the subjects. The issue may be compounded when, although many researchers are aware of the affect that their personal bias exerts on their own research, they are pressured to produce research that is accepted in their field of study as "legitimate" experimental research.
The researcher does bring bias to experimentation, but bias does not limit an ability to be reflective . An ethical researcher thinks critically about results and reports those results after careful reflection. Concerns over bias can be leveled against any research method.
Often, the sample may not be representative of a population, because the researcher does not have an opportunity to ensure a representative sample. For example, subjects could be limited to one location, limited in number, studied under constrained conditions and for too short a time.
Despite such inconsistencies in educational research, the researcher has control over the variables , increasing the possibility of more precisely determining individual effects of each variable. Also, determining interaction between variables is more possible.
Even so, artificial results may result . It can be argued that variables are manipulated so the experiment measures what researchers want to examine; therefore, the results are merely contrived products and have no bearing in material reality. Artificial results are difficult to apply in practical situations, making generalizing from the results of a controlled study questionable. Experimental research essentially first decontextualizes a single question from a "real world" scenario, studies it under controlled conditions, and then tries to recontextualize the results back on the "real world" scenario. Results may be difficult to replicate .
Perhaps, groups in an experiment may not be comparable . Quasi-experimentation in educational research is widespread because not only are many researchers also teachers, but many subjects are also students. With the classroom as laboratory, it is difficult to implement randomizing or matching strategies. Often, students self-select into certain sections of a course on the basis of their own agendas and scheduling needs. Thus when, as often happens, one class is treated and the other used for a control, the groups may not actually be comparable. As one might imagine, people who register for a class which meets three times a week at eleven o'clock in the morning (young, no full-time job, night people) differ significantly from those who register for one on Monday evenings from seven to ten p.m. (older, full-time job, possibly more highly motivated). Each situation presents different variables and your group might be completely different from that in the study. Long-term studies are expensive and hard to reproduce. And although often the same hypotheses are tested by different researchers, various factors complicate attempts to compare or synthesize them. It is nearly impossible to be as rigorous as the natural sciences model dictates.
Even when randomization of students is possible, problems arise. First, depending on the class size and the number of classes, the sample may be too small for the extraneous variables to cancel out. Second, the study population is not strictly a sample, because the population of students registered for a given class at a particular university is obviously not representative of the population of all students at large. For example, students at a suburban private liberal-arts college are typically young, white, and upper-middle class. In contrast, students at an urban community college tend to be older, poorer, and members of a racial minority. The differences can be construed as confounding variables: the first group may have fewer demands on its time, have less self-discipline, and benefit from superior secondary education. The second may have more demands, including a job and/or children, have more self-discipline, but an inferior secondary education. Selecting a population of subjects which is representative of the average of all post-secondary students is also a flawed solution, because the outcome of a treatment involving this group is not necessarily transferable to either the students at a community college or the students at the private college, nor are they universally generalizable.
When a human population is involved, experimental research becomes concerned if behavior can be predicted or studied with validity. Human response can be difficult to measure . Human behavior is dependent on individual responses. Rationalizing behavior through experimentation does not account for the process of thought, making outcomes of that process fallible (Eisenberg, 1996).
Nevertheless, we perform experiments daily anyway . When we brush our teeth every morning, we are experimenting to see if this behavior will result in fewer cavities. We are relying on previous experimentation and we are transferring the experimentation to our daily lives.
Moreover, experimentation can be combined with other research methods to ensure rigor . Other qualitative methods such as case study, ethnography, observational research and interviews can function as preconditions for experimentation or conducted simultaneously to add validity to a study.
We have few alternatives to experimentation. Mere anecdotal research , for example is unscientific, unreplicatable, and easily manipulated. Should we rely on Ed walking into a faculty meeting and telling the story of Sally? Sally screamed, "I love writing!" ten times before she wrote her essay and produced a quality paper. Therefore, all the other faculty members should hear this anecdote and know that all other students should employ this similar technique.
On final disadvantage: frequently, political pressure drives experimentation and forces unreliable results. Specific funding and support may drive the outcomes of experimentation and cause the results to be skewed. The reader of these results may not be aware of these biases and should approach experimentation with a critical eye.
Advantages and Disadvantages of Experimental Research: Quick Reference List
Experimental and quasi-experimental research can be summarized in terms of their advantages and disadvantages. This section combines and elaborates upon many points mentioned previously in this guide.
|
|
gain insight into methods of instruction | subject to human error |
intuitive practice shaped by research | personal bias of researcher may intrude |
teachers have bias but can be reflective | sample may not be representative |
researcher can have control over variables | can produce artificial results |
humans perform experiments anyway | results may only apply to one situation and may be difficult to replicate |
can be combined with other research methods for rigor | groups may not be comparable |
use to determine what is best for population | human response can be difficult to measure |
provides for greater transferability than anecdotal research | political pressure may skew results |
Ethical Concerns
Experimental research may be manipulated on both ends of the spectrum: by researcher and by reader. Researchers who report on experimental research, faced with naive readers of experimental research, encounter ethical concerns. While they are creating an experiment, certain objectives and intended uses of the results might drive and skew it. Looking for specific results, they may ask questions and look at data that support only desired conclusions. Conflicting research findings are ignored as a result. Similarly, researchers, seeking support for a particular plan, look only at findings which support that goal, dismissing conflicting research.
Editors and journals do not publish only trouble-free material. As readers of experiments members of the press might report selected and isolated parts of a study to the public, essentially transferring that data to the general population which may not have been intended by the researcher. Take, for example, oat bran. A few years ago, the press reported how oat bran reduces high blood pressure by reducing cholesterol. But that bit of information was taken out of context. The actual study found that when people ate more oat bran, they reduced their intake of saturated fats high in cholesterol. People started eating oat bran muffins by the ton, assuming a causal relationship when in actuality a number of confounding variables might influence the causal link.
Ultimately, ethical use and reportage of experimentation should be addressed by researchers, reporters and readers alike.
Reporters of experimental research often seek to recognize their audience's level of knowledge and try not to mislead readers. And readers must rely on the author's skill and integrity to point out errors and limitations. The relationship between researcher and reader may not sound like a problem, but after spending months or years on a project to produce no significant results, it may be tempting to manipulate the data to show significant results in order to jockey for grants and tenure.
Meanwhile, the reader may uncritically accept results that receive validity by being published in a journal. However, research that lacks credibility often is not published; consequentially, researchers who fail to publish run the risk of being denied grants, promotions, jobs, and tenure. While few researchers are anything but earnest in their attempts to conduct well-designed experiments and present the results in good faith, rhetorical considerations often dictate a certain minimization of methodological flaws.
Concerns arise if researchers do not report all, or otherwise alter, results. This phenomenon is counterbalanced, however, in that professionals are also rewarded for publishing critiques of others' work. Because the author of an experimental study is in essence making an argument for the existence of a causal relationship, he or she must be concerned not only with its integrity, but also with its presentation. Achieving persuasiveness in any kind of writing involves several elements: choosing a topic of interest, providing convincing evidence for one's argument, using tone and voice to project credibility, and organizing the material in a way that meets expectations for a logical sequence. Of course, what is regarded as pertinent, accepted as evidence, required for credibility, and understood as logical varies according to context. If the experimental researcher hopes to make an impact on the community of professionals in their field, she must attend to the standards and orthodoxy's of that audience.
Related Links
Contrasts: Traditional and computer-supported writing classrooms. This Web presents a discussion of the Transitions Study, a year-long exploration of teachers and students in computer-supported and traditional writing classrooms. Includes description of study, rationale for conducting the study, results and implications of the study.
http://kairos.technorhetoric.net/2.2/features/reflections/page1.htm
Annotated Bibliography
A cozy world of trivial pursuits? (1996, June 28) The Times Educational Supplement . 4174, pp. 14-15.
A critique discounting the current methods Great Britain employs to fund and disseminate educational research. The belief is that research is performed for fellow researchers not the teaching public and implications for day to day practice are never addressed.
Anderson, J. A. (1979, Nov. 10-13). Research as argument: the experimental form. Paper presented at the annual meeting of the Speech Communication Association, San Antonio, TX.
In this paper, the scientist who uses the experimental form does so in order to explain that which is verified through prediction.
Anderson, Linda M. (1979). Classroom-based experimental studies of teaching effectiveness in elementary schools . (Technical Report UTR&D-R- 4102). Austin: Research and Development Center for Teacher Education, University of Texas.
Three recent large-scale experimental studies have built on a database established through several correlational studies of teaching effectiveness in elementary school.
Asher, J. W. (1976). Educational research and evaluation methods . Boston: Little, Brown.
Abstract unavailable by press time.
Babbie, Earl R. (1979). The Practice of Social Research . Belmont, CA: Wadsworth.
A textbook containing discussions of several research methodologies used in social science research.
Bangert-Drowns, R.L. (1993). The word processor as instructional tool: a meta-analysis of word processing in writing instruction. Review of Educational Research, 63 (1), 69-93.
Beach, R. (1993). The effects of between-draft teacher evaluation versus student self-evaluation on high school students' revising of rough drafts. Research in the Teaching of English, 13 , 111-119.
The question of whether teacher evaluation or guided self-evaluation of rough drafts results in increased revision was addressed in Beach's study. Differences in the effects of teacher evaluations, guided self-evaluation (using prepared guidelines,) and no evaluation of rough drafts were examined. The final drafts of students (10th, 11th, and 12th graders) were compared with their rough drafts and rated by judges according to degree of change.
Beishuizen, J. & Moonen, J. (1992). Research in technology enriched schools: a case for cooperation between teachers and researchers . (ERIC Technical Report ED351006).
This paper describes the research strategies employed in the Dutch Technology Enriched Schools project to encourage extensive and intensive use of computers in a small number of secondary schools, and to study the effects of computer use on the classroom, the curriculum, and school administration and management.
Borg, W. P. (1989). Educational Research: an Introduction . (5th ed.). New York: Longman.
An overview of educational research methodology, including literature review and discussion of approaches to research, experimental design, statistical analysis, ethics, and rhetorical presentation of research findings.
Campbell, D. T., & Stanley, J. C. (1963). Experimental and quasi-experimental designs for research . Boston: Houghton Mifflin.
A classic overview of research designs.
Campbell, D.T. (1988). Methodology and epistemology for social science: selected papers . ed. E. S. Overman. Chicago: University of Chicago Press.
This is an overview of Campbell's 40-year career and his work. It covers in seven parts measurement, experimental design, applied social experimentation, interpretive social science, epistemology and sociology of science. Includes an extensive bibliography.
Caporaso, J. A., & Roos, Jr., L. L. (Eds.). Quasi-experimental approaches: Testing theory and evaluating policy. Evanston, WA: Northwestern University Press.
A collection of articles concerned with explicating the underlying assumptions of quasi-experimentation and relating these to true experimentation. With an emphasis on design. Includes a glossary of terms.
Collier, R. Writing and the word processor: How wary of the gift-giver should we be? Unpublished manuscript.
Unpublished typescript. Charts the developments to date in computers and composition and speculates about the future within the framework of Willie Sypher's model of the evolution of creative discovery.
Cook, T.D. & Campbell, D.T. (1979). Quasi-experimentation: design and analysis issues for field settings . Boston: Houghton Mifflin Co.
The authors write that this book "presents some quasi-experimental designs and design features that can be used in many social research settings. The designs serve to probe causal hypotheses about a wide variety of substantive issues in both basic and applied research."
Cutler, A. (1970). An experimental method for semantic field study. Linguistic Communication, 2 , N. pag.
This paper emphasizes the need for empirical research and objective discovery procedures in semantics, and illustrates a method by which these goals may be obtained.
Daniels, L. B. (1996, Summer). Eisenberg's Heisenberg: The indeterminancies of rationality. Curriculum Inquiry, 26 , 181-92.
Places Eisenberg's theories in relation to the death of foundationalism by showing that he distorts rational studies into a form of relativism. He looks at Eisenberg's ideas on indeterminacy, methods and evidence, what he is against and what we should think of what he says.
Danziger, K. (1990). Constructing the subject: Historical origins of psychological research. Cambridge: Cambridge University Press.
Danzinger stresses the importance of being aware of the framework in which research operates and of the essentially social nature of scientific activity.
Diener, E., et al. (1972, December). Leakage of experimental information to potential future subjects by debriefed subjects. Journal of Experimental Research in Personality , 264-67.
Research regarding research: an investigation of the effects on the outcome of an experiment in which information about the experiment had been leaked to subjects. The study concludes that such leakage is not a significant problem.
Dudley-Marling, C., & Rhodes, L. K. (1989). Reflecting on a close encounter with experimental research. Canadian Journal of English Language Arts. 12 , 24-28.
Researchers, Dudley-Marling and Rhodes, address some problems they met in their experimental approach to a study of reading comprehension. This article discusses the limitations of experimental research, and presents an alternative to experimental or quantitative research.
Edgington, E. S. (1985). Random assignment and experimental research. Educational Administration Quarterly, 21 , N. pag.
Edgington explores ways on which random assignment can be a part of field studies. The author discusses both non-experimental and experimental research and the need for using random assignment.
Eisenberg, J. (1996, Summer). Response to critiques by R. Floden, J. Zeuli, and L. Daniels. Curriculum Inquiry, 26 , 199-201.
A response to critiques of his argument that rational educational research methods are at best suspect and at worst futile. He believes indeterminacy controls this method and worries that chaotic research is failing students.
Eisner, E. (1992, July). Are all causal claims positivistic? A reply to Francis Schrag. Educational Researcher, 21 (5), 8-9.
Eisner responds to Schrag who claimed that critics like Eisner cannot escape a positivistic paradigm whatever attempts they make to do so. Eisner argues that Schrag essentially misses the point for trying to argue for the paradigm solely on the basis of cause and effect without including the rest of positivistic philosophy. This weakens his argument against multiple modal methods, which Eisner argues provides opportunities to apply the appropriate research design where it is most applicable.
Floden, R.E. (1996, Summer). Educational research: limited, but worthwhile and maybe a bargain. (response to J.A. Eisenberg). Curriculum Inquiry, 26 , 193-7.
Responds to John Eisenberg critique of educational research by asserting the connection between improvement of practice and research results. He places high value of teacher discrepancy and knowledge that research informs practice.
Fortune, J. C., & Hutson, B. A. (1994, March/April). Selecting models for measuring change when true experimental conditions do not exist. Journal of Educational Research, 197-206.
This article reviews methods for minimizing the effects of nonideal experimental conditions by optimally organizing models for the measurement of change.
Fox, R. F. (1980). Treatment of writing apprehension and tts effects on composition. Research in the Teaching of English, 14 , 39-49.
The main purpose of Fox's study was to investigate the effects of two methods of teaching writing on writing apprehension among entry level composition students, A conventional teaching procedure was used with a control group, while a workshop method was employed with the treatment group.
Gadamer, H-G. (1976). Philosophical hermeneutics . (D. E. Linge, Trans.). Berkeley, CA: University of California Press.
A collection of essays with the common themes of the mediation of experience through language, the impossibility of objectivity, and the importance of context in interpretation.
Gaise, S. J. (1981). Experimental vs. non-experimental research on classroom second language learning. Bilingual Education Paper Series, 5 , N. pag.
Aims on classroom-centered research on second language learning and teaching are considered and contrasted with the experimental approach.
Giordano, G. (1983). Commentary: Is experimental research snowing us? Journal of Reading, 27 , 5-7.
Do educational research findings actually benefit teachers and students? Giordano states his opinion that research may be helpful to teaching, but is not essential and often is unnecessary.
Goldenson, D. R. (1978, March). An alternative view about the role of the secondary school in political socialization: A field-experimental study of theory and research in social education. Theory and Research in Social Education , 44-72.
This study concludes that when political discussion among experimental groups of secondary school students is led by a teacher, the degree to which the students' views were impacted is proportional to the credibility of the teacher.
Grossman, J., and J. P. Tierney. (1993, October). The fallibility of comparison groups. Evaluation Review , 556-71.
Grossman and Tierney present evidence to suggest that comparison groups are not the same as nontreatment groups.
Harnisch, D. L. (1992). Human judgment and the logic of evidence: A critical examination of research methods in special education transition literature. In D. L. Harnisch et al. (Eds.), Selected readings in transition.
This chapter describes several common types of research studies in special education transition literature and the threats to their validity.
Hawisher, G. E. (1989). Research and recommendations for computers and composition. In G. Hawisher and C. Selfe. (Eds.), Critical Perspectives on Computers and Composition Instruction . (pp. 44-69). New York: Teacher's College Press.
An overview of research in computers and composition to date. Includes a synthesis grid of experimental research.
Hillocks, G. Jr. (1982). The interaction of instruction, teacher comment, and revision in teaching the composing process. Research in the Teaching of English, 16 , 261-278.
Hillock conducted a study using three treatments: observational or data collecting activities prior to writing, use of revisions or absence of same, and either brief or lengthy teacher comments to identify effective methods of teaching composition to seventh and eighth graders.
Jenkinson, J. C. (1989). Research design in the experimental study of intellectual disability. International Journal of Disability, Development, and Education, 69-84.
This article catalogues the difficulties of conducting experimental research where the subjects are intellectually disables and suggests alternative research strategies.
Jones, R. A. (1985). Research Methods in the Social and Behavioral Sciences. Sunderland, MA: Sinauer Associates, Inc..
A textbook designed to provide an overview of research strategies in the social sciences, including survey, content analysis, ethnographic approaches, and experimentation. The author emphasizes the importance of applying strategies appropriately and in variety.
Kamil, M. L., Langer, J. A., & Shanahan, T. (1985). Understanding research in reading and writing . Newton, Massachusetts: Allyn and Bacon.
Examines a wide variety of problems in reading and writing, with a broad range of techniques, from different perspectives.
Kennedy, J. L. (1985). An Introduction to the Design and Analysis of Experiments in Behavioral Research . Lanham, MD: University Press of America.
An introductory textbook of psychological and educational research.
Keppel, G. (1991). Design and analysis: a researcher's handbook . Englewood Cliffs, NJ: Prentice Hall.
This updates Keppel's earlier book subtitled "a student's handbook." Focuses on extensive information about analytical research and gives a basic picture of research in psychology. Covers a range of statistical topics. Includes a subject and name index, as well as a glossary.
Knowles, G., Elija, R., & Broadwater, K. (1996, Spring/Summer). Teacher research: enhancing the preparation of teachers? Teaching Education, 8 , 123-31.
Researchers looked at one teacher candidate who participated in a class which designed their own research project correlating to a question they would like answered in the teaching world. The goal of the study was to see if preservice teachers developed reflective practice by researching appropriate classroom contexts.
Lace, J., & De Corte, E. (1986, April 16-20). Research on media in western Europe: A myth of sisyphus? Paper presented at the annual meeting of the American Educational Research Association. San Francisco.
Identifies main trends in media research in western Europe, with emphasis on three successive stages since 1960: tools technology, systems technology, and reflective technology.
Latta, A. (1996, Spring/Summer). Teacher as researcher: selected resources. Teaching Education, 8 , 155-60.
An annotated bibliography on educational research including milestones of thought, practical applications, successful outcomes, seminal works, and immediate practical applications.
Lauer. J.M. & Asher, J. W. (1988). Composition research: Empirical designs . New York: Oxford University Press.
Approaching experimentation from a humanist's perspective to it, authors focus on eight major research designs: Case studies, ethnographies, sampling and surveys, quantitative descriptive studies, measurement, true experiments, quasi-experiments, meta-analyses, and program evaluations. It takes on the challenge of bridging language of social science with that of the humanist. Includes name and subject indexes, as well as a glossary and a glossary of symbols.
Mishler, E. G. (1979). Meaning in context: Is there any other kind? Harvard Educational Review, 49 , 1-19.
Contextual importance has been largely ignored by traditional research approaches in social/behavioral sciences and in their application to the education field. Developmental and social psychologists have increasingly noted the inadequacies of this approach. Drawing examples for phenomenology, sociolinguistics, and ethnomethodology, the author proposes alternative approaches for studying meaning in context.
Mitroff, I., & Bonoma, T. V. (1978, May). Psychological assumptions, experimentations, and real world problems: A critique and an alternate approach to evaluation. Evaluation Quarterly , 235-60.
The authors advance the notion of dialectic as a means to clarify and examine the underlying assumptions of experimental research methodology, both in highly controlled situations and in social evaluation.
Muller, E. W. (1985). Application of experimental and quasi-experimental research designs to educational software evaluation. Educational Technology, 25 , 27-31.
Muller proposes a set of guidelines for the use of experimental and quasi-experimental methods of research in evaluating educational software. By obtaining empirical evidence of student performance, it is possible to evaluate if programs are making the desired learning effect.
Murray, S., et al. (1979, April 8-12). Technical issues as threats to internal validity of experimental and quasi-experimental designs . San Francisco: University of California.
The article reviews three evaluation models and analyzes the flaws common to them. Remedies are suggested.
Muter, P., & Maurutto, P. (1991). Reading and skimming from computer screens and books: The paperless office revisited? Behavior and Information Technology, 10 (4), 257-66.
The researchers test for reading and skimming effectiveness, defined as accuracy combined with speed, for written text compared to text on a computer monitor. They conclude that, given optimal on-line conditions, both are equally effective.
O'Donnell, A., Et al. (1992). The impact of cooperative writing. In J. R. Hayes, et al. (Eds.). Reading empirical research studies: The rhetoric of research . (pp. 371-84). Hillsdale, NJ: Lawrence Erlbaum Associates.
A model of experimental design. The authors investigate the efficacy of cooperative writing strategies, as well as the transferability of skills learned to other, individual writing situations.
Palmer, D. (1988). Looking at philosophy . Mountain View, CA: Mayfield Publishing.
An introductory text with incisive but understandable discussions of the major movements and thinkers in philosophy from the Pre-Socratics through Sartre. With illustrations by the author. Includes a glossary.
Phelps-Gunn, T., & Phelps-Terasaki, D. (1982). Written language instruction: Theory and remediation . London: Aspen Systems Corporation.
The lack of research in written expression is addressed and an application on the Total Writing Process Model is presented.
Poetter, T. (1996, Spring/Summer). From resistance to excitement: becoming qualitative researchers and reflective practitioners. Teaching Education , 8109-19.
An education professor reveals his own problematic research when he attempted to institute a educational research component to a teacher preparation program. He encountered dissent from students and cooperating professionals and ultimately was rewarded with excitement towards research and a recognized correlation to practice.
Purves, A. C. (1992). Reflections on research and assessment in written composition. Research in the Teaching of English, 26 .
Three issues concerning research and assessment is writing are discussed: 1) School writing is a matter of products not process, 2) school writing is an ill-defined domain, 3) the quality of school writing is what observers report they see. Purves discusses these issues while looking at data collected in a ten-year study of achievement in written composition in fourteen countries.
Rathus, S. A. (1987). Psychology . (3rd ed.). Poughkeepsie, NY: Holt, Rinehart, and Winston.
An introductory psychology textbook. Includes overviews of the major movements in psychology, discussions of prominent examples of experimental research, and a basic explanation of relevant physiological factors. With chapter summaries.
Reiser, R. A. (1982). Improving the research skills of instructional designers. Educational Technology, 22 , 19-21.
In his paper, Reiser starts by stating the importance of research in advancing the field of education, and points out that graduate students in instructional design lack the proper skills to conduct research. The paper then goes on to outline the practicum in the Instructional Systems Program at Florida State University which includes: 1) Planning and conducting an experimental research study; 2) writing the manuscript describing the study; 3) giving an oral presentation in which they describe their research findings.
Report on education research . (Journal). Washington, DC: Capitol Publication, Education News Services Division.
This is an independent bi-weekly newsletter on research in education and learning. It has been publishing since Sept. 1969.
Rossell, C. H. (1986). Why is bilingual education research so bad?: Critique of the Walsh and Carballo study of Massachusetts bilingual education programs . Boston: Center for Applied Social Science, Boston University. (ERIC Working Paper 86-5).
The Walsh and Carballo evaluation of the effectiveness of transitional bilingual education programs in five Massachusetts communities has five flaws and the five flaws are discussed in detail.
Rubin, D. L., & Greene, K. (1992). Gender-typical style in written language. Research in the Teaching of English, 26.
This study was designed to find out whether the writing styles of men and women differ. Rubin and Green discuss the pre-suppositions that women are better writers than men.
Sawin, E. (1992). Reaction: Experimental research in the context of other methods. School of Education Review, 4 , 18-21.
Sawin responds to Gage's article on methodologies and issues in educational research. He agrees with most of the article but suggests the concept of scientific should not be regarded in absolute terms and recommends more emphasis on scientific method. He also questions the value of experiments over other types of research.
Schoonmaker, W. E. (1984). Improving classroom instruction: A model for experimental research. The Technology Teacher, 44, 24-25.
The model outlined in this article tries to bridge the gap between classroom practice and laboratory research, using what Schoonmaker calls active research. Research is conducted in the classroom with the students and is used to determine which two methods of classroom instruction chosen by the teacher is more effective.
Schrag, F. (1992). In defense of positivist research paradigms. Educational Researcher, 21, (5), 5-8.
The controversial defense of the use of positivistic research methods to evaluate educational strategies; the author takes on Eisner, Erickson, and Popkewitz.
Smith, J. (1997). The stories educational researchers tell about themselves. Educational Researcher, 33 (3), 4-11.
Recapitulates main features of an on-going debate between advocates for using vocabularies of traditional language arts and whole language in educational research. An "impasse" exists were advocates "do not share a theoretical disposition concerning both language instruction and the nature of research," Smith writes (p. 6). He includes a very comprehensive history of the debate of traditional research methodology and qualitative methods and vocabularies. Definitely worth a read by graduates.
Smith, N. L. (1980). The feasibility and desirability of experimental methods in evaluation. Evaluation and Program Planning: An International Journal , 251-55.
Smith identifies the conditions under which experimental research is most desirable. Includes a review of current thinking and controversies.
Stewart, N. R., & Johnson, R. G. (1986, March 16-20). An evaluation of experimental methodology in counseling and counselor education research. Paper presented at the annual meeting of the American Educational Research Association, San Francisco.
The purpose of this study was to evaluate the quality of experimental research in counseling and counselor education published from 1976 through 1984.
Spector, P. E. (1990). Research Designs. Newbury Park, California: Sage Publications.
In this book, Spector introduces the basic principles of experimental and nonexperimental design in the social sciences.
Tait, P. E. (1984). Do-it-yourself evaluation of experimental research. Journal of Visual Impairment and Blindness, 78 , 356-363 .
Tait's goal is to provide the reader who is unfamiliar with experimental research or statistics with the basic skills necessary for the evaluation of research studies.
Walsh, S. M. (1990). The current conflict between case study and experimental research: A breakthrough study derives benefits from both . (ERIC Document Number ED339721).
This paper describes a study that was not experimentally designed, but its major findings were generalizable to the overall population of writers in college freshman composition classes. The study was not a case study, but it provided insights into the attitudes and feelings of small clusters of student writers.
Waters, G. R. (1976). Experimental designs in communication research. Journal of Business Communication, 14 .
The paper presents a series of discussions on the general elements of experimental design and the scientific process and relates these elements to the field of communication.
Welch, W. W. (March 1969). The selection of a national random sample of teachers for experimental curriculum evaluation. Scholastic Science and Math , 210-216.
Members of the evaluation section of Harvard project physics describe what is said to be the first attempt to select a national random sample of teachers, and list 6 steps to do so. Cost and comparison with a volunteer group are also discussed.
Winer, B.J. (1971). Statistical principles in experimental design , (2nd ed.). New York: McGraw-Hill.
Combines theory and application discussions to give readers a better understanding of the logic behind statistical aspects of experimental design. Introduces the broad topic of design, then goes into considerable detail. Not for light reading. Bring your aspirin if you like statistics. Bring morphine is you're a humanist.
Winn, B. (1986, January 16-21). Emerging trends in educational technology research. Paper presented at the Annual Convention of the Association for Educational Communication Technology.
This examination of the topic of research in educational technology addresses four major areas: (1) why research is conducted in this area and the characteristics of that research; (2) the types of research questions that should or should not be addressed; (3) the most appropriate methodologies for finding answers to research questions; and (4) the characteristics of a research report that make it good and ultimately suitable for publication.
Citation Information
Luann Barnes, Jennifer Hauser, Luana Heikes, Anthony J. Hernandez, Paul Tim Richard, Katherine Ross, Guo Hua Yang, and Mike Palmquist. (1994-2024). Experimental and Quasi-Experimental Research. The WAC Clearinghouse. Colorado State University. Available at https://wac.colostate.edu/repository/writing/guides/.
Copyright Information
Copyright © 1994-2024 Colorado State University and/or this site's authors, developers, and contributors . Some material displayed on this site is used with permission.
Have a language expert improve your writing
Run a free plagiarism check in 10 minutes, automatically generate references for free.
- Knowledge Base
- Methodology
- Case Study | Definition, Examples & Methods
Case Study | Definition, Examples & Methods
Published on 5 May 2022 by Shona McCombes . Revised on 30 January 2023.
A case study is a detailed study of a specific subject, such as a person, group, place, event, organisation, or phenomenon. Case studies are commonly used in social, educational, clinical, and business research.
A case study research design usually involves qualitative methods , but quantitative methods are sometimes also used. Case studies are good for describing , comparing, evaluating, and understanding different aspects of a research problem .
Table of contents
When to do a case study, step 1: select a case, step 2: build a theoretical framework, step 3: collect your data, step 4: describe and analyse the case.
A case study is an appropriate research design when you want to gain concrete, contextual, in-depth knowledge about a specific real-world subject. It allows you to explore the key characteristics, meanings, and implications of the case.
Case studies are often a good choice in a thesis or dissertation . They keep your project focused and manageable when you don’t have the time or resources to do large-scale research.
You might use just one complex case study where you explore a single subject in depth, or conduct multiple case studies to compare and illuminate different aspects of your research problem.
Research question | Case study |
---|---|
What are the ecological effects of wolf reintroduction? | Case study of wolf reintroduction in Yellowstone National Park in the US |
How do populist politicians use narratives about history to gain support? | Case studies of Hungarian prime minister Viktor Orbán and US president Donald Trump |
How can teachers implement active learning strategies in mixed-level classrooms? | Case study of a local school that promotes active learning |
What are the main advantages and disadvantages of wind farms for rural communities? | Case studies of three rural wind farm development projects in different parts of the country |
How are viral marketing strategies changing the relationship between companies and consumers? | Case study of the iPhone X marketing campaign |
How do experiences of work in the gig economy differ by gender, race, and age? | Case studies of Deliveroo and Uber drivers in London |
Prevent plagiarism, run a free check.
Once you have developed your problem statement and research questions , you should be ready to choose the specific case that you want to focus on. A good case study should have the potential to:
- Provide new or unexpected insights into the subject
- Challenge or complicate existing assumptions and theories
- Propose practical courses of action to resolve a problem
- Open up new directions for future research
Unlike quantitative or experimental research, a strong case study does not require a random or representative sample. In fact, case studies often deliberately focus on unusual, neglected, or outlying cases which may shed new light on the research problem.
If you find yourself aiming to simultaneously investigate and solve an issue, consider conducting action research . As its name suggests, action research conducts research and takes action at the same time, and is highly iterative and flexible.
However, you can also choose a more common or representative case to exemplify a particular category, experience, or phenomenon.
While case studies focus more on concrete details than general theories, they should usually have some connection with theory in the field. This way the case study is not just an isolated description, but is integrated into existing knowledge about the topic. It might aim to:
- Exemplify a theory by showing how it explains the case under investigation
- Expand on a theory by uncovering new concepts and ideas that need to be incorporated
- Challenge a theory by exploring an outlier case that doesn’t fit with established assumptions
To ensure that your analysis of the case has a solid academic grounding, you should conduct a literature review of sources related to the topic and develop a theoretical framework . This means identifying key concepts and theories to guide your analysis and interpretation.
There are many different research methods you can use to collect data on your subject. Case studies tend to focus on qualitative data using methods such as interviews, observations, and analysis of primary and secondary sources (e.g., newspaper articles, photographs, official records). Sometimes a case study will also collect quantitative data .
The aim is to gain as thorough an understanding as possible of the case and its context.
In writing up the case study, you need to bring together all the relevant aspects to give as complete a picture as possible of the subject.
How you report your findings depends on the type of research you are doing. Some case studies are structured like a standard scientific paper or thesis, with separate sections or chapters for the methods , results , and discussion .
Others are written in a more narrative style, aiming to explore the case from various angles and analyse its meanings and implications (for example, by using textual analysis or discourse analysis ).
In all cases, though, make sure to give contextual details about the case, connect it back to the literature and theory, and discuss how it fits into wider patterns or debates.
Cite this Scribbr article
If you want to cite this source, you can copy and paste the citation or click the ‘Cite this Scribbr article’ button to automatically add the citation to our free Reference Generator.
McCombes, S. (2023, January 30). Case Study | Definition, Examples & Methods. Scribbr. Retrieved 27 September 2024, from https://www.scribbr.co.uk/research-methods/case-studies/
Is this article helpful?
Shona McCombes
Other students also liked, correlational research | guide, design & examples, a quick guide to experimental design | 5 steps & examples, descriptive research design | definition, methods & examples.
Observational vs. Experimental Study: A Comprehensive Guide
Explore the fundamental disparities between experimental and observational studies in this comprehensive guide by Santos Research Center, Corp. Uncover concepts such as control group, random sample, cohort studies, response variable, and explanatory variable that shape the foundation of these methodologies. Discover the significance of randomized controlled trials and case control studies, examining causal relationships and the role of dependent variables and independent variables in research designs.
This enlightening exploration also delves into the meticulous scientific study process, involving survey members, systematic reviews, and statistical analyses. Investigate the careful balance of control group and treatment group dynamics, highlighting how researchers meticulously assign variables and analyze statistical patterns to discern meaningful insights. From dissecting issues like lung cancer to understanding sleep patterns, this guide emphasizes the precision of controlled experiments and controlled trials, where variables are isolated and scrutinized, paving the way for a deeper comprehension of the world through empirical research.
Introduction to Observational and Experimental Studies
These two studies are the cornerstones of scientific inquiry, each offering a distinct approach to unraveling the mysteries of the natural world.
Observational studies allow us to observe, document, and gather data without direct intervention. They provide a means to explore real-world scenarios and trends, making them valuable when manipulating variables is not feasible or ethical. From surveys to meticulous observations, these studies shed light on existing conditions and relationships.
Experimental studies , in contrast, put researchers in the driver's seat. They involve the deliberate manipulation of variables to understand their impact on specific outcomes. By controlling the conditions, experimental studies establish causal relationships, answering questions of causality with precision. This approach is pivotal for hypothesis testing and informed decision-making.
At Santos Research Center, Corp., we recognize the importance of both observational and experimental studies. We employ these methodologies in our diverse research projects to ensure the highest quality of scientific investigation and to answer a wide range of research questions.
Observational Studies: A Closer Look
In our exploration of research methodologies, let's zoom in on observational research studies—an essential facet of scientific inquiry that we at Santos Research Center, Corp., expertly employ in our diverse research projects.
What is an Observational Study?
Observational research studies involve the passive observation of subjects without any intervention or manipulation by researchers. These studies are designed to scrutinize the relationships between variables and test subjects, uncover patterns, and draw conclusions grounded in real-world data.
Researchers refrain from interfering with the natural course of events in controlled experiment. Instead, they meticulously gather data by keenly observing and documenting information about the test subjects and their surroundings. This approach permits the examination of variables that cannot be ethically or feasibly manipulated, making it particularly valuable in certain research scenarios.
Types of Observational Studies
Now, let's delve into the various forms that observational studies can take, each with its distinct characteristics and applications.
Cohort Studies: A cohort study is a type of observational study that entails tracking one group of individuals over an extended period. Its primary goal is to identify potential causes or risk factors for specific outcomes or treatment group. Cohort studies provide valuable insights into the development of conditions or diseases and the factors that influence them.
Case-Control Studies: Case-control studies, on the other hand, involve the comparison of individuals with a particular condition or outcome to those without it (the control group). These studies aim to discern potential causal factors or associations that may have contributed to the development of the condition under investigation.
Cross-Sectional Studies: Cross-sectional studies take a snapshot of a diverse group of individuals at a single point in time. By collecting data from this snapshot, researchers gain insights into the prevalence of a specific condition or the relationships between variables at that precise moment. Cross-sectional studies are often used to assess the health status of the different groups within a population or explore the interplay between various factors.
Advantages and Limitations of Observational Studies
Observational studies, as we've explored, are a vital pillar of scientific research, offering unique insights into real-world phenomena. In this section, we will dissect the advantages and limitations that characterize these studies, shedding light on the intricacies that researchers grapple with when employing this methodology.
Advantages: One of the paramount advantages of observational studies lies in their utilization of real-world data. Unlike controlled experiments that operate in artificial settings, observational studies embrace the complexities of the natural world. This approach enables researchers to capture genuine behaviors, patterns, and occurrences as they unfold. As a result, the data collected reflects the intricacies of real-life scenarios, making it highly relevant and applicable to diverse settings and populations.
Moreover, in a randomized controlled trial, researchers looked to randomly assign participants to a group. Observational studies excel in their capacity to examine long-term trends. By observing one group of subjects over extended periods, research scientists gain the ability to track developments, trends, and shifts in behavior or outcomes. This longitudinal perspective is invaluable when studying phenomena that evolve gradually, such as chronic diseases, societal changes, or environmental shifts. It allows for the detection of subtle nuances that may be missed in shorter-term investigations.
Limitations: However, like any research methodology, observational studies are not without their limitations. One significant challenge of statistical study lies in the potential for biases. Since researchers do not intervene in the subjects' experiences, various biases can creep into the data collection process. These biases may arise from participant self-reporting, observer bias, or selection bias in random sample, among others. Careful design and rigorous data analysis are crucial for mitigating these biases.
Another limitation is the presence of confounding variables. In observational studies, it can be challenging to isolate the effect of a specific variable from the myriad of other factors at play. These confounding variables can obscure the true relationship between the variables of interest, making it difficult to establish causation definitively. Research scientists must employ statistical techniques to control for or adjust these confounding variables.
Additionally, observational studies face constraints in their ability to establish causation. While they can identify associations and correlations between variables, they cannot prove causality or causal relationship. Establishing causation typically requires controlled experiments where researchers can manipulate independent variables systematically. In observational studies, researchers can only infer potential causation based on the observed associations.
Experimental Studies: Delving Deeper
In the intricate landscape of scientific research, we now turn our gaze toward experimental studies—a dynamic and powerful method that Santos Research Center, Corp. skillfully employs in our pursuit of knowledge.
What is an Experimental Study?
While some studies observe and gather data passively, experimental studies take a more proactive approach. Here, researchers actively introduce an intervention or treatment to an experiment group study its effects on one or more variables. This methodology empowers researchers to manipulate independent variables deliberately and examine their direct impact on dependent variables.
Experimental research are distinguished by their exceptional ability to establish cause-and-effect relationships. This invaluable characteristic allows researchers to unlock the mysteries of how one variable influences another, offering profound insights into the scientific questions at hand. Within the controlled environment of an experimental study, researchers can systematically test hypotheses, shedding light on complex phenomena.
Key Features of Experimental Studies
Central to statistical analysis, the rigor and reliability of experimental studies are several key features that ensure the validity of their findings.
Randomized Controlled Trials: Randomization is a critical element in experimental studies, as it ensures that subjects are assigned to groups in a random assignment. This randomly assigned allocation minimizes the risk of unintentional biases and confounding variables, strengthening the credibility of the study's outcomes.
Control Groups: Control groups play a pivotal role in experimental studies by serving as a baseline for comparison. They enable researchers to assess the true impact of the intervention being studied. By comparing the outcomes of the intervention group to those of survey members of the control group, researchers can discern whether the intervention caused the observed changes.
Blinding: Both single-blind and double-blind techniques are employed in experimental studies to prevent biases from influencing the study or controlled trial's outcomes. Single-blind studies keep either the subjects or the researchers unaware of certain aspects of the study, while double-blind studies extend this blindness to both parties, enhancing the objectivity of the study.
These key features work in concert to uphold the integrity and trustworthiness of the results generated through experimental studies.
Advantages and Limitations of Experimental Studies
As with any research methodology, this one comes with its unique set of advantages and limitations.
Advantages: These studies offer the distinct advantage of establishing causal relationships between two or more variables together. The controlled environment allows researchers to exert authority over variables, ensuring that changes in the dependent variable can be attributed to the independent variable. This meticulous control results in high-quality, reliable data that can significantly contribute to scientific knowledge.
Limitations: However, experimental ones are not without their challenges. They may raise ethical concerns, particularly when the interventions involve potential risks to subjects. Additionally, their controlled nature can limit their real-world applicability, as the conditions in experiments may not accurately mirror those in the natural world. Moreover, executing an experimental study in randomized controlled, often demands substantial resources, with other variables including time, funding, and personnel.
Observational vs Experimental: A Side-by-Side Comparison
Having previously examined observational and experimental studies individually, we now embark on a side-by-side comparison to illuminate the key distinctions and commonalities between these foundational research approaches.
Key Differences and Notable Similarities
Methodologies
- Observational Studies : Characterized by passive observation, where researchers collect data without direct intervention, allowing the natural course of events to unfold.
- Experimental Studies : Involve active intervention, where researchers deliberately manipulate variables to discern their impact on specific outcomes, ensuring control over the experimental conditions.
- Observational Studies : Designed to identify patterns, correlations, and associations within existing data, shedding light on relationships within real-world settings.
- Experimental Studies : Geared toward establishing causality by determining the cause-and-effect relationships between variables, often in controlled laboratory environments.
- Observational Studies : Yield real-world data, reflecting the complexities and nuances of natural phenomena.
- Experimental Studies : Generate controlled data, allowing for precise analysis and the establishment of clear causal connections.
Observational studies excel at exploring associations and uncovering patterns within the intricacies of real-world settings, while experimental studies shine as the gold standard for discerning cause-and-effect relationships through meticulous control and manipulation in controlled environments. Understanding these differences and similarities empowers researchers to choose the most appropriate method for their specific research objectives.
When to Use Which: Practical Applications
The decision to employ either observational or experimental studies hinges on the research objectives at hand and the available resources. Observational studies prove invaluable when variable manipulation is impractical or ethically challenging, making them ideal for delving into long-term trends and uncovering intricate associations between certain variables (response variable or explanatory variable). On the other hand, experimental studies emerge as indispensable tools when the aim is to definitively establish causation and methodically control variables.
At Santos Research Center, Corp., our approach to both scientific study and methodology is characterized by meticulous consideration of the specific research goals. We recognize that the quality of outcomes hinges on selecting the most appropriate method of research study. Our unwavering commitment to employing both observational and experimental research studies further underscores our dedication to advancing scientific knowledge across diverse domains.
Conclusion: The Synergy of Experimental and Observational Studies in Research
In conclusion, both observational and experimental studies are integral to scientific research, offering complementary approaches with unique strengths and limitations. At Santos Research Center, Corp., we leverage these methodologies to contribute meaningfully to the scientific community.
Explore our projects and initiatives at Santos Research Center, Corp. by visiting our website or contacting us at (813) 249-9100, where our unwavering commitment to rigorous research practices and advancing scientific knowledge awaits.
Recent Posts
At Santos Research Center, a medical research facility dedicated to advancing TBI treatments, we emphasize the importance of tailored rehabilitation...
Learn about COVID-19 rebound after Paxlovid, its symptoms, causes, and management strategies. Join our study at Santos Research Center. Apply now!
Learn everything about Respiratory Syncytial Virus (RSV), from symptoms and diagnosis to treatment and prevention. Stay informed and protect your health with...
Discover key insights on Alzheimer's disease, including symptoms, stages, and care tips. Learn how to manage the condition and find out how you can...
Discover expert insights on migraines, from symptoms and causes to management strategies, and learn about our specialized support at Santos Research Center.
Explore our in-depth guide on UTIs, covering everything from symptoms and causes to effective treatments, and learn how to manage and prevent urinary tract infections.
Your definitive guide to COVID symptoms. Dive deep into the signs of COVID-19, understand the new variants, and get answers to your most pressing questions.
Santos Research Center, Corp. is a research facility conducting paid clinical trials, in partnership with major pharmaceutical companies & CROs. We work with patients from across the Tampa Bay area.
Contact Details
Navigation menu.
American Speech-Language-Hearing Association
- Certification
- Publications
- Continuing Education
- Practice Management
- Audiologists
- Speech-Language Pathologists
- Academic & Faculty
- Audiology & SLP Assistants
Understanding Research Designs and External Scientific Evidence
- Evidence-Based Practice
- How to Search for Evidence in Communication Sciences and Disorders
- Understanding Research Designs and External Scientific Evidence
- Bias, Appraisal Tools, and Levels of Evidence
- Statistics Refresher
External scientific evidence is a component of evidence-based practice (EBP) and refers to sources outside of everyday clinical practice. This page discusses
- primary research ;
- secondary research ; and
- matching your clinical question to a type of evidence .
Primary Research
Primary research pertains to individual studies attempting to answer a specific research question using raw data collected by the researcher(s). In experimental studies, the investigator manipulates one or more variables to compare those that received the manipulated condition to those that did not. In qualitative and observational/non-experimental studies, although there is no experimental manipulation, they may involve a comparison group.
Primary research can be conducted
- retrospectively (i.e., researchers collect data on the study participants’ past) or
- prospectively (i.e., researchers follow study participants over time and collect data to capture change).
Here are some common types of study designs:
Experimental Study Designs
- Randomized controlled trial (RCT) – Participants are randomly assigned to either the control group or an experimental group. Researchers compare outcomes from each group to determine whether the intervention caused any change.
- Controlled trial – A study involving non-randomized groups (i.e., experimental, comparison/control), which helps determine the effects of the intervention.
- Single-subject designs – Also known as single-case experimental designs , this type of experimental design allows researchers to closely examine specific changes in each participant. Each participant serves as their own control (i.e., compared to themselves) and researchers measure the outcome or dependent variable repeatedly across phases (e.g., baseline phase, intervention phase, withdraw phase). There are many variations of a single-subject design study.
- Cross-over trial – This is a study in which participants first receive one type of treatment and then researchers switch them to a different type of treatment.
Observational/Non-Experimental Study Designs
- Cohort – A cohort is an observational design study, possibly including a control group, in which researchers follow participants over time to determine the factors leading to different outcomes. Cohort studies can be retrospective or prospective.
- Case–control – This retrospective, observational study identifies an outcome of interest and compares a sample of people with that outcome ( case ) and a sample of people without that outcome ( control ). This design enables researchers to determine possible differences of previous exposures, experiences, and risk factors—any of which could explain their different outcomes.
- Cross-sectional – This is a study of a single sample at one point in time to understand the relationships among variables in the sample.
- Case study – A case study is an uncontrolled, observational study of events and outcomes in a single case.
- Case series – A description of uncontrolled, non-experimental events and outcomes for a series of similar cases who receive the same intervention or have the same outcome.
The chart below can help you better understand the features of the study designs commonly seen in audiology and speech-language pathology research.
Study Design Type | Experimental | Observational | Retrospective | Prospective | Random Assignment of Groups |
---|---|---|---|---|---|
Controlled Trial | Y | Y | S | ||
Single-Subject | Y | Y | |||
Cross-over | Y | Y | S | ||
Cohort | Y | S | S | ||
Case-Control | Y | Y | |||
Cross-Sectional | Y | Y | |||
Case Study/Series | Y | S | S |
Note: Y=yes; S=sometimes.
Secondary Research
Secondary research , also called synthesized research , combines the findings from primary research studies and provides conclusions about that body of evidence. Below are three common types of synthesized research, which are also found on the ASHA Evidence Maps :
Systematic Reviews
Systematic reviews use systematic methods to search for and compile a body of evidence to answer a research or clinical question about the efficacy/effectiveness of an assessment or treatment approach. Typically, studies included in a systematic review have met predetermined eligibility and quality criteria (e.g., studies must be experimental designs). The systematic review then provides qualitative conclusions based on the included studies.
Well-done systematic reviews offer greater transparency because they provide details about their inclusion/exclusion process. They also typically assess each study for its methodological quality and level of evidence. Using transparent methods reduces bias and increases the confidence of the findings and conclusions of the research. Systematic reviews can provide a synopsis of the state of the evidence about a given clinical topic.
Meta-Analyses
Meta-analyses use systematic and statistical methods to answer a research or clinical question about a specific assessment or treatment approach. Like systematic reviews, included primary studies must meet predetermined eligibility and quality criteria. The meta-analyses provide quantitative conclusions (e.g., pooled effect size, confidence interval) to determine the overall treatment effect or effect size across studies. The additional statistical measures can provide a better picture of the clinical significance.
Clinical Practice Guidelines
Clinical practice guidelines are systematically developed statements created by a group of subject matter experts to provide a comprehensive overview of a disorder, detail the benefits and harms of specific assessment and treatment approaches, and optimize delivery of services. Guidelines grade recommendations based on the quality and amount of available evidence and classify them as either of the following two types of recommendations:
- Evidence-based recommendations : A systematic review of the evidence informs the group of experts and their recommendations.
- Consensus-based recommendations : These recommendations are based on a summary of expert opinions.
Matching Your Clinical Question to a Type of Evidence
Your clinical question determines the study design (e.g., randomized controlled trials, single-subject design) needed to address your question and impacts your search for evidence . Systematic reviews and meta-analyses should also include the study designs with the highest likelihood of answering your clinical question.
Keep in mind that if you are looking for research on a newer treatment or assessment approach, you may only find early-stage research designs, such as case studies and case series. These designs may provide preliminary evidence but cannot demonstrate the efficacy of the newer approaches.
Quality Control
Once you find study designs appropriate for your clinical question, you need to determine the methodological quality of the primary or secondary studies. There are different methods and checklists to appraise the methodological quality of primary and synthesized research.
See below to find out which study design best addresses your clinical question:
For Screening/Diagnosis Questions
Assess accuracy in differentiating clients with or without a condition.
Example question: Is an auditory brainstem response or an otoacoustic emissions screening more accurate in identifying newborns with hearing loss?
Preferred Study Design(s): Prospective, blind comparison to reference standard
Other Study Design(s): Cross-sectional
For Treatment/Service Delivery Questions
Determine the efficacy of an intervention.
Example question: What is the most effective treatment to improve cognition in adults with traumatic brain injury?
Preferred Study Design(s): Randomized controlled trial (RCT)
Other Study Design(s): Controlled trial (non-randomized) Single-subject/single-case experimental design
For Etiology Questions
Identify causes or risk factors of a condition.
Example question: What are the risk factors for speech and language disorders?
Preferred Study Design(s): Cohort
Other Study Design(s): Case–contro Case series
For Quality of Life/Perspective Questions
Obtain and assess clients’ opinions and experiences.
Example question: How do parents feel about implementing parent-mediated interventions?
Preferred Study Design(s): Qualitative studies (e.g., case study, case series)
Other Study Design(s): Not Applicable
For Prognosis Questions
Predict client’s likelihood of outcomes over time due to factors other than intervention.
Example question: What is the prognosis of a child with autism spectrum disorder?
Other Study Design(s): Case–control Case series
For Cost Q uestions
Compare cost of treatments, tests, and other factors due to the disorder.
Example question: What is the cost of care for individuals with dysphagia requiring a feeding tube compared to those requiring diet modification?
Preferred Study Design(s): Economic analysis
For Prevention Q uestions
Identify factors to reduce likelihood of a disorder.
Example question: What are some strategies to prevent hearing loss?
Preferred Study Design(s): Randomized control trial
Other Study Design(s): Controlled trial (non-randomized) Cohort Case-control
In This Section
- Awards, Grants & Funding
- Evidence-Based Practice (EBP)
- ASHA Survey Reports
- ASHA Member Data Reports
- National Outcomes Measurement System (NOMS)
- ASHA Journals
- Advertising Disclaimer
- Advertise with us
ASHA Corporate Partners
- Become A Corporate Partner
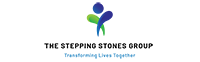
The American Speech-Language-Hearing Association (ASHA) is the national professional, scientific, and credentialing association for 234,000 members, certificate holders, and affiliates who are audiologists; speech-language pathologists; speech, language, and hearing scientists; audiology and speech-language pathology assistants; and students.
- All ASHA Websites
- Work at ASHA
- Marketing Solutions
Information For
Get involved.
- ASHA Community
- Become a Mentor
- Become a Volunteer
- Special Interest Groups (SIGs)
Connect With ASHA
American Speech-Language-Hearing Association 2200 Research Blvd., Rockville, MD 20850 Members: 800-498-2071 Non-Member: 800-638-8255
MORE WAYS TO CONNECT
Media Resources
- Press Queries
Site Help | A–Z Topic Index | Privacy Statement | Terms of Use © 1997- American Speech-Language-Hearing Association
Case Study vs. Research
What's the difference.
Case study and research are both methods used in academic and professional settings to gather information and gain insights. However, they differ in their approach and purpose. A case study is an in-depth analysis of a specific individual, group, or situation, aiming to understand the unique characteristics and dynamics involved. It often involves qualitative data collection methods such as interviews, observations, and document analysis. On the other hand, research is a systematic investigation conducted to generate new knowledge or validate existing theories. It typically involves a larger sample size and employs quantitative data collection methods such as surveys, experiments, or statistical analysis. While case studies provide detailed and context-specific information, research aims to generalize findings to a broader population.
Attribute | Case Study | Research |
---|---|---|
Definition | A detailed examination of a particular subject or situation over a period of time. | A systematic investigation to establish facts, principles, or to collect information on a subject. |
Purpose | To gain in-depth understanding of a specific case or phenomenon. | To contribute to existing knowledge and generate new insights. |
Scope | Usually focuses on a single case or a small number of cases. | Can cover a wide range of cases or subjects. |
Data Collection | Relies on various sources such as interviews, observations, documents, and artifacts. | Uses methods like surveys, experiments, observations, and interviews to collect data. |
Data Analysis | Often involves qualitative analysis, thematic coding, and pattern recognition. | Can involve both qualitative and quantitative analysis techniques. |
Generalizability | Findings may not be easily generalized due to the specific nature of the case. | Strives for generalizability to larger populations or contexts. |
Timeframe | Can be conducted over a relatively short or long period of time. | Can span from short-term studies to long-term longitudinal studies. |
Application | Often used in fields such as social sciences, business, and psychology. | Applied in various disciplines including natural sciences, social sciences, and humanities. |
Further Detail
Introduction.
When it comes to conducting studies and gathering information, researchers have various methods at their disposal. Two commonly used approaches are case study and research. While both methods aim to explore and understand a particular subject, they differ in their approach, scope, and the type of data they collect. In this article, we will delve into the attributes of case study and research, highlighting their similarities and differences.
A case study is an in-depth analysis of a specific individual, group, event, or phenomenon. It involves a detailed examination of a particular case to gain insights into its unique characteristics, context, and dynamics. Case studies often employ multiple sources of data, such as interviews, observations, and documents, to provide a comprehensive understanding of the subject under investigation.
One of the key attributes of a case study is its focus on a specific case, which allows researchers to explore complex and nuanced aspects of the subject. By examining a single case in detail, researchers can uncover rich and detailed information that may not be possible with broader research methods. Case studies are particularly useful when studying rare or unique phenomena, as they provide an opportunity to deeply analyze and understand them.
Furthermore, case studies often employ qualitative research methods, emphasizing the collection of non-numerical data. This qualitative approach allows researchers to capture the subjective experiences, perspectives, and motivations of the individuals or groups involved in the case. By using open-ended interviews and observations, researchers can gather rich and detailed data that provides a holistic view of the subject.
However, it is important to note that case studies have limitations. Due to their focus on a specific case, the findings may not be easily generalized to a larger population or context. The small sample size and unique characteristics of the case may limit the generalizability of the results. Additionally, the subjective nature of qualitative data collection in case studies may introduce bias or interpretation challenges.
Research, on the other hand, is a systematic investigation aimed at discovering new knowledge or validating existing theories. It involves the collection, analysis, and interpretation of data to answer research questions or test hypotheses. Research can be conducted using various methods, including surveys, experiments, and statistical analysis, depending on the nature of the study.
One of the primary attributes of research is its emphasis on generating generalizable knowledge. By using representative samples and statistical techniques, researchers aim to draw conclusions that can be applied to a larger population or context. This allows for the identification of patterns, trends, and relationships that can inform theories, policies, or practices.
Research often employs quantitative methods, focusing on the collection of numerical data that can be analyzed using statistical techniques. Surveys, experiments, and statistical analysis allow researchers to measure variables, establish correlations, and test hypotheses. This objective approach provides a level of objectivity and replicability that is crucial for scientific inquiry.
However, research also has its limitations. The focus on generalizability may sometimes sacrifice the depth and richness of understanding that case studies offer. The reliance on quantitative data may overlook important qualitative aspects of the subject, such as individual experiences or contextual factors. Additionally, the controlled nature of research settings may not fully capture the complexity and dynamics of real-world situations.
Similarities
Despite their differences, case studies and research share some common attributes. Both methods aim to gather information and generate knowledge about a particular subject. They require careful planning, data collection, analysis, and interpretation. Both case studies and research contribute to the advancement of knowledge in their respective fields.
Furthermore, both case studies and research can be used in various disciplines, including social sciences, psychology, business, and healthcare. They provide valuable insights and contribute to evidence-based decision-making. Whether it is understanding the impact of a new treatment, exploring consumer behavior, or investigating social phenomena, both case studies and research play a crucial role in expanding our understanding of the world.
In conclusion, case study and research are two distinct yet valuable approaches to studying and understanding a subject. Case studies offer an in-depth analysis of a specific case, providing rich and detailed information that may not be possible with broader research methods. On the other hand, research aims to generate generalizable knowledge by using representative samples and quantitative methods. While case studies emphasize qualitative data collection, research focuses on quantitative analysis. Both methods have their strengths and limitations, and their choice depends on the research objectives, scope, and context. By utilizing the appropriate method, researchers can gain valuable insights and contribute to the advancement of knowledge in their respective fields.
Comparisons may contain inaccurate information about people, places, or facts. Please report any issues.
- Experimental Research Designs: Types, Examples & Methods

Experimental research is the most familiar type of research design for individuals in the physical sciences and a host of other fields. This is mainly because experimental research is a classical scientific experiment, similar to those performed in high school science classes.
Imagine taking 2 samples of the same plant and exposing one of them to sunlight, while the other is kept away from sunlight. Let the plant exposed to sunlight be called sample A, while the latter is called sample B.
If after the duration of the research, we find out that sample A grows and sample B dies, even though they are both regularly wetted and given the same treatment. Therefore, we can conclude that sunlight will aid growth in all similar plants.
What is Experimental Research?
Experimental research is a scientific approach to research, where one or more independent variables are manipulated and applied to one or more dependent variables to measure their effect on the latter. The effect of the independent variables on the dependent variables is usually observed and recorded over some time, to aid researchers in drawing a reasonable conclusion regarding the relationship between these 2 variable types.
The experimental research method is widely used in physical and social sciences, psychology, and education. It is based on the comparison between two or more groups with a straightforward logic, which may, however, be difficult to execute.
Mostly related to a laboratory test procedure, experimental research designs involve collecting quantitative data and performing statistical analysis on them during research. Therefore, making it an example of quantitative research method .
What are The Types of Experimental Research Design?
The types of experimental research design are determined by the way the researcher assigns subjects to different conditions and groups. They are of 3 types, namely; pre-experimental, quasi-experimental, and true experimental research.
Pre-experimental Research Design
In pre-experimental research design, either a group or various dependent groups are observed for the effect of the application of an independent variable which is presumed to cause change. It is the simplest form of experimental research design and is treated with no control group.
Although very practical, experimental research is lacking in several areas of the true-experimental criteria. The pre-experimental research design is further divided into three types
- One-shot Case Study Research Design
In this type of experimental study, only one dependent group or variable is considered. The study is carried out after some treatment which was presumed to cause change, making it a posttest study.
- One-group Pretest-posttest Research Design:
This research design combines both posttest and pretest study by carrying out a test on a single group before the treatment is administered and after the treatment is administered. With the former being administered at the beginning of treatment and later at the end.
- Static-group Comparison:
In a static-group comparison study, 2 or more groups are placed under observation, where only one of the groups is subjected to some treatment while the other groups are held static. All the groups are post-tested, and the observed differences between the groups are assumed to be a result of the treatment.
Quasi-experimental Research Design
The word “quasi” means partial, half, or pseudo. Therefore, the quasi-experimental research bearing a resemblance to the true experimental research, but not the same. In quasi-experiments, the participants are not randomly assigned, and as such, they are used in settings where randomization is difficult or impossible.
This is very common in educational research, where administrators are unwilling to allow the random selection of students for experimental samples.
Some examples of quasi-experimental research design include; the time series, no equivalent control group design, and the counterbalanced design.

True Experimental Research Design
The true experimental research design relies on statistical analysis to approve or disprove a hypothesis. It is the most accurate type of experimental design and may be carried out with or without a pretest on at least 2 randomly assigned dependent subjects.
The true experimental research design must contain a control group, a variable that can be manipulated by the researcher, and the distribution must be random. The classification of true experimental design include:
- The posttest-only Control Group Design: In this design, subjects are randomly selected and assigned to the 2 groups (control and experimental), and only the experimental group is treated. After close observation, both groups are post-tested, and a conclusion is drawn from the difference between these groups.
- The pretest-posttest Control Group Design: For this control group design, subjects are randomly assigned to the 2 groups, both are presented, but only the experimental group is treated. After close observation, both groups are post-tested to measure the degree of change in each group.
- Solomon four-group Design: This is the combination of the pretest-only and the pretest-posttest control groups. In this case, the randomly selected subjects are placed into 4 groups.
The first two of these groups are tested using the posttest-only method, while the other two are tested using the pretest-posttest method.
Examples of Experimental Research
Experimental research examples are different, depending on the type of experimental research design that is being considered. The most basic example of experimental research is laboratory experiments, which may differ in nature depending on the subject of research.
Administering Exams After The End of Semester
During the semester, students in a class are lectured on particular courses and an exam is administered at the end of the semester. In this case, the students are the subjects or dependent variables while the lectures are the independent variables treated on the subjects.
Only one group of carefully selected subjects are considered in this research, making it a pre-experimental research design example. We will also notice that tests are only carried out at the end of the semester, and not at the beginning.
Further making it easy for us to conclude that it is a one-shot case study research.
Employee Skill Evaluation
Before employing a job seeker, organizations conduct tests that are used to screen out less qualified candidates from the pool of qualified applicants. This way, organizations can determine an employee’s skill set at the point of employment.
In the course of employment, organizations also carry out employee training to improve employee productivity and generally grow the organization. Further evaluation is carried out at the end of each training to test the impact of the training on employee skills, and test for improvement.
Here, the subject is the employee, while the treatment is the training conducted. This is a pretest-posttest control group experimental research example.
Evaluation of Teaching Method
Let us consider an academic institution that wants to evaluate the teaching method of 2 teachers to determine which is best. Imagine a case whereby the students assigned to each teacher is carefully selected probably due to personal request by parents or due to stubbornness and smartness.
This is a no equivalent group design example because the samples are not equal. By evaluating the effectiveness of each teacher’s teaching method this way, we may conclude after a post-test has been carried out.
However, this may be influenced by factors like the natural sweetness of a student. For example, a very smart student will grab more easily than his or her peers irrespective of the method of teaching.
What are the Characteristics of Experimental Research?
Experimental research contains dependent, independent and extraneous variables. The dependent variables are the variables being treated or manipulated and are sometimes called the subject of the research.
The independent variables are the experimental treatment being exerted on the dependent variables. Extraneous variables, on the other hand, are other factors affecting the experiment that may also contribute to the change.
The setting is where the experiment is carried out. Many experiments are carried out in the laboratory, where control can be exerted on the extraneous variables, thereby eliminating them.
Other experiments are carried out in a less controllable setting. The choice of setting used in research depends on the nature of the experiment being carried out.
- Multivariable
Experimental research may include multiple independent variables, e.g. time, skills, test scores, etc.
Why Use Experimental Research Design?
Experimental research design can be majorly used in physical sciences, social sciences, education, and psychology. It is used to make predictions and draw conclusions on a subject matter.
Some uses of experimental research design are highlighted below.
- Medicine: Experimental research is used to provide the proper treatment for diseases. In most cases, rather than directly using patients as the research subject, researchers take a sample of the bacteria from the patient’s body and are treated with the developed antibacterial
The changes observed during this period are recorded and evaluated to determine its effectiveness. This process can be carried out using different experimental research methods.
- Education: Asides from science subjects like Chemistry and Physics which involves teaching students how to perform experimental research, it can also be used in improving the standard of an academic institution. This includes testing students’ knowledge on different topics, coming up with better teaching methods, and the implementation of other programs that will aid student learning.
- Human Behavior: Social scientists are the ones who mostly use experimental research to test human behaviour. For example, consider 2 people randomly chosen to be the subject of the social interaction research where one person is placed in a room without human interaction for 1 year.
The other person is placed in a room with a few other people, enjoying human interaction. There will be a difference in their behaviour at the end of the experiment.
- UI/UX: During the product development phase, one of the major aims of the product team is to create a great user experience with the product. Therefore, before launching the final product design, potential are brought in to interact with the product.
For example, when finding it difficult to choose how to position a button or feature on the app interface, a random sample of product testers are allowed to test the 2 samples and how the button positioning influences the user interaction is recorded.
What are the Disadvantages of Experimental Research?
- It is highly prone to human error due to its dependency on variable control which may not be properly implemented. These errors could eliminate the validity of the experiment and the research being conducted.
- Exerting control of extraneous variables may create unrealistic situations. Eliminating real-life variables will result in inaccurate conclusions. This may also result in researchers controlling the variables to suit his or her personal preferences.
- It is a time-consuming process. So much time is spent on testing dependent variables and waiting for the effect of the manipulation of dependent variables to manifest.
- It is expensive.
- It is very risky and may have ethical complications that cannot be ignored. This is common in medical research, where failed trials may lead to a patient’s death or a deteriorating health condition.
- Experimental research results are not descriptive.
- Response bias can also be supplied by the subject of the conversation.
- Human responses in experimental research can be difficult to measure.
What are the Data Collection Methods in Experimental Research?
Data collection methods in experimental research are the different ways in which data can be collected for experimental research. They are used in different cases, depending on the type of research being carried out.
1. Observational Study
This type of study is carried out over a long period. It measures and observes the variables of interest without changing existing conditions.
When researching the effect of social interaction on human behavior, the subjects who are placed in 2 different environments are observed throughout the research. No matter the kind of absurd behavior that is exhibited by the subject during this period, its condition will not be changed.
This may be a very risky thing to do in medical cases because it may lead to death or worse medical conditions.
2. Simulations
This procedure uses mathematical, physical, or computer models to replicate a real-life process or situation. It is frequently used when the actual situation is too expensive, dangerous, or impractical to replicate in real life.
This method is commonly used in engineering and operational research for learning purposes and sometimes as a tool to estimate possible outcomes of real research. Some common situation software are Simulink, MATLAB, and Simul8.
Not all kinds of experimental research can be carried out using simulation as a data collection tool . It is very impractical for a lot of laboratory-based research that involves chemical processes.
A survey is a tool used to gather relevant data about the characteristics of a population and is one of the most common data collection tools. A survey consists of a group of questions prepared by the researcher, to be answered by the research subject.
Surveys can be shared with the respondents both physically and electronically. When collecting data through surveys, the kind of data collected depends on the respondent, and researchers have limited control over it.
Formplus is the best tool for collecting experimental data using survey s. It has relevant features that will aid the data collection process and can also be used in other aspects of experimental research.
Differences between Experimental and Non-Experimental Research
1. In experimental research, the researcher can control and manipulate the environment of the research, including the predictor variable which can be changed. On the other hand, non-experimental research cannot be controlled or manipulated by the researcher at will.
This is because it takes place in a real-life setting, where extraneous variables cannot be eliminated. Therefore, it is more difficult to conclude non-experimental studies, even though they are much more flexible and allow for a greater range of study fields.
2. The relationship between cause and effect cannot be established in non-experimental research, while it can be established in experimental research. This may be because many extraneous variables also influence the changes in the research subject, making it difficult to point at a particular variable as the cause of a particular change
3. Independent variables are not introduced, withdrawn, or manipulated in non-experimental designs, but the same may not be said about experimental research.
Experimental Research vs. Alternatives and When to Use Them
1. experimental research vs causal comparative.
Experimental research enables you to control variables and identify how the independent variable affects the dependent variable. Causal-comparative find out the cause-and-effect relationship between the variables by comparing already existing groups that are affected differently by the independent variable.
For example, in an experiment to see how K-12 education affects children and teenager development. An experimental research would split the children into groups, some would get formal K-12 education, while others won’t. This is not ethically right because every child has the right to education. So, what we do instead would be to compare already existing groups of children who are getting formal education with those who due to some circumstances can not.
Pros and Cons of Experimental vs Causal-Comparative Research
- Causal-Comparative: Strengths: More realistic than experiments, can be conducted in real-world settings. Weaknesses: Establishing causality can be weaker due to the lack of manipulation.
2. Experimental Research vs Correlational Research
When experimenting, you are trying to establish a cause-and-effect relationship between different variables. For example, you are trying to establish the effect of heat on water, the temperature keeps changing (independent variable) and you see how it affects the water (dependent variable).
For correlational research, you are not necessarily interested in the why or the cause-and-effect relationship between the variables, you are focusing on the relationship. Using the same water and temperature example, you are only interested in the fact that they change, you are not investigating which of the variables or other variables causes them to change.
Pros and Cons of Experimental vs Correlational Research
3. experimental research vs descriptive research.
With experimental research, you alter the independent variable to see how it affects the dependent variable, but with descriptive research you are simply studying the characteristics of the variable you are studying.
So, in an experiment to see how blown glass reacts to temperature, experimental research would keep altering the temperature to varying levels of high and low to see how it affects the dependent variable (glass). But descriptive research would investigate the glass properties.
Pros and Cons of Experimental vs Descriptive Research
4. experimental research vs action research.
Experimental research tests for causal relationships by focusing on one independent variable vs the dependent variable and keeps other variables constant. So, you are testing hypotheses and using the information from the research to contribute to knowledge.
However, with action research, you are using a real-world setting which means you are not controlling variables. You are also performing the research to solve actual problems and improve already established practices.
For example, if you are testing for how long commutes affect workers’ productivity. With experimental research, you would vary the length of commute to see how the time affects work. But with action research, you would account for other factors such as weather, commute route, nutrition, etc. Also, experimental research helps know the relationship between commute time and productivity, while action research helps you look for ways to improve productivity
Pros and Cons of Experimental vs Action Research
Conclusion .
Experimental research designs are often considered to be the standard in research designs. This is partly due to the common misconception that research is equivalent to scientific experiments—a component of experimental research design.
In this research design, one or more subjects or dependent variables are randomly assigned to different treatments (i.e. independent variables manipulated by the researcher) and the results are observed to conclude. One of the uniqueness of experimental research is in its ability to control the effect of extraneous variables.
Experimental research is suitable for research whose goal is to examine cause-effect relationships, e.g. explanatory research. It can be conducted in the laboratory or field settings, depending on the aim of the research that is being carried out.
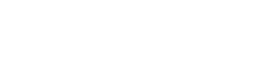
Connect to Formplus, Get Started Now - It's Free!
- examples of experimental research
- experimental research methods
- types of experimental research
- busayo.longe
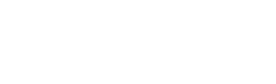
You may also like:
Response vs Explanatory Variables: Definition & Examples
In this article, we’ll be comparing the two types of variables, what they both mean and see some of their real-life applications in research

Experimental Vs Non-Experimental Research: 15 Key Differences
Differences between experimental and non experimental research on definitions, types, examples, data collection tools, uses, advantages etc.
What is Experimenter Bias? Definition, Types & Mitigation
In this article, we will look into the concept of experimental bias and how it can be identified in your research
Simpson’s Paradox & How to Avoid it in Experimental Research
In this article, we are going to look at Simpson’s Paradox from its historical point and later, we’ll consider its effect in...
Formplus - For Seamless Data Collection
Collect data the right way with a versatile data collection tool. try formplus and transform your work productivity today..
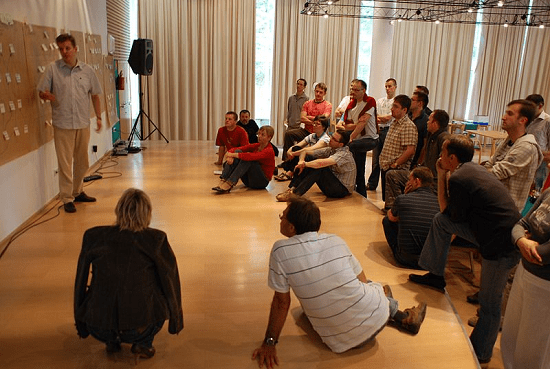
Distinguishing Between Case Studies & Experiments

Case Study vs Experiment
Case studies and experiments are two distinct research methods used across various disciplines, providing researchers with the ability to study and analyze a subject through different approaches. This variety in research methods allows the researcher to gather both qualitative and quantitative data, cross-check the data, and assign greater validity to the conclusions and overall findings of the research. A case study is a research method in which the researcher explores the subject in depth, while an experiment is a research method where two specific groups or variables are used to test a hypothesis. This article will examine the differences between case study and experiment further.
What is a Case Study?
A case study is a research method where an individual, event, or significant place is studied in depth. In the case of an individual, the researcher studies the person’s life history, which can include important days or special experiences. The case study method is used in various social sciences such as sociology, anthropology, and psychology. Through a case study, the researcher can identify and understand the subjective experiences of an individual regarding a specific topic. For example, a researcher studying the impact of second rape on the lives of rape victims can conduct several case studies to understand the subjective experiences of individuals and social mechanisms that contribute to this phenomenon. The case study is a qualitative research method that can be subjective.
What is an Experiment?
An experiment, unlike a case study, can be classified as a quantitative research method, as it provides statistically significant data and an objective, empirical approach. Experiments are primarily used in natural sciences, as they allow the scientist to control variables. In social sciences, controlling variables can be challenging and may lead to faulty conclusions. In an experiment, there are mainly two variables: the independent variable and the dependent variable. The researcher tries to test their hypothesis by manipulating these variables. There are different types of experiments, such as laboratory experiments (conducted in laboratories where conditions can be strictly controlled) and natural experiments (which take place in real-life settings). As seen, case study methods and experiments are very different from one another. However, most researchers prefer to use triangulation when conducting research to minimize biases.
Key Takeaways
- Case studies are in-depth explorations of a subject, providing qualitative data, while experiments test hypotheses by manipulating variables, providing quantitative data.
- Experiments are primarily used in natural sciences, whereas case studies are primarily used in social sciences.
- Experiments involve testing the correlation between two variables (independent and dependent), while case studies focus on exploring a subject in depth without testing correlations between variables.
LEAVE A REPLY Cancel reply
Save my name, email, and website in this browser for the next time I comment.
Related Articles
Difference between power & authority, distinguishing could of & could have, distinguishing pixie & bob haircuts, distinguishing between debate & discussion, distinguishing between dialogue & conversation, distinguishing between a present & a gift, distinguishing between will & can, distinguishing between up & upon.

An official website of the United States government
The .gov means it’s official. Federal government websites often end in .gov or .mil. Before sharing sensitive information, make sure you’re on a federal government site.
The site is secure. The https:// ensures that you are connecting to the official website and that any information you provide is encrypted and transmitted securely.
- Publications
- Account settings
- My Bibliography
- Collections
- Citation manager
Save citation to file
Email citation, add to collections.
- Create a new collection
- Add to an existing collection
Add to My Bibliography
Your saved search, create a file for external citation management software, your rss feed.
- Search in PubMed
- Search in NLM Catalog
- Add to Search
How to choose your study design
Affiliation.
- 1 Department of Medicine, Sydney Medical School, Faculty of Medicine and Health, University of Sydney, Sydney, New South Wales, Australia.
- PMID: 32479703
- DOI: 10.1111/jpc.14929
Research designs are broadly divided into observational studies (i.e. cross-sectional; case-control and cohort studies) and experimental studies (randomised control trials, RCTs). Each design has a specific role, and each has both advantages and disadvantages. Moreover, while the typical RCT is a parallel group design, there are now many variants to consider. It is important that both researchers and paediatricians are aware of the role of each study design, their respective pros and cons, and the inherent risk of bias with each design. While there are numerous quantitative study designs available to researchers, the final choice is dictated by two key factors. First, by the specific research question. That is, if the question is one of 'prevalence' (disease burden) then the ideal is a cross-sectional study; if it is a question of 'harm' - a case-control study; prognosis - a cohort and therapy - a RCT. Second, by what resources are available to you. This includes budget, time, feasibility re-patient numbers and research expertise. All these factors will severely limit the choice. While paediatricians would like to see more RCTs, these require a huge amount of resources, and in many situations will be unethical (e.g. potentially harmful intervention) or impractical (e.g. rare diseases). This paper gives a brief overview of the common study types, and for those embarking on such studies you will need far more comprehensive, detailed sources of information.
Keywords: experimental studies; observational studies; research method.
© 2020 Paediatrics and Child Health Division (The Royal Australasian College of Physicians).
PubMed Disclaimer
Similar articles
- Observational Studies. Hess DR. Hess DR. Respir Care. 2023 Nov;68(11):1585-1597. doi: 10.4187/respcare.11170. Epub 2023 Jun 20. Respir Care. 2023. PMID: 37339891
- Observational designs in clinical multiple sclerosis research: Particulars, practices and potentialities. Jongen PJ. Jongen PJ. Mult Scler Relat Disord. 2019 Oct;35:142-149. doi: 10.1016/j.msard.2019.07.006. Epub 2019 Jul 20. Mult Scler Relat Disord. 2019. PMID: 31394404 Review.
- Study designs in clinical research. Noordzij M, Dekker FW, Zoccali C, Jager KJ. Noordzij M, et al. Nephron Clin Pract. 2009;113(3):c218-21. doi: 10.1159/000235610. Epub 2009 Aug 18. Nephron Clin Pract. 2009. PMID: 19690439 Review.
- Study Types in Orthopaedics Research: Is My Study Design Appropriate for the Research Question? Zaniletti I, Devick KL, Larson DR, Lewallen DG, Berry DJ, Maradit Kremers H. Zaniletti I, et al. J Arthroplasty. 2022 Oct;37(10):1939-1944. doi: 10.1016/j.arth.2022.05.028. Epub 2022 Sep 6. J Arthroplasty. 2022. PMID: 36162926 Free PMC article.
- Design choices for observational studies of the effect of exposure on disease incidence. Gail MH, Altman DG, Cadarette SM, Collins G, Evans SJ, Sekula P, Williamson E, Woodward M. Gail MH, et al. BMJ Open. 2019 Dec 9;9(12):e031031. doi: 10.1136/bmjopen-2019-031031. BMJ Open. 2019. PMID: 31822541 Free PMC article.
- Effects of Electronic Serious Games on Older Adults With Alzheimer's Disease and Mild Cognitive Impairment: Systematic Review With Meta-Analysis of Randomized Controlled Trials. Zuo X, Tang Y, Chen Y, Zhou Z. Zuo X, et al. JMIR Serious Games. 2024 Jul 31;12:e55785. doi: 10.2196/55785. JMIR Serious Games. 2024. PMID: 39083796 Free PMC article. Review.
- Nurses' Adherence to the Portuguese Standard to Prevent Catheter-Associated Urinary Tract Infections (CAUTIs): An Observational Study. Paiva-Santos F, Santos-Costa P, Bastos C, Graveto J. Paiva-Santos F, et al. Nurs Rep. 2023 Oct 10;13(4):1432-1441. doi: 10.3390/nursrep13040120. Nurs Rep. 2023. PMID: 37873827 Free PMC article.
- Effects of regional anaesthesia on mortality in patients undergoing lower extremity amputation: A retrospective pooled analysis. Quak SM, Pillay N, Wong SN, Karthekeyan RB, Chan DXH, Liu CWY. Quak SM, et al. Indian J Anaesth. 2022 Jun;66(6):419-430. doi: 10.4103/ija.ija_917_21. Epub 2022 Jun 21. Indian J Anaesth. 2022. PMID: 35903599 Free PMC article.
- Peat J, Mellis CM, Williams K, Xuan W. Health Science Research: A Handbook of Quantitative Methods Chapter 2, Planning the Study. Sydney: Allen & Unwin; 2001.
- Guyatt G, Rennie D, Meade MO, Cook DJ. Users Guide to the Medical Literature: A Manual for Evidence-Based Clinical Practice, 3rd edn; Chapter 14, Harm (observational studies). New York, NY: McGraw-Hill; 2015.
- Centre for Evidence Based Medicine. Oxford EBM ‘Critical Appraisal tools’. Oxford University, UK. Available from: cebm.net [Accessed March 2020].
- Kahlert J, Bjerge Gribsholt S, Gammelager H, Dekkers OMet al. Control of confounding in the analysis phase - An overview for clinicians. Clin. Epidemiol. 2017; 9: 195-204.
- Sedgwick P. Cross sectional studies: Advantages and disadvantages. BMJ 2014; 348: g2276.
- Search in MeSH
LinkOut - more resources
Full text sources.
- Ovid Technologies, Inc.
Miscellaneous
- NCI CPTAC Assay Portal
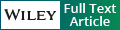
- Citation Manager
NCBI Literature Resources
MeSH PMC Bookshelf Disclaimer
The PubMed wordmark and PubMed logo are registered trademarks of the U.S. Department of Health and Human Services (HHS). Unauthorized use of these marks is strictly prohibited.
Thank you for visiting nature.com. You are using a browser version with limited support for CSS. To obtain the best experience, we recommend you use a more up to date browser (or turn off compatibility mode in Internet Explorer). In the meantime, to ensure continued support, we are displaying the site without styles and JavaScript.
- View all journals
- Explore content
- About the journal
- Publish with us
- Sign up for alerts
- Open access
- Published: 27 September 2024
Experimental study on bioaerosols behavior and purification measures in a subway compartment
- Renze Xu 1 ,
- Fan Wu 2 , 3 ,
- Lian Shen 1 ,
- Zhiqiang Fan 2 , 3 ,
- Jianci Yu 2 , 3 &
- Zhen Huang 4
Scientific Reports volume 14 , Article number: 22082 ( 2024 ) Cite this article
20 Accesses
Metrics details
- Engineering
- Environmental sciences
Bioaerosols in public transportation systems raise critical environmental concerns, seriously threatening passenger health and safety. In this study, we investigate the spread characteristics of bioaerosols in a standard type-B subway compartment using both air sampling and sediment sampling methods. Additionally, without compromising indoor passenger comfort, two self-designed air purification devices, based on intense field dielectric (IFD) and dielectric barrier discharge (DBD) technologies, respectively, are successfully applied for the improvement of the subway air quality. The results show that bioaerosols can propagate rapidly throughout the entire compartment in 5 min via airborne transmission. Under the effect of the symmetric air ducts and compartment structure, the difference in bioaerosol concentration in the air is less than 10% between both ends of the compartment. Concurrent substantial bioaerosol deposition on the ground, seats, and windows underscores the risk of contact transmission. Furthermore, the real-time purification rates of the two devices integrated into the air conditioning system reach 59.40% and 44.98%, respectively. With their demonstrated high efficiency in purifying bioaerosols and modular design featuring low energy consumption, easy cleaning, and reusability, these devices stand out as viable long-term solutions for large traffic vehicles. These research findings provide practical equipment recommendations and installation strategies for optimizing indoor air quality in subways and are applicable to other similar transportation systems.
Similar content being viewed by others
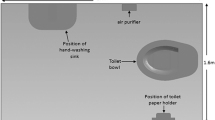
Exploring toilet plume bioaerosol exposure dynamics in public toilets using a Design of Experiments approach
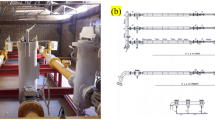
The influence of nano filter elements on pressure drop and pollutant elimination efficiency in town border stations
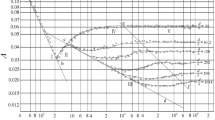
Experimental study on the influence of longitudinal slope on airflow-dust migration behavior after tunnel blasting
Introduction.
In recent years, respiratory infectious diseases carried by bioaerosols have increasingly posed considerable challenges to public health, economic growth, and societal progress, with the global emergence of Coronavirus disease 2019 (COVID-19) exacerbating these concerns 1 . On the other hand, as a useful strategy to alleviate surface traffic congestion, subway systems have gained prominence as the primary mode of transportation for daily commuters 2 . However, the predominantly underground operation of subway trains may result in restricted access to fresh air. Additionally, in conjunction with the rapid progression of urbanization, passenger density within subway systems has consistently grown, not only during peak commuting hours but also throughout other periods of the day. The risk of respiratory disease transmission inside subways may be positively correlated with the number of passengers 3 . Therefore, there is an urgent need to understand the dispersion patterns of bioaerosols in subway cabins and to establish effective measures to mitigate the risk of respiratory infections. This is crucial for the sustainable growth of rail transportation systems.
Indoor aerosol transmission patterns have been a topic of significant interest in the academic community 4 , 5 , 6 . Large size aerosols (with a diameter of 50 μm or more) tend to sediment due to their great mass, contaminating surfaces in indoor environments 7 , 8 . The dynamic characteristics of small size aerosols (with a diameter of 10 μm or less) are influenced by the surrounding flow field (including air velocity, temperature, and humidity), which could result in prolonged suspension and widespread dissemination of the aerosols 9 , 10 . Therefore, small size aerosols are considered to potentially facilitate more widespread disease transmission compared to large size aerosols. For these fine particles, upon being released, they evaporate rapidly within approximately 0.1 s, transforming into droplet nuclei 11 . Their evaporation and dispersion behaviors are relatively insensitive to changes in ambient humidity 11 , 12 , 13 . According to studies by Yan et al. (2020) 14 , the prevailing indoor airflow patterns were found to primarily govern the long-term movement and spread of these aerosols. Besides, previous literature 15 emphasized the need to consider the complete air duct system when studying indoor environments. Overly simplified ventilation models may fail to accurately predict indoor airflow patterns, potentially leading to incorrect distributions of aerosol dispersion within the cabin.
There has been extensive research on the diffusion and exposure risks of droplets, aerosols, and tracer gases in conventional indoor environments, such as hospitals and classrooms 16 , 17 . Various ventilation modes, formed by adjusting the positions of air supply and exhaust vents, have been applied to control the behavior of droplets. However, unlike these common indoor environments, which adopt air supply-exhaust systems or air supply-return systems, subway cabins typically utilize a combined air supply-return-exhaust circulation mode to reduce fan noise, save energy consumption, and maintain passenger comfort. Meanwhile, this ventilation system can result in extremely complex airflow patterns inside the cabin. Su et al. (2021) 18 discovered that cross-sectional changes within subway air ducts could cause local recirculation phenomena and reduce the uniformity of air distribution. Through field experiments, Wang et al. (2024) 19 observed that the airflow velocity distribution in a carriage exhibits an approximate ‘W-shape’, and the profile of the maximum airflow velocity is equivalent to a ‘parabolic surface’. Additionally, Tao et al. (2019) 20 employed porous media models and porous step-face models as substitutes for actual ventilation plates in subway trains. Currently, research on subway cabins primarily focuses on passenger comfort and airflow organization. However, investigations into aerosol motion, exposure risks, and optimal measures in subway indoor environments remain limited.
In scenarios where ventilation is constrained, the utilization of air purification devices has seen a marked expansion in many applications. The incorporation of physical-mechanical filters in buildings equipped with heating, ventilation, and air conditioning systems can capably decrease particulate matter concentrations in the air to levels below established thresholds 21 , 22 . Other fine air filtration devices, such as plasma air filters 23 , 24 and polytetrafluoroethylene multi-tube high efficiency membrane air filters 25 , are capable of removing pollutants. In general, the pollutant removal process can be categorized into two phases (adsorption and destruction). The process of capturing pollutants through physical or chemical means by a specific medium is referred to as adsorption 26 , 27 , while oxidation techniques like ozone generation, plasma-driven oxidation, and photocatalysis are classified as destructive processes 28 . However, it is essential to acknowledge that the feasibility and adaptability of air purification technologies still face significant challenges in practical engineering applications 29 . For instance, some highly efficient purification methods, such as portable air cleaners, may come with a notable increase in energy consumption, potentially rendering them uneconomical in the long run 30 . Moreover, some technologies (e.g., ozone generators) might not be suitable for densely populated or enclosed settings. Each control technology has its limitations and significance in addressing various indoor air pollutants. To date, air purification devices for reducing bioaerosols have not been applied in subways, and their effectiveness remains unknown. Given the ongoing pandemic, there is a pressing need for further exploration and design of appropriate air purification devices for subway environments.
In this study, we perform experiments on bioaerosols in a conventional type-B subway cabin, which is widely used for global railway transport 31 , 32 . Our experimental setup takes into account the air conditioning cycles and duct configuration. Using two sampling techniques, air and sediment sampling, we collect bioaerosols. These bioaerosols are then cultivated and analyzed to determine their propagation modes under various subway operational conditions, including fully enclosed scenarios and semi-enclosed settings. Moreover, aiming for the copresence of humans and machines in traffic environments, we develop two distinct air purification devices based on intense field dielectric (IFD) and dielectric barrier discharge (DBD) technologies, respectively. These devices have shown a high purification rate of bioaerosols in large traffic environments. Importantly, the concentration of ozone and other byproducts from these devices adheres to indoor air quality standards. To the best of our knowledge, this is the first application of such technologies to mitigate bioaerosols in subway cabin environments.
Materials and methods
Experimental setup.
The experimental approach can take into account some practical factors, such as air conditioning recirculation and air duct structure, among others, all of which present challenges when attempting to couple and evaluate their impact using simulation methods. The study investigates the characteristics of pathogen transmission inside the subway cabin using a combined microbiological and engineering approach. The entire research process is divided into three main stages: the preparation of the experimental environment and materials, the execution of specific test cases, and the collection and analysis of data.
Initially, the study constructs a subway train laboratory characterizing an authentic geometric configuration (refer to Fig. 1 a). The laboratory encompasses an indoor space with dimensions of 17.34 m (width) × 2.58 m (length) × 2.35 m (height). It is equipped with a comprehensive duct system and interior cabin furniture, enabling the assessment of the effects of diverse ventilation approaches and operation conditions on aerosol transmission. To enhance indoor thermal comfort, insulating panels have been embedded in the layered composition of the sidewalls, floor, and ceiling. The cabin furnishes six windows (dimensions of 1.51 m × 0.85 m), six doors (dimensions of 1.8 m × 1.4 m), five seating units (each extending 2.0 m in length), and four vertical supports (each rising 2.1 m in height).

Sketch of the generic train laboratory. ( a ) Compartment geometric. ( b ) Ventilaton mode.
This section provides a more detailed description of the ventilation system for the subway cabin. The entire ventilation system consists of air conditioning units, supply air ducts, return air inlets, and exhaust air ducts. Air treated by two air conditioning units is channeled into the compartment through supply air grilles located on the roof. A portion of this air re-enters the air conditioning units via the return air inlets, where it mixes with fresh air and is recirculated into the cabin. Simultaneously, the rest of the air is discharged directly into the external environment through exhaust air outlets. In accordance with the majority of operational subway trains, the current experimental laboratory employs a typical ventilation mode featuring a ‘top air supply-top air return-bottom air exhaust’ configuration (Fig. 1 b). This ventilation pattern is advantageous for efficiently expelling heat and pollutants within the cabin 33 . It also has positive performance in both energy efficiency and thermal comfort. Relying on the intelligent control cabinet of the air conditioning units, a variety of ventilation modes can be implemented. These encompass external circulation operation and internal circulation operation (without air exchange between the indoor and outdoor environments), each equipped with air supply frequency modulation capabilities in a frequency range of 30–50 Hz. In the context of the standard ventilation mode, the overall supply air volume in the cabin reaches 8,500 m³/h, incorporating a fresh air volume of 2,600 m³/h and a return air volume of 5,900 m³/h. The air conditioning system allows for precise regulation of indoor temperatures, maintaining a range between 16 °C and 28 °C with an accuracy of 0.1 °C.
Experimental devices
Aerosol generation and collection device.
Staphylococcus aureus ( S. aureus ), classified as a biosafety level (BSL) II organism, is routinely employed in bioaerosol dispersion studies within indoor settings and for testing the effectiveness of air purification systems 34 , 35 , 36 . In this investigation, pure cultures of S. aureus are inoculated onto Luria Bertani (LB) liquid medium. The cultures are maintained under controlled conditions at 37 °C with a shaking rate of 150 r/min for a duration of 24 h. The concentration of the microbial culture is ascertained utilizing the viable plate count methodology. The suspensions of S. aureus are diluted with phosphate-buffered saline to achieve a final concentration of 10 6 cfu/ml, which serves as the aerosol generation solution.
Research indicates that most particles emitted from human respiration are primarily within 10 μm 37 , 38 . For consistency, an atomizer is implemented as the aerosol generation system, producing particles that predominantly fall within this size range. To accurately emulate the authentic process of human aerosol release, a manikin representing a standing passenger (approximately 1.7 m in height) is connected to the atomizer via a flexible hose. This arrangement enables aerosol to be released through the nasal region, thus simulating the human breathing process (Fig. 2 a). The aerosol system is designed to exhibit time-varying respiratory flow rate based on tested human subjects, and this measured rate is compared with the existing respiratory data from a previous experiment 39 , as illustrated in Fig. 2 b. The results show the bioaerosol emission process closely resembles human respiratory activities. Moreover, the manikin engages in multiple breathing actions, with a 0.2 s interval between each breath.
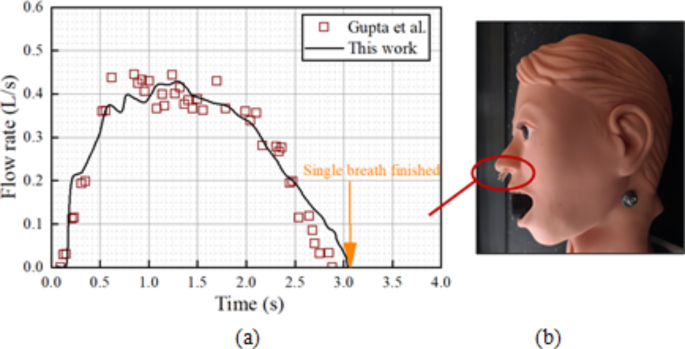
Flow curve of a single breath simulated by the aerosol generator. ( a ) Respiratory flow rate. ( b ) Aerosol generation.
This study uses two methods for collecting S. aureus . The first approach involves using Andersen six-stage air samplers for a 3.0 min collection duration at a flow rate of 28.3 L/min 40 to monitor the variations in bioaerosol concentration within the air. The second method entails placing Columbia blood agar plates directly onto compartment surfaces (such as windows and seats) to observe the deposition characteristics of S. aureus particles with small diameters under the effects of indoor turbulence. Upon completion of the experiments, all agar plates are incubated at 37 °C for 24 h in a constant temperature and humidity shaking incubator (HZQ-F160). Subsequently, the number of colonies on each plate is recorded and counted (i.e., colony forming unit: CFU) using an HCC-01 colony counter.
Air purification device
Given the frequent presence of passengers in traffic environments, air purification devices need to exhibit the characteristics of copresence of human-machine. Moreover, pathogen disinfection in subway cabins requires a targeted approach that is simultaneously implemented in the cabin’s airflow, air ducts, and air conditioning units. We ultimately design two distinct air purification devices based on the suitable techniques and proceeded to carry out experimental evaluations of their performance.
The first purification device employs an intense field dielectric (IFD) technique, utilizing dielectric materials as the medium. Although many traditional physical-mechanical filters 41 excel at capturing fine particulates, their design often results in high air resistance, which can negatively impact the overall airflow efficiency of the air conditioning system. With this in mind, we opt for the IFD filter. A notable feature of this filter is its low air resistance 42 , allowing a great volume of air to flow seamlessly into the air conditioning system. This choice stems from in the unique demands of a train environment, especially during peak times and within the confined spaces of train carriages. In such scenarios, maintaining a stable and sufficient air intake is crucial to guarantee good air circulation and ventilation inside the carriage. The purification process for the designed IFD filter is divided into three steps, as shown in Fig. 3 a. The first step involves the removal of large particles through a low-resistance filter (the thickness is 1.5 mm). Next, the particles are charged in the holes where the pin electrodes are located. Finally, the dielectric materials form honeycomb-shaped microchannels, generating a powerful electric field with the wrapped electrodes. This field attracts charged particles, thereby diminishing the presence of fine particulate matter. Additionally, the IFD filter neutralizes bacteria and microorganisms adhering to particulates in the strong electric field. This neutralization helps inhibit microbial growth on the filter surface, potentially reducing health risks to maintenance personnel who might come into contact with these microorganisms during inspections or replacements. According to the actual test, the operational power of the IFD filter used in the experiment is 12 W.
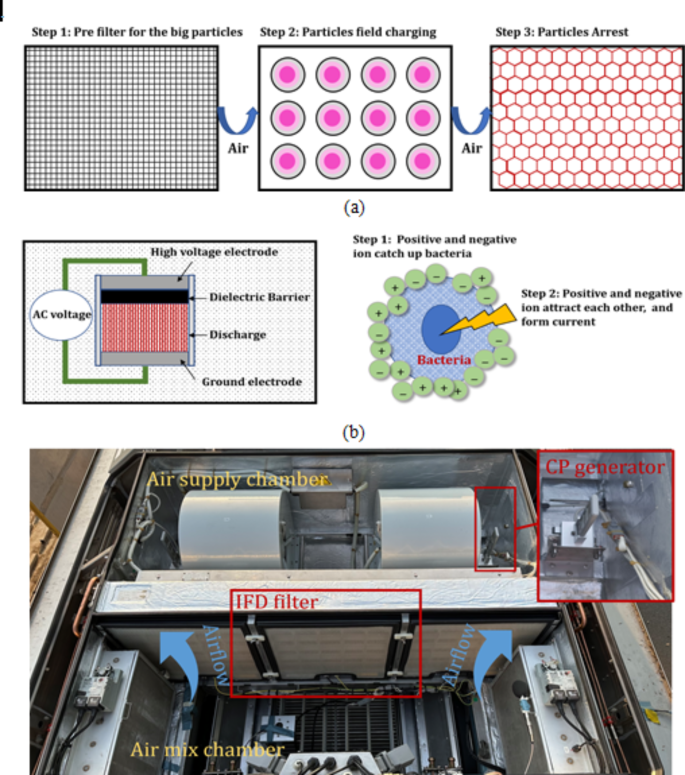
Air purification device and installation. ( a ) Operating mode of the IFD filter. ( b ) Operating mode of the CP generator. ( c ) Field installation of the IFD filter and CP generator in the air conditioning system.
The effective deployment of air purification devices is another central concern in numerous environmental safety studies. In the context of trains, the air conditioning system serves as the exclusive conduit linking the sealed cabin with the external operating environment, playing an important role in maintaining both indoor airflow quality and air cleanliness 43 . As a result, in this study, the IFD filter is installed in the mixing chamber of the rooftop air conditioning unit. This location represents the juncture where fresh and recirculated air streams merge, as depicted in Fig. 3 c. Strategically situated within the mixing chamber, the IFD filter exerts a considerable influence on the air entering the cabin.
The second purification device, a cold plasma (CP) generator, exploits a non-equilibrium gas discharge technology that features insulating materials integrated into the discharge space (illustrated in Fig. 3 b). The technology of negative ion air purification, known for its energy efficiency and low noise, has already been applied 41 , 44 . For the tested device, each distinct module generates a remarkable 3.5-5 million/cm³ positive and negative ions during the running process. Furthermore, the equipment mitigates potential hazards linked to high voltage exposure, a critical aspect in safeguarding the well-being of operators and the adjacent environment. In order to fill the entire cabin space, including the air duct system, with positive and negative ions, this study installs the CP generator in the air supply chamber of the air conditioning unit, as shown in Fig. 3 c.
Byproduct evaluation
Considering the long-time and high-density passenger distribution in rail transit systems, certain air purification devices (e.g., ultraviolet lamps) that generate many byproducts may not be suitable. This work also includes testing the indoor ozone concentrations when the air purification devices are operational.
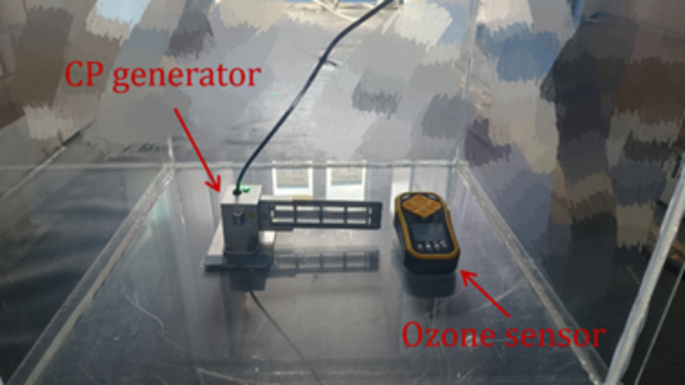
Test for ozone emission rate from CP generator.
We conduct experiments in a sealed chamber with dimensions of 0.5 m × 0.5 m × 0.5 m, as depicted in Fig. 4 . During the tests, we utilize an ozone monitor with the model number PR-MG41-O3, which has a resolution of 0.01 ppm. After several trials, we find that the ozone concentration within the chamber increases by an average of 1.75 ppm over a span of 10 min. Based on this, we estimate the ozone emission rate to be approximately 2.80 mg/h. On the other hand, the ozone test for the IFD device is carried out in an indoor environment, with measurements taken at the outlet of the duct equipped with the IFD filter. Using the same monitoring equipment, the ozone concentration at the air duct outlet is lower than the detector’s minimum measurement limit. The result indicates that the indoor ozone concentration meets the health standards for indoor air quality 45 . This finding suggests that both the IFD and CP devices are safe for scenarios emphasizing the copresence of human-machine.
In response to concerns related to ozone emissions, particularly the potential hazards stemming from secondary reaction byproducts, relevant experiments are undertaken. Upon activation of the air purification devices, we subject the indoor air to a continuous 4 h monitoring using TVOC (with a resolution of 0.001 ppm) and NO/NO 2 sensors (with a resolution of 0.1 ppm). Our experimental results indicate that there is no increase in the concentrations of TVOC and NO/NO 2 , suggesting that the operation of the devices does not significantly contribute to the generation of secondary reaction byproducts.
Experimental condition and devised cases
As temperature and humidity have been found to influence aerosol diffusion patterns 15 , full-scale experiments are conducted in a subway cabin environment with regulated temperature and humidity. The indoor temperature is maintained at approximately 20 °C, with a humidity level of 55-60%. Meanwhile, to evaluate the effects of subway door operations during station arrivals and departures, and to examine the efficiency of the air purification devices, five different experimental conditions are established, as presented in Table 1 . In actual subway operations, air conditioning systems employ sponge filters for particle filtration. This type of filter is also used in case 1 and case 2 to align with subway conditions. Some established standards related to indoor particulate matter, formaldehyde 46 , and comfort 47 recommend tests in an unoccupied setting to clearly discern characteristics like ventilation patterns. Therefore, our experimental scenarios are conducted in a vacant vehicle. In this controlled environment, a conventional external air circulation approach is employed with only the mannequin model releasing bioaerosols inside the cabin.
In our experiments, the aerosol generation apparatus is set to be located at the cabin’s center. The aerosol emission is directed towards the y -axis, which corresponds to the door opening direction in case 2. To explore the transmission of aerosols carrying active bacteria in the cabin’s complex flow field, two six-stage air samplers are positioned at both ends of the cabin, as shown in Fig. 5 (a). These samplers monitor changes in pathogen concentrations at the breathing zone height of seated passengers (1.1 m). Notably, in the door opening scenario (case 2), an additional six-stage air sampler is installed near the outside door to measure the external environment’s bioaerosol concentration. Furthermore, Columbia agar plates are strategically arranged on high-touch physical surfaces, such as seats and window ledges, to evaluate the potential contact risk related to pathogens, as shown in Fig. 5 (b). The placement and nomenclature of all measurement points can be seen in Fig. 5 (c).
At the same time, the measurements of airflow and bioaerosols are conducted separately to maintain consistency in the initial environmental conditions across different experimental scenarios. Prior to the commencement of each bioaerosol experimental round, portable hotwire anemometer devices are utilized to monitor the flow field data. Once the stability of the flow field conditions is confirmed, the occupant model commences the release of bioaerosols for a duration of 10 min 48 . The six-stage air sampler collects samples at the 5th, 15th, and 25th minutes during the experiment process, each of which lasts for 3 min (Fig. 6 ). Sample collection on the surface plates is carried out within the time range of 0 to 20 min. The two devices remain operational throughout the circumstances of case 3, case 4, and case 5 (designed for evaluating the efficiency of the purification devices). Within the time interval following each experimental round, the indoor environment is first cleaned using a disinfectant solution, followed by the air conditioner operating in the full fresh air mode for 1 h. Finally, the six-stage air sampler collects samples under the standard air supply mode to ensure that subsequent experiments are not influenced by previous ones or the background environment. The detailed steps of the bioaerosol experiment for different conditions are repeated in four rounds to reduce the potential for occasional errors.
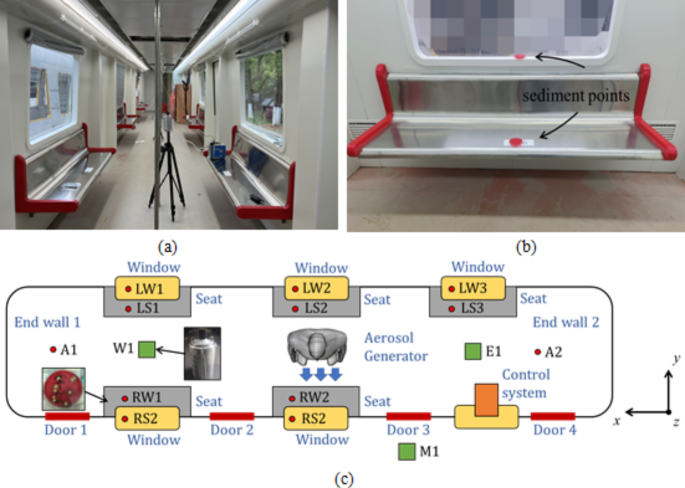
Locations of bioaerosol sampling points. ( a ) Air sampling points inside compartment. ( b ) Sediment sampling points inside compartment. ( c ) Overall measuring point layout.
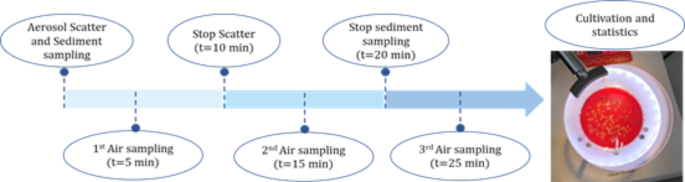
Procedures for bioaerosol experiment in the subway compartment.
Results and discussion
The distribution of flow field under standard ventilation mode.
The motion of aerosols is closely linked to the characteristics of indoor airflow patterns. Therefore, before conducting investigations concerning bioaerosols, it is crucial to understand the distribution of flow fields within the subway compartment. In compliance with EN14750-1 (2006) 47 , we employ hot-wire anemometer devices, CLIMOMASTER 6501, equipped with probe model 6543 and boasting a precision of 2%, to measure the temperature and velocity parameters within the interior space under the standard ventilation mode. In most areas of the compartment, the air velocities are observed to be below 0.5 m/s. Due to the relative positioning of the supply and return air vents, the peak velocity, registering at 0.51 m/s, manifests at the sampling sites located in the central portion of the cabin (Fig. 7 ). Furthermore, in the central region (region 2) of the cabin, the five sampling points show an average air velocity of 0.32 m/s, which slightly exceeds that of both ends (0.26 m/s for region 1 and 0.28 m/s for region 3). This is because the resistance and momentum loss in the air ducts decrease as the proximity to the air conditioning unit increases.
On the other hand, when examining the temperature distribution within the compartment, it is discernible that across both vertical and horizontal cross-sections, the temperature variation among the different sampling points does not exceed a difference of 2 °C. And the average temperature recorded at a height of 1.7 m from the floor is lower than that at 1.1 m. In general, under the influence of the top-dispersed air supply mode and the compartment’s symmetrical structure, the temperature and velocity fields within the compartment are uniform.
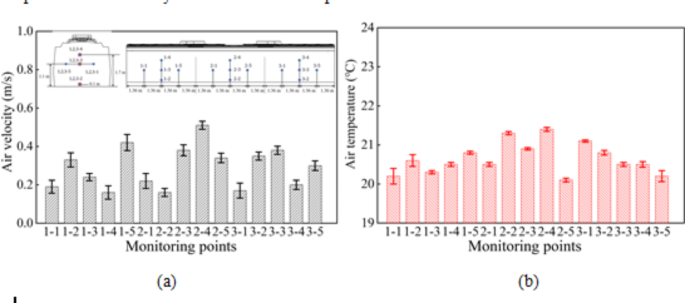
The average indoor airflow velocity and temperature under standard ventilation mode (The standard deviation of experimental results is used as the error bar). ( a ) Air velocity. ( b ) Air temperature.
The diffusion characteristics of bioaerosols
Figure 8 captures the single release process of bioaerosols from the manikin equipped with the aerosol generator through camera imaging. Clearly, in the initial phase of the release, the jet, driven by the strong momentum from the respiratory action, propels particles directly forward. With the decrease of respiratory flow, the compartment’s airflow starts to dominate. As a result, aerosols disperse into the surrounding space, subsequently causing a reduction in particle concentration directly in front of the manikin.
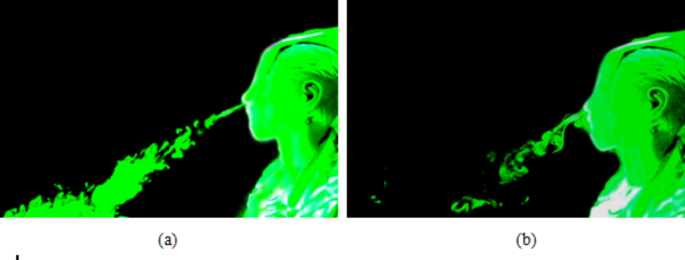
Visualization of bioaerosol process through breath mode at different time. ( a ) t = 1.5 s. ( b ) t = 2.5 s.
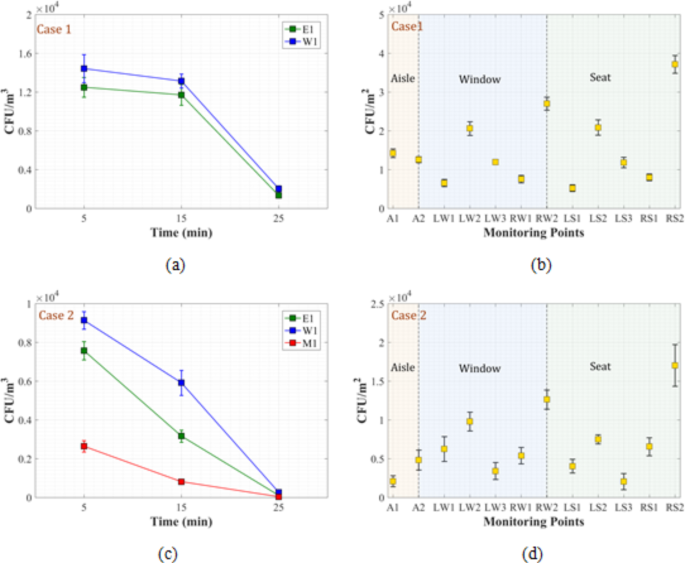
CFU of bioaerosol at different sampling methods under case 1 and case 2. ( a ) Air monitoring points under case 1. ( b ) Sediment monitoring points under case (1) ( c ) Air monitoring points under case (2) ( d ) Sediment monitoring points under case 2.
Figure 9 quantitatively summarizes the measurement results of the S. aureus bioaerosol particles collected using both methods (air sampling and sediment sampling). The coefficient of variation (the proportion of the sample standard deviation relative to the mean) is employed to evaluate the dependability of the experimental approach. The results show that the coefficient of variation for most measurement points ranges between 5% and 20%. The more considerable experimental deviations observed for some measurement points may be attributable to the intrinsic randomness of cabin turbulence (cabin air velocity below 0.3 Ma ) and the inescapable disturbances in the surrounding airflow induced by researchers collecting or replacing the plates. According to the research findings of Kunkel et al. (2017) 49 , the fluctuations in the experimental results of this study are within an acceptable range. From Fig. 9 a, the two air measurement points (E1 and W1) detect high concentrations of bioaerosols, indicating that the predominant mechanism for airborne transmission of bioaerosols in subway cabins is long-distance transport. The longitudinal transmission distance of bioaerosols can extend the entire length of the cabin. This means the recommended indoor safety distances 50 are not applicable to passengers in subway environments. Moreover, under the influence of the nearly symmetrical cabin structures and air ducts, the spatial distribution of bioaerosols exhibits relative uniformity (the difference in S. aureus colony counts between the two measurement points at the 15th minute is less than 10%), in line with the flow field characteristics detailed in Sect. 3.1 . To further understand the bioaerosol dispersion in the air, a comparative analysis of the quantity of bioaerosols across different particle size ranges is presented in Table 2 . Remarkably, peak concentrations at both ends (approximately accounting for 35% of the concentrations at the sampling sites) are predominantly observed within the 1.1 ~ 2.0 μm particle size range.
Upon the cessation of bioaerosol release, a significant portion of bacteria is either carried directly into the external cabin environment along with indoor airflow or deposited onto cabin surfaces, as depicted in Fig. 9 (b). At the 25th minute, there is a notable decrease in the S. aureus concentration within the air, showing a reduction of up to 90%. Simultaneously, the release direction significantly impacts aerosol deposition, with RS2 showing the highest concentration (surpassing 3 \(\:\times\:\) 10 4 CFU/m 2 ) among all measurement points. Additionally, the bacterial count detected on the window and seat surfaces via sedimentation plates demonstrates a sharp decline with an increasing distance from the release source, as illustrated in Fig. 10 . Some experimental studies employ tracer gases to simulate the flows of small size (less than 10 μm) aerosols 51 , 52 , neglecting the settling characteristics of such particles. However, bioaerosols carrying pathogens may adhere to any touchable surfaces in subway compartments due to the combined influence of the subway ventilation pattern and distinct indoor geometric structures. The results align with previous investigations, like a large-scale infection event within a Boeing 747 cabin, which underscored the significance of fomite transmission 53 . It is important to note that the actual risk of transmission may vary. Factors including the viability and the inactivation rate of the microorganism, as well as the frequency of human contact, all play a role in determining the real transmission potential. Meantime, our experimental outcomes also verify that tracer gases are not suitable for simulating the diffusion process of small sized aerosols capable of depositing on physical surfaces.
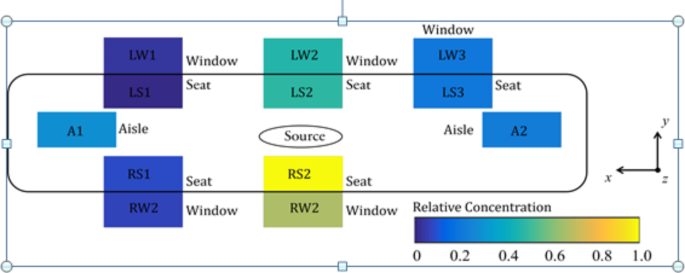
Distribution of bioaerosol settled on different physical surfaces.
Considering the practical operation of subway systems, frequent passenger boarding and alighting can alter the airflow dynamics of the compartments. Therefore, this study further analyzes the influence of the door-open scenario (case 2) on the bioaerosol distribution within the cabin, as displayed in Fig. 9 (c) and Fig. 9 (d). In conjunction with the data presented in Fig. 9 a and Fig. 9 b, it is found that a significant reduction of bacteria occurs in the air and on physical surfaces when the door is open, as compared to the door-closed condition (case 1). Air measurement point E1 exhibits a 39.38% reduction (at t = 5 min), while the sedimentation measurement point LS2 decreases by 54.17%. The reason may lie in the following aspects. Firstly, when the door opens, it changes the cabin’s pressure field and airflow pattern, which causes some of the airflow to move directly outside. Second, the open door reduces the physical barriers inside the cabin, decreasing in the overall accumulation of bioaerosols. Additionally, it should be noted that the door measurement point M1 outside the compartment detects a significant amount of S. aureus , comprising approximately 35.89% of the amount found at the air measurement point E1 inside the compartment. The escape of bioaerosols through the vehicle door highlights the potential exposure risk for passengers on the platform. This feature can explain instances of infection due to brief contact during subway travel.
The performance of air purification devices
Air purification devices are commonly used in relatively small indoor environments, but their efficiency and energy consumption in larger spaces require further investigation. In this section, the IFD filter and CP generator are assessed for their individual and combined effectiveness in purifying the air. To better quantify the performance of these devices, this study introduces the bioaerosol purification rate R :
where \(\:\stackrel{-}{N}\) and \(\:\stackrel{-}{{N}_{a}}\) represent the average values of four rounds of experiments for the control group (case 1) and the experimental group (i.e., cases 3, 4, and 5, with air purification devices activated), respectively 34 .
Figure 11 depicts the efficiency of various air purification devices in the cabin environment. For air sampling measurement points, during the continuous bacterial dispersal phase (t = 5 min), the R values for the IFD filter and the CP generator can reach 52.46-66.33% and 42.87-47.09%, respectively. Compared to the fresh air dilution effect achieved by relying solely on standard ventilation modes (Case 1), these purification devices can effectively reduce the bioaerosol concentration in the breathing zone of seated passengers in the entire cabin, thereby decreasing the risk of inhaling respiratory pathogens. As the operation time of the devices increases, the efficiency of bioaerosol purification for both devices gradually improves. After 25 min, the bioaerosol concentration in the cabin air approaches zero, demonstrating the rapid bioaerosol purification capability of the IFD and CP devices. In addition, for sediment sampling measurement points, the purification devices effectively reduced the detection amounts on the settling plates at various locations. The average purification ability of the CP generator (87.52%) surpasses that of the IFD filter (83.65%). It is speculated that the enhanced performance of the CP generator is attributed to its pulse discharge, which further inactivates S. aureus deposited on the surface of the material.
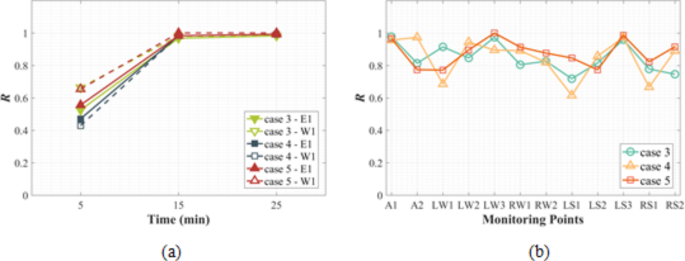
Purification rate test for different devices. ( a ) Air monitoring points. ( b ) Sediment monitoring points.
Another noteworthy finding is that at t = 5 min, the purification efficiency of air sampling point W1 in Case 5 (with both devices activated simultaneously) is marginally lower than that in Case 3 (with only the IFD filter activated). A possible explanation for this observation is that the electric field formed in the IFD filter concurrently absorbs the plasma produced by the CP generator in the indoor airflow and diminishes the combined efficiency. More research is required to provide detailed information about the underlying mechanisms. In future work, we plan to combine experimental and numerical simulation methods to investigate these issues.
We have compared the purification efficiency of air cleaners in different scenarios, as shown in Table 3 . While some literature reports that the purification efficiency of air cleaning devices can exceed 69% under certain conditions, the efficiency observed in our study deviates from these findings due to the specific experimental environment and application context. Specifically, in subway carriages, the released particles do not immediately enter the air conditioning system equipped with a purifier but may first disperse to other areas of the carriage. Furthermore, the spacious structure of the subway carriages constrains airflow circulation, and certain stagnant areas further hinder the efficient filtration of particles. Therefore, although our purification efficiency might appear slightly less optimal compared to other studies, our findings still offer valuable insights for evaluating the actual effects of air purification devices in such settings.
In conclusion, the IFD filter and the CP generator are both viable options when the air conditioning systems are functioning normally. The IFD filter purifies all air passing through the mixing chamber, including both return air and fresh air, thereby reducing the concentration of microorganisms in the cabin air. As for the CP generator, its functionality initially relies on the driving force provided by the air conditioning system, and subsequently, through the airflow organization created by the air duct structure, it disperses purifying materials throughout the indoor environment. These devices possess cleanability and reusability. From a technical standpoint, the installation and maintenance of the IFD filter and the CP generator are relatively convenient, and their operation does not have adverse effects on indoor temperature and humidity conditions. Moreover, with power consumption of 12 W for the IFD filter and 5 W for the CP generator, respectively, these devices avoid excessive energy consumption. Especially when considering the CP generator, its small size grants a high degree of flexibility, making it suitable for various scenarios. The concurrent deployment of multiple CP generator units in practical applications to enhance pathogen purification rates is also a feasible strategy.
Limitation and future work
While this study offers valuable insights into the transmission of bioaerosols within subway cabins, there are several limitations that should be pointed out. The observed transmission patterns derive from simulated experiments in empty subway cabins. In actual scenarios, human activities and resource location in the cabin may introduce variations to the transmission patterns. Future research will consider these additional influencing factors for a more accurate understanding. Moreover, developing an infection risk model allows for a concrete assessment and prediction of infection risks in indoor environments. Given the significant role particle size plays in disease transmission, it is worth further incorporating this factor into the model to give a more specific suggestion for passenger health in different traffic environments.
In this study, experimental methods are used to explore the diffusion of bioaerosols in a typical subway cabin. The application of both air and sediment sampling methods determines the primary modes of indoor aerosol propagation. Moreover, to decrease the spread of bioaerosols in large public transportation vehicles, our work assesses the individual and combined performance of the two designed air purification devices. The main conclusions of this research are as follows:
(1) Under the coupled effects of multiple flow fields in subway cabins, especially without passenger interference, bioaerosols can disperse throughout the entire cabin via airborne transmission. The symmetric indoor structure and air ducts further contribute to this relatively uniform bioaerosols distribution. Consequently, the concentration difference of aerosols in the air at both ends of the cabin is less than 10%.
(2) The phenomenon of bioaerosols deposition on surfaces at varying heights, including aisles, seats, and windows, further emphasizes the potential risk of contact transmission. However, the actual risk depends on factors such as the inactivation rate and viability of the microorganisms on these surfaces. On the other hand, tracer gases are unable to fully replicate the flow characteristics of small size aerosols in indoor settings featuring multiple obstacles.
(3) The opening of subway cabin doors can alter the diffusion patterns of indoor bioaerosols, leading to a decrease in the concentration of bioaerosols in the indoor air by approximately 38.37% (t = 5 min). Simultaneously, a considerable amount of aerosols is found to escape outside through the cabin door. This flow feature may pose a potential exposure risk to passengers on the platform.
(4) Without compromising the original airflow quality in the cabin, installing devices based on intense field dielectric (IFD) or dielectric barrier discharge (DBD) technologies in the air conditioning system can effectively reduce bioaerosol concentrations in large indoor spaces. During the continuous release of microbial aerosols, the real-time purification rates for the IFD filter and the CP generator reach 59.40% and 44.98%, respectively. However, when both devices operate simultaneously, the purification rate does not exhibit a significant increase, which may be related to the installation positions of the devices.
Data availability
The datasets and materials used and/or analyzed during the current study are available from the corresponding author on reasonable request.
Saccani, C. et al. Experimental testing of air filter efficiency against the SARS-CoV-2 virus: the role of droplet and airborne transmission. Build. Environ. 210 , 108728 (2022).
Article PubMed Google Scholar
Mao, Y. H. et al. A stratum ventilation system for pollutants and an improved prediction model for infection in subway cars. Atmospheric Pollution Res. 13 , 101354 (2022).
Article CAS Google Scholar
Harris, J. E. The Subways Seeded the Massive Coronavirus Epidemic in New York City. (2020).
Kumar, S. & King, M. D. Numerical investigation on indoor environment decontamination after sneezing. Environ. Res. 213 , 113665 (2022).
Article CAS PubMed PubMed Central Google Scholar
Bahramian, A., Mohammadi, M. & Ahmadi, G. Effect of indoor temperature on the velocity fields and airborne transmission of sneeze droplets: an experimental study and transient CFD modeling. Sci. Total Environ. 858 , 159444 (2023).
Article CAS PubMed Google Scholar
Paddy, E. N., Afolabi, O. O. D. & Sohail, M. Exploring toilet plume bioaerosol exposure dynamics in public toilets using a design of experiments approach. Sci. Rep. 14 , 10665 (2024).
Ahmadzadeh, M. & Shams, M. Passenger exposure to respiratory aerosols in a train cabin: effects of window, injection source, output flow location. Sustainable Cities Soc. 75 , 103280 (2021).
Article Google Scholar
Xu, R. Z. et al. Numerical comparison of ventilation modes on the transmission of coughing droplets in a train compartment. J. Wind Eng. Ind. Aerodyn. 231 , 105240 (2022).
Quinones, J. J. et al. Prediction of respiratory droplets evolution for safer academic facilities planning amid COVID-19 and future pandemics: a numerical approach. J. Building Eng. 54 , 104593 (2022).
Wu, J. L. & Weng, W. G. COVID-19 virus released from larynx might cause a higher exposure dose in indoor environment. Environ. Res. 199 , 111361 (2021).
Yang, X. et al. Transmission of pathogen-laden expiratory droplets in a coach bus. J. Hazard. Mater. 397 , 122609 (2020).
Peng, G. & Liu, F. Efect of an accelerating metro cabin on the difusion of cough droplets. Sci. Rep. 14 , 14150 (2024).
Wei, J. J. & Li, Y. G. Enhanced spread of expiratory droplets by turbulence in a cough jet. Build. Environ. 93 , 86–96 (2015).
Yan, Y. H. et al. Evaluation of cough-jet effects on the transport characteristics of respiratory-induced contaminants in airline passengers’ local environments. Build. Environ. 183 , 107206 (2020).
Article PubMed PubMed Central Google Scholar
Xu, R. Z. et al. Dispersion of evaporating droplets in the passenger compartment of high-speed train. J. Building Eng. 48 , 104001 (2022).
Fan, J. N. et al. Emission and purification of evaporated-condensed oil droplets affected by condensation nuclei during machining. J. Hazard. Mater. 459 , 132170 (2023).
Nie, W. et al. Research on airborne air curtain dust control technology and air volume optimization. Process Saf. Environ. Prot. 172 , 113–123 (2023).
Su, W. H. et al. Structural optimization of the tapered air ducts of a subway train. J. Railway Sci. Eng. 18 , 2138–2144 (2021).
Google Scholar
Wang, T. T. et al. Field study on the through-draught characteristics and its influencing factors in subway carriages. Tunn. Undergr. Space Technol. 143 , 105463 (2024).
Tao, Y. et al. Numerical and experimental study on Ventilation Panel Models in a Subway passenger compartment. Engineering . 5 , 329–336 (2019).
Lu, J. Y. et al. COVID-19 Outbreak Associated with Air Conditioning in Restaurant, Guangzhou, China, 2020. Emerg. Infect. Dis. 26 , 1628–1631 (2020).
Zhu, N. et al. A novel coronavirus from patients with Pneumonia in China, 2019. N. Engl. J. Med. 382 , 727–733 (2020).
Jung, J. S. & Kim, J. G. An indoor air purification technology using a non-thermal plasma reactor with multiple-wire-to-wire type electrodes and a fiber air filter. J. Electrostat. 86 , 12–17 (2017).
Luo, J. B. et al. Effect of regeneration method and ash deposition on diesel particulate filter performance: a review. Environ. Sci. Pollut. Res. 30 , 45607–45642 (2023).
Xu, H. et al. Study of the PTFE multi-tube high efficiency air filter for indoor air purification. Process Saf. Environ. Prot. 151 , 28–38 (2021).
Li, S. et al. Study on the influence of built-in open-hole dust cleaner on the cleaning performance of cartridge filter. Process Saf. Environ. Prot. 173 , 786–799 (2023).
Sublett, J. L. et al. Air filters and air cleaners: Rostrum by the American Academy of Allergy, Asthma & Immunology Indoor Allergen Committee. J. Allergy Clin. Immunol. 125 , 32–38 (2010).
Ongwandee, M. & Kruewan, A. Evaluation of Portable Household and In-Car air cleaners for air cleaning potential and ozone-initiated pollutants. Indoor Built Environ. 22 , 659–668 (2013).
Azimi, P. & Stephens, B. HVAC filtration for controlling infectious airborne disease transmission in indoor environments: Predicting risk reductions and operational costs. Build. Environ. 70 , 150–160 (2013).
Botvinnik, I., Taylor, C. E. & Snyder, G. High-efficiency portable electrostatic air cleaner with insulated electrodes (January 2007). IEEE Trans. Ind. Appl. 44 , 468–472 (2008).
Jin, M. T. et al. Pollution characteristics and source identification of PBDEs in public transport microenvironments. Sci. Total Environ. 820 , 153159 (2022).
Li, P. K. et al. Risk assessment of COVID-19 infection for subway commuters integrating dynamic changes in passenger numbers. Environ. Sci. Pollut. Res. 29 , 74715–74724 (2022).
Zhou, X. et al. Temperature control of air supply for Metro train air conditioner based on passenger density. Acta Aerodynamica Sinica . 39 , 162–169 (2021).
Liang, J. et al. Pump-inject antimicrobial and biodegradable aerogel as mask intermediate filter layer for medical protection of air filtration. Mater. Today Sustain. 19 , 100211 (2022).
Wang, C. et al. Use of nano titanium hydroxide and nano zirconium hydroxide fixed filter paper for rapid detection of Staphylococcus aureus in dairy products by PCR without pre-enrichment. Food Control . 148 , 109664 (2023).
Chang, C. W., Lin, Y. N., Huang, S. H. & Horng, Y. J. Efficiencies of filtration sampling and extraction for recovery of viable Staphylococcus aureus bioaerosols. J. Aerosol. Sci. 179 , 106390 (2024).
Borro, L. et al. The role of air conditioning in the diffusion of Sars-CoV-2 in indoor environments: a first computational fluid dynamic model, based on investigations performed at the Vatican State Children’s hospital. Environ. Res. 193 , 110343 (2021).
Zhao, Y., Feng, Y. & Ma, L. D. Numerical evaluation on indoor environment quality during high numbers of occupied passengers in the departure hall of an airport terminal. J. Building Eng. 51 , 104276 (2022).
Gupta, J. K., Lin, C. H. & Chen, Q. Y. Characterizing exhaled airflow from breathing and talking. Indoor Air . 20 , 31–39 (2010).
Liu, H. Y. et al. Distribution of droplets/droplet nuclei from coughing and breathing of patients with different postures in a hospital isolation ward. Build. Environ. 225 , 109690 (2022).
Li, J. C. et al. Plasma air filtration system for intercepting and inactivation of pathogenic microbial aerosols. J. Environ. Chem. Eng. 11 , 110728 (2023).
Ren, J. L. & Liu, J. J. Fine particulate matter control performance of a new kind of suspended fan filter unit for use in office buildings. Build. Environ. 149 , 468–476 (2019).
Elsaid, A. M., Mohamed, H. A., Abdelaziz, G. B. & Ahmed, M. S. A critical review of heating, ventilation, and air conditioning (HVAC) systems within the context of a global SARS-CoV-2 epidemic. Process Saf. Environ. Prot. 155 , 230–261 (2021).
Liu, W. et al. Negative ions offset cardiorespiratory benefits of PM 2.5 reduction from residential use of negative ion air purifiers. Indoor Air . 31 , 220–228 (2021).
Lai, A. C. K., Cheung, A. C. T., Wong, M. M. L. & Li, W. S. Evaluation of cold plasma inactivation efficacy against different airborne bacteria in ventilation duct flow. Build. Environ. 98 , 39–46 (2016).
TB/T3139. Decorating materials and indoor air limit of harmful substance for railway locomotive and vehicle. (2021).
EN14750-1. Railway Applications-Air Conditioning for Urban and Suburban Rolling Stock-Part 1: Comfort Parameters. (2006).
Wang, Y. X. et al. Droplet aerosols transportation and deposition for three respiratory behaviors in a typical negative pressure isolation ward. Build. Environ. 219 (2022).
Kunkel, S. A. et al. Quantifying the size-resolved dynamics of indoor bioaerosol transport and control. Indoor Air . 27 , 977–987 (2017).
Yamakawa, M. et al. Computational investigation of prolonged airborne dispersion of novel coronavirus-laden droplets. J. Aerosol. Sci. 155 , 105769 (2021).
Li, F. et al. Experimental study of gaseous and particulate contaminants distribution in an aircraft cabin. Atmos. Environ. 85 , 223–233 (2014).
Lu, Y. L., Oladokun, M. & Lin, Z. Reducing the exposure risk in hospital wards by applying stratum ventilation system. Build. Environ. 183 , 107204 (2020).
Lei, H. et al. Logistic growth of a surface contamination network and its role in disease spread. Sci. Rep. 7 , 1–10 (2017).
Zhang, Y. F. et al. Experimental study on PM 2.5 purification characteristics of different filter units in enclosed environments. Aerosol Air Qual. Res. 22 , 220184 (2022).
Bluyssen, P. M., Ortiz, M. & Zhang, D. The effect of a mobile HEPA filter system on ‘infectious’ aerosols, sound and air velocity in the SenseLab. Build. Environ. 188 , 107475 (2021).
Download references
Acknowledgements
This work was supported by the Hunan Provincial Talent Engineering Project (Grant No. 2023RC3192, 2023TJ-N17), the Training Program for Excellent Young Innovators of Changsha (Grant NO. kq1905004), and the National Natural Science Foundation of China (Grant NO. 52072413).
Author information
Authors and affiliations.
School of Civil Engineering, Changsha University, Changsha, 410022, Hunan, China
Renze Xu & Lian Shen
Key Laboratory of Traffic Safety on Track, School of Traffic & Transportation Engineering, Ministry of Education, Central South University, Changsha, 410075, Hunan, China
Fan Wu, Zhiqiang Fan & Jianci Yu
Joint International Research Laboratory of Key Technologies for Rail Traffic Safety, Changsha, 410075, Hunan, China
Design Institute of Hunan University, Changsha, 410082, Hunan, China
You can also search for this author in PubMed Google Scholar
Contributions
X.R.: Writing—original draft, Software, Experiments, Investigation; W.F.: Methodology, Investigation, Validation, Data curation; S.L.: Writing—review & editing, Funding acquisition, Investigation; F.Z.: Methodology, Investigation, Validation; Y.J.: Data curation, Investigation; H.Z.: Data curation, Investigation.
Corresponding author
Correspondence to Lian Shen .
Ethics declarations
Competing interests.
The authors declare no competing interests.
Additional information
Publisher’s note.
Springer Nature remains neutral with regard to jurisdictional claims in published maps and institutional affiliations.
Rights and permissions
Open Access This article is licensed under a Creative Commons Attribution-NonCommercial-NoDerivatives 4.0 International License, which permits any non-commercial use, sharing, distribution and reproduction in any medium or format, as long as you give appropriate credit to the original author(s) and the source, provide a link to the Creative Commons licence, and indicate if you modified the licensed material. You do not have permission under this licence to share adapted material derived from this article or parts of it. The images or other third party material in this article are included in the article’s Creative Commons licence, unless indicated otherwise in a credit line to the material. If material is not included in the article’s Creative Commons licence and your intended use is not permitted by statutory regulation or exceeds the permitted use, you will need to obtain permission directly from the copyright holder. To view a copy of this licence, visit http://creativecommons.org/licenses/by-nc-nd/4.0/ .
Reprints and permissions
About this article
Cite this article.
Xu, R., Wu, F., Shen, L. et al. Experimental study on bioaerosols behavior and purification measures in a subway compartment. Sci Rep 14 , 22082 (2024). https://doi.org/10.1038/s41598-024-73933-4
Download citation
Received : 10 July 2024
Accepted : 23 September 2024
Published : 27 September 2024
DOI : https://doi.org/10.1038/s41598-024-73933-4
Share this article
Anyone you share the following link with will be able to read this content:
Sorry, a shareable link is not currently available for this article.
Provided by the Springer Nature SharedIt content-sharing initiative
- Subway compartment
- Airborne transmission
- Bioaerosol concentration
- Purification efficiency
By submitting a comment you agree to abide by our Terms and Community Guidelines . If you find something abusive or that does not comply with our terms or guidelines please flag it as inappropriate.
Quick links
- Explore articles by subject
- Guide to authors
- Editorial policies
Sign up for the Nature Briefing newsletter — what matters in science, free to your inbox daily.

Information
- Author Services
Initiatives
You are accessing a machine-readable page. In order to be human-readable, please install an RSS reader.
All articles published by MDPI are made immediately available worldwide under an open access license. No special permission is required to reuse all or part of the article published by MDPI, including figures and tables. For articles published under an open access Creative Common CC BY license, any part of the article may be reused without permission provided that the original article is clearly cited. For more information, please refer to https://www.mdpi.com/openaccess .
Feature papers represent the most advanced research with significant potential for high impact in the field. A Feature Paper should be a substantial original Article that involves several techniques or approaches, provides an outlook for future research directions and describes possible research applications.
Feature papers are submitted upon individual invitation or recommendation by the scientific editors and must receive positive feedback from the reviewers.
Editor’s Choice articles are based on recommendations by the scientific editors of MDPI journals from around the world. Editors select a small number of articles recently published in the journal that they believe will be particularly interesting to readers, or important in the respective research area. The aim is to provide a snapshot of some of the most exciting work published in the various research areas of the journal.
Original Submission Date Received: .
- Active Journals
- Find a Journal
- Journal Proposal
- Proceedings Series
- For Authors
- For Reviewers
- For Editors
- For Librarians
- For Publishers
- For Societies
- For Conference Organizers
- Open Access Policy
- Institutional Open Access Program
- Special Issues Guidelines
- Editorial Process
- Research and Publication Ethics
- Article Processing Charges
- Testimonials
- Preprints.org
- SciProfiles
- Encyclopedia
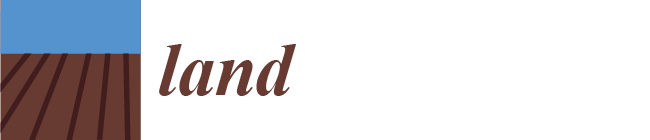
Article Menu

- Subscribe SciFeed
- Recommended Articles
- Google Scholar
- on Google Scholar
- Table of Contents
Find support for a specific problem in the support section of our website.
Please let us know what you think of our products and services.
Visit our dedicated information section to learn more about MDPI.
JSmol Viewer
Comparative study of cognitive differences in rural landscapes based on eye movement experiments.
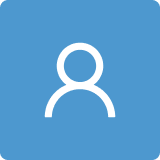
1. Introduction
1.1. research on rural landscapes from different perspectives, 1.2. research on rural landscape cognition, 1.3. classification methods for rural landscape image elements, 2. materials and methods, 2.1. sample site selection, 2.2. eye movement experiment, 2.2.1. selection of experimental photos, 2.2.2. selection of subjects, 2.2.3. experimental equipment and environment, 2.2.4. process of eye movement experiment, 2.2.5. data handling of eye movement experiment, 2.3. semi-structured interviews, 2.3.1. process of semi-structured interviews, 2.3.2. data handling of semi-structured interview, 3.1. analysis of eye movement indicators, 3.1.1. analysis of inter-group differences, 3.1.2. analysis of intra-group differences, 3.2. analysis of eye movement heat maps, 3.3. analysis of semi-structured interviews, 4. discussion, 5. conclusions, author contributions, data availability statement, acknowledgments, conflicts of interest.
- Kilos, T.; Primdahl, J.; Kristensen, L.S.; Busck, A.G. Introduction: Landscape change and rural development. Landsc. Res. 2010 , 35 , 571–576. [ Google Scholar ] [ CrossRef ]
- Liu, L.L.; Wu, R.N.; Lou, Y.R.; Luo, P.P.; Sun, Y.; He, B.; Hu, M.C.; Herath, S. Exploring the comprehensive evaluation of sustainable development in rural tourism: A perspective and method based on the AVC theory. Land 2023 , 12 , 1473. [ Google Scholar ] [ CrossRef ]
- Bartolini, F.; Vergamini, D. Introduction: Trade-offs and synergies between ecosystem services provided by different rural landscape. Agronomy 2023 , 13 , 997. [ Google Scholar ] [ CrossRef ]
- Yang, Q. Introduction: Research on the changes in cultural landscape of tourist-type traditional Chinese villages from the perspective of cultural memory: Taking Anshan Village in Chongqing as an example. Land 2023 , 12 , 816. [ Google Scholar ] [ CrossRef ]
- Xu, Z.Y. Research on the Planning and Design of Tourism Rural Landscape Trails. Master’ Thesis, Shandong Jianzhu University, Jinan, China, 2024. [ Google Scholar ]
- Zhao, Z.D. Research on Rural Tourism Landscape Planning and Design Based on Cultural Experience. Master’ Thesis, Henan Agricultural University, Zhengzhou, China, 2023. [ Google Scholar ] [ CrossRef ]
- Xie, H.L.; Zhu, Z.H.; He, Y.F.; Zeng, X.J.; Wen, Y.Y. Integrated framework of rural landscape research: Based on the global perspective. Landsc. Ecol. 2022 , 37 , 1161–1184. [ Google Scholar ] [ CrossRef ]
- Luo, W.B.; Lei, J.Q.; Shen, S.N.; Xu, F.X. Research on the influence mechanism of urban residents’ rural landscape cognition on tourism behavior intention. J. Hunan Fina. Econ. Univ. 2020 , 36 , 51–60. [ Google Scholar ] [ CrossRef ]
- Wang, X.C.; Luo, C.X.; Cai, W.T. Progress of Chinese Rural Landscape Characteristic System Research in Recent 20 Years. CHN Landsc. Arch. 2022 , 38 , 44–49. [ Google Scholar ] [ CrossRef ]
- Kim, E.-J.; Lim, C.; Choi, J. A Study on Planning Trails of Natural and Historic-Cultural Landscapes in Rural Villages. J. Kr. Soc. Rural Plan. 2014 , 20 , 159–171. [ Google Scholar ] [ CrossRef ]
- Zeng, X.G.; Zhao, Y.N. The Characteristics of Rural Settlement Landscape in Hilly Area from the Perspective of Ecological Environment. J. Coast. Res. 2020 , 103 , 506–510. [ Google Scholar ] [ CrossRef ]
- Li, W.W.; Zhou, Y.J.; Dai, X.G.; Hu, F. Evaluation of rural tourism landscape resources in terms of carbon neutrality and rural revitalization. Sustainability 2022 , 14 , 2863. [ Google Scholar ] [ CrossRef ]
- Yang, H.Y.; Wu, R.Z.; Qiu, B.; Zhang, Z.H.; Hu, T.Z.; Zou, J.H.; Wang, H. The next step in suburban rural revitalization: Integrated whole-process landscape management linking ecosystem services and landscape characteristics. Ecol. Indic. 2024 , 126 , 111999. [ Google Scholar ] [ CrossRef ]
- Cong, L.; Zhang, Y.J.; Su, C.H.; Chen, M.H.; Wang, J.N. Understanding tourists’ willingness-to-pay for rural landscape improvement and preference heterogeneity. Sustainability 2019 , 11 , 7001. [ Google Scholar ] [ CrossRef ]
- Li, M.X.; Yan, Y.; Ying, Z.Y.; Zhou, L. Measuring villagers’ perceptions of changes in the landscape values of traditional villages. ISPRS Int. J. Geo-Inf. 2024 , 13 , 60. [ Google Scholar ] [ CrossRef ]
- Chen, Z.F.; Wang, C.Y. The spatial ecological wisdom of rural landscape unit identification and cognition: A case study of Pai fang Community in Nanjing City. Landscape Arch. 2022 , 29 , 30–36. [ Google Scholar ] [ CrossRef ]
- Chen, H.; Guan, J.M.; Yang, H.T.; He, Y.X.; Zhu, B.; Chen, J. A review of domestic research on rural human settlement environment planning-Bibliometric and visual analysis based on CNKI academic journals. J. Manyang Tchr. Coll. 2022 , 41 , 117–123. [ Google Scholar ] [ CrossRef ]
- John, L. Land and Life: A Selection from the Writing of Carl Sauer , 1st ed.; University of California Press: Berkeley, CA, USA, 1963; pp. 12–15. [ Google Scholar ] [ CrossRef ]
- Dong, X. The significance, principles and index system of rural landscape classification. HM. Geo. 1990 , 1990 , 49–52+78. (In Chinese) [ Google Scholar ]
- Agnolotti, M. Rural landscape, nature conservation and culture: Some notes on research trends and management approaches from a (southern) European perspective. Landscape Urban Plan. 2014 , 126 , 66–73. [ Google Scholar ] [ CrossRef ]
- Torregiani, D.; Ludwiczak, Z.; Dall, A.E.; Benni, S.; Maino, E.; Tassinari, P. Trula: A high-resolution method for multi-time analysis of traditional rural landscapes and its application in Emilia-Romagna, Italy. Landsc. Urban Plan. 2014 , 124 , 93–103. [ Google Scholar ] [ CrossRef ]
- Dupont, L.; Ooms, K.; Antro, M.; Reveled, V.V. Comparing saliency maps and eye-tracking focus maps: The potential use in visual impact assessment based on landscape photographs. Landsc. Urban Plan. 2016 , 148 , 17–26. [ Google Scholar ] [ CrossRef ]
- Su, T.T.; Wang, K.P.; Li, S.S.; Wang, X.Y.; Li, H.; Ding, H.R.; Chen, Y.F.; Liu, C.H.; Liu, M.; Zhang, Y.L. Analysis and Optimization of Landscape Preference Characteristics of Rural Public Space Based on Eye-Tracking Technology: The Case of Huangshan Dian Village, China. Sustainability 2023 , 15 , 212. [ Google Scholar ] [ CrossRef ]
- Grindsted, A. Interactive resources used in semi-structured research interviewing. J. Pragma. 2005 , 37 , 1015–1035. [ Google Scholar ] [ CrossRef ]
- Gugu, P.C.; Rodríguez-Campos, L. Semi-structured interview protocol for constructing logic models. Eval. Program Plan. 2007 , 30 , 339–350. [ Google Scholar ] [ CrossRef ] [ PubMed ]
- Guo, S.L.; Zhao, N.X.; Zhang, J.X.; Xue, T.; Liu, P.X.; Xu, S.; Xu, D.D. Research Landscape visual quality assessment based on eye movement: College student eye-tracking experiments on Hongcun tourism landscape pictures. Res. Sci. 2017 , 39 , 1137–1147. (In Chinese) [ Google Scholar ]
- Duan, H.L.; Han, L. Research on the development of rural tourism. Res. Dev. Mkt. 2000 , 5 , 314–315. (In Chinese) [ Google Scholar ]
- Wang, Y.C.; Liu, B.Y. On Chinese rural landscape and rural landscape planning. CHN. Landscape Arch. 2003 , 1 , 56–59. (In Chinese) [ Google Scholar ]
- Liu, Y.F. A Study on the Imagery of Urban Historical Landscape in the Old City of Beijing , 1st ed.; Science Press: Beijing, China, 2019; pp. 23–25. [ Google Scholar ]
- Lynch, K. The Image of the City , 1st ed.; Fang, Y.; He, X., Translators; Huaxia Publishing House: Beijing, China, 2001; pp. 37–66. [ Google Scholar ]
- Liu, J.Y.; Li, L.M. Notice of the general office of the ministry of agriculture and rural affairs on the announcement of beautiful leisure villages in China in 2020. China Agric. Yearb. 2020 , 2020 , 521–524. [ Google Scholar ]
- Ma, L.; Xu, Y.Z.; He, S.Y.; Tang, Z.; Li, T.Z. Exploring cognition differences in the traditional village landscape based on eye-tracking experiment. Urban Dev. Stud. 2023 , 30 , 86–94. (In Chinese) [ Google Scholar ]
- Yang, M.M. Eye-Tracking and Chinese Reading , 1st ed.; Social Science Literature Publishing House: Beijing, China, 2018; pp. 36–56. [ Google Scholar ]
- Zhao, Y.; Lin, J.H.; Liu, Y. Research on visual evaluation of tourism scenery based on eye movement experiment: A case of Tangjia Ancient Town in Zhuhai. Human Geography. 2020 , 35 , 130–140. [ Google Scholar ] [ CrossRef ]
- Wu, C.J.; Min, Y.C.; Fei, B.H.; Song, S.S. A study on the aesthetic preference of bamboo weaving patterns based on eye movement experiments. Buildings 2023 , 13 , 1558. [ Google Scholar ] [ CrossRef ]
- Zhang, T.; Liu, D.D.; Zhang, H.W.; Huang, L.F. Landscape Aesthetic Experience of Longi Rice Terraces based on the Eye-Tracking Experiment. South Arch. 2023 , 2023 , 52–61. (In Chinese) [ Google Scholar ]
- Mead, W.R. The Iconography of Landscape: Essays on the Symbolic Representation, Design and Use of Past Environments ; Cambridge University Press: Cambridge, UK; Cosgrove, D., Daniels, S., Eds.; 1989; Volume 155, p. 129. [ Google Scholar ] [ CrossRef ]
- Wang, M. Eye Movement Analysis for Landscape Visual Quality Evaluation—A Case Study of Danxia Landform in Sunan, Gansu Province. Ph.D. Thesis, Nanjing University, Nanjing, China, 2011. [ Google Scholar ]
- Zhao, J.Z.; Yan, Y.; Deng, H.B.; Liu, G.H.; Dai, L.M.; Tang, L.N.; Shi, L.Y.; Shao, G.F. Remarks about land senses ecology and ecosystem services. Int. J. Sust. Dev. World 2020 , 27 , 196–201. [ Google Scholar ] [ CrossRef ]
- Treichler, D.G. Are you missing the boat in training aid? Film AV Comm. 1967 , 1 , 14–16. [ Google Scholar ]
- Amati, M.; Parmer, E.G.; McCarthy, C.; Sita, J. How eye-catching are natural features when walking through a park? Eye-tracking responses to videos of walks. Urban For. Urban Green. 2018 , 31 , 67–78. [ Google Scholar ] [ CrossRef ]
- Meidenbauer, L.K.; Stenfors, U.C.; Young, J.; Layden, E.A.; Schertz, K.E.; Kardan, O.; Decay, J.; Berman, M.G. The gradual development of the preference for natural environments. J. Environ. Psychol. 2019 , 65 , 101328. [ Google Scholar ] [ CrossRef ]
- Van Den Berg, A.E.; Vlei, C.A.J.; Couturier, J.F. Group differences in the aesthetic evaluation of nature development plans: A multilevel approach. J. Environ. Psychol. 1998 , 18 , 141–157. [ Google Scholar ] [ CrossRef ]
- Muro, R.S.T.; Granados, C.S.D.; Romero, E.J.R. Green infrastructures in the peri-urban landscape: Exploring local perception of well-being through ‘Go-Along’ and ‘Semi-Structured Interviews’. Sustainability 2020 , 12 , 6836. [ Google Scholar ] [ CrossRef ]
- Johnson, M.L.; Campbell, L.K.; Svendsen, E.S.; McMillen, H.L. Mapping urban park cultural ecosystem services: A comparison of twitter and semi-structured interview methods. Sustainability 2019 , 11 , 6137. [ Google Scholar ] [ CrossRef ]
Click here to enlarge figure
Group | Mann–Whitney U Test Results | |||||
---|---|---|---|---|---|---|
Average Fixation Points/No./Person | Z | p | Average Fixation Time/s/Person | Z | p | |
Villagers | 11.418 | −1.183 | 0.237 | 0.588 | −5.530 | 0.001 *** |
(8.800, 14.220) | (0.475, 0.898) | |||||
Tourists | 11.820 | 0.771 | ||||
(9.400, 13.680) | (0.568, 1.105) |
Element Type | Group | Mann–Whitney U Test Results | |||||
---|---|---|---|---|---|---|---|
Average Fixation Points/No./Person | Z | p | Average Fixation Time/s/Person | Z | p | ||
Landmark | Villagers | 11.628 (8.700, 14.300) | −0.462 | 0.644 | 0.621 (0.466, 1.045) | −4.820 | 0.001 *** |
Tourists | 11.464 (8.300, 13.800) | 0.803 (0.536, 1.147) | |||||
Edge | Villagers | 11.381 (8.200, 14.800) | −2.203 | 0.028 ** | 0.555 (0.428, 0.898) | −5.620 | 0.001 *** |
Tourists | 12.214 (9.900, 15.500) | 0.749 (0.505, 0.961) | |||||
District | Villagers | 11.583 (7.800, 15.300) | −0.873 | 0.383 | 0.604 (0.479, 0.923) | −4.156 | 0.001 *** |
Tourists | 11.894 (8.100, 14.200) | 0.778 (0.515, 1.431) | |||||
Node | Villagers | 11.211 (8.600, 14.900) | −1.933 | 0.053 | 0.556 (0.416, 0.878) | −5.451 | 0.001 *** |
Tourists | 11.933 (7.700, 14.500) | 0.762 (0.504, 1.164) | |||||
Path | Villagers | 11.289 (8.300, 13.900) | −0.975 | 0.770 | 0.603 (0.462, 0.973) | −3.367 | 0.001 *** |
Tourists | 11.594 (7.300, 15.000) | 0.764 (0.490, 1.652) |
Group | Kruskal–Wallis H Test Results | |||||
---|---|---|---|---|---|---|
Average Fixation Points/Number/Person | Average Fixation Time/Second/Person | |||||
M | SD | p | M | SD | p | |
Villagers | 11.418 | 1.642 | 0.742 | 0.588 | 0.129 | 0.019 ** |
Tourists | 11.820 | 1.572 | 0.347 | 0.711 | 0.191 | 0.365 |
Group | Element Type Sample Pairing | Average Fixation Points (Significance/p) | Average Fixation Time (Significance/p) |
---|---|---|---|
Villagers | Landmark-Edge | 0.471 | 0.009 ** |
Landmark-District | 0.973 | 0.224 | |
Landmark-Node | 0.248 | 0.003 ** | |
Landmark-Path | 0.373 | 0.392 | |
Edge-District | 0.554 | 0.156 | |
Edge-Node | 0.681 | 0.411 | |
Edge-Path | 0.933 | 0.220 | |
District-Node | 0.338 | 0.039 ** | |
District-Path | 0.389 | 0.884 | |
Node-Path | 0.791 | 0.080 | |
Tourists | Landmark-Edge | 0.039 ** | 0.215 |
Landmark-District | 0.164 | 0.173 | |
Landmark-Node | 0.162 | 0.260 | |
Landmark-Path | 0.408 | 0.057 | |
Edge-District | 0.800 | 0.937 | |
Edge-Node | 0.612 | 0.796 | |
Edge-Path | 0.282 | 0.417 | |
District-Node | 0.951 | 0.884 | |
District-Path | 0.414 | 0.417 | |
Node-Path | 0.681 | 0.305 |
The statements, opinions and data contained in all publications are solely those of the individual author(s) and contributor(s) and not of MDPI and/or the editor(s). MDPI and/or the editor(s) disclaim responsibility for any injury to people or property resulting from any ideas, methods, instructions or products referred to in the content. |
Share and Cite
Wang, Y.; Li, K.; Li, J.; Hao, T.; Zhou, Z. Comparative Study of Cognitive Differences in Rural Landscapes Based on Eye Movement Experiments. Land 2024 , 13 , 1592. https://doi.org/10.3390/land13101592
Wang Y, Li K, Li J, Hao T, Zhou Z. Comparative Study of Cognitive Differences in Rural Landscapes Based on Eye Movement Experiments. Land . 2024; 13(10):1592. https://doi.org/10.3390/land13101592
Wang, Yanbo, Kankan Li, Jiaxin Li, Tiange Hao, and Zhishu Zhou. 2024. "Comparative Study of Cognitive Differences in Rural Landscapes Based on Eye Movement Experiments" Land 13, no. 10: 1592. https://doi.org/10.3390/land13101592
Article Metrics
Further information, mdpi initiatives, follow mdpi.
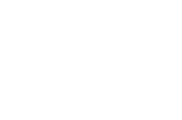
Subscribe to receive issue release notifications and newsletters from MDPI journals
Frequently asked questions
What’s the difference between correlational and experimental research.
Controlled experiments establish causality, whereas correlational studies only show associations between variables.
- In an experimental design , you manipulate an independent variable and measure its effect on a dependent variable. Other variables are controlled so they can’t impact the results.
- In a correlational design , you measure variables without manipulating any of them. You can test whether your variables change together, but you can’t be sure that one variable caused a change in another.
In general, correlational research is high in external validity while experimental research is high in internal validity .
Frequently asked questions: Methodology
Attrition refers to participants leaving a study. It always happens to some extent—for example, in randomized controlled trials for medical research.
Differential attrition occurs when attrition or dropout rates differ systematically between the intervention and the control group . As a result, the characteristics of the participants who drop out differ from the characteristics of those who stay in the study. Because of this, study results may be biased .
Action research is conducted in order to solve a particular issue immediately, while case studies are often conducted over a longer period of time and focus more on observing and analyzing a particular ongoing phenomenon.
Action research is focused on solving a problem or informing individual and community-based knowledge in a way that impacts teaching, learning, and other related processes. It is less focused on contributing theoretical input, instead producing actionable input.
Action research is particularly popular with educators as a form of systematic inquiry because it prioritizes reflection and bridges the gap between theory and practice. Educators are able to simultaneously investigate an issue as they solve it, and the method is very iterative and flexible.
A cycle of inquiry is another name for action research . It is usually visualized in a spiral shape following a series of steps, such as “planning → acting → observing → reflecting.”
To make quantitative observations , you need to use instruments that are capable of measuring the quantity you want to observe. For example, you might use a ruler to measure the length of an object or a thermometer to measure its temperature.
Criterion validity and construct validity are both types of measurement validity . In other words, they both show you how accurately a method measures something.
While construct validity is the degree to which a test or other measurement method measures what it claims to measure, criterion validity is the degree to which a test can predictively (in the future) or concurrently (in the present) measure something.
Construct validity is often considered the overarching type of measurement validity . You need to have face validity , content validity , and criterion validity in order to achieve construct validity.
Convergent validity and discriminant validity are both subtypes of construct validity . Together, they help you evaluate whether a test measures the concept it was designed to measure.
- Convergent validity indicates whether a test that is designed to measure a particular construct correlates with other tests that assess the same or similar construct.
- Discriminant validity indicates whether two tests that should not be highly related to each other are indeed not related. This type of validity is also called divergent validity .
You need to assess both in order to demonstrate construct validity. Neither one alone is sufficient for establishing construct validity.
- Discriminant validity indicates whether two tests that should not be highly related to each other are indeed not related
Content validity shows you how accurately a test or other measurement method taps into the various aspects of the specific construct you are researching.
In other words, it helps you answer the question: “does the test measure all aspects of the construct I want to measure?” If it does, then the test has high content validity.
The higher the content validity, the more accurate the measurement of the construct.
If the test fails to include parts of the construct, or irrelevant parts are included, the validity of the instrument is threatened, which brings your results into question.
Face validity and content validity are similar in that they both evaluate how suitable the content of a test is. The difference is that face validity is subjective, and assesses content at surface level.
When a test has strong face validity, anyone would agree that the test’s questions appear to measure what they are intended to measure.
For example, looking at a 4th grade math test consisting of problems in which students have to add and multiply, most people would agree that it has strong face validity (i.e., it looks like a math test).
On the other hand, content validity evaluates how well a test represents all the aspects of a topic. Assessing content validity is more systematic and relies on expert evaluation. of each question, analyzing whether each one covers the aspects that the test was designed to cover.
A 4th grade math test would have high content validity if it covered all the skills taught in that grade. Experts(in this case, math teachers), would have to evaluate the content validity by comparing the test to the learning objectives.
Snowball sampling is a non-probability sampling method . Unlike probability sampling (which involves some form of random selection ), the initial individuals selected to be studied are the ones who recruit new participants.
Because not every member of the target population has an equal chance of being recruited into the sample, selection in snowball sampling is non-random.
Snowball sampling is a non-probability sampling method , where there is not an equal chance for every member of the population to be included in the sample .
This means that you cannot use inferential statistics and make generalizations —often the goal of quantitative research . As such, a snowball sample is not representative of the target population and is usually a better fit for qualitative research .
Snowball sampling relies on the use of referrals. Here, the researcher recruits one or more initial participants, who then recruit the next ones.
Participants share similar characteristics and/or know each other. Because of this, not every member of the population has an equal chance of being included in the sample, giving rise to sampling bias .
Snowball sampling is best used in the following cases:
- If there is no sampling frame available (e.g., people with a rare disease)
- If the population of interest is hard to access or locate (e.g., people experiencing homelessness)
- If the research focuses on a sensitive topic (e.g., extramarital affairs)
The reproducibility and replicability of a study can be ensured by writing a transparent, detailed method section and using clear, unambiguous language.
Reproducibility and replicability are related terms.
- Reproducing research entails reanalyzing the existing data in the same manner.
- Replicating (or repeating ) the research entails reconducting the entire analysis, including the collection of new data .
- A successful reproduction shows that the data analyses were conducted in a fair and honest manner.
- A successful replication shows that the reliability of the results is high.
Stratified sampling and quota sampling both involve dividing the population into subgroups and selecting units from each subgroup. The purpose in both cases is to select a representative sample and/or to allow comparisons between subgroups.
The main difference is that in stratified sampling, you draw a random sample from each subgroup ( probability sampling ). In quota sampling you select a predetermined number or proportion of units, in a non-random manner ( non-probability sampling ).
Purposive and convenience sampling are both sampling methods that are typically used in qualitative data collection.
A convenience sample is drawn from a source that is conveniently accessible to the researcher. Convenience sampling does not distinguish characteristics among the participants. On the other hand, purposive sampling focuses on selecting participants possessing characteristics associated with the research study.
The findings of studies based on either convenience or purposive sampling can only be generalized to the (sub)population from which the sample is drawn, and not to the entire population.
Random sampling or probability sampling is based on random selection. This means that each unit has an equal chance (i.e., equal probability) of being included in the sample.
On the other hand, convenience sampling involves stopping people at random, which means that not everyone has an equal chance of being selected depending on the place, time, or day you are collecting your data.
Convenience sampling and quota sampling are both non-probability sampling methods. They both use non-random criteria like availability, geographical proximity, or expert knowledge to recruit study participants.
However, in convenience sampling, you continue to sample units or cases until you reach the required sample size.
In quota sampling, you first need to divide your population of interest into subgroups (strata) and estimate their proportions (quota) in the population. Then you can start your data collection, using convenience sampling to recruit participants, until the proportions in each subgroup coincide with the estimated proportions in the population.
A sampling frame is a list of every member in the entire population . It is important that the sampling frame is as complete as possible, so that your sample accurately reflects your population.
Stratified and cluster sampling may look similar, but bear in mind that groups created in cluster sampling are heterogeneous , so the individual characteristics in the cluster vary. In contrast, groups created in stratified sampling are homogeneous , as units share characteristics.
Relatedly, in cluster sampling you randomly select entire groups and include all units of each group in your sample. However, in stratified sampling, you select some units of all groups and include them in your sample. In this way, both methods can ensure that your sample is representative of the target population .
A systematic review is secondary research because it uses existing research. You don’t collect new data yourself.
The key difference between observational studies and experimental designs is that a well-done observational study does not influence the responses of participants, while experiments do have some sort of treatment condition applied to at least some participants by random assignment .
An observational study is a great choice for you if your research question is based purely on observations. If there are ethical, logistical, or practical concerns that prevent you from conducting a traditional experiment , an observational study may be a good choice. In an observational study, there is no interference or manipulation of the research subjects, as well as no control or treatment groups .
It’s often best to ask a variety of people to review your measurements. You can ask experts, such as other researchers, or laypeople, such as potential participants, to judge the face validity of tests.
While experts have a deep understanding of research methods , the people you’re studying can provide you with valuable insights you may have missed otherwise.
Face validity is important because it’s a simple first step to measuring the overall validity of a test or technique. It’s a relatively intuitive, quick, and easy way to start checking whether a new measure seems useful at first glance.
Good face validity means that anyone who reviews your measure says that it seems to be measuring what it’s supposed to. With poor face validity, someone reviewing your measure may be left confused about what you’re measuring and why you’re using this method.
Face validity is about whether a test appears to measure what it’s supposed to measure. This type of validity is concerned with whether a measure seems relevant and appropriate for what it’s assessing only on the surface.
Statistical analyses are often applied to test validity with data from your measures. You test convergent validity and discriminant validity with correlations to see if results from your test are positively or negatively related to those of other established tests.
You can also use regression analyses to assess whether your measure is actually predictive of outcomes that you expect it to predict theoretically. A regression analysis that supports your expectations strengthens your claim of construct validity .
When designing or evaluating a measure, construct validity helps you ensure you’re actually measuring the construct you’re interested in. If you don’t have construct validity, you may inadvertently measure unrelated or distinct constructs and lose precision in your research.
Construct validity is often considered the overarching type of measurement validity , because it covers all of the other types. You need to have face validity , content validity , and criterion validity to achieve construct validity.
Construct validity is about how well a test measures the concept it was designed to evaluate. It’s one of four types of measurement validity , which includes construct validity, face validity , and criterion validity.
There are two subtypes of construct validity.
- Convergent validity : The extent to which your measure corresponds to measures of related constructs
- Discriminant validity : The extent to which your measure is unrelated or negatively related to measures of distinct constructs
Naturalistic observation is a valuable tool because of its flexibility, external validity , and suitability for topics that can’t be studied in a lab setting.
The downsides of naturalistic observation include its lack of scientific control , ethical considerations , and potential for bias from observers and subjects.
Naturalistic observation is a qualitative research method where you record the behaviors of your research subjects in real world settings. You avoid interfering or influencing anything in a naturalistic observation.
You can think of naturalistic observation as “people watching” with a purpose.
A dependent variable is what changes as a result of the independent variable manipulation in experiments . It’s what you’re interested in measuring, and it “depends” on your independent variable.
In statistics, dependent variables are also called:
- Response variables (they respond to a change in another variable)
- Outcome variables (they represent the outcome you want to measure)
- Left-hand-side variables (they appear on the left-hand side of a regression equation)
An independent variable is the variable you manipulate, control, or vary in an experimental study to explore its effects. It’s called “independent” because it’s not influenced by any other variables in the study.
Independent variables are also called:
- Explanatory variables (they explain an event or outcome)
- Predictor variables (they can be used to predict the value of a dependent variable)
- Right-hand-side variables (they appear on the right-hand side of a regression equation).
As a rule of thumb, questions related to thoughts, beliefs, and feelings work well in focus groups. Take your time formulating strong questions, paying special attention to phrasing. Be careful to avoid leading questions , which can bias your responses.
Overall, your focus group questions should be:
- Open-ended and flexible
- Impossible to answer with “yes” or “no” (questions that start with “why” or “how” are often best)
- Unambiguous, getting straight to the point while still stimulating discussion
- Unbiased and neutral
A structured interview is a data collection method that relies on asking questions in a set order to collect data on a topic. They are often quantitative in nature. Structured interviews are best used when:
- You already have a very clear understanding of your topic. Perhaps significant research has already been conducted, or you have done some prior research yourself, but you already possess a baseline for designing strong structured questions.
- You are constrained in terms of time or resources and need to analyze your data quickly and efficiently.
- Your research question depends on strong parity between participants, with environmental conditions held constant.
More flexible interview options include semi-structured interviews , unstructured interviews , and focus groups .
Social desirability bias is the tendency for interview participants to give responses that will be viewed favorably by the interviewer or other participants. It occurs in all types of interviews and surveys , but is most common in semi-structured interviews , unstructured interviews , and focus groups .
Social desirability bias can be mitigated by ensuring participants feel at ease and comfortable sharing their views. Make sure to pay attention to your own body language and any physical or verbal cues, such as nodding or widening your eyes.
This type of bias can also occur in observations if the participants know they’re being observed. They might alter their behavior accordingly.
The interviewer effect is a type of bias that emerges when a characteristic of an interviewer (race, age, gender identity, etc.) influences the responses given by the interviewee.
There is a risk of an interviewer effect in all types of interviews , but it can be mitigated by writing really high-quality interview questions.
A semi-structured interview is a blend of structured and unstructured types of interviews. Semi-structured interviews are best used when:
- You have prior interview experience. Spontaneous questions are deceptively challenging, and it’s easy to accidentally ask a leading question or make a participant uncomfortable.
- Your research question is exploratory in nature. Participant answers can guide future research questions and help you develop a more robust knowledge base for future research.
An unstructured interview is the most flexible type of interview, but it is not always the best fit for your research topic.
Unstructured interviews are best used when:
- You are an experienced interviewer and have a very strong background in your research topic, since it is challenging to ask spontaneous, colloquial questions.
- Your research question is exploratory in nature. While you may have developed hypotheses, you are open to discovering new or shifting viewpoints through the interview process.
- You are seeking descriptive data, and are ready to ask questions that will deepen and contextualize your initial thoughts and hypotheses.
- Your research depends on forming connections with your participants and making them feel comfortable revealing deeper emotions, lived experiences, or thoughts.
The four most common types of interviews are:
- Structured interviews : The questions are predetermined in both topic and order.
- Semi-structured interviews : A few questions are predetermined, but other questions aren’t planned.
- Unstructured interviews : None of the questions are predetermined.
- Focus group interviews : The questions are presented to a group instead of one individual.
Deductive reasoning is commonly used in scientific research, and it’s especially associated with quantitative research .
In research, you might have come across something called the hypothetico-deductive method . It’s the scientific method of testing hypotheses to check whether your predictions are substantiated by real-world data.
Deductive reasoning is a logical approach where you progress from general ideas to specific conclusions. It’s often contrasted with inductive reasoning , where you start with specific observations and form general conclusions.
Deductive reasoning is also called deductive logic.
There are many different types of inductive reasoning that people use formally or informally.
Here are a few common types:
- Inductive generalization : You use observations about a sample to come to a conclusion about the population it came from.
- Statistical generalization: You use specific numbers about samples to make statements about populations.
- Causal reasoning: You make cause-and-effect links between different things.
- Sign reasoning: You make a conclusion about a correlational relationship between different things.
- Analogical reasoning: You make a conclusion about something based on its similarities to something else.
Inductive reasoning is a bottom-up approach, while deductive reasoning is top-down.
Inductive reasoning takes you from the specific to the general, while in deductive reasoning, you make inferences by going from general premises to specific conclusions.
In inductive research , you start by making observations or gathering data. Then, you take a broad scan of your data and search for patterns. Finally, you make general conclusions that you might incorporate into theories.
Inductive reasoning is a method of drawing conclusions by going from the specific to the general. It’s usually contrasted with deductive reasoning, where you proceed from general information to specific conclusions.
Inductive reasoning is also called inductive logic or bottom-up reasoning.
A hypothesis states your predictions about what your research will find. It is a tentative answer to your research question that has not yet been tested. For some research projects, you might have to write several hypotheses that address different aspects of your research question.
A hypothesis is not just a guess — it should be based on existing theories and knowledge. It also has to be testable, which means you can support or refute it through scientific research methods (such as experiments, observations and statistical analysis of data).
Triangulation can help:
- Reduce research bias that comes from using a single method, theory, or investigator
- Enhance validity by approaching the same topic with different tools
- Establish credibility by giving you a complete picture of the research problem
But triangulation can also pose problems:
- It’s time-consuming and labor-intensive, often involving an interdisciplinary team.
- Your results may be inconsistent or even contradictory.
There are four main types of triangulation :
- Data triangulation : Using data from different times, spaces, and people
- Investigator triangulation : Involving multiple researchers in collecting or analyzing data
- Theory triangulation : Using varying theoretical perspectives in your research
- Methodological triangulation : Using different methodologies to approach the same topic
Many academic fields use peer review , largely to determine whether a manuscript is suitable for publication. Peer review enhances the credibility of the published manuscript.
However, peer review is also common in non-academic settings. The United Nations, the European Union, and many individual nations use peer review to evaluate grant applications. It is also widely used in medical and health-related fields as a teaching or quality-of-care measure.
Peer assessment is often used in the classroom as a pedagogical tool. Both receiving feedback and providing it are thought to enhance the learning process, helping students think critically and collaboratively.
Peer review can stop obviously problematic, falsified, or otherwise untrustworthy research from being published. It also represents an excellent opportunity to get feedback from renowned experts in your field. It acts as a first defense, helping you ensure your argument is clear and that there are no gaps, vague terms, or unanswered questions for readers who weren’t involved in the research process.
Peer-reviewed articles are considered a highly credible source due to this stringent process they go through before publication.
In general, the peer review process follows the following steps:
- First, the author submits the manuscript to the editor.
- Reject the manuscript and send it back to author, or
- Send it onward to the selected peer reviewer(s)
- Next, the peer review process occurs. The reviewer provides feedback, addressing any major or minor issues with the manuscript, and gives their advice regarding what edits should be made.
- Lastly, the edited manuscript is sent back to the author. They input the edits, and resubmit it to the editor for publication.
Exploratory research is often used when the issue you’re studying is new or when the data collection process is challenging for some reason.
You can use exploratory research if you have a general idea or a specific question that you want to study but there is no preexisting knowledge or paradigm with which to study it.
Exploratory research is a methodology approach that explores research questions that have not previously been studied in depth. It is often used when the issue you’re studying is new, or the data collection process is challenging in some way.
Explanatory research is used to investigate how or why a phenomenon occurs. Therefore, this type of research is often one of the first stages in the research process , serving as a jumping-off point for future research.
Exploratory research aims to explore the main aspects of an under-researched problem, while explanatory research aims to explain the causes and consequences of a well-defined problem.
Explanatory research is a research method used to investigate how or why something occurs when only a small amount of information is available pertaining to that topic. It can help you increase your understanding of a given topic.
Clean data are valid, accurate, complete, consistent, unique, and uniform. Dirty data include inconsistencies and errors.
Dirty data can come from any part of the research process, including poor research design , inappropriate measurement materials, or flawed data entry.
Data cleaning takes place between data collection and data analyses. But you can use some methods even before collecting data.
For clean data, you should start by designing measures that collect valid data. Data validation at the time of data entry or collection helps you minimize the amount of data cleaning you’ll need to do.
After data collection, you can use data standardization and data transformation to clean your data. You’ll also deal with any missing values, outliers, and duplicate values.
Every dataset requires different techniques to clean dirty data , but you need to address these issues in a systematic way. You focus on finding and resolving data points that don’t agree or fit with the rest of your dataset.
These data might be missing values, outliers, duplicate values, incorrectly formatted, or irrelevant. You’ll start with screening and diagnosing your data. Then, you’ll often standardize and accept or remove data to make your dataset consistent and valid.
Data cleaning is necessary for valid and appropriate analyses. Dirty data contain inconsistencies or errors , but cleaning your data helps you minimize or resolve these.
Without data cleaning, you could end up with a Type I or II error in your conclusion. These types of erroneous conclusions can be practically significant with important consequences, because they lead to misplaced investments or missed opportunities.
Data cleaning involves spotting and resolving potential data inconsistencies or errors to improve your data quality. An error is any value (e.g., recorded weight) that doesn’t reflect the true value (e.g., actual weight) of something that’s being measured.
In this process, you review, analyze, detect, modify, or remove “dirty” data to make your dataset “clean.” Data cleaning is also called data cleansing or data scrubbing.
Research misconduct means making up or falsifying data, manipulating data analyses, or misrepresenting results in research reports. It’s a form of academic fraud.
These actions are committed intentionally and can have serious consequences; research misconduct is not a simple mistake or a point of disagreement but a serious ethical failure.
Anonymity means you don’t know who the participants are, while confidentiality means you know who they are but remove identifying information from your research report. Both are important ethical considerations .
You can only guarantee anonymity by not collecting any personally identifying information—for example, names, phone numbers, email addresses, IP addresses, physical characteristics, photos, or videos.
You can keep data confidential by using aggregate information in your research report, so that you only refer to groups of participants rather than individuals.
Research ethics matter for scientific integrity, human rights and dignity, and collaboration between science and society. These principles make sure that participation in studies is voluntary, informed, and safe.
Ethical considerations in research are a set of principles that guide your research designs and practices. These principles include voluntary participation, informed consent, anonymity, confidentiality, potential for harm, and results communication.
Scientists and researchers must always adhere to a certain code of conduct when collecting data from others .
These considerations protect the rights of research participants, enhance research validity , and maintain scientific integrity.
In multistage sampling , you can use probability or non-probability sampling methods .
For a probability sample, you have to conduct probability sampling at every stage.
You can mix it up by using simple random sampling , systematic sampling , or stratified sampling to select units at different stages, depending on what is applicable and relevant to your study.
Multistage sampling can simplify data collection when you have large, geographically spread samples, and you can obtain a probability sample without a complete sampling frame.
But multistage sampling may not lead to a representative sample, and larger samples are needed for multistage samples to achieve the statistical properties of simple random samples .
These are four of the most common mixed methods designs :
- Convergent parallel: Quantitative and qualitative data are collected at the same time and analyzed separately. After both analyses are complete, compare your results to draw overall conclusions.
- Embedded: Quantitative and qualitative data are collected at the same time, but within a larger quantitative or qualitative design. One type of data is secondary to the other.
- Explanatory sequential: Quantitative data is collected and analyzed first, followed by qualitative data. You can use this design if you think your qualitative data will explain and contextualize your quantitative findings.
- Exploratory sequential: Qualitative data is collected and analyzed first, followed by quantitative data. You can use this design if you think the quantitative data will confirm or validate your qualitative findings.
Triangulation in research means using multiple datasets, methods, theories and/or investigators to address a research question. It’s a research strategy that can help you enhance the validity and credibility of your findings.
Triangulation is mainly used in qualitative research , but it’s also commonly applied in quantitative research . Mixed methods research always uses triangulation.
In multistage sampling , or multistage cluster sampling, you draw a sample from a population using smaller and smaller groups at each stage.
This method is often used to collect data from a large, geographically spread group of people in national surveys, for example. You take advantage of hierarchical groupings (e.g., from state to city to neighborhood) to create a sample that’s less expensive and time-consuming to collect data from.
No, the steepness or slope of the line isn’t related to the correlation coefficient value. The correlation coefficient only tells you how closely your data fit on a line, so two datasets with the same correlation coefficient can have very different slopes.
To find the slope of the line, you’ll need to perform a regression analysis .
Correlation coefficients always range between -1 and 1.
The sign of the coefficient tells you the direction of the relationship: a positive value means the variables change together in the same direction, while a negative value means they change together in opposite directions.
The absolute value of a number is equal to the number without its sign. The absolute value of a correlation coefficient tells you the magnitude of the correlation: the greater the absolute value, the stronger the correlation.
These are the assumptions your data must meet if you want to use Pearson’s r :
- Both variables are on an interval or ratio level of measurement
- Data from both variables follow normal distributions
- Your data have no outliers
- Your data is from a random or representative sample
- You expect a linear relationship between the two variables
Quantitative research designs can be divided into two main categories:
- Correlational and descriptive designs are used to investigate characteristics, averages, trends, and associations between variables.
- Experimental and quasi-experimental designs are used to test causal relationships .
Qualitative research designs tend to be more flexible. Common types of qualitative design include case study , ethnography , and grounded theory designs.
A well-planned research design helps ensure that your methods match your research aims, that you collect high-quality data, and that you use the right kind of analysis to answer your questions, utilizing credible sources . This allows you to draw valid , trustworthy conclusions.
The priorities of a research design can vary depending on the field, but you usually have to specify:
- Your research questions and/or hypotheses
- Your overall approach (e.g., qualitative or quantitative )
- The type of design you’re using (e.g., a survey , experiment , or case study )
- Your sampling methods or criteria for selecting subjects
- Your data collection methods (e.g., questionnaires , observations)
- Your data collection procedures (e.g., operationalization , timing and data management)
- Your data analysis methods (e.g., statistical tests or thematic analysis )
A research design is a strategy for answering your research question . It defines your overall approach and determines how you will collect and analyze data.
Questionnaires can be self-administered or researcher-administered.
Self-administered questionnaires can be delivered online or in paper-and-pen formats, in person or through mail. All questions are standardized so that all respondents receive the same questions with identical wording.
Researcher-administered questionnaires are interviews that take place by phone, in-person, or online between researchers and respondents. You can gain deeper insights by clarifying questions for respondents or asking follow-up questions.
You can organize the questions logically, with a clear progression from simple to complex, or randomly between respondents. A logical flow helps respondents process the questionnaire easier and quicker, but it may lead to bias. Randomization can minimize the bias from order effects.
Closed-ended, or restricted-choice, questions offer respondents a fixed set of choices to select from. These questions are easier to answer quickly.
Open-ended or long-form questions allow respondents to answer in their own words. Because there are no restrictions on their choices, respondents can answer in ways that researchers may not have otherwise considered.
A questionnaire is a data collection tool or instrument, while a survey is an overarching research method that involves collecting and analyzing data from people using questionnaires.
The third variable and directionality problems are two main reasons why correlation isn’t causation .
The third variable problem means that a confounding variable affects both variables to make them seem causally related when they are not.
The directionality problem is when two variables correlate and might actually have a causal relationship, but it’s impossible to conclude which variable causes changes in the other.
Correlation describes an association between variables : when one variable changes, so does the other. A correlation is a statistical indicator of the relationship between variables.
Causation means that changes in one variable brings about changes in the other (i.e., there is a cause-and-effect relationship between variables). The two variables are correlated with each other, and there’s also a causal link between them.
While causation and correlation can exist simultaneously, correlation does not imply causation. In other words, correlation is simply a relationship where A relates to B—but A doesn’t necessarily cause B to happen (or vice versa). Mistaking correlation for causation is a common error and can lead to false cause fallacy .
A correlation is usually tested for two variables at a time, but you can test correlations between three or more variables.
A correlation coefficient is a single number that describes the strength and direction of the relationship between your variables.
Different types of correlation coefficients might be appropriate for your data based on their levels of measurement and distributions . The Pearson product-moment correlation coefficient (Pearson’s r ) is commonly used to assess a linear relationship between two quantitative variables.
A correlational research design investigates relationships between two variables (or more) without the researcher controlling or manipulating any of them. It’s a non-experimental type of quantitative research .
A correlation reflects the strength and/or direction of the association between two or more variables.
- A positive correlation means that both variables change in the same direction.
- A negative correlation means that the variables change in opposite directions.
- A zero correlation means there’s no relationship between the variables.
Random error is almost always present in scientific studies, even in highly controlled settings. While you can’t eradicate it completely, you can reduce random error by taking repeated measurements, using a large sample, and controlling extraneous variables .
You can avoid systematic error through careful design of your sampling , data collection , and analysis procedures. For example, use triangulation to measure your variables using multiple methods; regularly calibrate instruments or procedures; use random sampling and random assignment ; and apply masking (blinding) where possible.
Systematic error is generally a bigger problem in research.
With random error, multiple measurements will tend to cluster around the true value. When you’re collecting data from a large sample , the errors in different directions will cancel each other out.
Systematic errors are much more problematic because they can skew your data away from the true value. This can lead you to false conclusions ( Type I and II errors ) about the relationship between the variables you’re studying.
Random and systematic error are two types of measurement error.
Random error is a chance difference between the observed and true values of something (e.g., a researcher misreading a weighing scale records an incorrect measurement).
Systematic error is a consistent or proportional difference between the observed and true values of something (e.g., a miscalibrated scale consistently records weights as higher than they actually are).
On graphs, the explanatory variable is conventionally placed on the x-axis, while the response variable is placed on the y-axis.
- If you have quantitative variables , use a scatterplot or a line graph.
- If your response variable is categorical, use a scatterplot or a line graph.
- If your explanatory variable is categorical, use a bar graph.
The term “ explanatory variable ” is sometimes preferred over “ independent variable ” because, in real world contexts, independent variables are often influenced by other variables. This means they aren’t totally independent.
Multiple independent variables may also be correlated with each other, so “explanatory variables” is a more appropriate term.
The difference between explanatory and response variables is simple:
- An explanatory variable is the expected cause, and it explains the results.
- A response variable is the expected effect, and it responds to other variables.
In a controlled experiment , all extraneous variables are held constant so that they can’t influence the results. Controlled experiments require:
- A control group that receives a standard treatment, a fake treatment, or no treatment.
- Random assignment of participants to ensure the groups are equivalent.
Depending on your study topic, there are various other methods of controlling variables .
There are 4 main types of extraneous variables :
- Demand characteristics : environmental cues that encourage participants to conform to researchers’ expectations.
- Experimenter effects : unintentional actions by researchers that influence study outcomes.
- Situational variables : environmental variables that alter participants’ behaviors.
- Participant variables : any characteristic or aspect of a participant’s background that could affect study results.
An extraneous variable is any variable that you’re not investigating that can potentially affect the dependent variable of your research study.
A confounding variable is a type of extraneous variable that not only affects the dependent variable, but is also related to the independent variable.
In a factorial design, multiple independent variables are tested.
If you test two variables, each level of one independent variable is combined with each level of the other independent variable to create different conditions.
Within-subjects designs have many potential threats to internal validity , but they are also very statistically powerful .
Advantages:
- Only requires small samples
- Statistically powerful
- Removes the effects of individual differences on the outcomes
Disadvantages:
- Internal validity threats reduce the likelihood of establishing a direct relationship between variables
- Time-related effects, such as growth, can influence the outcomes
- Carryover effects mean that the specific order of different treatments affect the outcomes
While a between-subjects design has fewer threats to internal validity , it also requires more participants for high statistical power than a within-subjects design .
- Prevents carryover effects of learning and fatigue.
- Shorter study duration.
- Needs larger samples for high power.
- Uses more resources to recruit participants, administer sessions, cover costs, etc.
- Individual differences may be an alternative explanation for results.
Yes. Between-subjects and within-subjects designs can be combined in a single study when you have two or more independent variables (a factorial design). In a mixed factorial design, one variable is altered between subjects and another is altered within subjects.
In a between-subjects design , every participant experiences only one condition, and researchers assess group differences between participants in various conditions.
In a within-subjects design , each participant experiences all conditions, and researchers test the same participants repeatedly for differences between conditions.
The word “between” means that you’re comparing different conditions between groups, while the word “within” means you’re comparing different conditions within the same group.
Random assignment is used in experiments with a between-groups or independent measures design. In this research design, there’s usually a control group and one or more experimental groups. Random assignment helps ensure that the groups are comparable.
In general, you should always use random assignment in this type of experimental design when it is ethically possible and makes sense for your study topic.
To implement random assignment , assign a unique number to every member of your study’s sample .
Then, you can use a random number generator or a lottery method to randomly assign each number to a control or experimental group. You can also do so manually, by flipping a coin or rolling a dice to randomly assign participants to groups.
Random selection, or random sampling , is a way of selecting members of a population for your study’s sample.
In contrast, random assignment is a way of sorting the sample into control and experimental groups.
Random sampling enhances the external validity or generalizability of your results, while random assignment improves the internal validity of your study.
In experimental research, random assignment is a way of placing participants from your sample into different groups using randomization. With this method, every member of the sample has a known or equal chance of being placed in a control group or an experimental group.
“Controlling for a variable” means measuring extraneous variables and accounting for them statistically to remove their effects on other variables.
Researchers often model control variable data along with independent and dependent variable data in regression analyses and ANCOVAs . That way, you can isolate the control variable’s effects from the relationship between the variables of interest.
Control variables help you establish a correlational or causal relationship between variables by enhancing internal validity .
If you don’t control relevant extraneous variables , they may influence the outcomes of your study, and you may not be able to demonstrate that your results are really an effect of your independent variable .
A control variable is any variable that’s held constant in a research study. It’s not a variable of interest in the study, but it’s controlled because it could influence the outcomes.
Including mediators and moderators in your research helps you go beyond studying a simple relationship between two variables for a fuller picture of the real world. They are important to consider when studying complex correlational or causal relationships.
Mediators are part of the causal pathway of an effect, and they tell you how or why an effect takes place. Moderators usually help you judge the external validity of your study by identifying the limitations of when the relationship between variables holds.
If something is a mediating variable :
- It’s caused by the independent variable .
- It influences the dependent variable
- When it’s taken into account, the statistical correlation between the independent and dependent variables is higher than when it isn’t considered.
A confounder is a third variable that affects variables of interest and makes them seem related when they are not. In contrast, a mediator is the mechanism of a relationship between two variables: it explains the process by which they are related.
A mediator variable explains the process through which two variables are related, while a moderator variable affects the strength and direction of that relationship.
There are three key steps in systematic sampling :
- Define and list your population , ensuring that it is not ordered in a cyclical or periodic order.
- Decide on your sample size and calculate your interval, k , by dividing your population by your target sample size.
- Choose every k th member of the population as your sample.
Systematic sampling is a probability sampling method where researchers select members of the population at a regular interval – for example, by selecting every 15th person on a list of the population. If the population is in a random order, this can imitate the benefits of simple random sampling .
Yes, you can create a stratified sample using multiple characteristics, but you must ensure that every participant in your study belongs to one and only one subgroup. In this case, you multiply the numbers of subgroups for each characteristic to get the total number of groups.
For example, if you were stratifying by location with three subgroups (urban, rural, or suburban) and marital status with five subgroups (single, divorced, widowed, married, or partnered), you would have 3 x 5 = 15 subgroups.
You should use stratified sampling when your sample can be divided into mutually exclusive and exhaustive subgroups that you believe will take on different mean values for the variable that you’re studying.
Using stratified sampling will allow you to obtain more precise (with lower variance ) statistical estimates of whatever you are trying to measure.
For example, say you want to investigate how income differs based on educational attainment, but you know that this relationship can vary based on race. Using stratified sampling, you can ensure you obtain a large enough sample from each racial group, allowing you to draw more precise conclusions.
In stratified sampling , researchers divide subjects into subgroups called strata based on characteristics that they share (e.g., race, gender, educational attainment).
Once divided, each subgroup is randomly sampled using another probability sampling method.
Cluster sampling is more time- and cost-efficient than other probability sampling methods , particularly when it comes to large samples spread across a wide geographical area.
However, it provides less statistical certainty than other methods, such as simple random sampling , because it is difficult to ensure that your clusters properly represent the population as a whole.
There are three types of cluster sampling : single-stage, double-stage and multi-stage clustering. In all three types, you first divide the population into clusters, then randomly select clusters for use in your sample.
- In single-stage sampling , you collect data from every unit within the selected clusters.
- In double-stage sampling , you select a random sample of units from within the clusters.
- In multi-stage sampling , you repeat the procedure of randomly sampling elements from within the clusters until you have reached a manageable sample.
Cluster sampling is a probability sampling method in which you divide a population into clusters, such as districts or schools, and then randomly select some of these clusters as your sample.
The clusters should ideally each be mini-representations of the population as a whole.
If properly implemented, simple random sampling is usually the best sampling method for ensuring both internal and external validity . However, it can sometimes be impractical and expensive to implement, depending on the size of the population to be studied,
If you have a list of every member of the population and the ability to reach whichever members are selected, you can use simple random sampling.
The American Community Survey is an example of simple random sampling . In order to collect detailed data on the population of the US, the Census Bureau officials randomly select 3.5 million households per year and use a variety of methods to convince them to fill out the survey.
Simple random sampling is a type of probability sampling in which the researcher randomly selects a subset of participants from a population . Each member of the population has an equal chance of being selected. Data is then collected from as large a percentage as possible of this random subset.
Quasi-experimental design is most useful in situations where it would be unethical or impractical to run a true experiment .
Quasi-experiments have lower internal validity than true experiments, but they often have higher external validity as they can use real-world interventions instead of artificial laboratory settings.
A quasi-experiment is a type of research design that attempts to establish a cause-and-effect relationship. The main difference with a true experiment is that the groups are not randomly assigned.
Blinding is important to reduce research bias (e.g., observer bias , demand characteristics ) and ensure a study’s internal validity .
If participants know whether they are in a control or treatment group , they may adjust their behavior in ways that affect the outcome that researchers are trying to measure. If the people administering the treatment are aware of group assignment, they may treat participants differently and thus directly or indirectly influence the final results.
- In a single-blind study , only the participants are blinded.
- In a double-blind study , both participants and experimenters are blinded.
- In a triple-blind study , the assignment is hidden not only from participants and experimenters, but also from the researchers analyzing the data.
Blinding means hiding who is assigned to the treatment group and who is assigned to the control group in an experiment .
A true experiment (a.k.a. a controlled experiment) always includes at least one control group that doesn’t receive the experimental treatment.
However, some experiments use a within-subjects design to test treatments without a control group. In these designs, you usually compare one group’s outcomes before and after a treatment (instead of comparing outcomes between different groups).
For strong internal validity , it’s usually best to include a control group if possible. Without a control group, it’s harder to be certain that the outcome was caused by the experimental treatment and not by other variables.
An experimental group, also known as a treatment group, receives the treatment whose effect researchers wish to study, whereas a control group does not. They should be identical in all other ways.
Individual Likert-type questions are generally considered ordinal data , because the items have clear rank order, but don’t have an even distribution.
Overall Likert scale scores are sometimes treated as interval data. These scores are considered to have directionality and even spacing between them.
The type of data determines what statistical tests you should use to analyze your data.
A Likert scale is a rating scale that quantitatively assesses opinions, attitudes, or behaviors. It is made up of 4 or more questions that measure a single attitude or trait when response scores are combined.
To use a Likert scale in a survey , you present participants with Likert-type questions or statements, and a continuum of items, usually with 5 or 7 possible responses, to capture their degree of agreement.
In scientific research, concepts are the abstract ideas or phenomena that are being studied (e.g., educational achievement). Variables are properties or characteristics of the concept (e.g., performance at school), while indicators are ways of measuring or quantifying variables (e.g., yearly grade reports).
The process of turning abstract concepts into measurable variables and indicators is called operationalization .
There are various approaches to qualitative data analysis , but they all share five steps in common:
- Prepare and organize your data.
- Review and explore your data.
- Develop a data coding system.
- Assign codes to the data.
- Identify recurring themes.
The specifics of each step depend on the focus of the analysis. Some common approaches include textual analysis , thematic analysis , and discourse analysis .
There are five common approaches to qualitative research :
- Grounded theory involves collecting data in order to develop new theories.
- Ethnography involves immersing yourself in a group or organization to understand its culture.
- Narrative research involves interpreting stories to understand how people make sense of their experiences and perceptions.
- Phenomenological research involves investigating phenomena through people’s lived experiences.
- Action research links theory and practice in several cycles to drive innovative changes.
Hypothesis testing is a formal procedure for investigating our ideas about the world using statistics. It is used by scientists to test specific predictions, called hypotheses , by calculating how likely it is that a pattern or relationship between variables could have arisen by chance.
Operationalization means turning abstract conceptual ideas into measurable observations.
For example, the concept of social anxiety isn’t directly observable, but it can be operationally defined in terms of self-rating scores, behavioral avoidance of crowded places, or physical anxiety symptoms in social situations.
Before collecting data , it’s important to consider how you will operationalize the variables that you want to measure.
When conducting research, collecting original data has significant advantages:
- You can tailor data collection to your specific research aims (e.g. understanding the needs of your consumers or user testing your website)
- You can control and standardize the process for high reliability and validity (e.g. choosing appropriate measurements and sampling methods )
However, there are also some drawbacks: data collection can be time-consuming, labor-intensive and expensive. In some cases, it’s more efficient to use secondary data that has already been collected by someone else, but the data might be less reliable.
Data collection is the systematic process by which observations or measurements are gathered in research. It is used in many different contexts by academics, governments, businesses, and other organizations.
There are several methods you can use to decrease the impact of confounding variables on your research: restriction, matching, statistical control and randomization.
In restriction , you restrict your sample by only including certain subjects that have the same values of potential confounding variables.
In matching , you match each of the subjects in your treatment group with a counterpart in the comparison group. The matched subjects have the same values on any potential confounding variables, and only differ in the independent variable .
In statistical control , you include potential confounders as variables in your regression .
In randomization , you randomly assign the treatment (or independent variable) in your study to a sufficiently large number of subjects, which allows you to control for all potential confounding variables.
A confounding variable is closely related to both the independent and dependent variables in a study. An independent variable represents the supposed cause , while the dependent variable is the supposed effect . A confounding variable is a third variable that influences both the independent and dependent variables.
Failing to account for confounding variables can cause you to wrongly estimate the relationship between your independent and dependent variables.
To ensure the internal validity of your research, you must consider the impact of confounding variables. If you fail to account for them, you might over- or underestimate the causal relationship between your independent and dependent variables , or even find a causal relationship where none exists.
Yes, but including more than one of either type requires multiple research questions .
For example, if you are interested in the effect of a diet on health, you can use multiple measures of health: blood sugar, blood pressure, weight, pulse, and many more. Each of these is its own dependent variable with its own research question.
You could also choose to look at the effect of exercise levels as well as diet, or even the additional effect of the two combined. Each of these is a separate independent variable .
To ensure the internal validity of an experiment , you should only change one independent variable at a time.
No. The value of a dependent variable depends on an independent variable, so a variable cannot be both independent and dependent at the same time. It must be either the cause or the effect, not both!
You want to find out how blood sugar levels are affected by drinking diet soda and regular soda, so you conduct an experiment .
- The type of soda – diet or regular – is the independent variable .
- The level of blood sugar that you measure is the dependent variable – it changes depending on the type of soda.
Determining cause and effect is one of the most important parts of scientific research. It’s essential to know which is the cause – the independent variable – and which is the effect – the dependent variable.
In non-probability sampling , the sample is selected based on non-random criteria, and not every member of the population has a chance of being included.
Common non-probability sampling methods include convenience sampling , voluntary response sampling, purposive sampling , snowball sampling, and quota sampling .
Probability sampling means that every member of the target population has a known chance of being included in the sample.
Probability sampling methods include simple random sampling , systematic sampling , stratified sampling , and cluster sampling .
Using careful research design and sampling procedures can help you avoid sampling bias . Oversampling can be used to correct undercoverage bias .
Some common types of sampling bias include self-selection bias , nonresponse bias , undercoverage bias , survivorship bias , pre-screening or advertising bias, and healthy user bias.
Sampling bias is a threat to external validity – it limits the generalizability of your findings to a broader group of people.
A sampling error is the difference between a population parameter and a sample statistic .
A statistic refers to measures about the sample , while a parameter refers to measures about the population .
Populations are used when a research question requires data from every member of the population. This is usually only feasible when the population is small and easily accessible.
Samples are used to make inferences about populations . Samples are easier to collect data from because they are practical, cost-effective, convenient, and manageable.
There are seven threats to external validity : selection bias , history, experimenter effect, Hawthorne effect , testing effect, aptitude-treatment and situation effect.
The two types of external validity are population validity (whether you can generalize to other groups of people) and ecological validity (whether you can generalize to other situations and settings).
The external validity of a study is the extent to which you can generalize your findings to different groups of people, situations, and measures.
Cross-sectional studies cannot establish a cause-and-effect relationship or analyze behavior over a period of time. To investigate cause and effect, you need to do a longitudinal study or an experimental study .
Cross-sectional studies are less expensive and time-consuming than many other types of study. They can provide useful insights into a population’s characteristics and identify correlations for further research.
Sometimes only cross-sectional data is available for analysis; other times your research question may only require a cross-sectional study to answer it.
Longitudinal studies can last anywhere from weeks to decades, although they tend to be at least a year long.
The 1970 British Cohort Study , which has collected data on the lives of 17,000 Brits since their births in 1970, is one well-known example of a longitudinal study .
Longitudinal studies are better to establish the correct sequence of events, identify changes over time, and provide insight into cause-and-effect relationships, but they also tend to be more expensive and time-consuming than other types of studies.
Longitudinal studies and cross-sectional studies are two different types of research design . In a cross-sectional study you collect data from a population at a specific point in time; in a longitudinal study you repeatedly collect data from the same sample over an extended period of time.
Longitudinal study | Cross-sectional study |
---|---|
observations | Observations at a in time |
Observes the multiple times | Observes (a “cross-section”) in the population |
Follows in participants over time | Provides of society at a given point |
There are eight threats to internal validity : history, maturation, instrumentation, testing, selection bias , regression to the mean, social interaction and attrition .
Internal validity is the extent to which you can be confident that a cause-and-effect relationship established in a study cannot be explained by other factors.
In mixed methods research , you use both qualitative and quantitative data collection and analysis methods to answer your research question .
The research methods you use depend on the type of data you need to answer your research question .
- If you want to measure something or test a hypothesis , use quantitative methods . If you want to explore ideas, thoughts and meanings, use qualitative methods .
- If you want to analyze a large amount of readily-available data, use secondary data. If you want data specific to your purposes with control over how it is generated, collect primary data.
- If you want to establish cause-and-effect relationships between variables , use experimental methods. If you want to understand the characteristics of a research subject, use descriptive methods.
A confounding variable , also called a confounder or confounding factor, is a third variable in a study examining a potential cause-and-effect relationship.
A confounding variable is related to both the supposed cause and the supposed effect of the study. It can be difficult to separate the true effect of the independent variable from the effect of the confounding variable.
In your research design , it’s important to identify potential confounding variables and plan how you will reduce their impact.
Discrete and continuous variables are two types of quantitative variables :
- Discrete variables represent counts (e.g. the number of objects in a collection).
- Continuous variables represent measurable amounts (e.g. water volume or weight).
Quantitative variables are any variables where the data represent amounts (e.g. height, weight, or age).
Categorical variables are any variables where the data represent groups. This includes rankings (e.g. finishing places in a race), classifications (e.g. brands of cereal), and binary outcomes (e.g. coin flips).
You need to know what type of variables you are working with to choose the right statistical test for your data and interpret your results .
You can think of independent and dependent variables in terms of cause and effect: an independent variable is the variable you think is the cause , while a dependent variable is the effect .
In an experiment, you manipulate the independent variable and measure the outcome in the dependent variable. For example, in an experiment about the effect of nutrients on crop growth:
- The independent variable is the amount of nutrients added to the crop field.
- The dependent variable is the biomass of the crops at harvest time.
Defining your variables, and deciding how you will manipulate and measure them, is an important part of experimental design .
Experimental design means planning a set of procedures to investigate a relationship between variables . To design a controlled experiment, you need:
- A testable hypothesis
- At least one independent variable that can be precisely manipulated
- At least one dependent variable that can be precisely measured
When designing the experiment, you decide:
- How you will manipulate the variable(s)
- How you will control for any potential confounding variables
- How many subjects or samples will be included in the study
- How subjects will be assigned to treatment levels
Experimental design is essential to the internal and external validity of your experiment.
I nternal validity is the degree of confidence that the causal relationship you are testing is not influenced by other factors or variables .
External validity is the extent to which your results can be generalized to other contexts.
The validity of your experiment depends on your experimental design .
Reliability and validity are both about how well a method measures something:
- Reliability refers to the consistency of a measure (whether the results can be reproduced under the same conditions).
- Validity refers to the accuracy of a measure (whether the results really do represent what they are supposed to measure).
If you are doing experimental research, you also have to consider the internal and external validity of your experiment.
A sample is a subset of individuals from a larger population . Sampling means selecting the group that you will actually collect data from in your research. For example, if you are researching the opinions of students in your university, you could survey a sample of 100 students.
In statistics, sampling allows you to test a hypothesis about the characteristics of a population.
Quantitative research deals with numbers and statistics, while qualitative research deals with words and meanings.
Quantitative methods allow you to systematically measure variables and test hypotheses . Qualitative methods allow you to explore concepts and experiences in more detail.
Methodology refers to the overarching strategy and rationale of your research project . It involves studying the methods used in your field and the theories or principles behind them, in order to develop an approach that matches your objectives.
Methods are the specific tools and procedures you use to collect and analyze data (for example, experiments, surveys , and statistical tests ).
In shorter scientific papers, where the aim is to report the findings of a specific study, you might simply describe what you did in a methods section .
In a longer or more complex research project, such as a thesis or dissertation , you will probably include a methodology section , where you explain your approach to answering the research questions and cite relevant sources to support your choice of methods.
Ask our team
Want to contact us directly? No problem. We are always here for you.
- Email [email protected]
- Start live chat
- Call +1 (510) 822-8066
- WhatsApp +31 20 261 6040
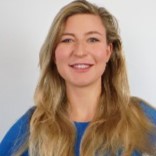
Our team helps students graduate by offering:
- A world-class citation generator
- Plagiarism Checker software powered by Turnitin
- Innovative Citation Checker software
- Professional proofreading services
- Over 300 helpful articles about academic writing, citing sources, plagiarism, and more
Scribbr specializes in editing study-related documents . We proofread:
- PhD dissertations
- Research proposals
- Personal statements
- Admission essays
- Motivation letters
- Reflection papers
- Journal articles
- Capstone projects
Scribbr’s Plagiarism Checker is powered by elements of Turnitin’s Similarity Checker , namely the plagiarism detection software and the Internet Archive and Premium Scholarly Publications content databases .
The add-on AI detector is powered by Scribbr’s proprietary software.
The Scribbr Citation Generator is developed using the open-source Citation Style Language (CSL) project and Frank Bennett’s citeproc-js . It’s the same technology used by dozens of other popular citation tools, including Mendeley and Zotero.
You can find all the citation styles and locales used in the Scribbr Citation Generator in our publicly accessible repository on Github .
Exploring the differences in syntactic complexity between lay summaries and abstracts: A case study of The New England Journal of Medicine
- September 2024
- Journal of English for Academic Purposes 72:101444

- South China Normal University

- Pennsylvania State University
- This person is not on ResearchGate, or hasn't claimed this research yet.
Discover the world's research
- 25+ million members
- 160+ million publication pages
- 2.3+ billion citations
No full-text available

To read the full-text of this research, you can request a copy directly from the authors.

- Douglas Biber

- Xiaopeng Zhang

- INFORM PROCESS MANAG

- Tiantian Li

- Danielle Marie Muscat

- Anita Chasiotis
- NEW ENGL J MED

- Caitlin Edgell
- Adeline Rosenberg
- BEHAV RES METHODS
- Aron Heintz,

- Agnes Malatinszky
- Scott A. Crossley
- J MED INTERNET RES
- Leia Martínez Silvagnoli
- Caroline Shepherd

- Jason Gardner

- Armin Günther

- J INFORMETR

- J CLIN EPIDEMIOL

- Elizabeth Wager

- ENGL SPECIF PURP

- Emily Pitler

- Inge Alferink

- Xiaohu Yang
- Frances Shiely

- Christine Feak
- Bethany Gray
- J CYST FIBROS
- Hannah L Anderson
- John E Moore

- Maryam Nasseri
- Julie Pallant

- Kristopher Kyle

- Charlene Polio
- Gunther Kress
- Theo V Van Leeuwen
- J SECOND LANG WRIT

- George R. Klare

- Recruit researchers
- Join for free
- Login Email Tip: Most researchers use their institutional email address as their ResearchGate login Password Forgot password? Keep me logged in Log in or Continue with Google Welcome back! Please log in. Email · Hint Tip: Most researchers use their institutional email address as their ResearchGate login Password Forgot password? Keep me logged in Log in or Continue with Google No account? Sign up
- Open access
- Published: 30 September 2024
Genome-wide comparative analysis of CC1 Staphylococcus aureus between colonization and infection
- Feifei Gu 1 na1 ,
- Weiping He 2 na1 ,
- Dedong Zhu 1 ,
- Qian Zeng 1 ,
- Xinxin Li 3 ,
- Shuzhen Xiao 1 ,
- Yuxing Ni 1 &
- Lizhong Han 1
European Journal of Medical Research volume 29 , Article number: 474 ( 2024 ) Cite this article
Metrics details
Staphylococcus aureus is one of the most important bacteria in human colonization and infection. Clonal complex1 (CC1) is one of the largest and most important S. aureus CCs, and it is a predominant clone in S. aureus colonization and can cause a series of S. aureus infections including bloodstream infections. No studies on the relationship of CC1 S. aureus between colonization and infection have been published.
To figure out if there are some significant factors in CC1 S. aureus help its colonization or infection, 15 CC1 S. aureus isolates including ten from colonization and five from bloodstream infections were enrolled in this study. Whole-genome sequencing and bioinformatics analysis were performed.
Virulence factor regulators XdrA, YSIRK signal peptide, CPBP family and OmpR family specifically found in infection isolates can promote virulence factors and enhance the pathogenicity of S. aureus . In addition, some significant differences in metabolism and human diseases were discovered between colonization and infection. Fst family of type I toxin–antitoxin system that mainly maintains stable inheritance was specifically found in CC1 S. aureus colonization isolates and might help S. aureus survive for colonization. No significant differences in genomic evolutionary relationship were found among CC1 S. aureus isolates between colonization and infection.
Conclusions
Virulence factor regulators and metabolic state can promote CC1 S. aureus pathogenic process compared with colonization, and it seems that the strains of colonization origin cannot have pathogenic potential. Experimental confirmation and a bigger number of CC1 S. aureus strains are necessary for further study about the details and mechanism between colonization and infection.
Introduction
Staphylococcus aureus is an opportunistic pathogen that can colonize any part of the integument or mucous membranes, and nares is the most common site of colonization with approximately 30% of individuals permanently colonized [ 1 ]. Furthermore, S. aureus is a leading cause of bloodstream infections (BSIs), infective endocarditis as well as osteoarticular, skin and soft tissue, pleuropulmonary, and device-related infections [ 2 ]. Staphylococcus aureus causes the vast majority of skin and soft tissue infections (SSTIs), and it usually progresses from asymptomatic colonization [ 3 ]. SSTIs can result in a range of severity from simple subcutaneous abscesses to severe necrotizing infections, and it may be both limb and life threatening as a potential source for bacteremia and metastatic abscesses [ 4 ]. The associations of colonization in different body sites with invasive infection have been proved in several studies [ 5 ]. It has been demonstrated that nasal S. aureus carriage might be a source of bacteremia; a substantial proportion of S. aureus bacteremia cases appear to be endogenous, since they originate from S. aureus colonized in nasal mucosa [ 6 ].
Nursing homes (NHs) for the elderly have been regarded as significant reservoirs for S. aureus and might play important roles in the spread of S. aureus in closed populations. It has been confirmed that the advanced aged were prone to intermittent S. aureus carriage, and the transmission of S. aureus among the participants living in a closed environment was revealed with a high genetic relatedness, suggesting its clonal spread in nursing homes [ 7 ]. A high prevalence of S. aureus colonization has been proved among nursing home residents in Shanghai in our previous studies, and clonal complex 1 (CC1) was the predominant clone among S. aureus- colonized residents both in 2014 (29.1%) and 2019 (36.3%) [ 8 , 9 ].
CC1 was previously one of the five largest and most important S. aureus CCs including CC1, CC5, CC8, CC15 and CC97, and CC1 is known to contain various community-associated methicillin-resistant S. aureus (CA-MRSA) including the first PVL-positive strain MW2 [ 10 ]. Multilocus sequence type 1 (ST1), also known as pulsed-field type USA400, were among the most prominent CA-MRSA clone in the USA, and recently, ST1 has been sporadically reported in other regions, including China [ 11 ]. CC1 has been found as a dominant clone (86.0%) of MRSA in the fecal and nasal specimens from neonatal intensive care units (NICUs) patients in Japan [ 12 ], revealing a high prevalence of CC1 MRSA colonization and a threatening risk of MRSA infection remaining in NICU patients despite of appropriate control measures. CC1 has been recognized as a somewhat problematic clone in Europe as the clone European CC1-MRSA IV in recent years, and disseminated rapidly throughout Europe [ 13 ]. CC1 was still an infection clone until now found in a range of S. aureus infections even invasive infections and BSIs all over the world including in neonates in China [ 14 , 15 , 16 , 17 , 18 , 19 , 20 , 21 , 22 ]. In our latest study, CC1 was still found as an infection clone in MRSA burn wound infection in southeast China from 2013 to 2022 [ 23 ].
Considering the high prevalence of CC1 clone in S. aureus colonization and its importance in S. aureus infections, whether there is something special in CC1 clone might help its progress in colonization or infection is what we are concerned about. Unfortunately, there are no published studies on the CC1 S. aureus between colonization and infection. The transmission and spread of CC1 S. aureus might occur from colonization to infection with the high colonization prevalence of CC1 S. aureus . The aim of our study is to figure out if there is something significant in CC1 S. aureus between colonization and infection to aid their processes. The differences between CC1 S. aureus colonization and infection could help us understand which ones can help CC1 S. aureus colonize or enhance pathogenic potential and lead to infections. The factors specifically identified in colonization or infection may contribute to the development of control strategies regarding decolonization or infection prevention.
Materials and methods
Study isolates.
Based on our previous studies on S. aureus colonization among NH residents in Shanghai, five CC1 S. aureus isolates in 2014 and five in 2019 refer to S. aureus colonization were enrolled in this study named G2014 (group 2014) and G2019 (group 2019), respectively. These ten CC1 S. aureus isolates in total were named GCOL (group colonization).
S. aureus isolates refer to infection were collected from S. aureus bloodstream infections from 2017 to 2019 in our previous studies. Five CC1 S. aureus isolates causing bloodstream infection were enrolled in this study named GBSI (group bloodstream infection). CC1 is not common in S. aureus bloodstream infection as other clones, and a limited number of five CC1 S. aureus infection isolates were all we found in S. aureus bloodstream infection in Shanghai during these years.
This study was approved by Ethics Committee of Ruijin Hospital affiliated to Shanghai Jiao Tong University School of Medicine. The strains enrolled in this study were collected from our previous studies [ 8 , 9 , 17 ] which all have been approved by Ethics Committee of Ruijin Hospital affiliated to Shanghai Jiao Tong University School of Medicine, and in this study we only focused on the bacteria without any more intervention. All methods in this study were carried out in accordance with relevant guidelines and regulations.
MLST and spa typing
Multilocus sequence typing (MLST) and spa typing were performed by polymerase chain reaction (PCR) on all S. aureus isolates collected according to the guidelines ( https://pubmlst.org/ , http://spa.ridom.de/index.shtml ). CC1 and spa type t127 S. aureus isolates were identified, and 15 isolates were included for whole-genome sequencing in this study.
mecA detection and SCC mec typing
MRSA was screened by cefoxitin (30 μg) using the disk diffusion method following the Clinical and Laboratory Standards Institute guidelines [ 24 ]. mecA detection was performed on all S. aureus isolates to confirm the existence of MRSA. SCC mec types of MRSA were determined by the method as described previously [ 25 ].
Genomic DNA extraction
The CC1 S. aureus strains were streaked on Columbia blood agar and cultured at 37 ℃ overnight. A single colony on the plate was inoculated into 50 ml of LB liquid medium and cultured at 37 ℃ for approximately 16 h at 200 rpm. The cell biomass was harvested after 10 min centrifugation at 4000 rmp. Genomic DNA of the strains was extracted by Wizard® Genomic DNA Purification Kit (Promega), and purified genomic DNA was quantified by TBS-380 fluorometer (Turner BioSystems Inc., Sunnyvale, CA). High quality DNA (OD260/280 = 1.8–2.0, > 1 μg) was used for further research.
Library construction and genome sequencing
The draft genome sequence analysis was carried out using the Hiseq X Ten sequencing platform (MajorBio Co., Shanghai, China). Briefly, DNA samples were sheared into 400–500 bp fragments using a Covaris M220 Focused Acoustic Shearer according to the manufacture’s protocol. Illumina sequencing libraries were prepared from the sheared fragments using the NEXTflex™ Rapid DNA-Seq Kit. 5′ primer ends were first end-repaired and phosphorylated. Next, the 3′ ends were A-tailed and ligated to sequencing adapters. The third step is to enrich the adapters-ligated products using PCR. The prepared libraries then were used for paired-end Illumina sequencing (2 × 150 bp) on an Illumina HiSeq X Ten sequencing platform.
Genome assembly and annotation
The data generated from Illumina platform were used for genomic analysis. The detailed procedures of genome assembly and annotation are as follows. Raw reads obtained after sequencing were filtered using a fastp software (version 0.19.6) [ 26 ] followed by assembly with SOPA de novo version 2.04. [ 27 ]. The final assembled genomes were submitted to GenBank with the accession number PRJNA847504. Glimmer [ 28 ] was used for CDS prediction, tRNA-scan-SE was used for tRNA prediction and Barrnap was used for rRNA prediction. The predicted CDSs were annotated from NR, Swiss-Prot, Pfam, GO, COG and KEGG database using sequence alignment tools such as BLASTP, Diamond and HMMER. Briefly, each set of query proteins were aligned with the databases, and annotations of best-matched subjects ( e < 10 –5 ) were obtained for gene annotation.
Bioinformatics analysis
All of the bioinformatics and genomic analysis were performed using the online platform of Majorbio Cloud Platform ( www.majorbio.com ) from Shanghai Majorbio Bio-pharm Technology Co., Ltd. Phylogenetic tree was built using IQ-TREE (maximum-likelihood, ML) based on 27 housekeeping genes including dnaG, nplT, infC, rpsJ, rplC, rplB, rpsS, rpsC, rplP, rplN, rplE, rplF, rpsE, rpsM, rpsK, rplM, nusA, frr, tsf, rpsB, rplS, pgk, pyrG, rplK, rplA, rplL, rpoB . Model prediction software was jModelTest, the model selection criteria was BIC, and bootstraps was 1000. Average nucleotide identity (ANI) analysis was performed using the pyani software with ANIm method. Average aminoacid identity (AAI) analysis was performed using the CompareM software ( e -value 10 –3 , identity 30%, alignment length 70%). Pan-genome and core-genome analysis were performed using the PGAP software with GF method ( e -value ≤ 10 –5 , score ≥ 40, identity ≥ 50%, coverage ≥ 0, inflation 1.5).
Isolates information
Fifteen CC1 (ST1, spa type t127) S. aureus isolates, including five from colonization in 2014 (G2014), five from colonization in 2019 (G2019), and five from bloodstream infection from 2017 to 2019 (GBSI), were enrolled in this study. Ten CC1 S. aureus colonization isolates (GCOL) were collected from nasal swabs of residents from seven NHs, and five CC1 S. aureus bloodstream infection isolates were collected from blood culture of patient with S. aureus bacteremia from three hospitals in Shanghai from 2017 to 2019. More details about the 15 CC1 S. aureus isolates are presented in Table 1 .
Phylogenetic analysis
A phylogenetic tree of the 15 CC1 S. aureus isolates was built as Fig. 1 to infer and evaluate the evolutionary relationships between different genomes. Obviously, isolates did not group according to their origin (colonization/infection, resident/patient, or NH/hospital). However, isolates C11 and C12, which were collected from patients with S. aureus bacteremia in 2017 and 2018 in the same hospital, were grouped into the same sub-clade. ANI and AAI analysis were performed for genetic relationships at the genome-wide level, and no more differences have been found between different groups.
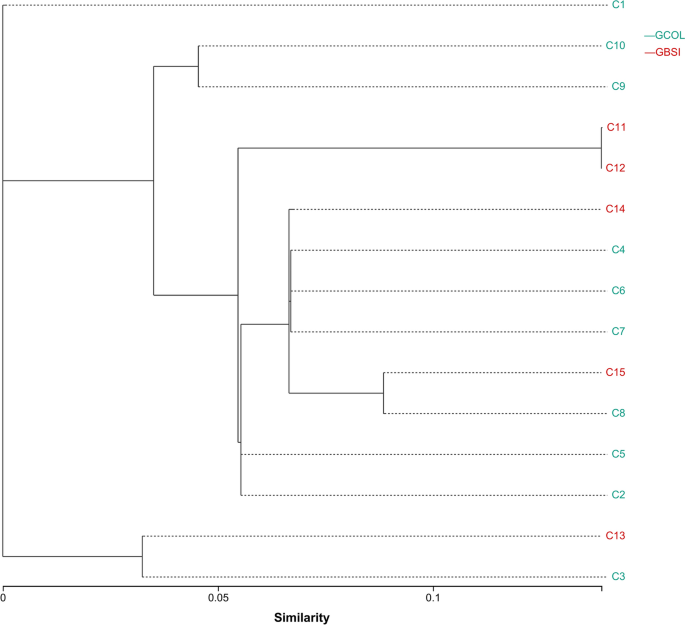
The tree shows an evolutionary of 15 CCI S. aureus isolates based on 27 housekeeping genes, in which each branch represents one isolate, and the length of the branch is the evolutionary distance between two isolates
Homologous genes analysis
Pan-genome and core-genome analysis have been performed to study the dynamic characteristics of bacterial genome and analyze the dynamic changes of bacterial genome in the process of evolution as shown in Fig. 2 . The equation for estimating pan and core-genome size were given with the respective graphs, and the equations were represented in the figures, respectively. The pan-genome size was 3361 genes, and the core-genome size was 2156 genes.
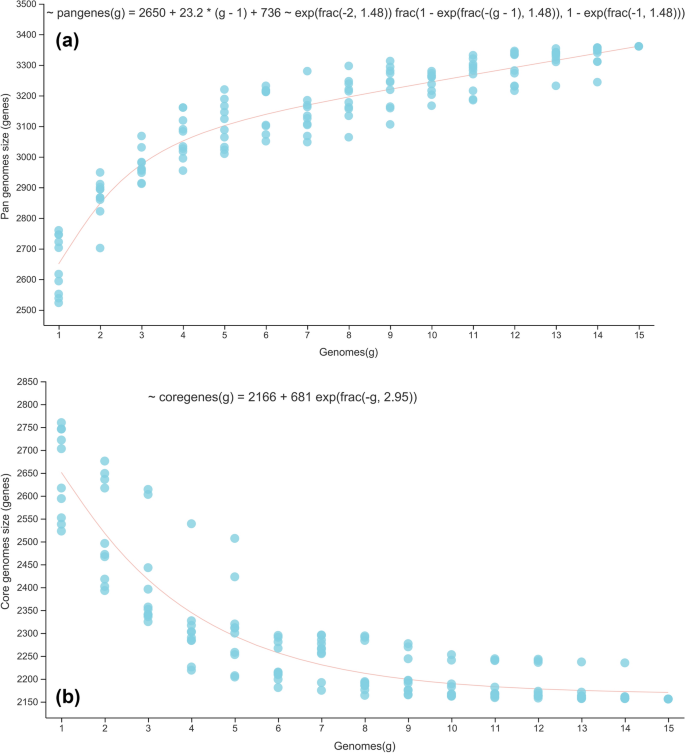
Estimation of pan and core-genome size with the number of genomes. a Pan-genome; b core-genome. The abscissa is the number of genomes, the ordinate is the size of pan or core-genome. The equation for estimating pan and core-genome size according to PGAP is given with the respective graphs
The Venn diagrams were used to display the distribution of pan-genome, and the number of core genes and specific genes in different groups can be seen intuitively. There were 198 and 212 specific genes in GBSI and GCOL, respectively (Fig. 3 a), and there were 76, 85 and 198 specific genes in G2014, G2019 and GBSI, respectively (Fig. 3 b). After blasting and analyzing the specific genes in different groups, some possible significant proteins encoded by the specific genes might play important roles in colonization or infection as shown in Table 2 . Fst family of type I toxin–antitoxin (TA) system which mainly probably helps maintaining stable inheritance was specifically found both in G2014 and 2019, but its detailed functions remain largely mysterious. It is worth noting that the vast majority of proteins uniquely discovered in GBSI are involved in the regulation of virulence factors, such as XdrA, YSIRK signal peptide, CPBP family and OmpR family. CRISPR–Cas (clustered regularly interspaced short palindromic repeat [CRISPR]-CRISPR-associated protein [Cas]) system, which was usually thought of as a genome editing tool, was specifically found in GBSI and might contribute to the pathogenesis of S. aureus human infections.
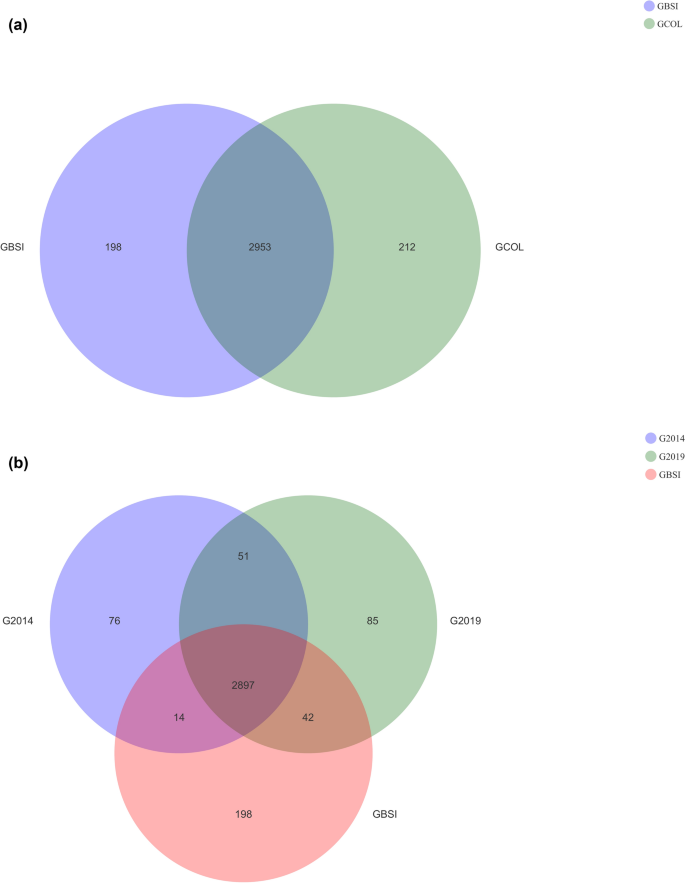
The ellipse or petal of each color in the figure represents a group, the number of crossed petals indicates the number of shared homologous genes, and the number of individual petals indicates the number of homologous genes specific to the group. a GBSI and GCOL; b G2014, G2019 and GBSI
Genomic clustering analysis
Principal component analysis (PCA) and principal coordinates analysis (PCoA) were performed to study the close relationships between genomes of CC1 S. aureus isolates based on COG (Clusters of Orthologous Groups of proteins) and KEGG (Kyoto Encyclopedia of Genes and Genomes). As shown in Fig. 4 , both in PCA and PCoA, the isolates in GBSI might not much closely compared with that in G2014 or/and G2019.
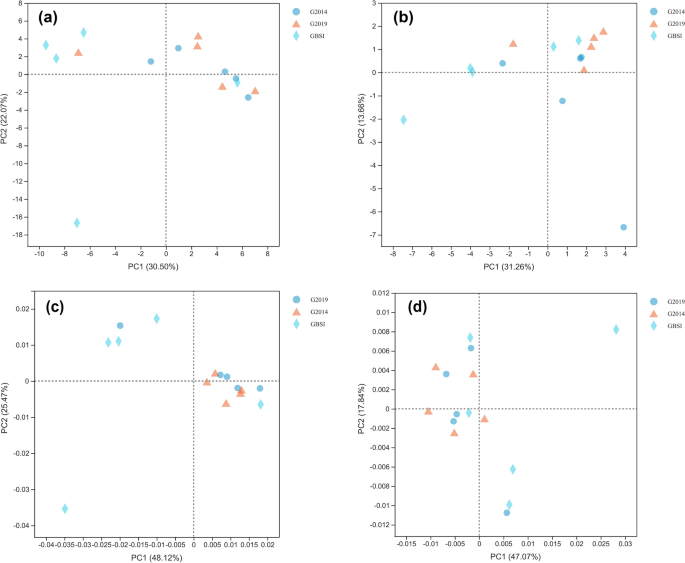
The horizontal and vertical coordinates represent the two selected principal components, and the percentage represents the contribution value of the principal component to the difference in sample composition. The scale of horizontal and vertical axes is relative distance and has no practical significance. Points with different colors or shapes represent isolates of different groups. The closer the two isolate points are, the more similar the two isolates are. a PCA-COG; b PCA-KEGG; c PCoA-COG; d PCoA-KEGG
Correlation analysis was performed (Fig. 5 ) to evaluate the close relationships between CC1 S. aureus isolates based on COG and KEGG. The closer the correlation coefficient is to 1, the better the correlation between two isolates is. Isolate C14 appears to be much more different both in COG and in KEGG in correlation analysis compared with others. Besides, isolates C11, C12, C15 and C8 might be a little more different from other isolates in COG (Fig. 5 a), and isolates C11, C15 and C8 might be more different from others in KEGG (Fig. 5 b).
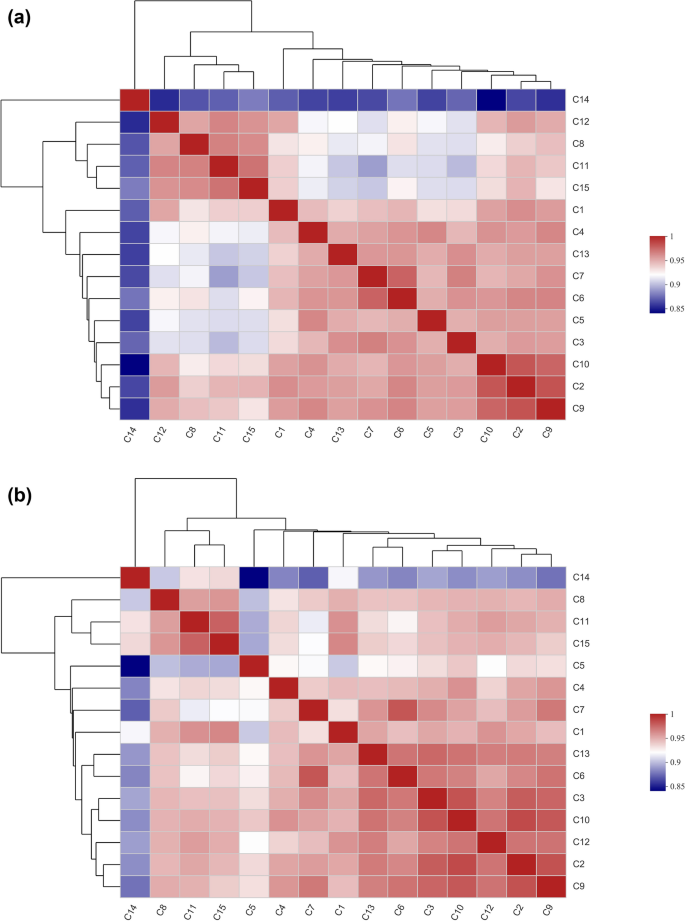
The right and lower sides are isolate names, the left and upper sides are isolate clustering, and squares of different colors represent the correlation between the two isolates. The closer the color is to 1, the better the correlation between the two isolates is. a Correlation heat-map based on COG; b correlation heat-map based on KEGG
Functional analysis
According to the obtained abundance data of function genes and mobile elements, statistical comparison and hypothesis testing were carried out to evaluate the difference and significance level between different groups. The genes in different classifications of pan-genome were annotated under different categories of COG and KEGG, and no significant difference was found including metabolism, information storage and processing, cellular processes and signaling, environmental information processing, genetic information processing, human diseases, organismal systems between GBSI and GCOL.
COG database comparison could be used in predicted proteins for functional annotation, classification, and protein evolution analysis. In COG comparative analysis, there might be significant different in metabolism ( P = 0.0433) between GBSI and GCOL, and no other differences were significantly found in COG.
The abundant pathway information in KEGG database can help us to understand the biological functions of genes at the system level, such as metabolic pathways, genetic information transmission, cytological processes, and some other complex biological processes. In KEGG comparative analysis, much more differences were significantly discovered in KEGG pathway level 1, level 2 and level 3 including in metabolism ( P = 0.0319) and human diseases ( P = 0.0272) between GCOL and GBSI. In addition, there were some significant differences in nucleotide metabolism ( P = 0.0119), pyrimidine metabolism ( P = 0.0119) and pentose phosphate pathway ( P = 0.0361) between G2014 and G2019 in KEGG pathway level 2 and level 3.
S. aureus is one of the most infamous and widespread bacteria both in colonization and infections. On the one hand, about 15% to 32% of the population is thought to be nasally colonized by MSSA, and 1% to 3% is colonized by MRSA [ 29 ]. The rates of S. aureus colonization are typically higher in elder people as increase with advancing age [ 30 ], and the high prevalence of S. aureus colonization in the elderly in Shanghai has been proved as we studied before [ 8 , 9 ]. Approximately 10–40% of people tested as outpatients or on admission are nasal carriages of S. aureus [ 6 ]. On the other hand, S. aureus can cause a hard-to-estimate number of uncomplicated skin infections and probably hundreds of thousands to millions of more severe, invasive infections including bloodstream infections globally per year [ 5 ]. The incidence of S. aureus bloodstream infections, leading high mortality rates (20–50%) despite proper treatment, has been rising in recent years in developed countries as reported [ 1 ].
CC1 is a predominant clone in S. aureus colonization, especially among the elderly people in nursing homes as we studied before. Meanwhile, CC1 is an infection clone in various types of S. aureus infections even in bloodstream infections and is one of the largest and most important S. aureus CCs. We believe that CC1 is an important clone deserved more attention considering the close relationship between colonization and infection that S. aureus infections usually arise from asymptomatic colonization [ 5 ].
According to the results of phylogenetic analysis, perhaps there is nothing remarkable about the evolutionary relationship between CC1 S. aureus colonization and infection. However, maybe it suggests that there is no obvious barrier in CC1 S. aureus between colonization and infection, and there are still continuous communication and transmission of CC1 S. aureus between colonization and infection, which means CC1 S. aureus colonization strains, may serve as endogenous reservoirs for overt clinical infections.
The homologous genes analysis, especially venn diagrams could provide more detailed information. After analyzing the specific genes and the proteins the genes encoded, a few proteins found might play important roles in S. aureus colonization or infection as presented in Table 2 . Toxin–antitoxin (TA) system and other numerous genes in S. aureus could help detect and respond to diverse environmental stimuli such as nutrient starvation and stress to increase its fitness by altering their expression [ 31 ]. S. aureus genome contains 11 TA systems, and Fst family is one of type I TA system. Type I toxins have been demonstrated as bactericidal and can induce cell death in S. aureus [ 31 ]. However, the speculations on function of Fst family were still unclear. The role of plasmid-encoded TA systems in maintaining stable inheritance has been well established; nevertheless, TA system functions remain largely mysterious and the case of Fst system is no exception [ 32 ]. The function of chromosome-encoded Fst system is even more speculative. As is common for TA systems in general, the deletion of the Fst TA system has not been associated with any phenotypic effect on host cells [ 32 ]. Maybe the conditions have not yet been found under which the functions can be observed, or their functions are redundant with other bacterial genes. Even though no effect of Fst system has been found on host cells, its ability to stabilize hereditary material could help S. aureus survive stably in host for colonization.
Except glucosyltransferase and CRISPR–Cas system, the other proteins found in GBSI are regulators of virulence factors. XdrA (XRE-like DNA-binding regulator, A) shares similarity with XRE (xenobiotic response element) family helix-turn-helix, antitoxin-like proteins, and is the main activator which has been proved to bind directly to the spa promoter [ 33 , 34 ]. XdrA regulates spa directly and does not join the interconnected regulatory network linking other well-characterized spa regulators [ 33 ]. Staphylococcal protein A (SPA) encoded by spa is a virulence factor whose function is to capture IgG molecules in the inverted orientation and therefore prevent phagocytosis of the bacterial cells by the host immune system [ 35 ]. Besides, YSIRK signal peptide, which is specifically found in GBSI as well, also has an effect on SPA that promotes SPA cross-wall targeting, though the mechanisms remain largely unknown [ 36 ].
The CPBP (CAAX proteases and bacteriocin-processing enzymes) family includes eukaryotic type II CAAX prenyl proteases and their related bacterial and archaeal homologs, and this family is widely represented in the current sequence database with more than 5,800 members present in all domains of life with most being bacterial [ 37 ]. Although most CPBP members are from bacteria, only a few have been experimentally studied, and the details of their function remain elusive. Based on available research, the function of many uncharacterized bacterial CPBP members might be related to bacteriocin maturation. It has been proposed that the protease activity of bacterial CPBP members could be utilized in the maturation and secretion process of bacteriocins, and/or help confer immunity against self-produced bacteriocins [ 37 ]. OmpR family, a DNA-binding response regulator transcription factor, regulates the production of virulence factors by activating gene expression at the transcriptional level, such as adhesins, toxins, and immunomodulatory proteins [ 38 ]. These virulence factors can cause host innate immune cells, such as macrophages and neutrophils, to release a variety of pro-inflammatory factors, leading to inflammatory damage, and induce cell apoptosis and necrosis of immune cells to escape the recognition and elimination of the innate immune system, leading to serious infectious diseases [ 39 , 40 ].
CRISPR, known as an amazing natural immune system, is widespread in bacteria as well as in archaea and protects them against viral infections [ 41 ]. Type III-A CRISPR–Cas system was found in GBSI and the value of CRISPR–Cas system in adaptive immunity is described in detail in Table 2 . Type III-A CRISPR–Cas systems can modulate bacterial genome stability by specifically preventing plasmid and/or phage transfer in a transcription-dependent manner, and may serve as a high-efficiency tool for deleting virulence or resistance genes in bacteria [ 42 , 43 ]. The relevance of staphylococcal CRISPR element in pathogenesis warrants further investigation. A bigger number of S. aureus strains from different sources especially those from infections origin are necessary for more research, hence additional features can be studied.
In functional analysis, some significant differences like metabolism and human diseases have been found in COG and KEGG between colonization and infection. The differences of metabolism were found both in COG and KEGG, suggesting maybe there are some metabolic differences indeed between colonization and infection. Manifestation of S. aureus disease is advanced by expression of virulence factors, which is directly influenced by the metabolic state of the strain [ 44 ]. It makes sense that the differences in metabolism and virulence factor regulators we found between colonization and infection might have an impact on the virulence factors and make S. aureus more aggressive and leading to infections. The difference of human diseases in KEGG makes the results clearer that it seems the strains of colonization origin cannot have pathogenic potential. Microbial metabolomics and experimental studies should be carried out to learn more about information and details in metabolism between colonization and infection.
In conclusion, no significant differences in genomic evolutionary relationship have been found among CC1 S. aureus isolates between colonization and infection, suggesting there is still ongoing communication and transmission between colonization and infection. Fst family of maintaining stable inheritance might help CC1 S. aureus survive for colonization. Virulence factor regulators XdrA, YSIRK signal peptide, CPBP family and OmpR family, and the metabolic state of the strain, which can promote virulence factors and contribute to the pathogenesis of S. aureus infections, reveal maybe virulence factor regulators and metabolic state can help and accelerate S. aureus pathogenic process compared with colonization. According to the COG and KEGG analysis, it seems that the strains of colonization origin cannot have pathogenic potential. Experimental confirmation is extremely needed about the roles of Fst family, the virulence factor regulators, and the differences in metabolism between CC1 S. aureus colonization and infection.
Data availability
All the sequence data in this study have been deposited in GenBank under BioProject ID PRJNA847504 ( http://www.ncbi.nlm.nih.gov/bioproject/847504 ).
Kwiecinski JM, Horswill AR. Staphylococcus aureus bloodstream infections: pathogenesis and regulatory mechanisms. Curr Opin Microbiol. 2020;53:51–60. https://doi.org/10.1016/j.mib.2020.02.005 .
Article CAS PubMed Central Google Scholar
Tong SY, Davis JS, Eichenberger E, Holland TL, Fowler VG Jr. Staphylococcus aureus infections: epidemiology, pathophysiology, clinical manifestations, and management. Clin Microbiol Rev. 2015;28:603–61. https://doi.org/10.1128/CMR.00134-14 .
Dryden MS. Skin and soft tissue infection: microbiology and epidemiology. Int J Antimicrob Agents. 2009;34(Suppl 1):S2-7. https://doi.org/10.1016/s0924-8579(09)70541-2 .
Article CAS Google Scholar
Cardona AF, Wilson SE. Skin and soft-tissue infections: a critical review and the role of telavancin in their treatment. Clin Infect Dis. 2015;61(Suppl 2):S69-78. https://doi.org/10.1093/cid/civ528 .
Cheung GYC, Bae JS, Otto M. Pathogenicity and virulence of Staphylococcus aureus . Virulence. 2021;12:547–69. https://doi.org/10.1080/21505594.2021.1878688 .
von Eiff C, Becker K, Machka K, Stammer H, Peters G. Nasal carriage as a source of Staphylococcus aureus bacteremia. Study Group. N Engl J Med. 2001;344:11–6. https://doi.org/10.1056/nejm200101043440102 .
Article Google Scholar
Kasela M, et al. Transmission and long-term colonization patterns of Staphylococcus aureus in a nursing home. Int J Environ Res Public Health. 2020. https://doi.org/10.3390/ijerph17218073 .
Article PubMed Central Google Scholar
Zhang J, et al. Prevalence and molecular epidemiology of Staphylococcus aureus among residents of seven nursing homes in Shanghai. PLoS ONE. 2015;10: e0137593. https://doi.org/10.1371/journal.pone.0137593 .
He WP, et al. Molecular characteristics and risk factor analysis of Staphylococcus aureus colonization put insight into CC1 colonization in three nursing homes in Shanghai. PLoS ONE. 2021;16: e0253858. https://doi.org/10.1371/journal.pone.0253858 .
Dabul AN, Camargo IL. Clonal complexes of Staphylococcus aureus : all mixed and together. FEMS Microbiol Lett. 2014;351:7–8. https://doi.org/10.1111/1574-6968.12358 .
Chen Y, et al. The novel fosfomycin resistance gene fosY is present on a genomic island in CC1 methicillin-resistant Staphylococcus aureus . Emerg Microb Infect. 2022;11:1166–73. https://doi.org/10.1080/22221751.2022.2058421 .
Tsujiwaki A, et al. Epidemiology of methicillin-resistant Staphylococcus aureus in a Japanese neonatal intensive care unit. Pediatrics Int. 2020;62:911–9. https://doi.org/10.1111/ped.14241 .
Earls MR, et al. Exploring the evolution and epidemiology of European CC1-MRSA-IV: tracking a multidrug-resistant community-associated methicillin-resistant Staphylococcus aureus clone. Microb Genom. 2021. https://doi.org/10.1099/mgen.0.000601 .
Senok A, et al. Genotyping of methicillin resistant Staphylococcus aureus from the United Arab Emirates. Sci Rep. 2020;10:18551. https://doi.org/10.1038/s41598-020-75565-w .
Noone JC, et al. Molecular characteristics of Staphylococcus aureus associated prosthetic joint infections after hip fractures treated with hemiarthroplasty: a retrospective genome-wide association study. Sci Rep. 2020;10:16553. https://doi.org/10.1038/s41598-020-73736-3 .
Monecke S, et al. Genotyping of methicillin-resistant Staphylococcus aureus from sepsis patients in Pakistan and detection of antibodies against staphylococcal virulence factors. Eur J Clin Microbiol Infect Dis. 2020;39:85–92. https://doi.org/10.1007/s10096-019-03695-9 .
Gu F, et al. Antimicrobial resistance and molecular epidemiology of Staphylococcus aureus causing bloodstream infections at Ruijin Hospital in Shanghai from 2013 to 2018. Sci Rep. 2020. https://doi.org/10.1038/s41598-020-63248-5 .
Firoozeh F, Omidi M, Saffari M, Sedaghat H, Zibaei M. Molecular analysis of methicillin-resistant Staphylococcus aureus isolates from four teaching hospitals in Iran: the emergence of novel MRSA clones. Antimicrob Resist Infect Control. 2020. https://doi.org/10.1186/s13756-020-00777-8 .
Dong Q, et al. Phenotypic and molecular characteristics of community-associated Staphylococcus aureus infection in neonates. Infect Drug Resist. 2020;13:4589–600. https://doi.org/10.2147/IDR.S284781 .
Boswihi SS, Udo EE, AlFouzan W. Antibiotic resistance and typing of the methicillin-resistant Staphylococcus aureus clones in Kuwait hospitals, 2016–2017. BMC Microbiol. 2020. https://doi.org/10.1186/s12866-020-02009-w .
Vidal C, et al. Fulminant arterial vasculitis as an unusual complication of disseminated staphylococcal disease due to the emerging CC1 methicillin-susceptible Staphylococcus aureus clone: a case report. BMC Infect Dis. 2019. https://doi.org/10.1186/s12879-019-3933-3 .
Monteiro AS, et al. Phylogenetic and molecular profile of Staphylococcus aureus isolated from bloodstream infections in Northeast Brazil. Microorganisms. 2019. https://doi.org/10.3390/microorganisms7070210 .
Gu F, et al. A 10-year retrospective study of methicillin-resistant Staphylococcus aureus from burn wound infection in southeast China from 2013 to 2022. Front Microbiol. 2023. https://doi.org/10.3389/fmicb.2023.1301744 .
Clinical and Laboratory Standards Institute. Performance standards for antimicrobial susceptibility testing. CLSI document M100-S29, Wayne, PA, USA 39. 2019.
Chongtrakool P, et al. Staphylococcal cassette chromosome mec (SCCmec) typing of methicillin-resistant Staphylococcus aureus strains isolated in 11 Asian countries: a proposal for a new nomenclature for SCCmec elements. Antimicrob Agents Chemother. 2006;50:1001–12. https://doi.org/10.1128/aac.50.3.1001-1012.2006 .
Chen S, Zhou Y, Chen Y, Gu J. fastp: an ultra-fast all-in-one FASTQ preprocessor. Bioinformatics. 2018;34:i884–90. https://doi.org/10.1093/bioinformatics/bty560 .
Luo R, et al. SOAPdenovo2: an empirically improved memory-efficient short-read de novo assembler. Gigascience. 2012;1:18. https://doi.org/10.1186/2047-217x-1-18 .
Delcher AL, Bratke KA, Powers EC, Salzberg SL. Identifying bacterial genes and endosymbiont DNA with Glimmer. Bioinformatics. 2007;23:673–9. https://doi.org/10.1093/bioinformatics/btm009 .
Sharara SL, Maragakis LL, Cosgrove SE. Decolonization of Staphylococcus aureus . Infect Dis Clin North Am. 2021;35:107–33. https://doi.org/10.1016/j.idc.2020.10.010 .
Baldwin NS, et al. Prevalence of methicillin-resistant Staphylococcus aureus colonization in residents and staff in nursing homes in Northern Ireland. J Am Geriatr Soc. 2009;57:620–6. https://doi.org/10.1111/j.1532-5415.2009.02181.x .
Habib G, Zhu Q, Sun B. Bioinformatics and functional assessment of toxin-antitoxin systems in Staphylococcus aureus . Toxins. 2018. https://doi.org/10.3390/toxins10110473 .
Weaver K. The Fst/Ldr family of type I TA system toxins: potential roles in stress response, metabolism and pathogenesis. Toxins. 2020. https://doi.org/10.3390/toxins12080474 .
McCallum N, Hinds J, Ender M, Berger-Bachi B, Stutzmann Meier P. Transcriptional profiling of XdrA, a new regulator of spa transcription in Staphylococcus aureus . J Bacteriol. 2010;192:5151–64. https://doi.org/10.1128/JB.00491-10 .
Oscarsson J. Regulatory role of proteins binding to the (protein A) and (Staphylococcal accessory regulator) promoter regions in NTCC 8325–4. Int J Med Microbiol. 2005;295:253–66. https://doi.org/10.1016/j.ijmm.2005.05.003 .
Votintseva AA, et al. Prevalence of Staphylococcus aureus protein A (spa) mutants in the community and hospitals in Oxfordshire. BMC Microbiol. 2014;14:63. https://doi.org/10.1186/1471-2180-14-63 .
Zhang R, et al. Spatial regulation of protein A in Staphylococcus aureus . Mol Microbiol. 2021;116:589–605. https://doi.org/10.1111/mmi.14734 .
Pei J, Mitchell DA, Dixon JE, Grishin NV. Expansion of type II CAAX proteases reveals evolutionary origin of gamma-secretase subunit APH-1. J Mol Biol. 2011;410:18–26. https://doi.org/10.1016/j.jmb.2011.04.066 .
Fan R, et al. The effects of l -arginine on protein stability and DNA binding ability of SaeR, a transcription factor in Staphylococcus aureus . Protein Expr Purif. 2021;177:105765. https://doi.org/10.1016/j.pep.2020.105765 .
Zecconi A, Scali F. Staphylococcus aureus virulence factors in evasion from innate immune defenses in human and animal diseases. Immunol Lett. 2013;150:12–22. https://doi.org/10.1016/j.imlet.2013.01.004 .
Liang X, et al. Inactivation of a two-component signal transduction system, SaeRS, eliminates adherence and attenuates virulence of Staphylococcus aureus . Infect Immun. 2006;74:4655–65. https://doi.org/10.1128/IAI.00322-06 .
Javed MR, et al. CRISPR-Cas system: history and prospects as a genome editing tool in microorganisms. Curr Microbiol. 2018;75:1675–83. https://doi.org/10.1007/s00284-018-1547-4 .
Guan J, Wang W, Sun B. Chromosomal targeting by the type III-A CRISPR-Cas system can reshape genomes in Staphylococcus aureus . mSphere. 2017. https://doi.org/10.1128/mSphere.00403-17 .
Cao L, et al. Identification and functional study of type III-A CRISPR-Cas systems in clinical isolates of Staphylococcus aureus . Int J Med Microbiol. 2016;306:686–96. https://doi.org/10.1016/j.ijmm.2016.08.005 .
Choueiry F, Xu R, Zhu J. Adaptive metabolism of Staphylococcus aureus revealed by untargeted metabolomics. J Proteome Res. 2022;21:470–81. https://doi.org/10.1021/acs.jproteome.1c00797 .
Thomer L, et al. N-acetylglucosaminylation of serine-aspartate repeat proteins promotes Staphylococcus aureus bloodstream infection. J Biol Chem. 2014;289:3478–86. https://doi.org/10.1074/jbc.M113.532655 .
Download references
This study was supported by National Natural Science Foundation of China (Grant Numbers 82102452). The funders had no role in study design, data collection and analysis, decision to publish, or preparation of the manuscript.
Author information
Feifei Gu and Weiping He have contributed equally to this study.
Authors and Affiliations
Department of Laboratory Medicine, Ruijin Hospital, Shanghai Jiao Tong University School of Medicine, Shanghai, China
Feifei Gu, Dedong Zhu, Qian Zeng, Shuzhen Xiao, Yuxing Ni & Lizhong Han
Department of Clinical Laboratory, Huaihe Hospital of Henan University, Kaifeng, Henan, China
Department of Infectious Diseases, Research Laboratory of Clinical Virology, Ruijin Hospital, Shanghai Jiao Tong University School of Medicine, Shanghai, China
You can also search for this author in PubMed Google Scholar
Contributions
Conceived and designed the study: F.G., W.H. and L.H. Performed the experiments: F.G., W.H. and D.Z. Analyzed the data F.G. and W.H. Contributed reagents/materials/analysis tools: Q.Z., X.L., S.X, D.Z and Y.N. Wrote the paper: F.G. Final approval of the submitted manuscript: All.
Corresponding author
Correspondence to Lizhong Han .
Ethics declarations
Ethical approval and consent to participate.
This study was approved by Ethics Committee of Ruijin Hospital affiliated to Shanghai Jiao Tong University School of Medicine, and the Review Committee removed the need for informed consent for this retrospective study which focused on bacteria and did not involve patient interventions.
Competing interests
The authors declare no competing interests.
Additional information
Publisher's note.
Springer Nature remains neutral with regard to jurisdictional claims in published maps and institutional affiliations.
Rights and permissions
Open Access This article is licensed under a Creative Commons Attribution-NonCommercial-NoDerivatives 4.0 International License, which permits any non-commercial use, sharing, distribution and reproduction in any medium or format, as long as you give appropriate credit to the original author(s) and the source, provide a link to the Creative Commons licence, and indicate if you modified the licensed material. You do not have permission under this licence to share adapted material derived from this article or parts of it. The images or other third party material in this article are included in the article’s Creative Commons licence, unless indicated otherwise in a credit line to the material. If material is not included in the article’s Creative Commons licence and your intended use is not permitted by statutory regulation or exceeds the permitted use, you will need to obtain permission directly from the copyright holder. To view a copy of this licence, visit http://creativecommons.org/licenses/by-nc-nd/4.0/ .
Reprints and permissions
About this article
Cite this article.
Gu, F., He, W., Zhu, D. et al. Genome-wide comparative analysis of CC1 Staphylococcus aureus between colonization and infection. Eur J Med Res 29 , 474 (2024). https://doi.org/10.1186/s40001-024-02076-z
Download citation
Received : 30 August 2023
Accepted : 24 September 2024
Published : 30 September 2024
DOI : https://doi.org/10.1186/s40001-024-02076-z
Share this article
Anyone you share the following link with will be able to read this content:
Sorry, a shareable link is not currently available for this article.
Provided by the Springer Nature SharedIt content-sharing initiative
- Staphylococcus aureus
- Colonization
European Journal of Medical Research
ISSN: 2047-783X
- General enquiries: [email protected]

IMAGES
VIDEO
COMMENTS
However, they differ in their approach and purpose. A case study involves in-depth analysis of a particular individual, group, or situation, aiming to provide a detailed understanding of a specific phenomenon. On the other hand, an experiment involves manipulating variables and observing the effects on a sample population, aiming to establish ...
One of the key differences between case study and experimental research is the issue of generalizability. Case study research is often criticized for its limited generalizability due to the focus on a single case. The findings may not be applicable to a larger population. In contrast, experimental research aims for generalizability by using ...
Key Differences. Case studies involve a detailed examination of a specific subject, providing rich qualitative data. Experiments are structured to test hypotheses, involving variable control and manipulation. In case studies, data is gathered from real-world observations, interviews, and documents. Experiments rely on quantitative data from ...
One of the key differences between case studies and single-case experimental designs is their generalizability. Case studies are often conducted on unique or rare cases, making it challenging to generalize the findings to a larger population. The focus of case studies is on providing detailed insights into specific cases rather than making ...
We may approach this study by 2 longitudinal designs: Prospective: we follow the individuals in the future to know who will develop the disease. Retrospective: we look to the past to know who developed the disease (e.g. using medical records) This design is the strongest among the observational studies. For example - to find out the relative ...
The current conflict between case study and experimental research: A breakthrough study derives benefits from both. (ERIC Document Number ED339721). This paper describes a study that was not experimentally designed, but its major findings were generalizable to the overall population of writers in college freshman composition classes.
One distinction worth making between two types of non-experimental research is the difference be- tween quasi-experimental research and case studies. The example I discussed earlier - in which we wanted to examine incidence of lung cancer among smokers and non-smokers, without trying to control who smokes and who doesn't - is a quasi ...
Revised on November 20, 2023. A case study is a detailed study of a specific subject, such as a person, group, place, event, organization, or phenomenon. Case studies are commonly used in social, educational, clinical, and business research. A case study research design usually involves qualitative methods, but quantitative methods are ...
Other interesting articles. If you want to know more about statistics, methodology, or research bias, make sure to check out some of our other articles with explanations and examples. Statistics. Normal distribution. Skewness. Kurtosis. Degrees of freedom. Variance. Null hypothesis.
Case studies tend to focus on qualitative data using methods such as interviews, observations, and analysis of primary and secondary sources (e.g., newspaper articles, photographs, official records). Sometimes a case study will also collect quantitative data. Example: Mixed methods case study. For a case study of a wind farm development in a ...
Observational research studies involve the passive observation of subjects without any intervention or manipulation by researchers. These studies are designed to scrutinize the relationships between variables and test subjects, uncover patterns, and draw conclusions grounded in real-world data. Researchers refrain from interfering with the ...
Case‐control studies are study designs that compare two groups, such as the subjects with disease (cases) to the subjects without disease (controls), and to look for differences in risk factors. 8 This study is used to study risk factors or etiologies for a disease, especially if the disease is rare. Thus, case‐control studies can also be ...
The differences are apparent in terms of emphasis (e.g., more observations in ethnog-raphy, more interviews in grounded theory) and extent of data collection (e.g., only interviews in phenomenology, multiple forms in case study research to provide the in-depth case picture). At the data analysis stage, the differences are most pronounced.
Case study - A case study is an uncontrolled, observational study of events and outcomes in a single case. Case series - A description of uncontrolled, non-experimental events and outcomes for a series of similar cases who receive the same intervention or have the same outcome.
Case study and research are both methods used in academic and professional settings to gather information and gain insights. However, they differ in their approach and purpose. A case study is an in-depth analysis of a specific individual, group, or situation, aiming to understand the unique characteristics and dynamics involved.
The pre-experimental research design is further divided into three types. One-shot Case Study Research Design. In this type of experimental study, only one dependent group or variable is considered. The study is carried out after some treatment which was presumed to cause change, making it a posttest study.
The difference between a case study and an experiment lies in their methodology and purpose, where a case study is an in-depth analysis of a specific situation or individual, while an experiment involves the manipulation of variables to test hypotheses and draw conclusions. ... A case study is a research method in which the researcher explores ...
The study design used to answer a particular research question depends on the nature of the question and the availability of resources. In this article, which is the first part of a series on "study designs," we provide an overview of research study designs and their classification. The subsequent articles will focus on individual designs.
Revised on January 22, 2024. Like a true experiment, a quasi-experimental design aims to establish a cause-and-effect relationship between an independent and dependent variable. However, unlike a true experiment, a quasi-experiment does not rely on random assignment. Instead, subjects are assigned to groups based on non-random criteria.
One distinction worth making between two types of non-experimental research is the difference between quasi-experimental research and case studies. The example I discussed earlier - in which we wanted to examine incidence of lung cancer among smokers and non-smokers, without trying to control who smokes and who doesn't - is a quasi ...
First, by the specific research question. That is, if the question is one of 'prevalence' (disease burden) then the ideal is a cross-sectional study; if it is a question of 'harm' - a case-control study; prognosis - a cohort and therapy - a RCT. Second, by what resources are available to you. This includes budget, time, feasibility re-patient ...
Single-case experimental designs (SCEDs) represent a family of research designs that use experimental methods to study the effects of treatments on outcomes. The fundamental unit of analysis is the single case—which can be an individual, clinic, or community—ideally with replications of effects within and/or between cases.
This study uses two methods for collecting S. aureus.The first approach involves using Andersen six-stage air samplers for a 3.0 min collection duration at a flow rate of 28.3 L/min 40 to monitor ...
With the booming development of rural tourism, the users of rural environments are gradually becoming more diverse. Both tourists and villagers are the main appreciators of rural landscapes, but the cognitive similarities and differences in rural landscape between the two have not yet been explored. Therefore, taking Wangshang Village, located in Shaanxi Province, China as a case study, this ...
Controlled experiments establish causality, whereas correlational studies only show associations between variables. In an experimental design, you manipulate an independent variable and measure its effect on a dependent variable. Other variables are controlled so they can't impact the results. In a correlational design, you measure variables ...
This study investigated the differences between emerging and expert international publication (IP) writers' engagement with syntactic complexity in seven research article (RA) part-genres.
Background Staphylococcus aureus is one of the most important bacteria in human colonization and infection. Clonal complex1 (CC1) is one of the largest and most important S. aureus CCs, and it is a predominant clone in S. aureus colonization and can cause a series of S. aureus infections including bloodstream infections. No studies on the relationship of CC1 S. aureus between colonization and ...