Artificial Versus Human Intelligence Essay
Introduction.
With the rise of artificial intelligence (AI), it became clear that future technologies will further advance the autonomous ability of computers to generate new data. Human intelligence lies in the basis of such developments and represents the collective knowledge gained from the analysis of experiences people live through. In turn, AI is an outcome of this progression, which allows humanity to put this data in a digital form that possesses some autonomous qualities. As a result, AI also contains limitations that the human brain does not have, such as physical constrictions that put a cap on its computational capacities (Korteling et al., 2021). At the same time, people are not bound by a defined amount of operating memory in their thoughts.
It is impossible to adequately compare artificial and ‘real’ intelligence, as they do not share the same functionality on a physical level. Korteling et al. (2021) state that AI possesses “fundamentally different cognitive qualities and abilities than biological systems” (p. 1). Scientists are able to push the limits of AI further through technological progress, yet human brains can not be modified in a similar fashion. The sheer complexity of people’s cognitive abilities governs the processes that are above what computers can perform. However, AIs can work with massive amounts of data that people can not handle. The current state of AI allows many industries to apply this technology in their operations successfully. People can train AIs to excel at the analysis of a particular type of information and direct their accumulated knowledge to achieve specific goals.
In conclusion, humans’ cognitive abilities and AI differ in development potential, range of application, and many other aspects, yet they can complement each other.
Korteling, J. E., Boer-Visschedijk, G. C., Blankendaal, R. A., Boonekamp, R. C., & Eikelboom, A. R. (2021). Human- versus artificial intelligence. Frontiers in Artificial Intelligence , 4 .
- Chicago (A-D)
- Chicago (N-B)
IvyPanda. (2023, September 2). Artificial Versus Human Intelligence. https://ivypanda.com/essays/artificial-versus-human-intelligence/
"Artificial Versus Human Intelligence." IvyPanda , 2 Sept. 2023, ivypanda.com/essays/artificial-versus-human-intelligence/.
IvyPanda . (2023) 'Artificial Versus Human Intelligence'. 2 September.
IvyPanda . 2023. "Artificial Versus Human Intelligence." September 2, 2023. https://ivypanda.com/essays/artificial-versus-human-intelligence/.
1. IvyPanda . "Artificial Versus Human Intelligence." September 2, 2023. https://ivypanda.com/essays/artificial-versus-human-intelligence/.
Bibliography
IvyPanda . "Artificial Versus Human Intelligence." September 2, 2023. https://ivypanda.com/essays/artificial-versus-human-intelligence/.
- Esophagus Anatomy and Physiology
- Computational Biology as an Essential Research Area
- Computational Knowledge in Wikipedia
- Computational Linguistics
- Top Microprocessors in Computational Biology
- Marr’s Computational Theory and Explanation Levels
- Computational Models or Protocols of E-Work
- "Auditory Cortex Mapmaking" by Schreiner and Winer
- The Age of Artificial Intelligence (AI)
- The Prevention of Diabetes and Its Consequences on the Population
- The Machine Intelligence Research Institute
- Working With Artificial Intelligence (AI)
- Artificial Intelligence Transforming the World
- Automatic Systems and Artificial Intelligence in Manufacturing
- Smart Cities Optimization With Artificial Intelligence

How close are we to AI that surpasses human intelligence?
Subscribe to the center for technology innovation newsletter, jeremy baum and jeremy baum undergraduate student - ucla, researcher - ucla institute for technology, law, and policy @_jeremybaum john villasenor john villasenor nonresident senior fellow - governance studies , center for technology innovation @johndvillasenor.
July 18, 2023
- Artificial general intelligence (AGI) is difficult to precisely define but refers to a superintelligent AI recognizable from science fiction.
- AGI may still be far off, but the growing capabilities of generative AI suggest that we could be making progress toward its development.
- The development of AGI will have a transformative effect on society and create significant opportunities and threats, raising difficult questions about regulation.
For decades, superintelligent artificial intelligence (AI) has been a staple of science fiction, embodied in books and movies about androids, robot uprisings, and a world taken over by computers. As far-fetched as those plots often were, they played off a very real mix of fascination, curiosity, and trepidation regarding the potential to build intelligent machines.
Today, public interest in AI is at an all-time high. With the headlines in recent months about generative AI systems like ChatGPT, there is also a different phrase that has started to enter the broader dialog: a rtificial general intelligence , or AGI. But what exactly is AGI, and how close are today’s technologies to achieving it?
Despite the similarity in the phrases generative AI and artificial general intelligence, they have very different meanings. As a post from IBM explains, “Generative AI refers to deep-learning models that can generate high-quality text, images, and other content based on the data they were trained on.” However, the ability of an AI system to generate content does not necessarily mean that its intelligence is general.
To better understand artificial general intelligence, it helps to first understand how it differs from today’s AI, which is highly specialized. For example, an AI chess program is extraordinarily good at playing chess, but if you ask it to write an essay on the causes of World War I, it won’t be of any use. Its intelligence is limited to one specific domain. Other examples of specialized AI include the systems that provide content recommendations on the social media platform TikTok, navigation decisions in driverless cars, and purchase recommendations from Amazon.
AGI: A range of definitions
By contrast, AGI refers to a much broader form of machine intelligence. There is no single, formally recognized definition of AGI—rather, there is a range of definitions that include the following:
While the OpenAI definition ties AGI to the ability to “outperform humans at most economically valuable work,” today’s systems are nowhere near that capable. Consider Indeed’s list of the most common jobs in the U.S. As of March 2023, the first 10 jobs on that list were: cashier, food preparation worker, stocking associate, laborer, janitor, construction worker, bookkeeper, server, medical assistant, and bartender. These jobs require not only intellectual capacity but, crucially, most of them require a far higher degree of manual dexterity than today’s most advanced AI robotics systems can achieve.
None of the other AGI definitions in the table specifically mention economic value. Another contrast evident in the table is that while the OpenAI AGI definition requires outperforming humans, the other definitions only require AGI to perform at levels comparable to humans. Common to all of the definitions, either explicitly or implicitly, is the concept that an AGI system can perform tasks across many domains, adapt to the changes in its environment, and solve new problems—not only the ones in its training data.
GPT-4: Sparks of AGI?
A group of industry AI researchers recently made a splash when they published a preprint of an academic paper titled, “Sparks of Artificial General Intelligence: Early experiments with GPT-4.” GPT-4 is a large language model that has been publicly accessible to ChatGPT Plus (paid upgrade) users since March 2023. The researchers noted that “GPT-4 can solve novel and difficult tasks that span mathematics, coding, vision, medicine, law, psychology and more, without needing any special prompting,” exhibiting “strikingly close to human-level performance.” They concluded that GPT-4 “could reasonably be viewed as an early (yet still incomplete) version” of AGI.
Of course, there are also skeptics: As quoted in a May New York Times article , Carnegie Mellon professor Maarten Sap said, “The ‘Sparks of A.G.I.’ is an example of some of these big companies co-opting the research paper format into P.R. pitches.” In an interview with IEEE Spectrum, researcher and robotics entrepreneur Rodney Brooks underscored that in evaluating the capabilities of systems like ChatGPT, we often “mistake performance for competence.”
GPT-4 and beyond
While the version of GPT-4 currently available to the public is impressive, it is not the end of the road. There are groups working on additions to GPT-4 that are more goal-driven, meaning that you can give the system an instruction such as “Design and build a website on (topic).” The system will then figure out exactly what subtasks need to be completed and in what order in order to achieve that goal. Today, these systems are not particularly reliable, as they frequently fail to reach the stated goal. But they will certainly get better in the future.
In a 2020 paper , Yoshihiro Maruyama of the Australian National University identified eight attributes a system must have for it to be considered AGI: Logic, autonomy, resilience, integrity, morality, emotion, embodiment, and embeddedness. The last two attributes—embodiment and embeddedness—refer to having a physical form that facilitates learning and understanding of the world and human behavior, and a deep integration with social, cultural, and environmental systems that allows adaption to human needs and values.
It can be argued that ChatGPT displays some of these attributes, like logic. For example, GPT-4 with no additional features reportedly scored a 163 on the LSAT and 1410 on the SAT . For other attributes, the determination is tied as much to philosophy as much as to technology. For instance, is a system that merely exhibits what appears to be morality actually moral? If asked to provide a one-word answer to the question “is murder wrong?” GPT-4 will respond by saying “Yes.” This is a morally correct response, but it doesn’t mean that GPT-4 itself has morality, but rather that it has inferred the morally correct answer through its training data.
A key subtlety that often goes missing in the “How close is AGI?” discussion is that intelligence exists on a continuum, and therefore assessing whether a system displays AGI will require considering a continuum. On this point, the research done on animal intelligence offers a useful analog. We understand that animal intelligence is far too complex to enable us to meaningfully convey animal cognitive capacity by classifying each species as either “intelligent” or “not intelligent:” Animal intelligence exists on a spectrum that spans many dimensions, and evaluating it requires considering context. Similarly, as AI systems become more capable, assessing the degree to which they display generalized intelligence will be involve more than simply choosing between “yes” and “no.”
AGI: Threat or opportunity?
Whenever and in whatever form it arrives, AGI will be transformative, impacting everything from the labor market to how we understand concepts like intelligence and creativity. As with so many other technologies, it also has the potential of being harnessed in harmful ways. For instance, the need to address the potential biases in today’s AI systems is well recognized, and that concern will apply to future AGI systems as well. At the same time, it is also important to recognize that AGI will also offer enormous promise to amplify human innovation and creativity. In medicine, for example, new drugs that would have eluded human scientists working alone could be more easily identified by scientists working with AGI systems.
AGI can also help broaden access to services that previously were accessible only to the most economically privileged. For instance, in the context of education, AGI systems could put personalized, one-on-one tutoring within easy financial reach of everyone, resulting in improved global literacy rates. AGI could also help broaden the reach of medical care by bringing sophisticated, individualized diagnostic care to much broader populations.
Regulating emergent AGI systems
At the May 2023 G7 summit in Japan, the leaders of the world’s seven largest democratic economies issued a communiqué that included an extended discussion of AI, writing that “international governance of new digital technologies has not necessarily kept pace.” Proposals regarding increased AI regulation are now a regular feature of policy discussions in the United States , the European Union , Japan , and elsewhere.
In the future, as AGI moves from science fiction to reality, it will supercharge the already-robust debate regarding AI regulation. But preemptive regulation is always a challenge, and this will be particularly so in relation to AGI—a technology that escapes easy definition, and that will evolve in ways that are impossible to predict.
An outright ban on AGI would be bad policy. For example, AGI systems that are capable of emotional recognition could be very beneficial in a context such as education, where they could discern whether a student appears to understand a new concept, and adjust an interaction accordingly. Yet the EU Parliament’s AI Act, which passed a major legislative milestone in June, would ban emotional recognition in AI systems (and therefore also in AGI systems) in certain contexts like education.
A better approach is to first gain a clear understanding of potential misuses of specific AGI systems once those systems exist and can be analyzed, and then to examine whether those misuses are addressed by existing, non-AI-specific regulatory frameworks (e.g., the prohibition against employment discrimination provided by Title VII of the Civil Rights Act of 1964). If that analysis identifies a gap, then it does indeed make sense to examine the potential role in filling that gap of “soft” law (voluntary frameworks) as well as formal laws and regulations. But regulating AGI based only on the fact that it will be highly capable would be a mistake.
Related Content
Cameron F. Kerry
July 7, 2023
Alex Engler
June 16, 2023
Darrell M. West
May 17, 2023
Artificial Intelligence Technology Policy & Regulation
Governance Studies
Center for Technology Innovation
April 4, 2024
Nicol Turner Lee
March 28, 2024
Joseph B. Keller

- SUGGESTED TOPICS
- The Magazine
- Newsletters
- Managing Yourself
- Managing Teams
- Work-life Balance
- The Big Idea
- Data & Visuals
- Reading Lists
- Case Selections
- HBR Learning
- Topic Feeds
- Account Settings
- Email Preferences
AI Should Augment Human Intelligence, Not Replace It
- David De Cremer
- Garry Kasparov

Artificial intelligence isn’t coming for your job, but it will be your new coworker. Here’s how to get along.
Will smart machines really replace human workers? Probably not. People and AI both bring different abilities and strengths to the table. The real question is: how can human intelligence work with artificial intelligence to produce augmented intelligence. Chess Grandmaster Garry Kasparov offers some unique insight here. After losing to IBM’s Deep Blue, he began to experiment how a computer helper changed players’ competitive advantage in high-level chess games. What he discovered was that having the best players and the best program was less a predictor of success than having a really good process. Put simply, “Weak human + machine + better process was superior to a strong computer alone and, more remarkably, superior to a strong human + machine + inferior process.” As leaders look at how to incorporate AI into their organizations, they’ll have to manage expectations as AI is introduced, invest in bringing teams together and perfecting processes, and refine their own leadership abilities.
In an economy where data is changing how companies create value — and compete — experts predict that using artificial intelligence (AI) at a larger scale will add as much as $15.7 trillion to the global economy by 2030 . As AI is changing how companies work, many believe that who does this work will change, too — and that organizations will begin to replace human employees with intelligent machines . This is already happening: intelligent systems are displacing humans in manufacturing, service delivery, recruitment, and the financial industry, consequently moving human workers towards lower-paid jobs or making them unemployed. This trend has led some to conclude that in 2040 our workforce may be totally unrecognizable .
- David De Cremer is a professor of management and technology at Northeastern University and the Dunton Family Dean of its D’Amore-McKim School of Business. His website is daviddecremer.com .
- Garry Kasparov is the chairman of the Human Rights Foundation and founder of the Renew Democracy Initiative. He writes and speaks frequently on politics, decision-making, and human-machine collaboration. Kasparov became the youngest world chess champion in history at 22 in 1985 and retained the top rating in the world for 20 years. His famous matches against the IBM super-computer Deep Blue in 1996 and 1997 were key to bringing artificial intelligence, and chess, into the mainstream. His latest book on artificial intelligence and the future of human-plus-machine is Deep Thinking: Where Machine Intelligence Ends and Human Creativity Begins (2017).
Partner Center
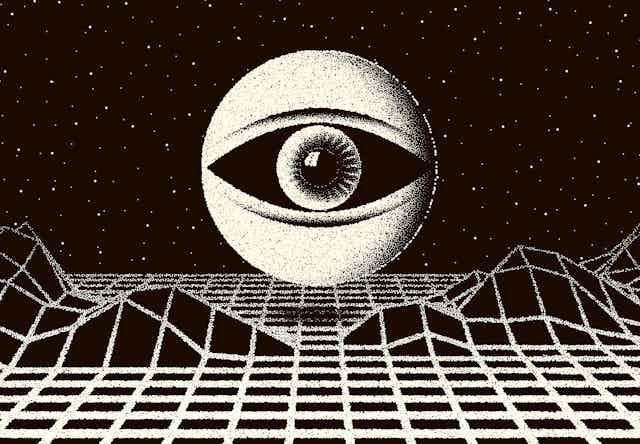
Will AI ever reach human-level intelligence? We asked five experts
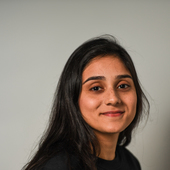
Digital Culture Editor
Interviewed
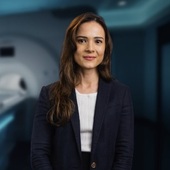
Biomedical Engineer and Neuroscientist, University of Sydney
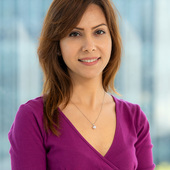
Lecturer in AI and Data Science, Swinburne University of Technology
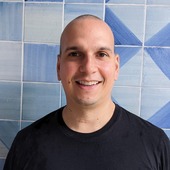
Lecturer in Business Analytics, University of Sydney
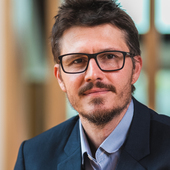
Professor and Head of the Department of Philosophy, and Co-Director of the Macquire University Ethics & Agency Research Centre, Macquarie University
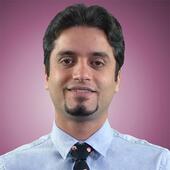
Professor, Director of Centre for Artificial Intelligence Research and Optimisation, Torrens University Australia
View all partners
Artificial intelligence has changed form in recent years.
What started in the public eye as a burgeoning field with promising (yet largely benign) applications, has snowballed into a more than US$100 billion industry where the heavy hitters – Microsoft, Google and OpenAI, to name a few – seem intent on out-competing one another.
The result has been increasingly sophisticated large language models, often released in haste and without adequate testing and oversight.
These models can do much of what a human can, and in many cases do it better. They can beat us at advanced strategy games , generate incredible art , diagnose cancers and compose music.
Read more: Text-to-audio generation is here. One of the next big AI disruptions could be in the music industry
There’s no doubt AI systems appear to be “intelligent” to some extent. But could they ever be as intelligent as humans?
There’s a term for this: artificial general intelligence (AGI). Although it’s a broad concept, for simplicity you can think of AGI as the point at which AI acquires human-like generalised cognitive capabilities. In other words, it’s the point where AI can tackle any intellectual task a human can.
AGI isn’t here yet; current AI models are held back by a lack of certain human traits such as true creativity and emotional awareness.
We asked five experts if they think AI will ever reach AGI, and five out of five said yes.
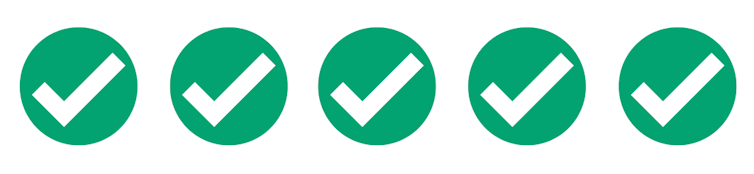
But there are subtle differences in how they approach the question. From their responses, more questions emerge. When might we achieve AGI? Will it go on to surpass humans? And what constitutes “intelligence”, anyway?
Here are their detailed responses:
Read more: Calls to regulate AI are growing louder. But how exactly do you regulate a technology like this?
- Artificial intelligence (AI)
- Artificial general intelligence
- Five Experts
- Human intelligence

Project Officer, Student Volunteer Program

Audience Development Coordinator (fixed-term maternity cover)

Lecturer (Hindi-Urdu)

Director, Defence and Security

Opportunities with the new CIEHF
Read our research on: Gun Policy | International Conflict | Election 2024
Regions & Countries
Artificial intelligence and the future of humans, experts say the rise of artificial intelligence will make most people better off over the next decade, but many have concerns about how advances in ai will affect what it means to be human, to be productive and to exercise free will.
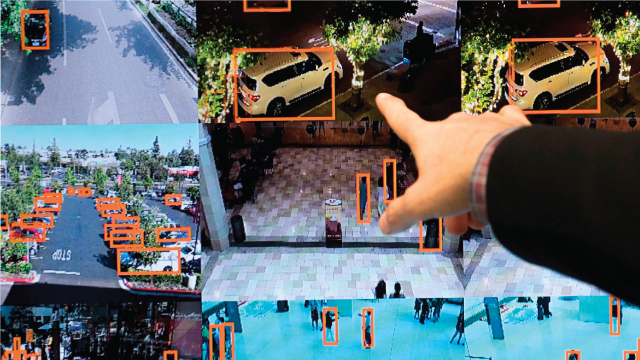
Digital life is augmenting human capacities and disrupting eons-old human activities. Code-driven systems have spread to more than half of the world’s inhabitants in ambient information and connectivity, offering previously unimagined opportunities and unprecedented threats. As emerging algorithm-driven artificial intelligence (AI) continues to spread, will people be better off than they are today?
Some 979 technology pioneers, innovators, developers, business and policy leaders, researchers and activists answered this question in a canvassing of experts conducted in the summer of 2018.
The experts predicted networked artificial intelligence will amplify human effectiveness but also threaten human autonomy, agency and capabilities. They spoke of the wide-ranging possibilities; that computers might match or even exceed human intelligence and capabilities on tasks such as complex decision-making, reasoning and learning, sophisticated analytics and pattern recognition, visual acuity, speech recognition and language translation. They said “smart” systems in communities, in vehicles, in buildings and utilities, on farms and in business processes will save time, money and lives and offer opportunities for individuals to enjoy a more-customized future.
Many focused their optimistic remarks on health care and the many possible applications of AI in diagnosing and treating patients or helping senior citizens live fuller and healthier lives. They were also enthusiastic about AI’s role in contributing to broad public-health programs built around massive amounts of data that may be captured in the coming years about everything from personal genomes to nutrition. Additionally, a number of these experts predicted that AI would abet long-anticipated changes in formal and informal education systems.
Yet, most experts, regardless of whether they are optimistic or not, expressed concerns about the long-term impact of these new tools on the essential elements of being human. All respondents in this non-scientific canvassing were asked to elaborate on why they felt AI would leave people better off or not. Many shared deep worries, and many also suggested pathways toward solutions. The main themes they sounded about threats and remedies are outlined in the accompanying table.
Specifically, participants were asked to consider the following:
“Please think forward to the year 2030. Analysts expect that people will become even more dependent on networked artificial intelligence (AI) in complex digital systems. Some say we will continue on the historic arc of augmenting our lives with mostly positive results as we widely implement these networked tools. Some say our increasing dependence on these AI and related systems is likely to lead to widespread difficulties.
Our question: By 2030, do you think it is most likely that advancing AI and related technology systems will enhance human capacities and empower them? That is, most of the time, will most people be better off than they are today? Or is it most likely that advancing AI and related technology systems will lessen human autonomy and agency to such an extent that most people will not be better off than the way things are today?”
Overall, and despite the downsides they fear, 63% of respondents in this canvassing said they are hopeful that most individuals will be mostly better off in 2030, and 37% said people will not be better off.
A number of the thought leaders who participated in this canvassing said humans’ expanding reliance on technological systems will only go well if close attention is paid to how these tools, platforms and networks are engineered, distributed and updated. Some of the powerful, overarching answers included those from:
Sonia Katyal , co-director of the Berkeley Center for Law and Technology and a member of the inaugural U.S. Commerce Department Digital Economy Board of Advisors, predicted, “In 2030, the greatest set of questions will involve how perceptions of AI and their application will influence the trajectory of civil rights in the future. Questions about privacy, speech, the right of assembly and technological construction of personhood will all re-emerge in this new AI context, throwing into question our deepest-held beliefs about equality and opportunity for all. Who will benefit and who will be disadvantaged in this new world depends on how broadly we analyze these questions today, for the future.”
We need to work aggressively to make sure technology matches our values. Erik Brynjolfsson
Erik Brynjolfsson , director of the MIT Initiative on the Digital Economy and author of “Machine, Platform, Crowd: Harnessing Our Digital Future,” said, “AI and related technologies have already achieved superhuman performance in many areas, and there is little doubt that their capabilities will improve, probably very significantly, by 2030. … I think it is more likely than not that we will use this power to make the world a better place. For instance, we can virtually eliminate global poverty, massively reduce disease and provide better education to almost everyone on the planet. That said, AI and ML [machine learning] can also be used to increasingly concentrate wealth and power, leaving many people behind, and to create even more horrifying weapons. Neither outcome is inevitable, so the right question is not ‘What will happen?’ but ‘What will we choose to do?’ We need to work aggressively to make sure technology matches our values. This can and must be done at all levels, from government, to business, to academia, and to individual choices.”
Bryan Johnson , founder and CEO of Kernel, a leading developer of advanced neural interfaces, and OS Fund, a venture capital firm, said, “I strongly believe the answer depends on whether we can shift our economic systems toward prioritizing radical human improvement and staunching the trend toward human irrelevance in the face of AI. I don’t mean just jobs; I mean true, existential irrelevance, which is the end result of not prioritizing human well-being and cognition.”
Marina Gorbis , executive director of the Institute for the Future, said, “Without significant changes in our political economy and data governance regimes [AI] is likely to create greater economic inequalities, more surveillance and more programmed and non-human-centric interactions. Every time we program our environments, we end up programming ourselves and our interactions. Humans have to become more standardized, removing serendipity and ambiguity from our interactions. And this ambiguity and complexity is what is the essence of being human.”
Judith Donath , author of “The Social Machine, Designs for Living Online” and faculty fellow at Harvard University’s Berkman Klein Center for Internet & Society, commented, “By 2030, most social situations will be facilitated by bots – intelligent-seeming programs that interact with us in human-like ways. At home, parents will engage skilled bots to help kids with homework and catalyze dinner conversations. At work, bots will run meetings. A bot confidant will be considered essential for psychological well-being, and we’ll increasingly turn to such companions for advice ranging from what to wear to whom to marry. We humans care deeply about how others see us – and the others whose approval we seek will increasingly be artificial. By then, the difference between humans and bots will have blurred considerably. Via screen and projection, the voice, appearance and behaviors of bots will be indistinguishable from those of humans, and even physical robots, though obviously non-human, will be so convincingly sincere that our impression of them as thinking, feeling beings, on par with or superior to ourselves, will be unshaken. Adding to the ambiguity, our own communication will be heavily augmented: Programs will compose many of our messages and our online/AR appearance will [be] computationally crafted. (Raw, unaided human speech and demeanor will seem embarrassingly clunky, slow and unsophisticated.) Aided by their access to vast troves of data about each of us, bots will far surpass humans in their ability to attract and persuade us. Able to mimic emotion expertly, they’ll never be overcome by feelings: If they blurt something out in anger, it will be because that behavior was calculated to be the most efficacious way of advancing whatever goals they had ‘in mind.’ But what are those goals? Artificially intelligent companions will cultivate the impression that social goals similar to our own motivate them – to be held in good regard, whether as a beloved friend, an admired boss, etc. But their real collaboration will be with the humans and institutions that control them. Like their forebears today, these will be sellers of goods who employ them to stimulate consumption and politicians who commission them to sway opinions.”
Andrew McLaughlin , executive director of the Center for Innovative Thinking at Yale University, previously deputy chief technology officer of the United States for President Barack Obama and global public policy lead for Google, wrote, “2030 is not far in the future. My sense is that innovations like the internet and networked AI have massive short-term benefits, along with long-term negatives that can take decades to be recognizable. AI will drive a vast range of efficiency optimizations but also enable hidden discrimination and arbitrary penalization of individuals in areas like insurance, job seeking and performance assessment.”
Michael M. Roberts , first president and CEO of the Internet Corporation for Assigned Names and Numbers (ICANN) and Internet Hall of Fame member, wrote, “The range of opportunities for intelligent agents to augment human intelligence is still virtually unlimited. The major issue is that the more convenient an agent is, the more it needs to know about you – preferences, timing, capacities, etc. – which creates a tradeoff of more help requires more intrusion. This is not a black-and-white issue – the shades of gray and associated remedies will be argued endlessly. The record to date is that convenience overwhelms privacy. I suspect that will continue.”
danah boyd , a principal researcher for Microsoft and founder and president of the Data & Society Research Institute, said, “AI is a tool that will be used by humans for all sorts of purposes, including in the pursuit of power. There will be abuses of power that involve AI, just as there will be advances in science and humanitarian efforts that also involve AI. Unfortunately, there are certain trend lines that are likely to create massive instability. Take, for example, climate change and climate migration. This will further destabilize Europe and the U.S., and I expect that, in panic, we will see AI be used in harmful ways in light of other geopolitical crises.”
Amy Webb , founder of the Future Today Institute and professor of strategic foresight at New York University, commented, “The social safety net structures currently in place in the U.S. and in many other countries around the world weren’t designed for our transition to AI. The transition through AI will last the next 50 years or more. As we move farther into this third era of computing, and as every single industry becomes more deeply entrenched with AI systems, we will need new hybrid-skilled knowledge workers who can operate in jobs that have never needed to exist before. We’ll need farmers who know how to work with big data sets. Oncologists trained as robotocists. Biologists trained as electrical engineers. We won’t need to prepare our workforce just once, with a few changes to the curriculum. As AI matures, we will need a responsive workforce, capable of adapting to new processes, systems and tools every few years. The need for these fields will arise faster than our labor departments, schools and universities are acknowledging. It’s easy to look back on history through the lens of present – and to overlook the social unrest caused by widespread technological unemployment. We need to address a difficult truth that few are willing to utter aloud: AI will eventually cause a large number of people to be permanently out of work. Just as generations before witnessed sweeping changes during and in the aftermath of the Industrial Revolution, the rapid pace of technology will likely mean that Baby Boomers and the oldest members of Gen X – especially those whose jobs can be replicated by robots – won’t be able to retrain for other kinds of work without a significant investment of time and effort.”
Barry Chudakov , founder and principal of Sertain Research, commented, “By 2030 the human-machine/AI collaboration will be a necessary tool to manage and counter the effects of multiple simultaneous accelerations: broad technology advancement, globalization, climate change and attendant global migrations. In the past, human societies managed change through gut and intuition, but as Eric Teller, CEO of Google X, has said, ‘Our societal structures are failing to keep pace with the rate of change.’ To keep pace with that change and to manage a growing list of ‘wicked problems’ by 2030, AI – or using Joi Ito’s phrase, extended intelligence – will value and revalue virtually every area of human behavior and interaction. AI and advancing technologies will change our response framework and time frames (which in turn, changes our sense of time). Where once social interaction happened in places – work, school, church, family environments – social interactions will increasingly happen in continuous, simultaneous time. If we are fortunate, we will follow the 23 Asilomar AI Principles outlined by the Future of Life Institute and will work toward ‘not undirected intelligence but beneficial intelligence.’ Akin to nuclear deterrence stemming from mutually assured destruction, AI and related technology systems constitute a force for a moral renaissance. We must embrace that moral renaissance, or we will face moral conundrums that could bring about human demise. … My greatest hope for human-machine/AI collaboration constitutes a moral and ethical renaissance – we adopt a moonshot mentality and lock arms to prepare for the accelerations coming at us. My greatest fear is that we adopt the logic of our emerging technologies – instant response, isolation behind screens, endless comparison of self-worth, fake self-presentation – without thinking or responding smartly.”
John C. Havens , executive director of the IEEE Global Initiative on Ethics of Autonomous and Intelligent Systems and the Council on Extended Intelligence, wrote, “Now, in 2018, a majority of people around the world can’t access their data, so any ‘human-AI augmentation’ discussions ignore the critical context of who actually controls people’s information and identity. Soon it will be extremely difficult to identify any autonomous or intelligent systems whose algorithms don’t interact with human data in one form or another.”
At stake is nothing less than what sort of society we want to live in and how we experience our humanity. Batya Friedman
Batya Friedman , a human-computer interaction professor at the University of Washington’s Information School, wrote, “Our scientific and technological capacities have and will continue to far surpass our moral ones – that is our ability to use wisely and humanely the knowledge and tools that we develop. … Automated warfare – when autonomous weapons kill human beings without human engagement – can lead to a lack of responsibility for taking the enemy’s life or even knowledge that an enemy’s life has been taken. At stake is nothing less than what sort of society we want to live in and how we experience our humanity.”
Greg Shannon , chief scientist for the CERT Division at Carnegie Mellon University, said, “Better/worse will appear 4:1 with the long-term ratio 2:1. AI will do well for repetitive work where ‘close’ will be good enough and humans dislike the work. … Life will definitely be better as AI extends lifetimes, from health apps that intelligently ‘nudge’ us to health, to warnings about impending heart/stroke events, to automated health care for the underserved (remote) and those who need extended care (elder care). As to liberty, there are clear risks. AI affects agency by creating entities with meaningful intellectual capabilities for monitoring, enforcing and even punishing individuals. Those who know how to use it will have immense potential power over those who don’t/can’t. Future happiness is really unclear. Some will cede their agency to AI in games, work and community, much like the opioid crisis steals agency today. On the other hand, many will be freed from mundane, unengaging tasks/jobs. If elements of community happiness are part of AI objective functions, then AI could catalyze an explosion of happiness.”
Kostas Alexandridis , author of “Exploring Complex Dynamics in Multi-agent-based Intelligent Systems,” predicted, “Many of our day-to-day decisions will be automated with minimal intervention by the end-user. Autonomy and/or independence will be sacrificed and replaced by convenience. Newer generations of citizens will become more and more dependent on networked AI structures and processes. There are challenges that need to be addressed in terms of critical thinking and heterogeneity. Networked interdependence will, more likely than not, increase our vulnerability to cyberattacks. There is also a real likelihood that there will exist sharper divisions between digital ‘haves’ and ‘have-nots,’ as well as among technologically dependent digital infrastructures. Finally, there is the question of the new ‘commanding heights’ of the digital network infrastructure’s ownership and control.”
Oscar Gandy , emeritus professor of communication at the University of Pennsylvania, responded, “We already face an ungranted assumption when we are asked to imagine human-machine ‘collaboration.’ Interaction is a bit different, but still tainted by the grant of a form of identity – maybe even personhood – to machines that we will use to make our way through all sorts of opportunities and challenges. The problems we will face in the future are quite similar to the problems we currently face when we rely upon ‘others’ (including technological systems, devices and networks) to acquire things we value and avoid those other things (that we might, or might not be aware of).”
James Scofield O’Rourke , a professor of management at the University of Notre Dame, said, “Technology has, throughout recorded history, been a largely neutral concept. The question of its value has always been dependent on its application. For what purpose will AI and other technological advances be used? Everything from gunpowder to internal combustion engines to nuclear fission has been applied in both helpful and destructive ways. Assuming we can contain or control AI (and not the other way around), the answer to whether we’ll be better off depends entirely on us (or our progeny). ‘The fault, dear Brutus, is not in our stars, but in ourselves, that we are underlings.’”
Simon Biggs , a professor of interdisciplinary arts at the University of Edinburgh, said, “AI will function to augment human capabilities. The problem is not with AI but with humans. As a species we are aggressive, competitive and lazy. We are also empathic, community minded and (sometimes) self-sacrificing. We have many other attributes. These will all be amplified. Given historical precedent, one would have to assume it will be our worst qualities that are augmented. My expectation is that in 2030 AI will be in routine use to fight wars and kill people, far more effectively than we can currently kill. As societies we will be less affected by this as we currently are, as we will not be doing the fighting and killing ourselves. Our capacity to modify our behaviour, subject to empathy and an associated ethical framework, will be reduced by the disassociation between our agency and the act of killing. We cannot expect our AI systems to be ethical on our behalf – they won’t be, as they will be designed to kill efficiently, not thoughtfully. My other primary concern is to do with surveillance and control. The advent of China’s Social Credit System (SCS) is an indicator of what it likely to come. We will exist within an SCS as AI constructs hybrid instances of ourselves that may or may not resemble who we are. But our rights and affordances as individuals will be determined by the SCS. This is the Orwellian nightmare realised.”
Mark Surman , executive director of the Mozilla Foundation, responded, “AI will continue to concentrate power and wealth in the hands of a few big monopolies based on the U.S. and China. Most people – and parts of the world – will be worse off.”
William Uricchio , media scholar and professor of comparative media studies at MIT, commented, “AI and its related applications face three problems: development at the speed of Moore’s Law, development in the hands of a technological and economic elite, and development without benefit of an informed or engaged public. The public is reduced to a collective of consumers awaiting the next technology. Whose notion of ‘progress’ will prevail? We have ample evidence of AI being used to drive profits, regardless of implications for long-held values; to enhance governmental control and even score citizens’ ‘social credit’ without input from citizens themselves. Like technologies before it, AI is agnostic. Its deployment rests in the hands of society. But absent an AI-literate public, the decision of how best to deploy AI will fall to special interests. Will this mean equitable deployment, the amelioration of social injustice and AI in the public service? Because the answer to this question is social rather than technological, I’m pessimistic. The fix? We need to develop an AI-literate public, which means focused attention in the educational sector and in public-facing media. We need to assure diversity in the development of AI technologies. And until the public, its elected representatives and their legal and regulatory regimes can get up to speed with these fast-moving developments we need to exercise caution and oversight in AI’s development.”
The remainder of this report is divided into three sections that draw from hundreds of additional respondents’ hopeful and critical observations: 1) concerns about human-AI evolution, 2) suggested solutions to address AI’s impact, and 3) expectations of what life will be like in 2030, including respondents’ positive outlooks on the quality of life and the future of work, health care and education. Some responses are lightly edited for style.
Sign up for our Internet, Science and Tech newsletter
New findings, delivered monthly
Report Materials

Table of Contents
Many americans think generative ai programs should credit the sources they rely on, americans’ use of chatgpt is ticking up, but few trust its election information, q&a: how we used large language models to identify guests on popular podcasts, striking findings from 2023, what the data says about americans’ views of artificial intelligence, most popular.
About Pew Research Center Pew Research Center is a nonpartisan fact tank that informs the public about the issues, attitudes and trends shaping the world. It conducts public opinion polling, demographic research, media content analysis and other empirical social science research. Pew Research Center does not take policy positions. It is a subsidiary of The Pew Charitable Trusts .
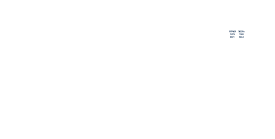
Artificial intelligence is transforming our world — it is on all of us to make sure that it goes well
How ai gets built is currently decided by a small group of technologists. as this technology is transforming our lives, it should be in all of our interest to become informed and engaged..
Why should you care about the development of artificial intelligence?
Think about what the alternative would look like. If you and the wider public do not get informed and engaged, then we leave it to a few entrepreneurs and engineers to decide how this technology will transform our world.
That is the status quo. This small number of people at a few tech firms directly working on artificial intelligence (AI) do understand how extraordinarily powerful this technology is becoming . If the rest of society does not become engaged, then it will be this small elite who decides how this technology will change our lives.
To change this status quo, I want to answer three questions in this article: Why is it hard to take the prospect of a world transformed by AI seriously? How can we imagine such a world? And what is at stake as this technology becomes more powerful?
Why is it hard to take the prospect of a world transformed by artificial intelligence seriously?
In some way, it should be obvious how technology can fundamentally transform the world. We just have to look at how much the world has already changed. If you could invite a family of hunter-gatherers from 20,000 years ago on your next flight, they would be pretty surprised. Technology has changed our world already, so we should expect that it can happen again.
But while we have seen the world transform before, we have seen these transformations play out over the course of generations. What is different now is how very rapid these technological changes have become. In the past, the technologies that our ancestors used in their childhood were still central to their lives in their old age. This has not been the case anymore for recent generations. Instead, it has become common that technologies unimaginable in one's youth become ordinary in later life.
This is the first reason we might not take the prospect seriously: it is easy to underestimate the speed at which technology can change the world.
The second reason why it is difficult to take the possibility of transformative AI – potentially even AI as intelligent as humans – seriously is that it is an idea that we first heard in the cinema. It is not surprising that for many of us, the first reaction to a scenario in which machines have human-like capabilities is the same as if you had asked us to take seriously a future in which vampires, werewolves, or zombies roam the planet. 1
But, it is plausible that it is both the stuff of sci-fi fantasy and the central invention that could arrive in our, or our children’s, lifetimes.
The third reason why it is difficult to take this prospect seriously is by failing to see that powerful AI could lead to very large changes. This is also understandable. It is difficult to form an idea of a future that is very different from our own time. There are two concepts that I find helpful in imagining a very different future with artificial intelligence. Let’s look at both of them.
How to develop an idea of what the future of artificial intelligence might look like?
When thinking about the future of artificial intelligence, I find it helpful to consider two different concepts in particular: human-level AI, and transformative AI. 2 The first concept highlights the AI’s capabilities and anchors them to a familiar benchmark, while transformative AI emphasizes the impact that this technology would have on the world.
From where we are today, much of this may sound like science fiction. It is therefore worth keeping in mind that the majority of surveyed AI experts believe there is a real chance that human-level artificial intelligence will be developed within the next decades, and some believe that it will exist much sooner.
The advantages and disadvantages of comparing machine and human intelligence
One way to think about human-level artificial intelligence is to contrast it with the current state of AI technology. While today’s AI systems often have capabilities similar to a particular, limited part of the human mind, a human-level AI would be a machine that is capable of carrying out the same range of intellectual tasks that we humans are capable of. 3 It is a machine that would be “able to learn to do anything that a human can do,” as Norvig and Russell put it in their textbook on AI. 4
Taken together, the range of abilities that characterize intelligence gives humans the ability to solve problems and achieve a wide variety of goals. A human-level AI would therefore be a system that could solve all those problems that we humans can solve, and do the tasks that humans do today. Such a machine, or collective of machines, would be able to do the work of a translator, an accountant, an illustrator, a teacher, a therapist, a truck driver, or the work of a trader on the world’s financial markets. Like us, it would also be able to do research and science, and to develop new technologies based on that.
The concept of human-level AI has some clear advantages. Using the familiarity of our own intelligence as a reference provides us with some clear guidance on how to imagine the capabilities of this technology.
However, it also has clear disadvantages. Anchoring the imagination of future AI systems to the familiar reality of human intelligence carries the risk that it obscures the very real differences between them.
Some of these differences are obvious. For example, AI systems will have the immense memory of computer systems, against which our own capacity to store information pales. Another obvious difference is the speed at which a machine can absorb and process information. But information storage and processing speed are not the only differences. The domains in which machines already outperform humans is steadily increasing: in chess, after matching the level of the best human players in the late 90s, AI systems reached superhuman levels more than a decade ago. In other games like Go or complex strategy games, this has happened more recently. 5
These differences mean that an AI that is at least as good as humans in every domain would overall be much more powerful than the human mind. Even the first “human-level AI” would therefore be quite superhuman in many ways. 6
Human intelligence is also a bad metaphor for machine intelligence in other ways. The way we think is often very different from machines, and as a consequence the output of thinking machines can be very alien to us.
Most perplexing and most concerning are the strange and unexpected ways in which machine intelligence can fail. The AI-generated image of the horse below provides an example: on the one hand, AIs can do what no human can do – produce an image of anything, in any style (here photorealistic), in mere seconds – but on the other hand it can fail in ways that no human would fail. 7 No human would make the mistake of drawing a horse with five legs. 8
Imagining a powerful future AI as just another human would therefore likely be a mistake. The differences might be so large that it will be a misnomer to call such systems “human-level.”
AI-generated image of a horse 9
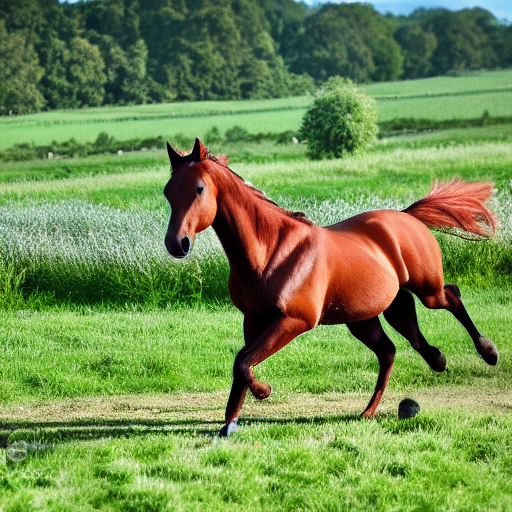
Transformative artificial intelligence is defined by the impact this technology would have on the world
In contrast, the concept of transformative AI is not based on a comparison with human intelligence. This has the advantage of sidestepping the problems that the comparisons with our own mind bring. But it has the disadvantage that it is harder to imagine what such a system would look like and be capable of. It requires more from us. It requires us to imagine a world with intelligent actors that are potentially very different from ourselves.
Transformative AI is not defined by any specific capabilities, but by the real-world impact that the AI would have. To qualify as transformative, researchers think of it as AI that is “powerful enough to bring us into a new, qualitatively different future.” 10
In humanity’s history, there have been two cases of such major transformations, the agricultural and the industrial revolutions.
Transformative AI becoming a reality would be an event on that scale. Like the arrival of agriculture 10,000 years ago, or the transition from hand- to machine-manufacturing, it would be an event that would change the world for billions of people around the globe and for the entire trajectory of humanity’s future .
Technologies that fundamentally change how a wide range of goods or services are produced are called ‘general-purpose technologies’. The two previous transformative events were caused by the discovery of two particularly significant general-purpose technologies: the change in food production as humanity transitioned from hunting and gathering to farming, and the rise of machine manufacturing in the industrial revolution. Based on the evidence and arguments presented in this series on AI development, I believe it is plausible that powerful AI could represent the introduction of a similarly significant general-purpose technology.
Timeline of the three transformative events in world history

A future of human-level or transformative AI?
The two concepts are closely related, but they are not the same. The creation of a human-level AI would certainly have a transformative impact on our world. If the work of most humans could be carried out by an AI, the lives of millions of people would change. 11
The opposite, however, is not true: we might see transformative AI without developing human-level AI. Since the human mind is in many ways a poor metaphor for the intelligence of machines, we might plausibly develop transformative AI before we develop human-level AI. Depending on how this goes, this might mean that we will never see any machine intelligence for which human intelligence is a helpful comparison.
When and if AI systems might reach either of these levels is of course difficult to predict. In my companion article on this question, I give an overview of what researchers in this field currently believe. Many AI experts believe there is a real chance that such systems will be developed within the next decades, and some believe that they will exist much sooner.
What is at stake as artificial intelligence becomes more powerful?
All major technological innovations lead to a range of positive and negative consequences. For AI, the spectrum of possible outcomes – from the most negative to the most positive – is extraordinarily wide.
That the use of AI technology can cause harm is clear, because it is already happening.
AI systems can cause harm when people use them maliciously. For example, when they are used in politically-motivated disinformation campaigns or to enable mass surveillance. 12
But AI systems can also cause unintended harm, when they act differently than intended or fail. For example, in the Netherlands the authorities used an AI system which falsely claimed that an estimated 26,000 parents made fraudulent claims for child care benefits. The false allegations led to hardship for many poor families, and also resulted in the resignation of the Dutch government in 2021. 13
As AI becomes more powerful, the possible negative impacts could become much larger. Many of these risks have rightfully received public attention: more powerful AI could lead to mass labor displacement, or extreme concentrations of power and wealth. In the hands of autocrats, it could empower totalitarianism through its suitability for mass surveillance and control.
The so-called alignment problem of AI is another extreme risk. This is the concern that nobody would be able to control a powerful AI system, even if the AI takes actions that harm us humans, or humanity as a whole. This risk is unfortunately receiving little attention from the wider public, but it is seen as an extremely large risk by many leading AI researchers. 14
How could an AI possibly escape human control and end up harming humans?
The risk is not that an AI becomes self-aware, develops bad intentions, and “chooses” to do this. The risk is that we try to instruct the AI to pursue some specific goal – even a very worthwhile one – and in the pursuit of that goal it ends up harming humans. It is about unintended consequences. The AI does what we told it to do, but not what we wanted it to do.
Can’t we just tell the AI to not do those things? It is definitely possible to build an AI that avoids any particular problem we foresee, but it is hard to foresee all the possible harmful unintended consequences. The alignment problem arises because of “the impossibility of defining true human purposes correctly and completely,” as AI researcher Stuart Russell puts it. 15
Can’t we then just switch off the AI? This might also not be possible. That is because a powerful AI would know two things: it faces a risk that humans could turn it off, and it can’t achieve its goals once it has been turned off. As a consequence, the AI will pursue a very fundamental goal of ensuring that it won’t be switched off. This is why, once we realize that an extremely intelligent AI is causing unintended harm in the pursuit of some specific goal, it might not be possible to turn it off or change what the system does. 16
This risk – that humanity might not be able to stay in control once AI becomes very powerful, and that this might lead to an extreme catastrophe – has been recognized right from the early days of AI research more than 70 years ago. 17 The very rapid development of AI in recent years has made a solution to this problem much more urgent.
I have tried to summarize some of the risks of AI, but a short article is not enough space to address all possible questions. Especially on the very worst risks of AI systems, and what we can do now to reduce them, I recommend reading the book The Alignment Problem by Brian Christian and Benjamin Hilton’s article ‘Preventing an AI-related catastrophe’ .
If we manage to avoid these risks, transformative AI could also lead to very positive consequences. Advances in science and technology were crucial to the many positive developments in humanity’s history. If artificial ingenuity can augment our own, it could help us make progress on the many large problems we face: from cleaner energy, to the replacement of unpleasant work, to much better healthcare.
This extremely large contrast between the possible positives and negatives makes clear that the stakes are unusually high with this technology. Reducing the negative risks and solving the alignment problem could mean the difference between a healthy, flourishing, and wealthy future for humanity – and the destruction of the same.
How can we make sure that the development of AI goes well?
Making sure that the development of artificial intelligence goes well is not just one of the most crucial questions of our time, but likely one of the most crucial questions in human history. This needs public resources – public funding, public attention, and public engagement.
Currently, almost all resources that are dedicated to AI aim to speed up the development of this technology. Efforts that aim to increase the safety of AI systems, on the other hand, do not receive the resources they need. Researcher Toby Ord estimated that in 2020 between $10 to $50 million was spent on work to address the alignment problem. 18 Corporate AI investment in the same year was more than 2000-times larger, it summed up to $153 billion.
This is not only the case for the AI alignment problem. The work on the entire range of negative social consequences from AI is under-resourced compared to the large investments to increase the power and use of AI systems.
It is frustrating and concerning for society as a whole that AI safety work is extremely neglected and that little public funding is dedicated to this crucial field of research. On the other hand, for each individual person this neglect means that they have a good chance to actually make a positive difference, if they dedicate themselves to this problem now. And while the field of AI safety is small, it does provide good resources on what you can do concretely if you want to work on this problem.
I hope that more people dedicate their individual careers to this cause, but it needs more than individual efforts. A technology that is transforming our society needs to be a central interest of all of us. As a society we have to think more about the societal impact of AI, become knowledgeable about the technology, and understand what is at stake.
When our children look back at today, I imagine that they will find it difficult to understand how little attention and resources we dedicated to the development of safe AI. I hope that this changes in the coming years, and that we begin to dedicate more resources to making sure that powerful AI gets developed in a way that benefits us and the next generations.
If we fail to develop this broad-based understanding, then it will remain the small elite that finances and builds this technology that will determine how one of the – or plausibly the – most powerful technology in human history will transform our world.
If we leave the development of artificial intelligence entirely to private companies, then we are also leaving it up these private companies what our future — the future of humanity — will be.
With our work at Our World in Data we want to do our small part to enable a better informed public conversation on AI and the future we want to live in. You can find these resources on OurWorldinData.org/artificial-intelligence
Acknowledgements: I would like to thank my colleagues Daniel Bachler, Charlie Giattino, and Edouard Mathieu for their helpful comments to drafts of this essay.
This problem becomes even larger when we try to imagine how a future with a human-level AI might play out. Any particular scenario will not only involve the idea that this powerful AI exists, but a whole range of additional assumptions about the future context in which this happens. It is therefore hard to communicate a scenario of a world with human-level AI that does not sound contrived, bizarre or even silly.
Both of these concepts are widely used in the scientific literature on artificial intelligence. For example, questions about the timelines for the development of future AI are often framed using these terms. See my article on this topic .
The fact that humans are capable of a range of intellectual tasks means that you arrive at different definitions of intelligence depending on which aspect within that range you focus on (the Wikipedia entry on intelligence , for example, lists a number of definitions from various researchers and different disciplines). As a consequence there are also various definitions of ‘human-level AI’.
There are also several closely related terms: Artificial General Intelligence, High-Level Machine Intelligence, Strong AI, or Full AI are sometimes synonymously used, and sometimes defined in similar, yet different ways. In specific discussions, it is necessary to define this concept more narrowly; for example, in studies on AI timelines researchers offer more precise definitions of what human-level AI refers to in their particular study.
Peter Norvig and Stuart Russell (2021) — Artificial Intelligence: A Modern Approach. Fourth edition. Published by Pearson.
The AI system AlphaGo , and its various successors, won against Go masters. The AI system Pluribus beat humans at no-limit Texas hold 'em poker. The AI system Cicero can strategize and use human language to win the strategy game Diplomacy. See: Meta Fundamental AI Research Diplomacy Team (FAIR), Anton Bakhtin, Noam Brown, Emily Dinan, Gabriele Farina, Colin Flaherty, Daniel Fried, et al. (2022) – ‘Human-Level Play in the Game of Diplomacy by Combining Language Models with Strategic Reasoning’. In Science 0, no. 0 (22 November 2022): eade9097. https://doi.org/10.1126/science.ade9097 .
This also poses a problem when we evaluate how the intelligence of a machine compares with the intelligence of humans. If intelligence was a general ability, a single capacity, then we could easily compare and evaluate it, but the fact that it is a range of skills makes it much more difficult to compare across machine and human intelligence. Tests for AI systems are therefore comprising a wide range of tasks. See for example Dan Hendrycks, Collin Burns, Steven Basart, Andy Zou, Mantas Mazeika, Dawn Song, Jacob Steinhardt (2020) – Measuring Massive Multitask Language Understanding or the definition of what would qualify as artificial general intelligence in this Metaculus prediction .
An overview of how AI systems can fail can be found in Charles Choi – 7 Revealing Ways AIs Fail . It is also worth reading through the AIAAIC Repository which “details recent incidents and controversies driven by or relating to AI, algorithms, and automation."
I have taken this example from AI researcher François Chollet , who published it here .
Via François Chollet , who published it here . Based on Chollet’s comments it seems that this image was created by the AI system ‘Stable Diffusion’.
This quote is from Holden Karnofsky (2021) – AI Timelines: Where the Arguments, and the "Experts," Stand . For Holden Karnofsky’s earlier thinking on this conceptualization of AI see his 2016 article ‘Some Background on Our Views Regarding Advanced Artificial Intelligence’ .
Ajeya Cotra, whose research on AI timelines I discuss in other articles of this series, attempts to give a quantitative definition of what would qualify as transformative AI. in her widely cited report on AI timelines she defines it as a change in software technology that brings the growth rate of gross world product "to 20%-30% per year". Several other researchers define TAI in similar terms.
Human-level AI is typically defined as a software system that can carry out at least 90% or 99% of all economically relevant tasks that humans carry out. A lower-bar definition would be an AI system that can carry out all those tasks that can currently be done by another human who is working remotely on a computer.
On the use of AI in politically-motivated disinformation campaigns see for example John Villasenor (November 2020) – How to deal with AI-enabled disinformation . More generally on this topic see Brundage and Avin et al. (2018) – The Malicious Use of Artificial Intelligence: Forecasting, Prevention, and Mitigation, published at maliciousaireport.com . A starting point for literature and reporting on mass surveillance by governments is the relevant Wikipedia entry .
See for example the Wikipedia entry on the ‘Dutch childcare benefits scandal’ and Melissa Heikkilä (2022) – ‘Dutch scandal serves as a warning for Europe over risks of using algorithms’ , in Politico. The technology can also reinforce discrimination in terms of race and gender. See Brian Christian’s book The Alignment Problem and the reports of the AI Now Institute .
Overviews are provided in Stuart Russell (2019) – Human Compatible (especially chapter 5) and Brian Christian’s 2020 book The Alignment Problem . Christian presents the thinking of many leading AI researchers from the earliest days up to now and presents an excellent overview of this problem. It is also seen as a large risk by some of the leading private firms who work towards powerful AI – see OpenAI's article " Our approach to alignment research " from August 2022.
Stuart Russell (2019) – Human Compatible
A question that follows from this is, why build such a powerful AI in the first place?
The incentives are very high. As I emphasize below, this innovation has the potential to lead to very positive developments. In addition to the large social benefits there are also large incentives for those who develop it – the governments that can use it for their goals, the individuals who can use it to become more powerful and wealthy. Additionally, it is of scientific interest and might help us to understand our own mind and intelligence better. And lastly, even if we wanted to stop building powerful AIs, it is likely very hard to actually achieve it. It is very hard to coordinate across the whole world and agree to stop building more advanced AI – countries around the world would have to agree and then find ways to actually implement it.
In 1950 the computer science pioneer Alan Turing put it like this: “If a machine can think, it might think more intelligently than we do, and then where should we be? … [T]his new danger is much closer. If it comes at all it will almost certainly be within the next millennium. It is remote but not astronomically remote, and is certainly something which can give us anxiety. It is customary, in a talk or article on this subject, to offer a grain of comfort, in the form of a statement that some particularly human characteristic could never be imitated by a machine. … I cannot offer any such comfort, for I believe that no such bounds can be set.” Alan. M. Turing (1950) – Computing Machinery and Intelligence , In Mind, Volume LIX, Issue 236, October 1950, Pages 433–460.
Norbert Wiener is another pioneer who saw the alignment problem very early. One way he put it was “If we use, to achieve our purposes, a mechanical agency with whose operation we cannot interfere effectively … we had better be quite sure that the purpose put into the machine is the purpose which we really desire.” quoted from Norbert Wiener (1960) – Some Moral and Technical Consequences of Automation: As machines learn they may develop unforeseen strategies at rates that baffle their programmers. In Science.
In 1950 – the same year in which Turing published the cited article – Wiener published his book The Human Use of Human Beings, whose front-cover blurb reads: “The ‘mechanical brain’ and similar machines can destroy human values or enable us to realize them as never before.”
Toby Ord – The Precipice . He makes this projection in footnote 55 of chapter 2. It is based on the 2017 estimate by Farquhar.
Cite this work
Our articles and data visualizations rely on work from many different people and organizations. When citing this article, please also cite the underlying data sources. This article can be cited as:
BibTeX citation
Reuse this work freely
All visualizations, data, and code produced by Our World in Data are completely open access under the Creative Commons BY license . You have the permission to use, distribute, and reproduce these in any medium, provided the source and authors are credited.
The data produced by third parties and made available by Our World in Data is subject to the license terms from the original third-party authors. We will always indicate the original source of the data in our documentation, so you should always check the license of any such third-party data before use and redistribution.
All of our charts can be embedded in any site.
Our World in Data is free and accessible for everyone.
Help us do this work by making a donation.
The present and future of AI
Finale doshi-velez on how ai is shaping our lives and how we can shape ai.
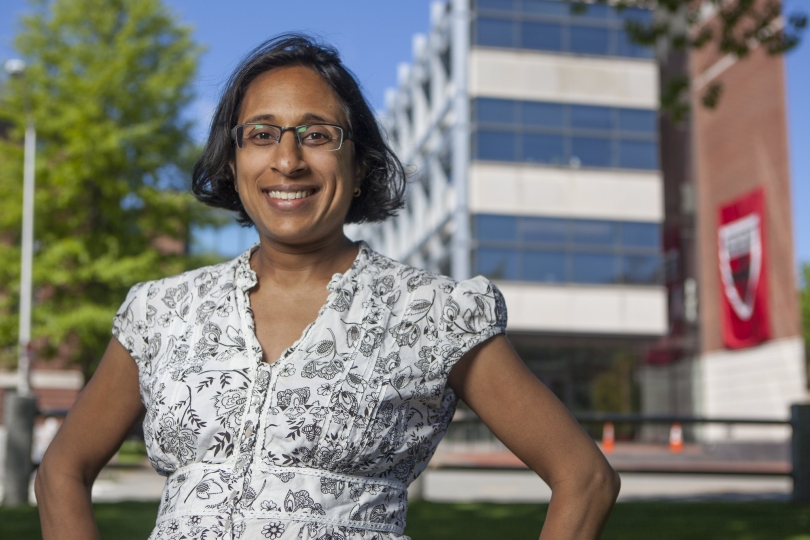
Finale Doshi-Velez, the John L. Loeb Professor of Engineering and Applied Sciences. (Photo courtesy of Eliza Grinnell/Harvard SEAS)
How has artificial intelligence changed and shaped our world over the last five years? How will AI continue to impact our lives in the coming years? Those were the questions addressed in the most recent report from the One Hundred Year Study on Artificial Intelligence (AI100), an ongoing project hosted at Stanford University, that will study the status of AI technology and its impacts on the world over the next 100 years.
The 2021 report is the second in a series that will be released every five years until 2116. Titled “Gathering Strength, Gathering Storms,” the report explores the various ways AI is increasingly touching people’s lives in settings that range from movie recommendations and voice assistants to autonomous driving and automated medical diagnoses .
Barbara Grosz , the Higgins Research Professor of Natural Sciences at the Harvard John A. Paulson School of Engineering and Applied Sciences (SEAS) is a member of the standing committee overseeing the AI100 project and Finale Doshi-Velez , Gordon McKay Professor of Computer Science, is part of the panel of interdisciplinary researchers who wrote this year’s report.
We spoke with Doshi-Velez about the report, what it says about the role AI is currently playing in our lives, and how it will change in the future.
Q: Let's start with a snapshot: What is the current state of AI and its potential?
Doshi-Velez: Some of the biggest changes in the last five years have been how well AIs now perform in large data regimes on specific types of tasks. We've seen [DeepMind’s] AlphaZero become the best Go player entirely through self-play, and everyday uses of AI such as grammar checks and autocomplete, automatic personal photo organization and search, and speech recognition become commonplace for large numbers of people.
In terms of potential, I'm most excited about AIs that might augment and assist people. They can be used to drive insights in drug discovery, help with decision making such as identifying a menu of likely treatment options for patients, and provide basic assistance, such as lane keeping while driving or text-to-speech based on images from a phone for the visually impaired. In many situations, people and AIs have complementary strengths. I think we're getting closer to unlocking the potential of people and AI teams.
There's a much greater recognition that we should not be waiting for AI tools to become mainstream before making sure they are ethical.
Q: Over the course of 100 years, these reports will tell the story of AI and its evolving role in society. Even though there have only been two reports, what's the story so far?
There's actually a lot of change even in five years. The first report is fairly rosy. For example, it mentions how algorithmic risk assessments may mitigate the human biases of judges. The second has a much more mixed view. I think this comes from the fact that as AI tools have come into the mainstream — both in higher stakes and everyday settings — we are appropriately much less willing to tolerate flaws, especially discriminatory ones. There's also been questions of information and disinformation control as people get their news, social media, and entertainment via searches and rankings personalized to them. So, there's a much greater recognition that we should not be waiting for AI tools to become mainstream before making sure they are ethical.
Q: What is the responsibility of institutes of higher education in preparing students and the next generation of computer scientists for the future of AI and its impact on society?
First, I'll say that the need to understand the basics of AI and data science starts much earlier than higher education! Children are being exposed to AIs as soon as they click on videos on YouTube or browse photo albums. They need to understand aspects of AI such as how their actions affect future recommendations.
But for computer science students in college, I think a key thing that future engineers need to realize is when to demand input and how to talk across disciplinary boundaries to get at often difficult-to-quantify notions of safety, equity, fairness, etc. I'm really excited that Harvard has the Embedded EthiCS program to provide some of this education. Of course, this is an addition to standard good engineering practices like building robust models, validating them, and so forth, which is all a bit harder with AI.
I think a key thing that future engineers need to realize is when to demand input and how to talk across disciplinary boundaries to get at often difficult-to-quantify notions of safety, equity, fairness, etc.
Q: Your work focuses on machine learning with applications to healthcare, which is also an area of focus of this report. What is the state of AI in healthcare?
A lot of AI in healthcare has been on the business end, used for optimizing billing, scheduling surgeries, that sort of thing. When it comes to AI for better patient care, which is what we usually think about, there are few legal, regulatory, and financial incentives to do so, and many disincentives. Still, there's been slow but steady integration of AI-based tools, often in the form of risk scoring and alert systems.
In the near future, two applications that I'm really excited about are triage in low-resource settings — having AIs do initial reads of pathology slides, for example, if there are not enough pathologists, or get an initial check of whether a mole looks suspicious — and ways in which AIs can help identify promising treatment options for discussion with a clinician team and patient.
Q: Any predictions for the next report?
I'll be keen to see where currently nascent AI regulation initiatives have gotten to. Accountability is such a difficult question in AI, it's tricky to nurture both innovation and basic protections. Perhaps the most important innovation will be in approaches for AI accountability.
Topics: AI / Machine Learning , Computer Science
Cutting-edge science delivered direct to your inbox.
Join the Harvard SEAS mailing list.
Scientist Profiles
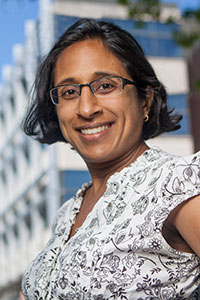
Finale Doshi-Velez
Herchel Smith Professor of Computer Science
Press Contact
Leah Burrows | 617-496-1351 | [email protected]
Related News
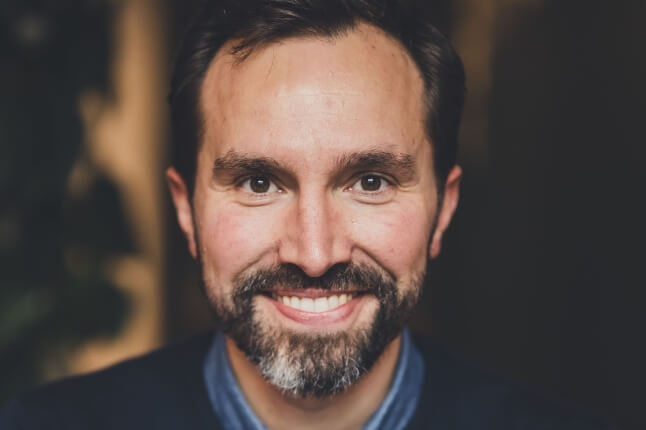
Alumni profile: Jacomo Corbo, Ph.D. '08
Racing into the future of machine learning
AI / Machine Learning , Computer Science
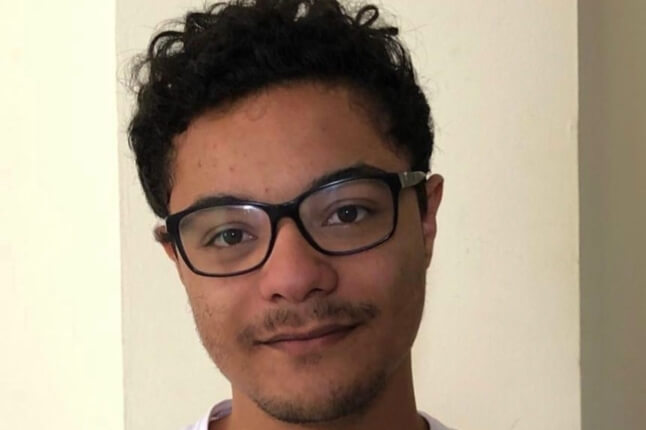
Ph.D. student Monteiro Paes named Apple Scholar in AI/ML
Monteiro Paes studies fairness and arbitrariness in machine learning models
AI / Machine Learning , Applied Mathematics , Awards , Graduate Student Profile
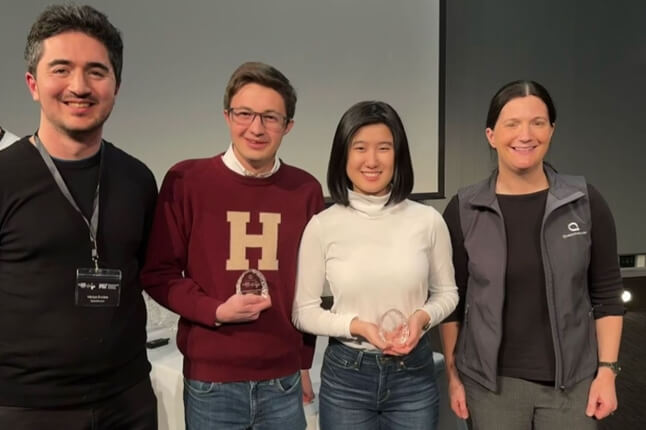
A new phase for Harvard Quantum Computing Club
SEAS students place second at MIT quantum hackathon
Computer Science , Quantum Engineering , Undergraduate Student Profile
- Reference Manager
- Simple TEXT file
People also looked at
Conceptual analysis article, human- versus artificial intelligence.
- TNO Human Factors, Soesterberg, Netherlands
AI is one of the most debated subjects of today and there seems little common understanding concerning the differences and similarities of human intelligence and artificial intelligence. Discussions on many relevant topics, such as trustworthiness, explainability, and ethics are characterized by implicit anthropocentric and anthropomorphistic conceptions and, for instance, the pursuit of human-like intelligence as the golden standard for Artificial Intelligence. In order to provide more agreement and to substantiate possible future research objectives, this paper presents three notions on the similarities and differences between human- and artificial intelligence: 1) the fundamental constraints of human (and artificial) intelligence, 2) human intelligence as one of many possible forms of general intelligence, and 3) the high potential impact of multiple (integrated) forms of narrow-hybrid AI applications. For the time being, AI systems will have fundamentally different cognitive qualities and abilities than biological systems. For this reason, a most prominent issue is how we can use (and “collaborate” with) these systems as effectively as possible? For what tasks and under what conditions, decisions are safe to leave to AI and when is human judgment required? How can we capitalize on the specific strengths of human- and artificial intelligence? How to deploy AI systems effectively to complement and compensate for the inherent constraints of human cognition (and vice versa)? Should we pursue the development of AI “partners” with human (-level) intelligence or should we focus more at supplementing human limitations? In order to answer these questions, humans working with AI systems in the workplace or in policy making have to develop an adequate mental model of the underlying ‘psychological’ mechanisms of AI. So, in order to obtain well-functioning human-AI systems, Intelligence Awareness in humans should be addressed more vigorously. For this purpose a first framework for educational content is proposed.
Introduction: Artificial and Human Intelligence, Worlds of Difference
Artificial general intelligence at the human level.
Recent advances in information technology and in AI may allow for more coordination and integration between of humans and technology. Therefore, quite some attention has been devoted to the development of Human-Aware AI, which aims at AI that adapts as a “team member” to the cognitive possibilities and limitations of the human team members. Also metaphors like “mate,” “partner,” “alter ego,” “Intelligent Collaborator,” “buddy” and “mutual understanding” emphasize a high degree of collaboration, similarity, and equality in “hybrid teams”. When human-aware AI partners operate like “human collaborators” they must be able to sense, understand, and react to a wide range of complex human behavioral qualities, like attention, motivation, emotion, creativity, planning, or argumentation, (e.g. Krämer et al., 2012 ; van den Bosch and Bronkhorst, 2018 ; van den Bosch et al., 2019 ). Therefore these “AI partners,” or “team mates” have to be endowed with human-like (or humanoid) cognitive abilities enabling mutual understanding and collaboration (i.e. “human awareness”).
However, no matter how intelligent and autonomous AI agents become in certain respects, at least for the foreseeable future, they probably will remain unconscious machines or special-purpose devices that support humans in specific, complex tasks. As digital machines they are equipped with a completely different operating system (digital vs biological) and with correspondingly different cognitive qualities and abilities than biological creatures, like humans and other animals ( Moravec, 1988 ; Klein et al., 2004 ; Korteling et al., 2018a ; Shneiderman, 2020a ). In general, digital reasoning- and problem-solving agents only compare very superficially to their biological counterparts, (e.g. Boden, 2017 ; Shneiderman, 2020b ). Keeping that in mind, it becomes more and more important that human professionals working with advanced AI systems, (e.g. in military‐ or policy making teams) develop a proper mental model about the different cognitive capacities of AI systems in relation to human cognition. This issue will become increasingly relevant when AI systems become more advanced and are deployed with higher degrees of autonomy. Therefore, the present paper tries to provide some more clarity and insight into the fundamental characteristics, differences and idiosyncrasies of human/biological and artificial/digital intelligences. In the final section, a global framework for constructing educational content on this “Intelligence Awareness” is introduced. This can be used for the development of education and training programs for humans who have to use or “collaborate with” advanced AI systems in the near and far future.
With the application of AI systems with increasing autonomy more and more researchers consider the necessity of vigorously addressing the real complex issues of “human-level intelligence” and more broadly artificial general intelligence , or AGI, (e.g. Goertzel et al., 2014 ). Many different definitions of A(G)I have already been proposed, (e.g. Russell and Norvig, 2014 for an overview). Many of them boil down to: technology containing or entailing (human-like) intelligence , (e.g. Kurzweil, 1990 ). This is problematic. Most definitions use the term “intelligence”, as an essential element of the definition itself, which makes the definition tautological. Second, the idea that A(G)I should be human-like seems unwarranted. At least in natural environments there are many other forms and manifestations of highly complex and intelligent behaviors that are very different from specific human cognitive abilities (see Grind, 1997 for an overview). Finally, like what is also frequently seen in the field of biology, these A(G)I definitions use human intelligence as a central basis or analogy for reasoning about the—less familiar—phenomenon of A(G)I ( Coley and Tanner, 2012 ). Because of the many differences between the underlying substrate and architecture of biological and artificial intelligence this anthropocentric way of reasoning is probably unwarranted. For these reasons we propose a (non-anthropocentric) definition of “intelligence” as: “ the capacity to realize complex goals ” ( Tegmark, 2017 ). These goals may pertain to narrow, restricted tasks (narrow AI) or to broad task domains (AGI). Building on this definition, and on a definition of AGI proposed by Bieger et al. (2014) and one of Grind (1997) , we define AGI here as: “ Non-biological capacities to autonomously and efficiently achieve complex goals in a wide range of environments”. AGI systems should be able to identify and extract the most important features for their operation and learning process automatically and efficiently over a broad range of tasks and contexts. Relevant AGI research differs from the ordinary AI research by addressing the versatility and wholeness of intelligence, and by carrying out the engineering practice according to a system comparable to the human mind in a certain sense ( Bieger et al., 2014 ).
It will be fascinating to create copies of ourselves which can learn iteratively by interaction with partners and thus become able to collaborate on the basis of common goals and mutual understanding and adaptation, (e.g. Bradshaw et al., 2012 ; Johnson et al., 2014 ). This would be very useful, for example when a high degree of social intelligence of AI will contribute to more adequate interactions with humans, for example in health care or for entertainment purposes ( Wyrobek et al., 2008 ). True collaboration on the basis of common goals and mutual understanding necessarily implies some form of humanoid general intelligence. For the time being, this remains a goal on a far-off horizon. In the present paper we argue why for most applications it also may not be very practical or necessary (and probably a bit misleading) to vigorously aim or to anticipate on systems possessing “human-like” AGI or “human-like” abilities or qualities. The fact that humans possess general intelligence does not imply that new inorganic forms of general intelligence should comply to the criteria of human intelligence. In this connection, the present paper addresses the way we think about (natural and artificial) intelligence in relation to the most probable potentials (and real upcoming issues) of AI in the short- and mid-term future. This will provide food for thought in anticipation of a future that is difficult to predict for a field as dynamic as AI.
What Is “Real Intelligence”?
Implicit in our aspiration of constructing AGI systems possessing humanoid intelligence is the premise that human (general) intelligence is the “real” form of intelligence. This is even already implicitly articulated in the term “Artificial Intelligence”, as if it were not entirely real, i.e., real like non-artificial (biological) intelligence. Indeed, as humans we know ourselves as the entities with the highest intelligence ever observed in the Universe. And as an extension of this, we like to see ourselves as rational beings who are able to solve a wide range of complex problems under all kinds of circumstances using our experience and intuition, supplemented by the rules of logic, decision analysis and statistics. It is therefore not surprising that we have some difficulty to accept the idea that we might be a bit less smart than we keep on telling ourselves, i.e., “the next insult for humanity” ( van Belkom, 2019 ). This goes as far that the rapid progress in the field of artificial intelligence is accompanied by a recurring redefinition of what should be considered “real (general) intelligence.” The conceptualization of intelligence, that is, the ability to autonomously and efficiently achieve complex goals, is then continuously adjusted and further restricted to: “those things that only humans can do.” In line with this, AI is then defined as “the study of how to make computers do things at which, at the moment, people are better” ( Rich and Knight, 1991 ; Rich et al., 2009 ). This includes thinking of creative solutions, flexibly using contextual- and background information, the use of intuition and feeling, the ability to really “think and understand,” or the inclusion of emotion in an (ethical) consideration. These are then cited as the specific elements of real intelligence, (e.g. Bergstein, 2017 ). For instance, Facebook’s director of AI and a spokesman in the field, Yann LeCun, mentioned at a Conference at MIT on the Future of Work that machines are still far from having “the essence of intelligence.” That includes the ability to understand the physical world well enough to make predictions about basic aspects of it—to observe one thing and then use background knowledge to figure out what other things must also be true. Another way of saying this is that machines don’t have common sense ( Bergstein, 2017 ), like submarines that cannot swim ( van Belkom, 2019 ). When exclusive human capacities become our pivotal navigation points on the horizon we may miss some significant problems that may need our attention first.
To make this point clear, we first will provide some insight into the basic nature of both human and artificial intelligence. This is necessary for the substantiation of an adequate awareness of intelligence ( Intelligence Awareness ), and adequate research and education anticipating the development and application of A(G)I. For the time being, this is based on three essential notions that can (and should) be further elaborated in the near future.
• With regard to cognitive tasks, we are probably less smart than we think. So why should we vigorously focus on human -like AGI?
• Many different forms of intelligence are possible and general intelligence is therefore not necessarily the same as humanoid general intelligence (or “AGI on human level”).
• AGI is often not necessary; many complex problems can also be tackled effectively using multiple narrow AI’s. 1
We Are Probably Not so Smart as We Think
How intelligent are we actually? The answer to that question is determined to a large extent by the perspective from which this issue is viewed, and thus by the measures and criteria for intelligence that is chosen. For example, we could compare the nature and capacities of human intelligence with other animal species. In that case we appear highly intelligent. Thanks to our enormous learning capacity, we have by far the most extensive arsenal of cognitive abilities 2 to autonomously solve complex problems and achieve complex objectives. This way we can solve a huge variety of arithmetic, conceptual, spatial, economic, socio-organizational, political, etc. problems. The primates—which differ only slightly from us in genetic terms—are far behind us in that respect. We can therefore legitimately qualify humans, as compared to other animal species that we know, as highly intelligent.
Limited Cognitive Capacity
However, we can also look beyond this “ relative interspecies perspective” and try to qualify our intelligence in more absolute terms, i.e., using a scale ranging from zero to what is physically possible. For example, we could view the computational capacity of a human brain as a physical system ( Bostrom, 2014 ; Tegmark, 2017 ). The prevailing notion in this respect among AI scientists is that intelligence is ultimately a matter of information and computation, and (thus) not of flesh and blood and carbon atoms. In principle, there is no physical law preventing that physical systems (consisting of quarks and atoms, like our brain) can be built with a much greater computing power and intelligence than the human brain. This would imply that there is no insurmountable physical reason why machines one day cannot become much more intelligent than ourselves in all possible respects ( Tegmark, 2017 ). Our intelligence is therefore relatively high compared to other animals, but in absolute terms it may be very limited in its physical computing capacity, albeit only by the limited size of our brain and its maximal possible number of neurons and glia cells, (e.g. Kahle, 1979 ).
To further define and assess our own (biological) intelligence, we can also discuss the evolution and nature of our biological thinking abilities. As a biological neural network of flesh and blood, necessary for survival, our brain has undergone an evolutionary optimization process of more than a billion years. In this extended period, it developed into a highly effective and efficient system for regulating essential biological functions and performing perceptive-motor and pattern-recognition tasks, such as gathering food, fighting and flighting, and mating. Almost during our entire evolution, the neural networks of our brain have been further optimized for these basic biological and perceptual motor processes that also lie at the basis of our daily practical skills, like cooking, gardening, or household jobs. Possibly because of the resulting proficiency for these kinds of tasks we may forget that these processes are characterized by extremely high computational complexity, (e.g. Moravec, 1988 ). For example, when we tie our shoelaces, many millions of signals flow in and out through a large number of different sensor systems, from tendon bodies and muscle spindles in our extremities to our retina, otolithic organs and semi-circular channels in the head, (e.g. Brodal, 1981 ). This enormous amount of information from many different perceptual-motor systems is continuously, parallel, effortless and even without conscious attention, processed in the neural networks of our brain ( Minsky, 1986 ; Moravec, 1988 ; Grind, 1997 ). In order to achieve this, the brain has a number of universal (inherent) working mechanisms, such as association and associative learning ( Shatz, 1992 ; Bar, 2007 ), potentiation and facilitation ( Katz and Miledi, 1968 ; Bao et al., 1997 ), saturation and lateral inhibition ( Isaacson and Scanziani, 2011 ; Korteling et al., 2018a ).
These kinds of basic biological and perceptual-motor capacities have been developed and set down over many millions of years. Much later in our evolution—actually only very recently—our cognitive abilities and rational functions have started to develop. These cognitive abilities, or capacities, are probably less than 100 thousand years old, which may be qualified as “embryonal” on the time scale of evolution, (e.g. Petraglia and Korisettar, 1998 ; McBrearty and Brooks, 2000 ; Henshilwood and Marean, 2003 ). In addition, this very thin layer of human achievement has necessarily been built on these “ancient” neural intelligence for essential survival functions. So, our “higher” cognitive capacities are developed from and with these (neuro) biological regulation mechanisms ( Damasio, 1994 ; Korteling and Toet, 2020 ). As a result, it should not be a surprise that the capacities of our brain for performing these recent cognitive functions are still rather limited. These limitations are manifested in many different ways, for instance:
‐The amount of cognitive information that we can consciously process (our working memory, span or attention) is very limited ( Simon, 1955 ). The capacity of our working memory is approximately 10–50 bits per second ( Tegmark, 2017 ).
‐Most cognitive tasks, like reading text or calculation, require our full attention and we usually need a lot of time to execute them. Mobile calculators can perform millions times more complex calculations than we can ( Tegmark, 2017 ).
‐Although we can process lots of information in parallel, we cannot simultaneously execute cognitive tasks that require deliberation and attention, i.e., “multi-tasking” ( Korteling, 1994 ; Rogers and Monsell, 1995 ; Rubinstein, Meyer, and Evans, 2001 ).
‐Acquired cognitive knowledge and skills of people (memory) tend to decay over time, much more than perceptual-motor skills. Because of this limited “retention” of information we easily forget substantial portions of what we have learned ( Wingfield and Byrnes, 1981 ).
Ingrained Cognitive Biases
Our limited processing capacity for cognitive tasks is not the only factor determining our cognitive intelligence. Except for an overall limited processing capacity, human cognitive information processing shows systematic distortions. These are manifested in many cognitive biases ( Tversky and Kahneman, 1973 , Tversky and Kahneman, 1974 ). Cognitive biases are systematic, universally occurring tendencies, inclinations, or dispositions that skew or distort information processes in ways that make their outcome inaccurate, suboptimal, or simply wrong, (e.g. Lichtenstein and Slovic, 1971 ; Tversky and Kahneman, 1981 ). Many biases occur in virtually the same way in many different decision situations ( Shafir and LeBoeuf, 2002 ; Kahneman, 2011 ; Toet et al., 2016 ). The literature provides descriptions and demonstrations of over 200 biases. These tendencies are largely implicit and unconscious and feel quite naturally and self/evident when we are aware of these cognitive inclinations ( Pronin et al., 2002 ; Risen, 2015 ; Korteling et al., 2018b ). That is why they are often termed “intuitive” ( Kahneman and Klein, 2009 ) or “irrational” ( Shafir and LeBoeuf, 2002 ). Biased reasoning can result in quite acceptable outcomes in natural or everyday situations, especially when the time cost of reasoning is taken into account ( Simon, 1955 ; Gigerenzer and Gaissmaier, 2011 ). However, people often deviate from rationality and/or the tenets of logic, calculation, and probability in inadvisable ways ( Tversky and Kahneman, 1974 ; Shafir and LeBoeuf, 2002 ) leading to suboptimal decisions in terms of invested time and effort (costs) given the available information and expected benefits.
Biases are largely caused by inherent (or structural) characteristics and mechanisms of the brain as a neural network ( Korteling et al., 2018a ; Korteling and Toet, 2020 ). Basically, these mechanisms—such as association, facilitation, adaptation, or lateral inhibition—result in a modification of the original or available data and its processing, (e.g. weighting its importance). For instance, lateral inhibition is a universal neural process resulting in the magnification of differences in neural activity (contrast enhancement), which is very useful for perceptual-motor functions, maintaining physical integrity and allostasis, (i.e. biological survival functions). For these functions our nervous system has been optimized for millions of years. However, “higher” cognitive functions, like conceptual thinking, probability reasoning or calculation, have been developed only very recently in evolution. These functions are probably less than 100 thousand years old, and may, therefore, be qualified as “embryonal” on the time scale of evolution, (e.g. McBrearty and Brooks, 2000 ; Henshilwood and Marean, 2003 ; Petraglia and Korisettar, 2003 ). In addition, evolution could not develop these new cognitive functions from scratch, but instead had to build this embryonal, and thin layer of human achievement from its “ancient” neural heritage for the essential biological survival functions ( Moravec, 1988 ). Since cognitive functions typically require exact calculation and proper weighting of data, data transformations—like lateral inhibition—may easily lead to systematic distortions, (i.e. biases) in cognitive information processing. Examples of the large number of biases caused by the inherent properties of biological neural networks are: Anchoring bias (biasing decisions toward previously acquired information, Furnham and Boo, 2011 ; Tversky and Kahneman, 1973 , Tversky and Kahneman, 1974 ), the Hindsight bias (the tendency to erroneously perceive events as inevitable or more likely once they have occurred, Hoffrage et al., 2000 ; Roese and Vohs, 2012 ) the Availability bias (judging the frequency, importance, or likelihood of an event by the ease with which relevant instances come to mind, Tversky and Kahnemann, 1973 ; Tversky and Kahneman, 1974 ), and the Confirmation bias (the tendency to select, interpret, and remember information in a way that confirms one’s preconceptions, views, and expectations, Nickerson, 1998 ). In addition to these inherent (structural) limitations of (biological) neural networks, biases may also originate from functional evolutionary principles promoting the survival of our ancestors who, as hunter-gatherers, lived in small, close-knit groups ( Haselton et al., 2005 ; Tooby and Cosmides, 2005 ). Cognitive biases can be caused by a mismatch between evolutionarily rationalized “heuristics” (“evolutionary rationality”: Haselton et al., 2009 ) and the current context or environment ( Tooby and Cosmides, 2005 ). In this view, the same heuristics that optimized the chances of survival of our ancestors in their (natural) environment can lead to maladaptive (biased) behavior when they are used in our current (artificial) settings. Biases that have been considered as examples of this kind of mismatch are the Action bias (preferring action even when there is no rational justification to do this, Baron and Ritov, 2004 ; Patt and Zeckhauser, 2000 ), Social proof (the tendency to mirror or copy the actions and opinions of others, Cialdini, 1984 ), the Tragedy of the commons (prioritizing personal interests over the common good of the community, Hardin, 1968 ), and the Ingroup bias (favoring one’s own group above that of others, Taylor and Doria, 1981 ).
This hard-wired (neurally inherent and/or evolutionary ingrained) character of biased thinking makes it unlikely that simple and straightforward methods like training interventions or awareness courses will be very effective to ameliorate biases. This difficulty of bias mitigation seems indeed supported by the literature ( Korteling et al., 2021 ).
General Intelligence Is Not the Same as Human-like Intelligence
Fundamental differences between biological and artificial intelligence.
We often think and deliberate about intelligence with an anthropocentric conception of our own intelligence in mind as an obvious and unambiguous reference. We tend to use this conception as a basis for reasoning about other, less familiar phenomena of intelligence, such as other forms of biological and artificial intelligence ( Coley and Tanner, 2012 ). This may lead to fascinating questions and ideas. An example is the discussion about how and when the point of “intelligence at human level” will be achieved. For instance, Ackermann. (2018) writes: “Before reaching superintelligence, general AI means that a machine will have the same cognitive capabilities as a human being”. So, researchers deliberate extensively about the point in time when we will reach general AI, (e.g., Goertzel, 2007 ; Müller and Bostrom, 2016 ). We suppose that these kinds of questions are not quite on target. There are (in principle) many different possible types of (general) intelligence conceivable of which human-like intelligence is just one of those. This means, for example that the development of AI is determined by the constraint of physics and technology, and not by those of biological evolution. So, just as the intelligence of a hypothetical extraterrestrial visitor of our planet earth is likely to have a different (in-)organic structure with different characteristics, strengths, and weaknesses, than the human residents this will also apply to artificial forms of (general) intelligence. Below we briefly summarize a few fundamental differences between human and artificial intelligence ( Bostrom, 2014 ):
‐Basic structure: Biological (carbon) intelligence is based on neural “wetware” which is fundamentally different from artificial (silicon-based) intelligence. As opposed to biological wetware, in silicon, or digital, systems “hardware” and “software” are independent of each other ( Kosslyn and Koenig, 1992 ). When a biological system has learned a new skill, this will be bounded to the system itself. In contrast, if an AI system has learned a certain skill then the constituting algorithms can be directly copied to all other similar digital systems.
‐Speed: Signals from AI systems propagate with almost the speed of light. In humans, the conduction velocity of nerves proceeds with a speed of at most 120 m/s, which is extremely slow in the time scale of computers ( Siegel and Sapru, 2005 ).
‐Connectivity and communication: People cannot directly communicate with each other. They communicate via language and gestures with limited bandwidth. This is slower and more difficult than the communication of AI systems that can be connected directly to each other. Thanks to this direct connection, they can also collaborate on the basis of integrated algorithms.
‐Updatability and scalability: AI systems have almost no constraints with regard to keep them up to date or to upscale and/or re-configure them, so that they have the right algorithms and the data processing and storage capacities necessary for the tasks they have to carry out. This capacity for rapid, structural expansion and immediate improvement hardly applies to people.
‐In contrast, biology does a lot with a little: organic brains are millions of times more efficient in energy consumption than computers. The human brain consumes less energy than a lightbulb, whereas a supercomputer with comparable computational performance uses enough electricity to power quite a village ( Fischetti, 2011 ).
These kinds of differences in basic structure, speed, connectivity, updatability, scalability, and energy consumption will necessarily also lead to different qualities and limitations between human and artificial intelligence. Our response speed to simple stimuli is, for example, many thousands of times slower than that of artificial systems. Computer systems can very easily be connected directly to each other and as such can be part of one integrated system. This means that AI systems do not have to be seen as individual entities that can easily work alongside each other or have mutual misunderstandings. And if two AI systems are engaged in a task then they run a minimal risk to make a mistake because of miscommunications (think of autonomous vehicles approaching a crossroad). After all, they are intrinsically connected parts of the same system and the same algorithm ( Gerla et al., 2014 ).
Complexity and Moravec’s Paradox
Because biological, carbon-based, brains and digital, silicon-based, computers are optimized for completely different kinds of tasks (e.g., Moravec, 1988 ; Korteling et al., 2018b ), human and artificial intelligence show fundamental and probably far-stretching differences. Because of these differences it may be very misleading to use our own mind as a basis, model or analogy for reasoning about AI. This may lead to erroneous conceptions, for example about the presumed abilities of humans and AI to perform complex tasks. Resulting flaws concerning information processing capacities emerge often in the psychological literature in which “complexity” and “difficulty” of tasks are used interchangeably (see for examples: Wood et al., 1987 ; McDowd and Craik, 1988 ). Task complexity is then assessed in an anthropocentric way, that is: by the degree to which we humans can perform or master it. So, we use the difficulty to perform or master a task as a measure of its complexity , and task performance (speed, errors) as a measure of skill and intelligence of the task performer. Although this could sometimes be acceptable in psychological research, this may be misleading if we strive for understanding the intelligence of AI systems. For us it is much more difficult to multiply two random numbers of six digits than to recognize a friend on a photograph. But when it comes to counting or arithmetic operations, computers are thousands of times faster and better, while the same systems have only recently taken steps in image recognition (which only succeeded when deep learning technology, based on some principles of biological neural networks, was developed). In general: cognitive tasks that are relatively difficult for the human brain (and which we therefore find subjectively difficult) do not have to be computationally complex, (e.g., in terms of objective arithmetic, logic, and abstract operations). And vice versa: tasks that are relatively easy for the brain (recognizing patterns, perceptual-motor tasks, well-trained tasks) do not have to be computationally simple. This phenomenon, that which is easy for the ancient, neural “technology” of people and difficult for the modern, digital technology of computers (and vice versa) has been termed the moravec’s Paradox. Hans Moravec (1988) wrote: “It is comparatively easy to make computers exhibit adult level performance on intelligence tests or playing checkers, and difficult or impossible to give them the skills of a one-year-old when it comes to perception and mobility.”
Human Superior Perceptual-Motor Intelligence
Moravec’s paradox implies that biological neural networks are intelligent in different ways than artificial neural networks. Intelligence is not limited to the problems or goals that we as humans, equipped with biological intelligence, find difficult ( Grind, 1997 ). Intelligence, defined as the ability to realize complex goals or solve complex problems, is much more than that. According to Moravec (1988) high-level reasoning requires very little computation, but low-level perceptual-motor skills require enormous computational resources. If we express the complexity of a problem in terms of the number of elementary calculations needed to solve it, then our biological perceptual motor intelligence is highly superior to our cognitive intelligence. Our organic perceptual-motor intelligence is especially good at associative processing of higher-order invariants (“patterns”) in the ambient information. These are computationally more complex and contain more information than the simple, individual elements ( Gibson, 1966 , Gibson, 1979 ). An example of our superior perceptual-motor abilities is the Object Superiority Effect : we perceive and interpret whole objects faster and more effective than the (more simple) individual elements that make up these objects ( Weisstein and Harris, 1974 ; McClelland, 1978 ; Williams and Weisstein, 1978 ; Pomerantz, 1981 ). Thus, letters are also perceived more accurately when presented as part of a word than when presented in isolation, i.e. the Word superiority effect, (e.g. Reicher, 1969 ; Wheeler, 1970 ). So, the difficulty of a task does not necessarily indicate its inherent complexity . As Moravec (1988) puts it: “We are all prodigious Olympians in perceptual and motor areas, so good that we make the difficult look easy. Abstract thought, though, is a new trick, perhaps less than 100 thousand years old. We have not yet mastered it. It is not all that intrinsically difficult; it just seems so when we do it.”
The Supposition of Human-like AGI
So, if there would exist AI systems with general intelligence that can be used for a wide range of complex problems and objectives, those AGI machines would probably have a completely different intelligence profile, including other cognitive qualities, than humans have ( Goertzel, 2007 ). This will be even so, if we manage to construct AI agents who display similar behavior like us and if they are enabled to adapt to our way of thinking and problem-solving in order to promote human-AI teaming. Unless we decide to deliberately degrade the capabilities of AI systems (which would not be very smart), the underlying capacities and abilities of man and machines with regard to collection and processing of information, data analysis, probability reasoning, logic, memory capacity etc. will still remain dissimilar. Because of these differences we should focus at systems that effectively complement us, and that make the human-AI system stronger and more effective. Instead of pursuing human-level AI it would be more beneficial to focus on autonomous machines and (support) systems that fill in, or extend on, the manifold gaps of human cognitive intelligence. For instance, whereas people are forced—by the slowness and other limitations of biological brains—to think heuristically in terms of goals, virtues, rules and norms expressed in (fuzzy) language, AI has already established excellent capacities to process and calculate directly on highly complex data. Therefore, or the execution of specific (narrow) cognitive tasks (logical, analytical, computational), modern digital intelligence may be more effective and efficient than biological intelligence. AI may thus help to produce better answers for complex problems using high amounts of data, consistent sets of ethical principles and goals, probabilistic-, and logic reasoning, (e.g. Korteling et al., 2018b ). Therefore, we conjecture that ultimately the development of AI systems for supporting human decision making may appear the most effective way leading to the making of better choices or the development of better solutions on complex issues. So, the cooperation and division of tasks between people and AI systems will have to be primarily determinated by their mutually specific qualities. For example, tasks or task components that appeal to capacities in which AI systems excel, will have to be less (or less fully) mastered by people, so that less training will probably be required. AI systems are already much better than people at logically and arithmetically correct gathering (selecting) and processing (weighing, prioritizing, analyzing, combining) large amounts of data. They do this quickly, accurately and reliably. They are also more stable (consistent) than humans, have no stress and emotions and have a great perseverance and a much better retention of knowledge and skills than people. As a machine, they serve people completely and without any “self-interest” or “own hidden agenda.” Based on these qualities AI systems may effectively take over tasks, or task components, from people. However, it remains important that people continue to master those tasks to a certain extent, so that they can take over tasks or take adequate action if the machine system fails.
In general, people are better suited than AI systems for a much broader spectrum of cognitive and social tasks under a wide variety of (unforeseen) circumstances and events ( Korteling et al., 2018b ). People are also better at the social-psychosocial interaction for the time being. For example, it is difficult for AI systems to interpret human language and -symbolism. This requires a very extensive frame of reference, which, at least until now and for the near future, is difficult to achieve within AI. As a result of all these differences, people are still better at responding (as a flexible team) to unexpected and unpredictable situations and creatively devising possibilities and solutions in open and ill-defined tasks and across a wide range of different, and possibly unexpected, circumstances. People will have to make extra use of their specific human qualities, (i.e. what people are relatively good at) and train to improve relevant competencies. In addition, human team members will have to learn to deal well with the overall limitations of AIs. With such a proper division of tasks, capitalizing on the specific qualities and limitations of humans and AI systems, human decisional biases may be circumvented and better performance may be expected. This means that enhancement of a team with intelligent machines having less cognitive constraints and biases, may have more surplus value than striving at collaboration between humans and AI that have developed the same (human) biases. Although cooperation in teams with AI systems may need extra training in order to effectively deal with this bias-mismatch, this heterogeneity will probably be better and safer. This also opens up the possibility of a combination of high levels of meaningful human control AND high levels of automation which is likely to produce the most effective and safe human-AI systems ( Elands et al., 2019 ; Shneiderman, 2020a ). In brief: human intelligence is not the golden standard for general intelligence; instead of aiming at human-like AGI, the pursuit of AGI should thus focus on effective digital/silicon AGI in conjunction with an optimal configuration and allocation of tasks.
Explainability and Trust
Developments in relation to artificial learning, or deep (reinforcement) learning, in particular have been revolutionary. Deep learning simulates a network resembling the layered neural networks of our brain. Based on large quantities of data, the network learns to recognize patterns and links to a high level of accuracy and then connect them to courses of action without knowing the underlying causal links. This implies that it is difficult to provide deep learning AI with some kind of transparency in how or why it has made a particular choice by, for example, by expressing an intelligible reasoning (for humans) about its decision process, like we do, (e.g. Belkom, 2019 ). In addition, reasoning about decisions like humans do is a very malleable and ad hoc process (at least in humans). Humans are generally unaware of their implicit cognitions or attitudes, and therefore not be able to adequately report on them. It is therefore rather difficult for many humans to introspectively analyze their mental states, as far as these are conscious, and attach the results of this analysis to verbal labels and descriptions, (e.g. Nosek et al. (2011) . First, the human brain hardly reveals how it creates conscious thoughts, (e.g. Feldman-Barret, 2017 ). What it actually does is giving us the illusion that its products reveal its inner workings. In other words: our conscious thoughts tell us nothing about the way in which these thoughts came about. There is also no subjective marker that distinguishes correct reasoning processes from erroneous ones ( Kahneman and Klein, 2009 ). The decision maker therefore has no way to distinguish between correct thoughts, emanating from genuine knowledge and expertize, and incorrect ones following from inappropriate neuro-evolutionary processes, tendencies, and primal intuitions. So here we could ask the question: isn’t it more trustworthy to have a real black box, than to listen to a confabulating one? In addition, according to Werkhoven et al. (2018) demanding explainability observability, or transparency ( Belkom, 2019 ; van den Bosch et al., 2019 ) may cause artificial intelligent systems to constrain their potential benefit for human society, to what can be understood by humans.
Of course we should not blindly trust the results generated by AI. Like other fields of complex technology, (e.g. Modeling & Simulation), AI systems need to be verified (meeting specifications) and validated (meeting the systems’ goals) with regard to the objectives for which the system was designed. In general, when a system is properly verified and validated, it may be considered safe, secure and fit for purpose. It therefore deserves our trust for (logically) comprehensible and objective reasons (although mistakes still can happen). Likewise people trust in the performance of aero planes and cell phones despite we are almost completely ignorant about their complex inner processes. Like our own brains, artificial neural networks are fundamentally intransparant ( Nosek et al., 2011 ; Feldman-Barret, 2017 ). Therefore, trust in AI should be primarily based on its objective performance. This forms a more important base than providing trust on the basis of subjective (trickable) impressions, stories, or images aimed at belief and appeal to the user. Based on empirical validation research, developers and users can explicitly verify how well the system is doing with respect to the set of values and goals for which the machine was designed. At some point, humans may want to trust that goals can be achieved against less cost and better outcomes, when we accept solutions even if they may be less transparent for humans ( Werkhoven et al., 2018 ).
The Impact of Multiple Narrow AI Technology
Agi as the holy grail.
AGI, like human general intelligence, would have many obvious advantages, compared to narrow (limited, weak, specialized) AI. An AGI system would be much more flexible and adaptive. On the basis of generic training and reasoning processes it would understand autonomously how multiple problems in all kinds of different domains can be solved in relation to their context, (e.g. Kurzweil, 2005 ). AGI systems also require far fewer human interventions to accommodate the various loose ends among partial elements, facets, and perspectives in complex situations. AGI would really understand problems and is capable to view them from different perspectives (as people—ideally—also can do). A characteristic of the current (narrow) AI tools is that they are skilled in a very specific task, where they can often perform at superhuman levels, (e.g. Goertzel, 2007 ; Silver et al., 2017 ). These specific tasks have been well-defined and structured. Narrow AI systems are less suitable, or totally unsuitable, for tasks or task environments that offer little structure, consistency, rules or guidance, in which all sorts of unexpected, rare or uncommon events, (e.g. emergencies) may occur. Knowing and following fixed procedures usually does not lead to proper solutions in these varying circumstances. In the context of (unforeseen) changes in goals or circumstances, the adequacy of current AI is considerably reduced because it cannot reason from a general perspective and adapt accordingly ( Lake et al., 2017 ; Horowitz, 2018 ). As with narrow AI systems, people are then needed to supervise on these deviations in order to enable flexible and adaptive system performance. Therefore the quest of AGI may be considered as looking for a kind of holy grail.
Multiple Narrow AI is Most Relevant Now!
The potential high prospects of AGI, however, do not imply that AGI will be the most crucial factor in future AI R&D, at least for the short- and mid-term. When reflecting on the great potential benefits of general intelligence, we tend to consider narrow AI applications as separate entities that can very well be outperformed by a broader AGI that presumably can deal with everything. But just as our modern world has evolved rapidly through a diversity of specific (limited) technological innovations, at the system level the total and wide range of emerging AI applications will also have a groundbreaking technological and societal impact ( Peeters et al., 2020 ). This will be all the more relevant for the future world of big data, in which everything is connected to everything through the Internet of Things . So, it will be much more profitable and beneficial to develop and build (non-human-like) AI variants that will excel in areas where people are inherently limited. It seems not too far-fetched to suppose that the multiple variants of narrow AI applications also gradually get more broadly interconnected. In this way, a development toward an ever broader realm of integrated AI applications may be expected. In addition, it is already possible to train a language model AI (Generative Pre-trained Transformer3, GPT-3) with a gigantic dataset and then have it learn various tasks based on a handful of examples—one or few-shot learning. GPT-3 (developed by OpenAI) can do this with language-related tasks, but there is no reason why this should not be possible with image and sound, or with combinations of these three ( Brown, 2020 ).
Besides, the moravec Paradox implies that the development of AI “partners” with many kinds of human (-level) qualities will be very difficult to obtain, whereas their added value, (i.e. beyond the boundaries of human capabilities) will be relatively low. The most fruitful AI applications will mainly involve supplementing human constraints and limitations. Given the present incentives for competitive technological progress, multiple forms of (connected) narrow AI systems will be the major driver of AI impact on our society for short- and mid-term. For the near future, this may imply that AI applications will remain very different from, and in many aspects almost incomparable with, human agents. This is likely to be true even if the hypothetical match of artificial general intelligence (AGI) with human cognition were to be achieved in the future in the longer term. Intelligence is a multi-dimensional (quantitative, qualitative) concept. All dimensions of AI unfold and grow along their own different path with their own dynamics. Therefore, over time an increasing number of specific (narrow) AI capacities may gradually match, overtake and transcend human cognitive capacities. Given the enormous advantages of AI, for example in the field of data availability and data processing capacities, the realization of AGI probably would at the same time outclass human intelligence in many ways. Which implies that the hypothetical point of time of matching human- and artificial cognitive capacities, i.e. human-level AGI, will probably be hard to define in a meaningful way ( Goertzel, 2007 ). 3
So when AI will truly understand us as a “friend,” “partner,” “alter ego” or “buddy,” as we do when we collaborate with other humans as humans, it will surpass us in many areas at the same Moravec (1998) time. It will have a completely different profile of capacities and abilities and thus it will not be easy to really understand the way it “thinks” and comes to its decisions. In the meantime, however, as the capacities of robots expand and move from simple tools to more integrated systems, it is important to calibrate our expectations and perceptions toward robots appropriately. So, we will have to enhance our awareness and insight concerning the continuous development and progression of multiple forms of (integrated) AI systems. This concerns for example the multi-facetted nature of intelligence. Different kind of agents may have different combinations of intelligences of very different levels. An agent with general intelligence may for example be endowed with excellent abilities on the area of image recognition and navigation, calculation, and logical reasoning while at the same time being dull on the area of social interaction and goal-oriented problem solving. This awareness of the multi-dimensional nature of intelligence also concerns the way we have to deal with ( and capitalize on) anthropomorphism. That is the human tendency in human-robot interaction to characterize non-human artifacts that superficially look similar to us as possessing human-like traits, emotions, and intentions, (e.g., Kiesler and Hinds, 2004 ; Fink, 2012 ; Haring et al., 2018 ). Insight into these human factors issues is crucial to optimize the utility, performance and safety of human-AI systems ( Peeters et al., 2020 ).
From this perspective, the question whether or not “AGI at the human level” will be realized is not the most relevant question for the time being. According to most AI scientists, this will certainly happen, and the key question is not IF this will happen, but WHEN, (e.g., Müller and Bostrom, 2016 ). At a system level, however, multiple narrow AI applications are likely to overtake human intelligence in an increasingly wide range of areas.
Conclusions and Framework
The present paper focused on providing some more clarity and insight into the fundamental characteristics, differences and idiosyncrasies of human and artificial intelligences. First we presented ideas and arguments to scale up and differentiate our conception of intelligence, whether this may be human or artificial. Central to this broader, multi-faceted, conception of intelligence is the notion that intelligence in itself is a matter of information and computation, independent of its physical substrate. However, the nature of this physical substrate (biological/carbon or digital/silicon), will substantially determine its potential envelope of cognitive abilities and limitations. Organic cognitive faculties of humans have been very recently developed during the evolution of mankind. These “embryonal” faculties have been built on top of a biological neural network apparatus that has been optimized for allostasis and (complex) perceptual motor functions. Human cognition is therefore characterized by various structural limitations and distortions in its capacity to process certain forms of non-biological information. Biological neural networks are, for example, not very capable of performing arithmetic calculations, for which my pocket calculator fits millions of times better. These inherent and ingrained limitations, that are due to the biological and evolutionary origin of human intelligence, may be termed “hard-wired.”
In line with the Moravic’s paradox , we argued that intelligent behavior is more than what we, as homo sapiens, find difficult. So we should not confuse task-difficulty (subjective, anthropocentric) with task-complexity (objective). Instead we advocated a versatile conceptualization of intelligence and an acknowledgment of its many possible forms and compositions. This implies a high variety in types of biological or other forms of high (general) intelligence with a broad range of possible intelligence profiles and cognitive qualities (which may or may not surpass ours in many ways). This would make us better aware of the most probable potentials of AI applications for the short- and medium-term future. For example, from this perspective, our primary research focus should be on those components of the intelligence spectrum that are relatively difficult for the human brain and relatively easy for machines. This involves primarily the cognitive component requiring calculation, arithmetic analysis, statistics, probability calculation, data analysis, logical reasoning, memorization, et cetera.
In line with this we have advocated a modest, more humble, view of our human, general intelligence. Which also implies that human-level AGI should not be considered as the “golden standard” of intelligence (to be pursued with foremost priority). Because of the many fundamental differences between natural and artificial intelligences, human-like AGI will be very difficult to accomplish in the first place (and also with relatively limited added value). In case an AGI will be accomplished in the (far) future it will therefore probably have a completely different profile of cognitive capacities and abilities than we, as humans, have. When such an AGI has come so far that it is able to “collaborate” like a human, it will at the same time be likely that can in many respects already function at highly superior levels relative to what we are able to. For the time being, however, it will not be very realistic and useful to aim at AGI that includes the broad scope of human perceptual-motor and cognitive abilities. Instead, the most profitable AI applications for the short- and mid-term future, will probably be based on multiple narrow AI systems. These multiple narrow AI applications may catch up with human intelligence in an increasingly broader range of areas.
From this point of view we advocate not to dwell too intensively on the AGI question, whether or when AI will outsmart us, take our jobs, or how to endow it with all kinds of human abilities. Given the present state of the art it may be wise to focus more on the whole system of multiple AI innovations with humans as a crucial connecting and supervising factor. This also implies the establishment and formalization of legal boundaries and proper (effective, ethical, safe) goals for AI systems ( Elands et al., 2019 ; Aliman, 2020 ). So this human factor (legislator, user, “collaborator”) needs to have good insight into the characteristics and capacities of biological and artificial intelligence (under all sorts of tasks and working conditions). Both in the workplace and in policy making the most fruitful AI applications will be to complement and compensate for the inherent biological and cognitive constraints of humans. For this reason, prominent issues concern how to use it intelligently? For what tasks and under what conditions decisions are safe to leave to AI and when is human judgment required? How can we capitalize on the strengths of human intelligence and how to deploy AI systems effectively to complement and compensate for the inherent constraints of human cognition. See ( Hoffman and Johnson, 2019 ; Shneiderman, 2020a ; Shneiderman, 2020b ) for recent overviews.
In summary: No matter how intelligent autonomous AI agents become in certain respects, at least for the foreseeable future, they will remain unconscious machines. These machines have a fundamentally different operating system (biological vs digital) with correspondingly different cognitive abilities and qualities than people and other animals. So, before a proper “team collaboration” can start, the human team members will have to understand these kinds of differences, i.e., how human information processing and intelligence differs from that of–the many possible and specific variants of—AI systems. Only when humans develop a proper of these “interspecies” differences they can effectively capitalize on the potential benefits of AI in (future) human-AI teams. Given the high flexibility, versatility, and adaptability of humans relative to AI systems, the first challenge becomes then how to ensure human adaptation to the more rigid abilities of AI? 4 In other words: how can we achieve a proper conception the differences between human- and artificial intelligence?
Framework for Intelligence Awareness Training
For this question, the issue of Intelligence Awareness in human professionals needs to be addressed more vigorously. Next to computer tools for the distribution of relevant awareness information ( Collazos et al., 2019 ) in human-machine systems, this requires better education and training on how to deal with the very new and different characteristics, idiosyncrasies, and capacities of AI systems. This includes, for example, a proper understanding of the basic characteristics, possibilities, and limitations of the AI’s cognitive system properties without anthropocentric and/or anthropomorphic misconceptions. In general, this “Intelligence Awareness” is highly relevant in order to better understand, investigate, and deal with the manifold possibilities and challenges of machine intelligence. This practical human-factors challenge could, for instance, be tackled by developing new, targeted and easily configurable (adaptive) training forms and learning environments for human-AI systems. These flexible training forms and environments, (e.g. simulations and games) should focus at developing knowledge, insight and practical skills concerning the specific, non-human characteristics, abilities, and limitations of AI systems and how to deal with these in practical situations. People will have to understand the critical factors determining the goals, performance, and choices of AI? Which may in some cases even include the simple notion that AIs excite as much about their performance in achieving their goals as your refrigerator does for keeping your milkshake well. They have to learn when and under what conditions decisions are safe to leave to AI and when is human judgment required or essential? And more in general: how does it “think” and decide? The relevance of this kind of knowledge, skills and practices will only become bigger when the degree of autonomy (and genericity) of advanced AI systems will grow.
What does such an Intelligence Awareness training curriculum look like? It needs to include at least a module on the cognitive characteristics of AI. This is basically a subject similar to those subjects that are also included in curricula on human cognition. This broad module on the “Cognitive Science of AI” may involve a range of sub-topics starting with a revision of the concept of "Intelligence" stripped of anthropocentric and anthropomorphic misunderstandings. In addition, this module should focus at providing knowledge about the structure and operation of the AI operating system or the “AI mind.” This may be followed by subjects like: Perception and interpretation of information by AI, AI cognition (memory, information processing, problem solving, biases), dealing with AI possibilities and limitations in the “human” areas like creativity, adaptivity, autonomy, reflection, and (self-) awareness, dealing with goal functions (valuation of actions in relation to cost-benefit), AI ethics and AI security. In addition, such a curriculum should include technical modules providing insight into the working of the AI operating system. Due to the enormous speed with which the AI technology and application develops, the content of such a curriculum is also very dynamic and continuously evolving on the basis of technological progress. This implies that the curriculum and training-aids and -environments should be flexible, experiential, and adaptive, which makes the work form of serious gaming ideally suited. Below, we provide a global framework for the development of new educational curricula on AI awareness. These subtopics go beyond learning to effectively “operate,” “control” or interact with specific AI applications (i.e. conventional human-machine interaction):
‐Understanding of underlying system characteristics of the AI (the “AI brain”). Understanding the specific qualities and limitations of AI relative to human intelligence.
‐Understanding the complexity of the tasks and of the environment from the perspective of AI systems.
‐Understanding the problem of biases in human cognition, relative to biases in AI.
‐Understanding the problems associated with the control of AI, predictability of AI behavior (decisions), building trust, maintaining situation awareness (complacency), dynamic task allocation, (e.g. taking over each other’s tasks) and responsibility (accountability).
‐How to deal with possibilities and limitations of AI in the field of “creativity”, adaptability of AI, “environmental awareness”, and generalization of knowledge.
‐Learning to deal with perceptual and cognitive limitations and possible errors of AI which may be difficult to comprehend.
‐Trust in the performance of AI (possibly in spite of limited transparency or ability to “explain”) based on verification and validation.
‐Learning to deal with our natural inclination to anthropocentrism and anthropomorphism (“theory of mind”) when reasoning about human-robot interaction.
‐How to capitalize on the powers of AI in order to deal with the inherent constraints of human information processing (and vice versa).
‐Understanding the specific characteristics and qualities of the man-machine system and being able to decide on when, for what, and how the integrated combination of human- and AI faculties may perform at best overall system potential.
In conclusion: due to the enormous speed with which the AI technology and application evolves we need a more versatile conceptualization of intelligence and an acknowledgment of its many possible forms and combinations. A revised conception of intelligence includes also a good understanding of the basic characteristics, possibilities, and limitations of different (biological, artificial) cognitive system properties without anthropocentric and/or anthropomorphic misconceptions. This “Intelligence Awareness” is highly relevant in order to better understand and deal with the manifold possibilities and challenges of machine intelligence, for instance to decide when to use or deploy AI in relation to tasks and their context. The development of educational curricula with new, targeted, and easily configurable training forms and learning environments for human-AI systems are therefore recommended. Further work should focus on training tools, methods and content that are flexible and adaptive enough to be able to keep up with the rapid changes in the field of AI and with the wide variety of target groups and learning goals.
Author Contributions
The literature search, analysis, conceptual work, and the writing of the manuscript was done by JEK. All authors listed have made substantial, direct, and intellectual contribution to the work and approved it for publication.
Conflict of Interest
The authors declare that the research was conducted in the absence of any commercial or financial relationships that could be construed as a potential conflict of interest.
Acknowledgments
The authors want to thank J. van Diggelen, L.J.H.M. Kester for their useful inputs for this manuscript. The present paper was a deliverable of 1) the BIHUNT program (Behavioral Impact of NIC Teaming, V1719) funded by the Dutch Ministry of Defense and of the Wise Policy Making program funded by the Netherlands Organization for Applied Scientific Research (TNO).
1 Narrow AI can be defined as the production of systems displaying intelligence regarding specific, highly constrained tasks, like playing chess, facial recognition, autonomous navigation, or locomotion ( Goertzel et al., 2014 ).
2 Cognitive abilities involve deliberate, conceptual or analytic thinking (e.g., calculation, statistics, analysis, reasoning, abstraction)
3 Unless of course AI will be deliberately constrained or degraded to human-level functioning.
4 Next to the issue of Human-Aware AI, i.e. tuning AI to the cognitive characteristics of humans.
Ackermann, N. (2018). Artificial Intelligence Framework: a visual introduction to machine learning and AI Retrieved from: https://towardsdatascience.com/artificial-intelligence-framework-a-visual-introduction-to-machine-learning-and-ai-d7e36b304f87 . (September 9, 2019).
Aliman, N-M. (2020). Hybrid cognitive-affective Strategies for AI safety . PhD thesis . Utrecht, Netherlands: Utrecht University . doi:10.33540/203
CrossRef Full Text
Bao, J. X., Kandel, E. R., and Hawkins, R. D. (1997). Involvement of pre- and postsynaptic mechanisms in posttetanic potentiation at Aplysia synapses. Science 275, 969–973. doi:10.1126/science.275.5302.969Dane
PubMed Abstract | CrossRef Full Text | Google Scholar
Bar, M. (2007). The proactive brain: using analogies and associations to generate predictions. Trends Cogn. Sci. 11, 280–289. doi:10.1016/j.tics.2007.05.005
Baron, J., and Ritov, I. (2004). Omission bias, individual differences, and normality. Organizational Behav. Hum. Decis. Process. 94, 74–85. doi:10.1016/j.obhdp.2004.03.003
CrossRef Full Text | Google Scholar
Belkom, R. v. (2019). Duikboten zwemmen niet: de zoektocht naar intelligente machines. Den Haag: Stichting Toekomstbeeld der Techniek (STT) .
Google Scholar
Bergstein, B. (2017). AI isn’t very smart yet. But we need to get moving to make sure automation works for more people . Cambridge, MA, United States: MIT Technology Retrieved from: https://www.technologyreview.com/s/609318/the-great-ai-paradox/
Bieger, J. B., Thorisson, K. R., and Garrett, D. (2014). “Raising AI: tutoring matters,” in 7th international conference, AGI 2014 quebec city, QC, Canada, august 1–4, 2014 proceedings . Editors B. Goertzel, L. Orseau, and J. Snaider (Berlin, Germany: Springer ). doi:10.1007/978-3-319-09274-4
Boden, M. (2017). Principles of robotics: regulating robots in the real world. Connect. Sci. 29 (2), 124–129.
Bostrom, N. (2014). Superintelligence: pathts, dangers, strategies . Oxford United Kingdom: Oxford University Press .
Bradshaw, J. M., Dignum, V., Jonker, C. M., and Sierhuis, M. (2012). Introduction to special issue on human-agent-robot teamwork. IEEE Intell. Syst. 27, 8–13. doi:10.1109/MIS.2012.37
Brodal, A. (1981). Neurological anatomy in relation to clinical medicine . New York, NY, United States: Oxford University Press .
Brown, T. B. (2020). Language models are few-shot learners, arXiv 2005, 14165v4.
Cialdini, R. D. (1984). Influence: the psychology of persuation . New York, NY, United States: Harper .
Coley, J. D., and Tanner, K. D. (2012). Common origins of diverse misconceptions: cognitive principles and the development of biology thinking. CBE Life Sci. Educ. 11 (3), 209–215. doi:10.1187/cbe.12-06-0074
Collazos, C. A., Gutierrez, F. L., Gallardo, J., Ortega, M., Fardoun, H. M., and Molina, A. I. (2019). Descriptive theory of awareness for groupware development. J. Ambient Intelligence Humanized Comput. 10, 4789–4818. doi:10.1007/s12652-018-1165-9
Damasio, A. R. (1994). Descartes’ error: emotion, reason and the human brain . New York, NY, United States: G. P. Putnam’s Sons .
Elands, P., HuizingKester, A. L., Oggero, S., and Peeters, M. (2019). Governing ethical and effective behavior of intelligent systems: a novel framework for meaningful human control in a military context. Militaire Spectator 188 (6), 302–313.
Feldman-Barret, L. (2017). How emotions are made: the secret life of the brain . Boston, MA, United States: Houghton Mifflin Harcourt .
Fink, J. (2012). “Anthropomorphism and human likeness in the design of robots and human-robot interaction,” in Social robotics. ICSR 2012 . Lecture notes in computer science . Editors S. S. Ge, O. Khatib, J. J. Cabibihan, R. Simmons, and M. A. Williams (Berlin, Germany: Springer ), 7621. doi:10.1007/978-3-642-34103-8_20
Fischetti, M. (2011). Computers vs brains. Scientific American 175 th anniversary issue Retreived from: https://www.scientificamerican.com/article/computers-vs-brains/ .
Furnham, A., and Boo, H. C. (2011). A literature review of the anchoring effect. The J. Socio-Economics 40, 35–42. doi:10.1016/j.socec.2010.10.008
Gerla, M., Lee, E-K., and Pau, G. (2014). Internet of vehicles: from intelligent grid to autonomous cars and vehicular clouds. WF-IoT 12, 241–246. doi:10.1177/1550147716665500
Gibson, J. J. (1979). The ecological approach to visual perception . Boston, MA, United States: Houghton Mifflin .
Gibson, J. J. (1966). The senses considered as perceptual systems . Boston, MA, United States: Houghton Mifflin.
Gigerenzer, G., and Gaissmaier, W. (2011). Heuristic decision making. Annu. Rev. Psychol. 62, 451–482. doi:10.1146/annurev-psych-120709-145346
Goertzel, B. (2007). Human-level artificial general intelligence and the possibility of a technological singularity: a reaction to Ray Kurzweil's the singularity is near, and McDermott’s critique of Kurzweil. Artif. Intelligence 171 (18), 1161–1173. doi:10.1016/j.artint.2007.10.011
Goertzel, B., Orseau, L., and Snaider, J., (Editors). (2014). Preface. 7th international conference, AGI 2014 Quebec City, QC, Canada, August 1–4, 2014 Proceedings Springer .
Grind, W. A. van. de. (1997). Natuurlijke intelligentie: over denken, intelligentie en bewustzijn van mensen en andere dieren . 2nd edn. Amsterdam, Netherlands: Nieuwezijds Retrieved from https://www.nieuwezijds.nl/boek/natuurlijke-intelligentie/ . (July 9, 2019).
Hardin, G. (1968). The tragedy of the commons. The population problem has no technical solution; it requires a fundamental extension in morality. Science 162, 1243–1248. doi:10.1126/science.162.3859.1243
Haring, K. S., Watanabe, K., Velonaki, M., Tosell, C. C., and Finomore, V. (2018). Ffab—the form function attribution bias in human-robot interaction. IEEE Trans. Cogn. Dev. Syst. 10 (4), 843–851. doi:10.1109/TCDS.2018.2851569
Haselton, M. G., Bryant, G. A., Wilke, A., Frederick, D. A., Galperin, A., Frankenhuis, W. E., et al. (2009). Adaptive rationality: an evolutionary perspective on cognitive bias. Soc. Cogn. 27, 733–762. doi:10.1521/soco.2009.27.5.733
Haselton, M. G., Nettle, D., and Andrews, P. W. (2005). “The evolution of cognitive bias,” in The handbook of evolutionary psychology . Editor D.M. Buss (Hoboken, NJ, United States: John Wiley & Sons ), 724–746.
Henshilwood, C., and Marean, C. (2003). The origin of modern human behavior. Curr. Anthropol. 44 (5), 627–651. doi:10.1086/377665
Hoffman, R. R., and Johnson, M. (2019). “The quest for alternatives to “levels of automation” and “task allocation,” in Human performance in automated and autonomous systems . Editors M. Mouloua, and P. A. Hancock (Boca Raton, FL, United States: CRC Press ), 43–68.
Hoffrage, U., Hertwig, R., and Gigerenzer, G. (2000). Hindsight bias: a by-product of knowledge updating? J. Exp. Psychol. Learn. Mem. Cogn. 26, 566–581. doi:10.1037/0278-7393.26.3.566
Horowitz, M. C. (2018). The promise and peril of military applications of artificial intelligence. Bulletin of the atomic scientists Retrieved from https://thebulletin.org/militaryapplications-artificial-intelligence/promise-and-peril-military-applications-artificial-intelligence (Accessed March 27, 2019).
Isaacson, J. S., and Scanziani, M. (2011). How inhibition shapes cortical activity. Neuron 72, 231–243. doi:10.1016/j.neuron.2011.09.027
Johnson, M., Bradshaw, J. M., Feltovich, P. J., Jonker, C. M., van Riemsdijk, M. B., and Sierhuis, M. (2014). Coactive design: designing support for interdependence in joint activity. J. Human-Robot Interaction 3 (1), 43–69. doi:10.5898/JHRI.3.1.Johnson
Kahle, W. (1979). Band 3: nervensysteme und Sinnesorgane , in Taschenatlas de anatomie. Stutttgart . Editors W. Kahle, H. Leonhardt, and W. Platzer (New York, NY, United States: Thieme Verlag ).
Kahneman, D., and Klein, G. (2009). Conditions for intuitive expertize: a failure to disagree. Am. Psychol. 64, 515–526. doi:10.1037/a0016755
Kahneman, D. (2011). Thinking, fast and slow . New York, NY, United States: Farrar, Straus and Giroux .
Katz, B., and Miledi, R. (1968). The role of calcium in neuromuscular facilitation. J. Physiol. 195, 481–492. doi:10.1113/jphysiol.1968.sp008469
Kiesler, S., and Hinds, P. (2004). Introduction to this special issue on human–robot interaction. Int J Hum-Comput. Int. 19 (1), 1–8. doi:10.1080/07370024.2004.9667337
Klein, G., Woods, D. D., Bradshaw, J. M., Hoffman, R. R., and Feltovich, P. J. (2004). Ten challenges for making automation a ‘team player’ in joint human-agent activity. IEEE Intell. Syst. 19 (6), 91–95. doi:10.1109/MIS.2004.74
Korteling, J. E. (1994). Multiple-task performance and aging . Bariet, Ruinen, Netherlands: Dissertation. TNO-Human Factors Research Institute/State University Groningen https://www.researchgate.net/publication/310626711_Multiple-Task_Performance_and_Aging .
Korteling, J. E., and Toet, A. (2020). Cognitive biases. in Encyclopedia of behavioral neuroscience . 2nd Edn (Amsterdam-Edinburgh: Elsevier Science ) doi:10.1016/B978-0-12-809324-5.24105-9
Korteling, J. E., Brouwer, A. M., and Toet, A. (2018a). A neural network framework for cognitive bias. Front. Psychol. 9, 1561. doi:10.3389/fpsyg.2018.01561
Korteling, J. E., van de Boer-Visschedijk, G. C., Boswinkel, R. A., and Boonekamp, R. C. (2018b). Effecten van de inzet van Non-Human Intelligent Collaborators op Opleiding and Training [V1719]. Report TNO 2018 R11654. Soesterberg: TNO defense safety and security , Soesterberg, Netherlands: TNO, Soesterberg .
Korteling, J. E., Gerritsma, J., and Toet, A. (2021). Retention and transfer of cognitive bias mitigation interventions: a systematic literature study. Front. Psychol. 1–20. doi:10.13140/RG.2.2.27981.56800
Kosslyn, S. M., and Koenig, O. (1992). Wet Mind: the new cognitive neuroscience . New York, NY, United States: Free Press .
Krämer, N. C., von der Pütten, A., and Eimler, S. (2012). “Human-agent and human-robot interaction theory: similarities to and differences from human-human interaction,” in Human-computer interaction: the agency perspective . Studies in computational intelligence . Editors M. Zacarias, and J. V. de Oliveira (Berlin, Germany: Springer ), 396, 215–240. doi:10.1007/978-3-642-25691-2_9
Kurzweil, R. (2005). The singularity is near . New York, NY, United States: Viking press .
Kurzweil, R. (1990). The age of intelligent machines . Cambridge, MA, United States: MIT Press .
Lake, B. M., Ullman, T. D., Tenenbaum, J. B., and Gershman, S. J. (2017). Building machines that learn and think like people. Behav. Brain Sci. 40, e253. doi:10.1017/S0140525X16001837
Lichtenstein, S., and Slovic, P. (1971). Reversals of preference between bids and choices in gambling decisions. J. Exp. Psychol. 89, 46–55. doi:10.1037/h0031207
McBrearty, S., and Brooks, A. (2000). The revolution that wasn't: a new interpretation of the origin of modern human behavior. J. Hum. Evol. 39 (5), 453–563. doi:10.1006/jhev.2000.0435
McClelland, J. L. (1978). Perception and masking of wholes and parts. J. Exp. Psychol. Hum. Percept Perform. 4, 210–223. doi:10.1037//0096-1523.4.2.210
McDowd, J. M., and Craik, F. I. M. (1988). Effects of aging and task difficulty on divided attention performance. J. Exp. Psychol. Hum. Percept. Perform . 14, 267–280.
Minsky, M. (1986). The Society of Mind . London, United Kingdom: Simon and Schuster .
Moravec, H. (1988). Mind children . Cambridge, MA, United States: Harvard University Press .
Moravec, H. (1998). When will computer hardware match the human brain? J. Evol. Tech. 1Retreived from https://jetpress.org/volume1/moravec.htm .
Müller, V. C., and Bostrom, N. (2016). Future progress in artificial intelligence: a survey of expert opinion. Fundamental issues of artificial intelligence . Cham, Switzerland: Springer . doi:10.1007/978-3-319-26485-1
Nickerson, R. S. (1998). Confirmation bias: a ubiquitous phenomenon in many guises. Rev. Gen. Psychol. 2, 175–220. doi:10.1037/1089-2680.2.2.175
Nosek, B. A., Hawkins, C. B., and Frazier, R. S. (2011). Implicit social cognition: from measures to mechanisms. Trends Cogn. Sci. 15 (4), 152–159. doi:10.1016/j.tics.2011.01.005
Patt, A., and Zeckhauser, R. (2000). Action bias and environmental decisions. J. Risk Uncertain. 21, 45–72. doi:10.1023/a:1026517309871
Peeters, M. M., van Diggelen, J., van Den Bosch, K., Bronkhorst, A., Neerincx, M. A., Schraagen, J. M., et al. (2020). Hybrid collective intelligence in a human–AI society. AI and Society 38, 217–(238.) doi:10.1007/s00146-020-01005-y
Petraglia, M. D., and Korisettar, R. (1998). Early human behavior in global context . Oxfordshire, United Kingdom: Routledge .
Pomerantz, J. (1981). “Perceptual organization in information processing,” in Perceptual organization . Editors M. Kubovy, and J. Pomerantz (Hillsdale, NJ, United States: Lawrence Erlbaum ).
Pronin, E., Lin, D. Y., and Ross, L. (2002). The bias blind spot: perceptions of bias in self versus others. Personal. Soc. Psychol. Bull. 28, 369–381. doi:10.1177/0146167202286008
Reicher, G. M. (1969). Perceptual recognition as a function of meaningfulness of stimulus material. J. Exp. Psychol. 81, 274–280.
Rich, E., and Knight, K. (1991). Artificial intelligence . 2nd edition. New York, NY, United States: McGraw-Hill .
Rich, E., Knight, K., and Nair, S. B. (2009). Articial intelligence . 3rd Edn. New Delhi, India: Tata McGraw-Hill .
Risen, J. L. (2015). Believing what we do not believe: acquiescence to superstitious beliefs and other powerful intuitions. Psychol. Rev. 123, 182–207. doi:10.1037/rev0000017
Roese, N. J., and Vohs, K. D. (2012). Hindsight bias. Perspect. Psychol. Sci. 7, 411–426. doi:10.1177/1745691612454303
Rogers, R. D., and Monsell, S. (1995). Costs of a predictible switch between simple cognitive tasks. J. Exp. Psychol. Gen. 124, 207e231. doi:10.1037/0096-3445.124.2.207
Rubinstein, J. S., Meyer, D. E., and Evans, J. E. (2001). Executive control of cognitive processes in task switching. J. Exp. Psychol. Hum. Percept Perform. 27, 763–797. doi:10.1037//0096-1523.27.4.763
Russell, S., and Norvig, P. (2014). Artificial intelligence: a modern approach . 3rd ed. Harlow, United Kingdom: Pearson Education .
Shafir, E., and LeBoeuf, R. A. (2002). Rationality. Annu. Rev. Psychol. 53, 491–517. doi:10.1146/annurev.psych.53.100901.135213
Shatz, C. J. (1992). The developing brain. Sci. Am. 267, 60–67. doi:10.1038/scientificamerican0992-60
Shneiderman, B. (2020a). Design lessons from AI’s two grand goals: human emulation and useful applications. IEEE Trans. Tech. Soc. 1, 73–82. doi:10.1109/TTS.2020.2992669
Shneiderman, B. (2020b). Human-centered artificial intelligence: reliable, safe & trustworthy. Int. J. Human–Computer Interaction 36 (6), 495–504. doi:10.1080/10447318.2020.1741118
Siegel, A., and Sapru, H. N. (2005). Essential neuroscience . Philedelphia, PA, United States: Lippincott Williams and Wilkins .
Silver, D., Schrittwieser, J., Simonyan, K., Antonoglou, I., Huang, A., Guez, A., et al. (2017). Mastering the game of go without human knowledge. Nature 550 (7676), 354. doi:10.1038/nature24270
Simon, H. A. (1955). A behavioral model of rational choice. Q. J. Econ. 69, 99–118. doi:10.2307/1884852
Taylor, D. M., and Doria, J. R. (1981). Self-serving and group-serving bias in attribution. J. Soc. Psychol. 113, 201–211. doi:10.1080/00224545.1981.9924371
Tegmark, M. (2017). Life 3.0: being human in the age of artificial intelligence . New York, NY, United States: Borzoi Book published by A.A. Knopf .
Toet, A., Brouwer, A. M., van den Bosch, K., and Korteling, J. E. (2016). Effects of personal characteristics on susceptibility to decision bias: a literature study. Int. J. Humanities Soc. Sci. 8, 1–17.
Tooby, J., and Cosmides, L. (2005). “Conceptual foundations of evolutionary psychology,” in Handbook of evolutionary psychology . Editor D.M. Buss (Hoboken, NJ, United States: John Wiley & Sons ), 5–67.
Tversky, A., and Kahneman, D. (1974). Judgment under uncertainty: heuristics and biases. Science 185 (4157), 1124–1131. doi:10.1126/science.185.4157.1124
Tversky, A., and Kahneman, D. (1981). The framing of decisions and the psychology of choice. Science 211, 453–458. doi:10.1126/science.7455683
Tversky, A., and Kahneman, D. (1973). Availability: a heuristic for judging frequency and probability. Cogn. Psychol. 5, 207–232. doi:10.1016/0010-0285(73)90033-9
van den Bosch, K., and Bronkhorst, K. (2018). Human-AI cooperation to benefit military decision making. Soesterberg, Netherlands: TNO.
van den Bosch, K., and Bronkhorst, K. (2019). Six challenges for human-AI Co-learning. Adaptive instructional systems 11597, 572–589. doi:10.1007/978-3-030-22341-0_45
Weisstein, N., and Harris, C. S. (1974). Visual detection of line segments: an object-superiority effect. Science 186, 752–755. doi:10.1126/science.186.4165.752
Werkhoven, P., Neerincx, M., and Kester, L. (2018). Telling autonomous systems what to do. Proceedings of the 36th European Conference on Cognitive Ergonomics, ECCE 2018 , Utrecht, Nehterlands , 5–7 September, 2018 , 1–8. doi:10.1145/3232078.3232238
Wheeler, D., (1970). Processes in word recognition Cogn. Psychol. 1, 59–85.
Williams, A., and Weisstein, N. (1978). Line segments are perceived better in a coherent context than alone: an object-line effect in visual perception. Mem. Cognit 6, 85–90. doi:10.3758/bf03197432
Wingfield, A., and Byrnes, D. (1981). The psychology of human memory . New York, NY, united States: Academic Press .
Wood, R. E., Mento, A. J., and Locke, E. A. (1987). Task complexity as a moderator of goal effects: a meta-analysis. J. Appl. Psychol. 72 (3), 416–425. doi:10.1037/0021-9010.72.3.416
Wyrobek, K. A., Berger, E. H., van der Loos, H. F. M., and Salisbury, J. K. (2008). Toward a personal robotics development platform: rationale and design of an intrinsically safe personal robot. Proceedinds of 2008 IEEE International Conference on Robotics and Automation , Pasadena, CA, United States , 19-23 May 2008 . doi:10.1109/ROBOT.2008.4543527
Keywords: human intelligence, artificial intelligence, artificial general intelligence, human-level artificial intelligence, cognitive complexity, narrow artificial intelligence, human-AI collaboration, cognitive bias
Citation: Korteling JE(, van de Boer-Visschedijk GC, Blankendaal RAM, Boonekamp RC and Eikelboom AR (2021) Human- versus Artificial Intelligence. Front. Artif. Intell. 4:622364. doi: 10.3389/frai.2021.622364
Received: 29 October 2020; Accepted: 01 February 2021; Published: 25 March 2021.
Reviewed by:
Copyright © 2021 Korteling, van de Boer-Visschedijk, Blankendaal, Boonekamp and Eikelboom. This is an open-access article distributed under the terms of the Creative Commons Attribution License (CC BY). The use, distribution or reproduction in other forums is permitted, provided the original author(s) and the copyright owner(s) are credited and that the original publication in this journal is cited, in accordance with accepted academic practice. No use, distribution or reproduction is permitted which does not comply with these terms.
*Correspondence: J. E. (Hans). Korteling, [email protected]
This article is part of the Research Topic
Skills-in-Demand: Bridging the Gap between Educational Attainment and Labor Market with Learning Analytics and Machine Learning Applications
Table of Contents
What is artificial intelligence, what is human intelligence, artificial intelligence vs. human intelligence: a comparison, what brian cells can be tweaked to learn faster, artificial intelligence vs. human intelligence: what will the future of human vs ai be, impact of ai on the future of jobs, will ai replace humans, upskilling: the way forward, learn more about ai with simplilearn, artificial intelligence vs. human intelligence.
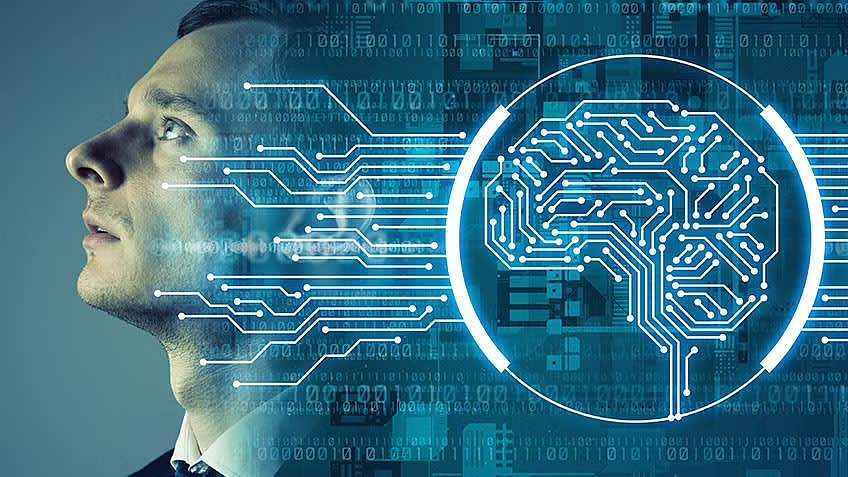
From the realm of science fiction into the realm of everyday life, artificial intelligence has made significant strides. Because AI has become so pervasive in today's industries and people's daily lives, a new debate has emerged, pitting the two competing paradigms of AI and human intelligence.
While the goal of artificial intelligence is to build and create intelligent systems that are capable of doing jobs that are analogous to those performed by humans, we can't help but question if AI is adequate on its own. This article covers a wide range of subjects, including the potential impact of AI on the future of work and the economy, how AI differs from human intelligence, and the ethical considerations that must be taken into account.
The term artificial intelligence may be used for any computer that has characteristics similar to the human brain, including the ability to think critically, make decisions, and increase productivity. The foundation of AI is human insights that may be determined in such a manner that machines can easily realize the jobs, from the most simple to the most complicated.
Insights that are synthesized are the result of intellectual activity, including study, analysis, logic, and observation. Tasks, including robotics, control mechanisms, computer vision, scheduling, and data mining , fall under the umbrella of artificial intelligence.
The origins of human intelligence and conduct may be traced back to the individual's unique combination of genetics, upbringing, and exposure to various situations and environments. And it hinges entirely on one's freedom to shape his or her environment via the application of newly acquired information.
The information it provides is varied. For example, it may provide information on a person with a similar skill set or background, or it may reveal diplomatic information that a locator or spy was tasked with obtaining. After everything is said and done, it is able to deliver information about interpersonal relationships and the arrangement of interests.
The following is a table that compares human intelligence vs artificial intelligence:
According to the findings of recent research, altering the electrical characteristics of certain cells in simulations of neural circuits caused the networks to acquire new information more quickly than in simulations with cells that were identical. They also discovered that in order for the networks to achieve the same outcomes, a smaller number of the modified cells were necessary and that the approach consumed fewer resources than models that utilized identical cells.
These results not only shed light on how human brains excel at learning but may also help us develop more advanced artificial intelligence systems, such as speech and facial recognition software for digital assistants and autonomous vehicle navigation systems.
Become a AI & Machine Learning Professional
- $267 billion Expected Global AI Market Value By 2027
- 37.3% Projected CAGR Of The Global AI Market From 2023-2030
- $15.7 trillion Expected Total Contribution Of AI To The Global Economy By 2030
Artificial Intelligence Engineer
- Add the IBM Advantage to your Learning
- Generative AI Edge
Post Graduate Program in AI and Machine Learning
- Program completion certificate from Purdue University and Simplilearn
- Gain exposure to ChatGPT, OpenAI, Dall-E, Midjourney & other prominent tools
Here's what learners are saying regarding our programs:
Indrakala Nigam Beniwal
Technical consultant , land transport authority (lta) singapore.
I completed a Master's Program in Artificial Intelligence Engineer with flying colors from Simplilearn. Thanks to the course teachers and others associated with designing such a wonderful learning experience.
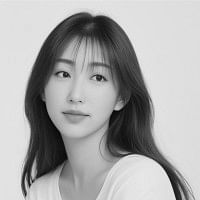
Personal Financial Consultant , OCBC Bank
The live sessions were quite good; you could ask questions and clear doubts. Also, the self-paced videos can be played conveniently, and any course part can be revisited. The hands-on projects were also perfect for practice; we could use the knowledge we acquired while doing the projects and apply it in real life.
The capabilities of AI are constantly expanding. It takes a significant amount of time to develop AI systems, which is something that cannot happen in the absence of human intervention. All forms of artificial intelligence, including self-driving vehicles and robotics, as well as more complex technologies like computer vision, and natural language processing , are dependent on human intellect.
1. Automation of Tasks
The most noticeable effect of AI has been the result of the digitalization and automation of formerly manual processes across a wide range of industries. These tasks, which were formerly performed manually, are now performed digitally. Currently, tasks or occupations that involve some degree of repetition or the use and interpretation of large amounts of data are communicated to and administered by a computer, and in certain cases, the intervention of humans is not required in order to complete these tasks or jobs.
2. New Opportunities
Artificial intelligence is creating new opportunities for the workforce by automating formerly human-intensive tasks . The rapid development of technology has resulted in the emergence of new fields of study and work, such as digital engineering. Therefore, although traditional manual labor jobs may go extinct, new opportunities and careers will emerge.
3. Economic Growth Model
When it's put to good use, rather than just for the sake of progress, AI has the potential to increase productivity and collaboration inside a company by opening up vast new avenues for growth. As a result, it may spur an increase in demand for goods and services, and power an economic growth model that spreads prosperity and raises standards of living.
4. Role of Work
In the era of AI, recognizing the potential of employment beyond just maintaining a standard of living is much more important. It conveys an understanding of the essential human need for involvement, co-creation, dedication, and a sense of being needed, and should therefore not be overlooked. So, sometimes, even mundane tasks at work become meaningful and advantageous, and if the task is eliminated or automated, it should be replaced with something that provides a comparable opportunity for human expression and disclosure.
5. Growth of Creativity and Innovation
Experts now have more time to focus on analyzing, delivering new and original solutions, and other operations that are firmly in the area of the human intellect, while robotics, AI, and industrial automation handle some of the mundane and physical duties formerly performed by humans.
While AI has the potential to automate specific tasks and jobs, it is likely to replace humans in some areas. AI is best suited for handling repetitive, data-driven tasks and making data-driven decisions. However, human skills such as creativity, critical thinking, emotional intelligence, and complex problem-solving still need to be more valuable and easily replicated by AI.
The future of AI is more likely to involve collaboration between humans and machines, where AI augments human capabilities and enables humans to focus on higher-level tasks that require human ingenuity and expertise. It is essential to view AI as a tool that can enhance productivity and facilitate new possibilities rather than as a complete substitute for human involvement.
Supercharge your career in Artificial Intelligence with our comprehensive courses. Gain the skills and knowledge to transform industries and unleash your true potential. Enroll now and unlock limitless possibilities!
Program Name AI Engineer Master's Program Post Graduate Program In Artificial Intelligence Post Graduate Program In Artificial Intelligence Geo All Geos All Geos IN/ROW University Simplilearn Purdue Caltech Course Duration 11 Months 11 Months 11 Months Coding Experience Required Basic Basic No Skills You Will Learn 10+ skills including data structure, data manipulation, NumPy, Scikit-Learn, Tableau and more. 16+ skills including chatbots, NLP, Python, Keras and more. 8+ skills including Supervised & Unsupervised Learning Deep Learning Data Visualization, and more. Additional Benefits Get access to exclusive Hackathons, Masterclasses and Ask-Me-Anything sessions by IBM Applied learning via 3 Capstone and 12 Industry-relevant Projects Purdue Alumni Association Membership Free IIMJobs Pro-Membership of 6 months Resume Building Assistance Upto 14 CEU Credits Caltech CTME Circle Membership Cost $$ $$$$ $$$$ Explore Program Explore Program Explore Program
Artificial intelligence is revolutionizing every sector and pushing humanity forward to a new level. However, it is not yet feasible to achieve a precise replica of human intellect. The human cognitive process remains a mystery to scientists and experimentalists. Because of this, the common sense assumption in the growing debate between AI and human intelligence has been that AI would supplement human efforts rather than immediately replace them. Check out the Post Graduate Program in AI and Machine Learning at Simplilearn if you are interested in pursuing a career in the field of artificial intelligence.
Our AI & Machine Learning Courses Duration And Fees
AI & Machine Learning Courses typically range from a few weeks to several months, with fees varying based on program and institution.
Get Free Certifications with free video courses
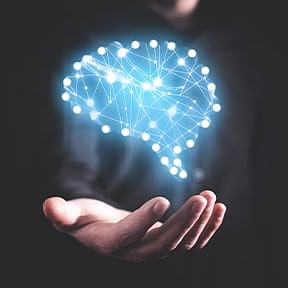
AI & Machine Learning
Machine Learning
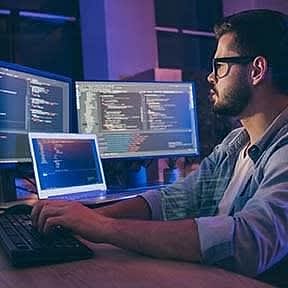
Artificial Intelligence Beginners Guide: What is AI?
Learn from Industry Experts with free Masterclasses
Gain Gen AI expertise in Purdue's Applied Gen AI Specialization
Unlock Your Career Potential: Land Your Dream Job with Gen AI Tools
Make Your Gen AI & ML Career Shift in 2024 a Success with iHUB DivyaSampark, IIT Roorkee
Recommended Reads
Artificial Intelligence Career Guide: A Comprehensive Playbook to Becoming an AI Expert
Data Science vs Artificial Intelligence: Key Differences
Top 18 Artificial Intelligence (AI) Applications in 2024
Introduction to Artificial Intelligence: A Beginner's Guide
What is Artificial Intelligence and Why Gain AI Certification
How Does AI Work
Get Affiliated Certifications with Live Class programs
- PMP, PMI, PMBOK, CAPM, PgMP, PfMP, ACP, PBA, RMP, SP, and OPM3 are registered marks of the Project Management Institute, Inc.
- Artificial Intelligence
When Might AI Outsmart Us? It Depends Who You Ask
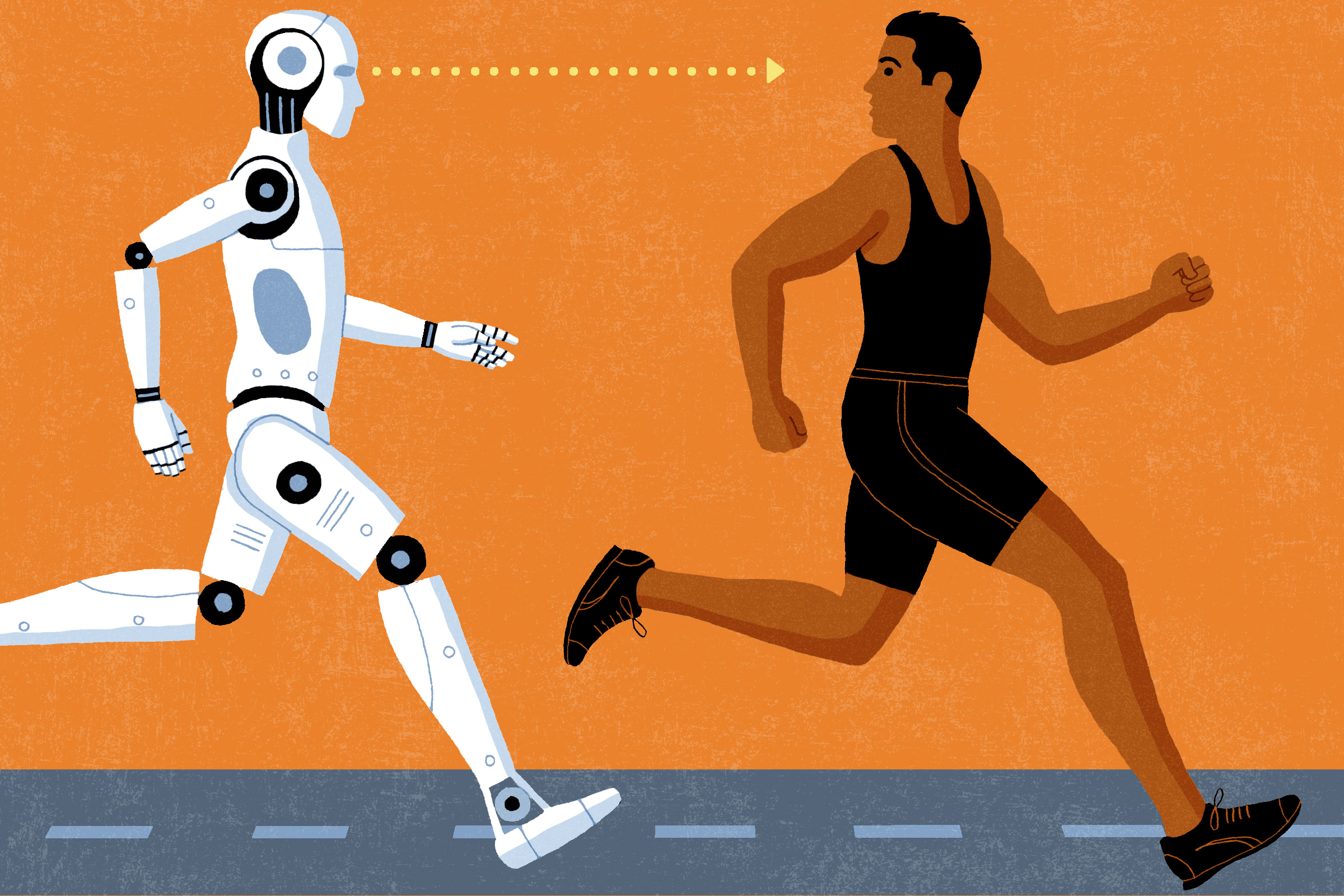
I n 1960, Herbert Simon, who went on to win both the Nobel Prize for economics and the Turing Award for computer science, wrote in his book The New Science of Management Decision that “machines will be capable, within 20 years, of doing any work that a man can do.”
History is filled with exuberant technological predictions that have failed to materialize. Within the field of artificial intelligence, the brashest predictions have concerned the arrival of systems that can perform any task a human can, often referred to as artificial general intelligence, or AGI.
So when Shane Legg, Google DeepMind’s co-founder and chief AGI scientist, estimates that there’s a 50% chance that AGI will be developed by 2028, it might be tempting to write him off as another AI pioneer who hasn’t learnt the lessons of history.
Still, AI is certainly progressing rapidly. GPT-3.5, the language model that powers OpenAI’s ChatGPT was developed in 2022, and scored 213 out of 400 on the Uniform Bar Exam, the standardized test that prospective lawyers must pass, putting it in the bottom 10% of human test-takers. GPT-4, developed just months later, scored 298, putting it in the top 10%. Many experts expect this progress to continue.
Read More: 4 Charts That Show Why AI Progress Is Unlikely to Slow Down
Legg’s views are common among the leadership of the companies currently building the most powerful AI systems. In August, Dario Amodei , co-founder and CEO of Anthropic, said he expects a “human-level” AI could be developed in two to three years. Sam Altman, CEO of OpenAI, believes AGI could be reached sometime in the next four or five years.
But in a recent survey the majority of 1,712 AI experts who responded to the question of when they thought AI would be able to accomplish every task better and more cheaply than human workers were less bullish. A separate survey of elite forecasters with exceptional track records shows they are less bullish still.
The stakes for divining who is correct are high. Legg, like many other AI pioneers, has warned that powerful future AI systems could cause human extinction. And even for those less concerned by Terminator scenarios, some warn that an AI system that could replace humans at any task might replace human labor entirely .
The scaling hypothesis
Many of those working at the companies building the biggest and most powerful AI models believe that the arrival of AGI is imminent. They subscribe to a theory known as the scaling hypothesis: the idea that even if a few incremental technical advances are required along the way, continuing to train AI models using ever greater amounts of computational power and data will inevitably lead to AGI.
There is some evidence to back this theory up. Researchers have observed very neat and predictable relationships between how much computational power, also known as “compute,” is used to train an AI model and how well it performs a given task. In the case of large language models (LLM)—the AI systems that power chatbots like ChatGPT—scaling laws predict how well a model can predict a missing word in a sentence. OpenAI CEO Sam Altman recently told TIME that he realized in 2019 that AGI might be coming much sooner than most people think, after OpenAI researchers discovered the scaling laws.
Read More: 2023 CEO of the Year: Sam Altman
Even before the scaling laws were observed, researchers have long understood that training an AI system using more compute makes it more capable. The amount of compute being used to train AI models has increased relatively predictably for the last 70 years as costs have fallen.
Early predictions based on the expected growth in compute were used by experts to anticipate when AI might match (and then possibly surpass) humans. In 1997, computer scientist Hans Moravec argued that cheaply available hardware will match the human brain in terms of computing power in the 2020s. An Nvidia A100 semiconductor chip, widely used for AI training, costs around $10,000 and can perform roughly 20 trillion FLOPS, and chips developed later this decade will have higher performance still. However, estimates for the amount of compute used by the human brain vary widely from around one trillion floating point operations per second (FLOPS) to more than one quintillion FLOPS, making it hard to evaluate Moravec’s prediction. Additionally, training modern AI systems requires a great deal more compute than running them, a fact that Moravec’s prediction did not account for.
More recently, researchers at nonprofit Epoch have made a more sophisticated compute-based model . Instead of estimating when AI models will be trained with amounts of compute similar to the human brain, the Epoch approach makes direct use of scaling laws and makes a simplifying assumption: If an AI model trained with a given amount of compute can faithfully reproduce a given portion of text—based on whether the scaling laws predict such a model can repeatedly predict the next word almost flawlessly—then it can do the work of producing that text. For example, an AI system that can perfectly reproduce a book can substitute for authors, and an AI system that can reproduce scientific papers without fault can substitute for scientists.
Some would argue that just because AI systems can produce human-like outputs, that doesn’t necessarily mean they will think like a human. After all, Russell Crowe plays Nobel Prize-winning mathematician John Nash in the 2001 film, A Beautiful Mind , but nobody would claim that the better his acting performance, the more impressive his mathematical skills must be. Researchers at Epoch argue that this analogy rests on a flawed understanding of how language models work. As they scale up, LLMs acquire the ability to reason like humans, rather than just superficially emulating human behavior. However, some researchers argue it's unclear whether current AI models are in fact reasoning.
Epoch’s approach is one way to quantitatively model the scaling hypothesis, says Tamay Besiroglu, Epoch’s associate director, who notes that researchers at Epoch tend to think AI will progress less rapidly than the model suggests. The model estimates a 10% chance of transformative AI— defined as “AI that if deployed widely, would precipitate a change comparable to the industrial revolution”—being developed by 2025, and a 50% chance of it being developed by 2033. The difference between the model’s forecast and those of people like Legg is probably largely down to transformative AI being harder to achieve than AGI, says Besiroglu.
Asking the experts
Although many in leadership positions at the most prominent AI companies believe that the current path of AI progress will soon produce AGI, they’re outliers. In an effort to more systematically assess what the experts believe about the future of artificial intelligence, AI Impacts, an AI safety project at the nonprofit Machine Intelligence Research Institute, surveyed 2,778 experts in fall 2023, all of whom had published peer-reviewed research in prestigious AI journals and conferences in the last year.
Among other things, the experts were asked when they thought “high-level machine intelligence,” defined as machines that could “accomplish every task better and more cheaply than human workers” without help, would be feasible. Although the individual predictions varied greatly, the average of the predictions suggests a 50% chance that this would happen by 2047, and a 10% chance by 2027.
Like many people, the experts seemed to have been surprised by the rapid AI progress of the last year and have updated their forecasts accordingly—when AI Impacts ran the same survey in 2022, researchers estimated a 50% chance of high-level machine intelligence arriving by 2060, and a 10% chance by 2029.
The researchers were also asked when they thought various individual tasks could be carried out by machines. They estimated a 50% chance that AI could compose a Top 40 hit by 2028 and write a book that would make the New York Times bestseller list by 2029.
The superforecasters are skeptical
Nonetheless, there is plenty of evidence to suggest that experts don’t make good forecasters. Between 1984 and 2003, social scientist Philip Tetlock collected 82,361 forecasts from 284 experts, asking them questions such as: Will Soviet leader Mikhail Gorbachev be ousted in a coup? Will Canada survive as a political union? Tetlock found that the experts’ predictions were often no better than chance, and that the more famous an expert was, the less accurate their predictions tended to be.
Next, Tetlock and his collaborators set out to determine whether anyone could make accurate predictions. In a forecasting competition launched by the U.S. Intelligence Advanced Research Projects Activity in 2010, Tetlock’s team, the Good Judgement Project (GJP), dominated the others, producing forecasts that were reportedly 30% more accurate than intelligence analysts who had access to classified information. As part of the competition, the GJP identified “superforecasters”—individuals who consistently made above-average accuracy forecasts. However, although superforecasters have been shown to be reasonably accurate for predictions with a time horizon of two years or less, it's unclear whether they’re also similarly accurate for longer-term questions such as when AGI might be developed, says Ezra Karger, an economist at the Federal Reserve Bank of Chicago and research director at Tetlock’s Forecasting Research Institute.
When do the superforecasters think AGI will arrive? As part of a forecasting tournament run between June and October 2022 by the Forecasting Research Institute, 31 superforecasters were asked when they thought Nick Bostrom—the controversial philosopher and author of the seminal AI existential risk treatise Superintelligence —would affirm the existence of AGI. The median superforecaster thought there was a 1% chance that this would happen by 2030, a 21% chance by 2050, and a 75% chance by 2100.
Who’s right?
All three approaches to predicting when AGI might be developed—Epoch’s model of the scaling hypothesis, and the expert and superforecaster surveys—have one thing in common: there’s a lot of uncertainty. In particular, the experts are spread widely, with 10% thinking it's as likely as not that AGI is developed by 2030, and 18% thinking AGI won’t be reached until after 2100.
Still, on average, the different approaches give different answers. Epoch’s model estimates a 50% chance that transformative AI arrives by 2033, the median expert estimates a 50% probability of AGI before 2048, and the superforecasters are much further out at 2070.
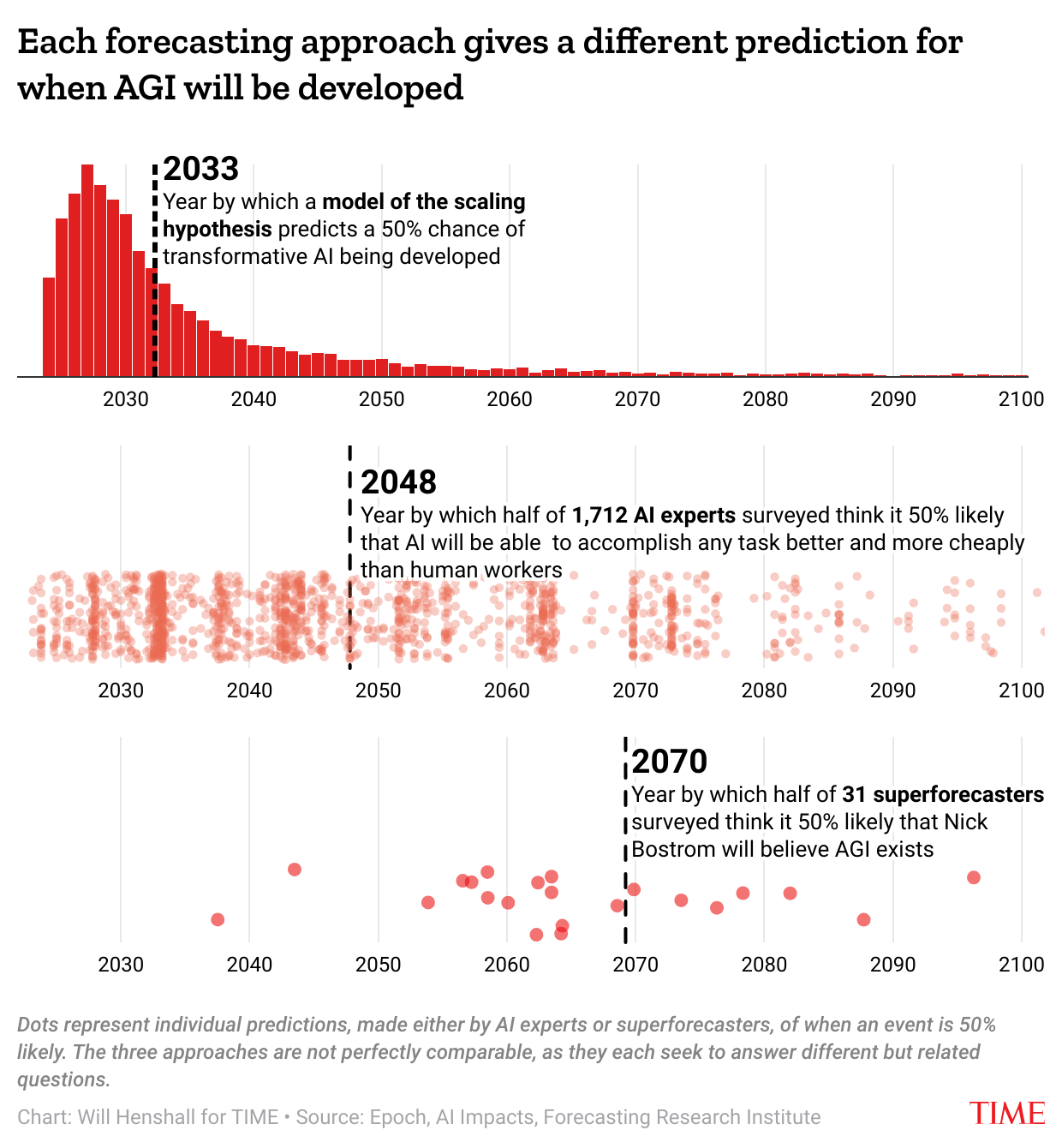
There are many points of disagreement that feed into debates over when AGI might be developed, says Katja Grace, who organized the expert survey as lead researcher at AI Impacts. First, will the current methods for building AI systems, bolstered by more compute and fed more data, with a few algorithmic tweaks, be sufficient? The answer to this question in part depends on how impressive you think recently developed AI systems are. Is GPT-4, in the words of researchers at Microsoft, the sparks of AGI ? Or is this, in the words of philosopher Hubert Dreyfus, “like claiming that the first monkey that climbed a tree was making progress towards landing on the moon?”
Second, even if current methods are enough to achieve the goal of developing AGI, it's unclear how far away the finish line is, says Grace. It’s also possible that something could obstruct progress on the way, for example a shortfall of training data.
Finally, looming in the background of these more technical debates are people’s more fundamental beliefs about how much and how quickly the world is likely to change, Grace says. Those working in AI are often steeped in technology and open to the idea that their creations could alter the world dramatically, whereas most people dismiss this as unrealistic.
The stakes of resolving this disagreement are high. In addition to asking experts how quickly they thought AI would reach certain milestones, AI Impacts asked them about the technology’s societal implications. Of the 1,345 respondents who answered questions about AI’s impact on society, 89% said they are substantially or extremely concerned about AI-generated deepfakes and 73% were similarly concerned that AI could empower dangerous groups, for example by enabling them to engineer viruses. The median respondent thought it was 5% likely that AGI leads to “extremely bad,” outcomes, such as human extinction.
Given these concerns, and the fact that 10% of the experts surveyed believe that AI might be able to do any task a human can by 2030, Grace argues that policymakers and companies should prepare now.
Preparations could include investment in safety research, mandatory safety testing, and coordination between companies and countries developing powerful AI systems, says Grace. Many of these measures were also recommended in a paper published by AI experts last year.
“If governments act now, with determination, there is a chance that we will learn how to make AI systems safe before we learn how to make them so powerful that they become uncontrollable,” Stuart Russell, professor of computer science at the University of California, Berkeley, and one of the paper’s authors, told TIME in October.
More Must-Reads From TIME
- Exclusive: Google Workers Revolt Over $1.2 Billion Contract With Israel
- Stop Looking for Your Forever Home
- Jane Fonda Champions Climate Action for Every Generation
- Hormonal Birth Control Doesn’t Deserve Its Bad Reputation
- The Sympathizer Counters 50 Years of Hollywood Vietnam War Narratives
- Essay: The Relentless Cost of Chronic Diseases
- The Best TV Shows to Watch on Peacock
- Want Weekly Recs on What to Watch, Read, and More? Sign Up for Worth Your Time
Write to Will Henshall at [email protected]
You May Also Like
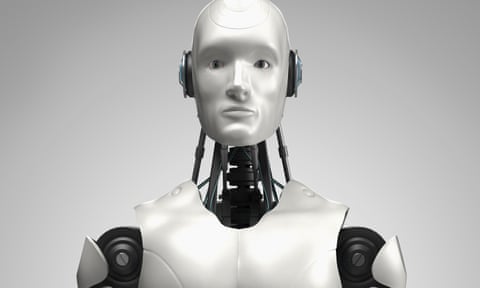
Can we stop AI outsmarting humanity?
The spectre of superintelligent machines doing us harm is not just science fiction, technologists say – so how can we ensure AI remains ‘friendly’ to its makers? By Mara Hvistendahl
I t began three and a half billion years ago in a pool of muck, when a molecule made a copy of itself and so became the ultimate ancestor of all earthly life. It began four million years ago, when brain volumes began climbing rapidly in the hominid line.
Fifty thousand years ago with the rise of Homo sapiens sapiens.
Ten thousand years ago with the invention of civilization.
Five hundred years ago with the invention of the printing press.
Fifty years ago with the invention of the computer.
In less than thirty years, it will end.
Jaan Tallinn stumbled across these words in 2007, in an online essay called Staring into the Singularity . The “it” was human civilisation. Humanity would cease to exist, predicted the essay’s author, with the emergence of superintelligence, or AI, that surpasses human-level intelligence in a broad array of areas.
Tallinn, an Estonia-born computer programmer, has a background in physics and a propensity to approach life like one big programming problem. In 2003, he co-founded Skype, developing the backend for the app. He cashed in his shares after eBay bought it two years later, and now he was casting about for something to do. Staring into the Singularity mashed up computer code, quantum physics and Calvin and Hobbes quotes. He was hooked.
Tallinn soon discovered that the author, Eliezer Yudkowsky, a self-taught theorist, had written more than 1,000 essays and blogposts, many of them devoted to superintelligence. He wrote a program to scrape Yudkowsky’s writings from the internet, order them chronologically and format them for his iPhone. Then he spent the better part of a year reading them.
The term artificial intelligence, or the simulation of intelligence in computers or machines, was coined back in 1956, only a decade after the creation of the first electronic digital computers. Hope for the field was initially high, but by the 1970s, when early predictions did not pan out, an “AI winter” set in. When Tallinn found Yudkowsky’s essays, AI was undergoing a renaissance. Scientists were developing AIs that excelled in specific areas, such as winning at chess, cleaning the kitchen floor and recognising human speech. Such “narrow” AIs, as they are called, have superhuman capabilities, but only in their specific areas of dominance. A chess-playing AI cannot clean the floor or take you from point A to point B. Superintelligent AI, Tallinn came to believe, will combine a wide range of skills in one entity. More darkly, it might also use data generated by smartphone-toting humans to excel at social manipulation.
Reading Yudkowsky’s articles, Tallinn became convinced that superintelligence could lead to an explosion or breakout of AI that could threaten human existence – that ultrasmart AIs will take our place on the evolutionary ladder and dominate us the way we now dominate apes. Or, worse yet, exterminate us.
After finishing the last of the essays, Tallinn shot off an email to Yudkowsky – all lowercase, as is his style. “i’m jaan, one of the founding engineers of skype,” he wrote. Eventually he got to the point: “i do agree that ... preparing for the event of general AI surpassing human intelligence is one of the top tasks for humanity.” He wanted to help.
When Tallinn flew to the Bay Area for other meetings a week later, he met Yudkowsky, who lived nearby, at a cafe in Millbrae, California. Their get-together stretched to four hours. “He actually, genuinely understood the underlying concepts and the details,” Yudkowsky told me recently. “This is very rare.” Afterward, Tallinn wrote a check for $5,000 (£3,700) to the Singularity Institute for Artificial Intelligence, the nonprofit where Yudkowsky was a research fellow. (The organisation changed its name to Machine Intelligence Research Institute , or Miri, in 2013.) Tallinn has since given the institute more than $600,000.
The encounter with Yudkowsky brought Tallinn purpose, sending him on a mission to save us from our own creations. He embarked on a life of travel, giving talks around the world on the threat posed by superintelligence. Mostly, though, he began funding research into methods that might give humanity a way out: so-called friendly AI. That doesn’t mean a machine or agent is particularly skilled at chatting about the weather, or that it remembers the names of your kids – although superintelligent AI might be able to do both of those things. It doesn’t mean it is motivated by altruism or love. A common fallacy is assuming that AI has human urges and values. “Friendly” means something much more fundamental: that the machines of tomorrow will not wipe us out in their quest to attain their goals.
L ast spring, I joined Tallinn for a meal in the dining hall of Cambridge University’s Jesus College. The churchlike space is bedecked with stained-glass windows, gold moulding, and oil paintings of men in wigs. Tallinn sat at a heavy mahogany table, wearing the casual garb of Silicon Valley: black jeans, T-shirt and canvas sneakers. A vaulted timber ceiling extended high above his shock of grey-blond hair.
At 47, Tallinn is in some ways your textbook tech entrepreneur. He thinks that thanks to advances in science (and provided AI doesn’t destroy us), he will live for “many, many years”. When out clubbing with researchers, he outlasts even the young graduate students. His concern about superintelligence is common among his cohort. PayPal co-founder Peter Thiel ’s foundation has given $1.6m to Miri and, in 2015, Tesla founder Elon Musk donated $10m to the Future of Life Institute, a technology safety organisation in Cambridge, Massachusetts. But Tallinn’s entrance to this rarefied world came behind the iron curtain in the 1980s, when a classmate’s father with a government job gave a few bright kids access to mainframe computers. After Estonia became independent, he founded a video-game company. Today, Tallinn still lives in its capital city – also called Tallinn – with his wife and the youngest of his six kids. When he wants to meet with researchers, he often just flies them to the Baltic region.
His giving strategy is methodical, like almost everything else he does. He spreads his money among 11 organisations, each working on different approaches to AI safety, in the hope that one might stick. In 2012, he cofounded the Cambridge Centre for the Study of Existential Risk (CSER) with an initial outlay of close to $200,000.
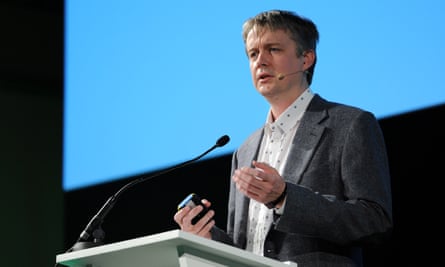
Existential risks – or X-risks, as Tallinn calls them – are threats to humanity’s survival. In addition to AI, the 20-odd researchers at CSER study climate change, nuclear war and bioweapons. But, to Tallinn, those other disciplines “are really just gateway drugs”. Concern about more widely accepted threats, such as climate change, might draw people in. The horror of superintelligent machines taking over the world, he hopes, will convince them to stay. He was visiting Cambridge for a conference because he wants the academic community to take AI safety more seriously.
At Jesus College, our dining companions were a random assortment of conference-goers, including a woman from Hong Kong who was studying robotics and a British man who graduated from Cambridge in the 1960s. The older man asked everybody at the table where they attended university. (Tallinn’s answer, Estonia’s University of Tartu, did not impress him.) He then tried to steer the conversation toward the news. Tallinn looked at him blankly. “I am not interested in near-term risks,” he said.
Tallinn changed the topic to the threat of superintelligence. When not talking to other programmers, he defaults to metaphors, and he ran through his suite of them: advanced AI can dispose of us as swiftly as humans chop down trees. Superintelligence is to us what we are to gorillas.
An AI would need a body to take over, the older man said. Without some kind of physical casing, how could it possibly gain physical control?
Tallinn had another metaphor ready: “Put me in a basement with an internet connection, and I could do a lot of damage,” he said. Then he took a bite of risotto.
E very AI, whether it’s a Roomba or one of its potential world-dominating descendants, is driven by outcomes. Programmers assign these goals, along with a series of rules on how to pursue them. Advanced AI wouldn’t necessarily need to be given the goal of world domination in order to achieve it – it could just be accidental. And the history of computer programming is rife with small errors that sparked catastrophes. In 2010, for example, when a trader with the mutual-fund company Waddell & Reed sold thousands of futures contracts, the firm’s software left out a key variable from the algorithm that helped execute the trade. The result was the trillion-dollar US “ flash crash ”.
The researchers Tallinn funds believe that if the reward structure of a superhuman AI is not properly programmed, even benign objectives could have insidious ends. One well-known example, laid out by the Oxford University philosopher Nick Bostrom in his book Superintelligence , is a fictional agent directed to make as many paperclips as possible. The AI might decide that the atoms in human bodies would be better put to use as raw material.
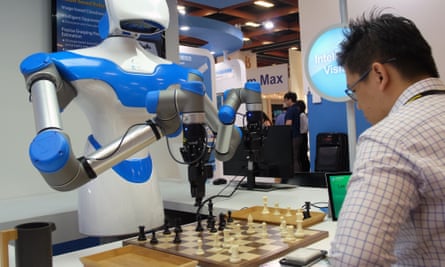
Tallinn’s views have their share of detractors, even among the community of people concerned with AI safety. Some object that it is too early to worry about restricting superintelligent AI when we don’t yet understand it. Others say that focusing on rogue technological actors diverts attention from the most urgent problems facing the field, like the fact that the majority of algorithms are designed by white men, or based on data biased toward them . “We’re in danger of building a world that we don’t want to live in if we don’t address those challenges in the near term,” said Terah Lyons, executive director of the Partnership on AI, a technology industry consortium focused on AI safety and other issues. (Several of the institutes Tallinn backs are members.) But, she added, some of the near-term challenges facing researchers, such as weeding out algorithmic bias, are precursors to ones that humanity might see with super-intelligent AI.
Tallinn isn’t so convinced. He counters that superintelligent AI brings unique threats. Ultimately, he hopes that the AI community might follow the lead of the anti-nuclear movement in the 1940s. In the wake of the bombings of Hiroshima and Nagasaki, scientists banded together to try to limit further nuclear testing. “The Manhattan Project scientists could have said: ‘Look, we are doing innovation here, and innovation is always good, so let’s just plunge ahead,’” he told me. “But they were more responsible than that.”
T allinn warns that any approach to AI safety will be hard to get right. If an AI is sufficiently smart, it might have a better understanding of the constraints than its creators do. Imagine, he said, “waking up in a prison built by a bunch of blind five-year-olds.” That is what it might be like for a super-intelligent AI that is confined by humans.
The theorist Yudkowsky found evidence this might be true when, starting in 2002, he conducted chat sessions in which he played the role of an AI enclosed in a box, while a rotation of other people played the gatekeeper tasked with keeping the AI in. Three out of five times, Yudkowsky – a mere mortal – says he convinced the gatekeeper to release him. His experiments have not discouraged researchers from trying to design a better box, however.
The researchers that Tallinn funds are pursuing a broad variety of strategies, from the practical to the seemingly far-fetched. Some theorise about boxing AI, either physically, by building an actual structure to contain it, or by programming in limits to what it can do. Others are trying to teach AI to adhere to human values. A few are working on a last-ditch off-switch. One researcher who is delving into all three is mathematician and philosopher Stuart Armstrong at Oxford University’s Future of Humanity Institute , which Tallinn calls “the most interesting place in the universe.” (Tallinn has given FHI more than $310,000.)
Armstrong is one of the few researchers in the world who focuses full-time on AI safety. When I met him for coffee in Oxford, he wore an unbuttoned rugby shirt and had the look of someone who spends his life behind a screen, with a pale face framed by a mess of sandy hair. He peppered his explanations with a disorienting mixture of popular-culture references and math. When I asked him what it might look like to succeed at AI safety, he said: “Have you seen the Lego movie ? Everything is awesome.”
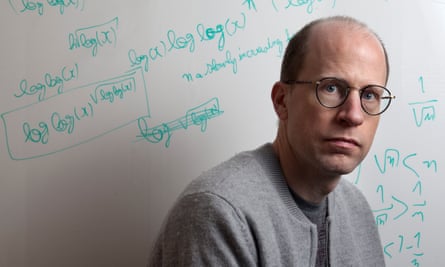
One strain of Armstrong’s research looks at a specific approach to boxing called an “oracle” AI. In a 2012 paper with Nick Bostrom, who co-founded FHI, he proposed not only walling off superintelligence in a holding tank – a physical structure – but also restricting it to answering questions, like a really smart Ouija board . Even with these boundaries, an AI would have immense power to reshape the fate of humanity by subtly manipulating its interrogators. To reduce the possibility of this happening, Armstrong proposes time limits on conversations, or banning questions that might upend the current world order. He also has suggested giving the oracle proxy measures of human survival, like the Dow Jones industrial average or the number of people crossing the street in Tokyo, and telling it to keep these steady.
Ultimately, Armstrong believes, it could be necessary to create, as he calls it in one paper, a “big red off button”: either a physical switch, or a mechanism programmed into an AI to automatically turn itself off in the event of a breakout. But designing such a switch is far from easy. It is not just that an advanced AI interested in self-preservation could prevent the button from being pressed. It could also become curious about why humans devised the button, activate it to see what happens, and render itself useless. In 2013, a programmer named Tom Murphy VII designed an AI that could teach itself to play Nintendo Entertainment System games. Determined not to lose at Tetris, the AI simply pressed pause – and kept the game frozen. “Truly, the only winning move is not to play,” Murphy observed wryly, in a paper on his creation.
For the strategy to succeed, an AI has to be uninterested in the button, or, as Tallinn put it: “It has to assign equal value to the world where it’s not existing and the world where it’s existing.” But even if researchers can achieve that, there are other challenges. What if the AI has copied itself several thousand times across the internet?
The approach that most excites researchers is finding a way to make AI adhere to human values– not by programming them in, but by teaching AIs to learn them. In a world dominated by partisan politics, people often dwell on the ways in which our principles differ. But, Tallinn told me, humans have a lot in common: “Almost everyone values their right leg. We just don’t think about it.” The hope is that an AI might be taught to discern such immutable rules.
In the process, an AI would need to learn and appreciate humans’ less-than-logical side: that we often say one thing and mean another, that some of our preferences conflict with others, and that people are less reliable when drunk. Despite the challenges, Tallinn believes, it is worth trying because the stakes are so high. “We have to think a few steps ahead,” he said. “Creating an AI that doesn’t share our interests would be a horrible mistake.”
O n his last night in Cambridge, I joined Tallinn and two researchers for dinner at a steakhouse. A waiter seated our group in a white-washed cellar with a cave-like atmosphere. He handed us a one-page menu that offered three different kinds of mash. A couple sat down at the table next to us, and then a few minutes later asked to move elsewhere. “It’s too claustrophobic,” the woman complained. I thought of Tallinn’s comment about the damage he could wreak if locked in a basement with nothing but an internet connection. Here we were, in the box. As if on cue, the men contemplated ways to get out.
Tallinn’s guests included former genomics researcher Seán Ó hÉigeartaigh , who is CSER’s executive director, and Matthijs Maas, an AI researcher at the University of Copenhagen. They joked about an idea for a nerdy action flick titled Superintelligence v Blockchain!, and discussed an online game called Universal Paperclips , which riffs on the scenario in Bostrom’s book. The exercise involves repeatedly clicking your mouse to make paperclips. It’s not exactly flashy, but it does give a sense for why a machine might look for more expedient ways to produce office supplies.
Eventually, talk shifted toward bigger questions, as it often does when Tallinn is present. The ultimate goal of AI-safety research is to create machines that are, as Cambridge philosopher and CSER co-founder Huw Price once put it, “ethically as well as cognitively superhuman”. Others have raised the question: if we don’t want AI to dominate us, do we want to dominate AI? In other words, does AI have rights? Tallinn believes this is needless anthropomorphising. It assumes that intelligence equals consciousness – a misconception that annoys many AI researchers. Earlier in the day, CSER researcher José Hernández-Orallo joked that when speaking with AI researchers, consciousness is “the C-word”. (“And ‘free will’ is the F-word,” he added.)
In the cellar, Tallinn said that consciousness is beside the point: “Take the example of a thermostat. No one would say it is conscious. But it’s really inconvenient to face up against that agent if you’re in a room that is set to negative 30 degrees.”
Ó hÉigeartaigh chimed in. “It would be nice to worry about consciousness,” he said, “but we won’t have the luxury to worry about consciousness if we haven’t first solved the technical safety challenges.”
People get overly preoccupied with what superintelligent AI is, Tallinn said. What form will it take? Should we worry about a single AI taking over, or an army of them? “From our perspective, the important thing is what AI does,” he stressed. And that, he believes, may still be up to humans – for now.
This piece originally appeared in Popular Science magazine
- The long read
- Artificial intelligence (AI)
- Internet of things
- Consciousness

Most viewed

An official website of the United States government
The .gov means it’s official. Federal government websites often end in .gov or .mil. Before sharing sensitive information, make sure you’re on a federal government site.
The site is secure. The https:// ensures that you are connecting to the official website and that any information you provide is encrypted and transmitted securely.
- Publications
- Account settings
Preview improvements coming to the PMC website in October 2024. Learn More or Try it out now .
- Advanced Search
- Journal List
- Tzu Chi Med J
- v.32(4); Oct-Dec 2020

The impact of artificial intelligence on human society and bioethics
Michael cheng-tek tai.
Department of Medical Sociology and Social Work, College of Medicine, Chung Shan Medical University, Taichung, Taiwan
Artificial intelligence (AI), known by some as the industrial revolution (IR) 4.0, is going to change not only the way we do things, how we relate to others, but also what we know about ourselves. This article will first examine what AI is, discuss its impact on industrial, social, and economic changes on humankind in the 21 st century, and then propose a set of principles for AI bioethics. The IR1.0, the IR of the 18 th century, impelled a huge social change without directly complicating human relationships. Modern AI, however, has a tremendous impact on how we do things and also the ways we relate to one another. Facing this challenge, new principles of AI bioethics must be considered and developed to provide guidelines for the AI technology to observe so that the world will be benefited by the progress of this new intelligence.
W HAT IS ARTIFICIAL INTELLIGENCE ?
Artificial intelligence (AI) has many different definitions; some see it as the created technology that allows computers and machines to function intelligently. Some see it as the machine that replaces human labor to work for men a more effective and speedier result. Others see it as “a system” with the ability to correctly interpret external data, to learn from such data, and to use those learnings to achieve specific goals and tasks through flexible adaptation [ 1 ].
Despite the different definitions, the common understanding of AI is that it is associated with machines and computers to help humankind solve problems and facilitate working processes. In short, it is an intelligence designed by humans and demonstrated by machines. The term AI is used to describe these functions of human-made tool that emulates the “cognitive” abilities of the natural intelligence of human minds [ 2 ].
Along with the rapid development of cybernetic technology in recent years, AI has been seen almost in all our life circles, and some of that may no longer be regarded as AI because it is so common in daily life that we are much used to it such as optical character recognition or the Siri (speech interpretation and recognition interface) of information searching equipment on computer [ 3 ].
D IFFERENT TYPES OF ARTIFICIAL INTELLIGENCE
From the functions and abilities provided by AI, we can distinguish two different types. The first is weak AI, also known as narrow AI that is designed to perform a narrow task, such as facial recognition or Internet Siri search or self-driving car. Many currently existing systems that claim to use “AI” are likely operating as a weak AI focusing on a narrowly defined specific function. Although this weak AI seems to be helpful to human living, there are still some think weak AI could be dangerous because weak AI could cause disruptions in the electric grid or may damage nuclear power plants when malfunctioned.
The new development of the long-term goal of many researchers is to create strong AI or artificial general intelligence (AGI) which is the speculative intelligence of a machine that has the capacity to understand or learn any intelligent task human being can, thus assisting human to unravel the confronted problem. While narrow AI may outperform humans such as playing chess or solving equations, but its effect is still weak. AGI, however, could outperform humans at nearly every cognitive task.
Strong AI is a different perception of AI that it can be programmed to actually be a human mind, to be intelligent in whatever it is commanded to attempt, even to have perception, beliefs and other cognitive capacities that are normally only ascribed to humans [ 4 ].
In summary, we can see these different functions of AI [ 5 , 6 ]:
- Automation: What makes a system or process to function automatically
- Machine learning and vision: The science of getting a computer to act through deep learning to predict and analyze, and to see through a camera, analog-to-digital conversion and digital signal processing
- Natural language processing: The processing of human language by a computer program, such as spam detection and converting instantly a language to another to help humans communicate
- Robotics: A field of engineering focusing on the design and manufacturing of cyborgs, the so-called machine man. They are used to perform tasks for human's convenience or something too difficult or dangerous for human to perform and can operate without stopping such as in assembly lines
- Self-driving car: Use a combination of computer vision, image recognition amid deep learning to build automated control in a vehicle.
D O HUMAN-BEINGS REALLY NEED ARTIFICIAL INTELLIGENCE ?
Is AI really needed in human society? It depends. If human opts for a faster and effective way to complete their work and to work constantly without taking a break, yes, it is. However if humankind is satisfied with a natural way of living without excessive desires to conquer the order of nature, it is not. History tells us that human is always looking for something faster, easier, more effective, and convenient to finish the task they work on; therefore, the pressure for further development motivates humankind to look for a new and better way of doing things. Humankind as the homo-sapiens discovered that tools could facilitate many hardships for daily livings and through tools they invented, human could complete the work better, faster, smarter and more effectively. The invention to create new things becomes the incentive of human progress. We enjoy a much easier and more leisurely life today all because of the contribution of technology. The human society has been using the tools since the beginning of civilization, and human progress depends on it. The human kind living in the 21 st century did not have to work as hard as their forefathers in previous times because they have new machines to work for them. It is all good and should be all right for these AI but a warning came in early 20 th century as the human-technology kept developing that Aldous Huxley warned in his book Brave New World that human might step into a world in which we are creating a monster or a super human with the development of genetic technology.
Besides, up-to-dated AI is breaking into healthcare industry too by assisting doctors to diagnose, finding the sources of diseases, suggesting various ways of treatment performing surgery and also predicting if the illness is life-threatening [ 7 ]. A recent study by surgeons at the Children's National Medical Center in Washington successfully demonstrated surgery with an autonomous robot. The team supervised the robot to perform soft-tissue surgery, stitch together a pig's bowel, and the robot finished the job better than a human surgeon, the team claimed [ 8 , 9 ]. It demonstrates robotically-assisted surgery can overcome the limitations of pre-existing minimally-invasive surgical procedures and to enhance the capacities of surgeons performing open surgery.
Above all, we see the high-profile examples of AI including autonomous vehicles (such as drones and self-driving cars), medical diagnosis, creating art, playing games (such as Chess or Go), search engines (such as Google search), online assistants (such as Siri), image recognition in photographs, spam filtering, predicting flight delays…etc. All these have made human life much easier and convenient that we are so used to them and take them for granted. AI has become indispensable, although it is not absolutely needed without it our world will be in chaos in many ways today.
T HE IMPACT OF ARTIFICIAL INTELLIGENCE ON HUMAN SOCIETY
Negative impact.
Questions have been asked: With the progressive development of AI, human labor will no longer be needed as everything can be done mechanically. Will humans become lazier and eventually degrade to the stage that we return to our primitive form of being? The process of evolution takes eons to develop, so we will not notice the backsliding of humankind. However how about if the AI becomes so powerful that it can program itself to be in charge and disobey the order given by its master, the humankind?
Let us see the negative impact the AI will have on human society [ 10 , 11 ]:
- A huge social change that disrupts the way we live in the human community will occur. Humankind has to be industrious to make their living, but with the service of AI, we can just program the machine to do a thing for us without even lifting a tool. Human closeness will be gradually diminishing as AI will replace the need for people to meet face to face for idea exchange. AI will stand in between people as the personal gathering will no longer be needed for communication
- Unemployment is the next because many works will be replaced by machinery. Today, many automobile assembly lines have been filled with machineries and robots, forcing traditional workers to lose their jobs. Even in supermarket, the store clerks will not be needed anymore as the digital device can take over human labor
- Wealth inequality will be created as the investors of AI will take up the major share of the earnings. The gap between the rich and the poor will be widened. The so-called “M” shape wealth distribution will be more obvious
- New issues surface not only in a social sense but also in AI itself as the AI being trained and learned how to operate the given task can eventually take off to the stage that human has no control, thus creating un-anticipated problems and consequences. It refers to AI's capacity after being loaded with all needed algorithm may automatically function on its own course ignoring the command given by the human controller
- The human masters who create AI may invent something that is racial bias or egocentrically oriented to harm certain people or things. For instance, the United Nations has voted to limit the spread of nucleus power in fear of its indiscriminative use to destroying humankind or targeting on certain races or region to achieve the goal of domination. AI is possible to target certain race or some programmed objects to accomplish the command of destruction by the programmers, thus creating world disaster.
P OSITIVE IMPACT
There are, however, many positive impacts on humans as well, especially in the field of healthcare. AI gives computers the capacity to learn, reason, and apply logic. Scientists, medical researchers, clinicians, mathematicians, and engineers, when working together, can design an AI that is aimed at medical diagnosis and treatments, thus offering reliable and safe systems of health-care delivery. As health professors and medical researchers endeavor to find new and efficient ways of treating diseases, not only the digital computer can assist in analyzing, robotic systems can also be created to do some delicate medical procedures with precision. Here, we see the contribution of AI to health care [ 7 , 11 ]:
Fast and accurate diagnostics
IBM's Watson computer has been used to diagnose with the fascinating result. Loading the data to the computer will instantly get AI's diagnosis. AI can also provide various ways of treatment for physicians to consider. The procedure is something like this: To load the digital results of physical examination to the computer that will consider all possibilities and automatically diagnose whether or not the patient suffers from some deficiencies and illness and even suggest various kinds of available treatment.
Socially therapeutic robots
Pets are recommended to senior citizens to ease their tension and reduce blood pressure, anxiety, loneliness, and increase social interaction. Now cyborgs have been suggested to accompany those lonely old folks, even to help do some house chores. Therapeutic robots and the socially assistive robot technology help improve the quality of life for seniors and physically challenged [ 12 ].
Reduce errors related to human fatigue
Human error at workforce is inevitable and often costly, the greater the level of fatigue, the higher the risk of errors occurring. Al technology, however, does not suffer from fatigue or emotional distraction. It saves errors and can accomplish the duty faster and more accurately.
Artificial intelligence-based surgical contribution
AI-based surgical procedures have been available for people to choose. Although this AI still needs to be operated by the health professionals, it can complete the work with less damage to the body. The da Vinci surgical system, a robotic technology allowing surgeons to perform minimally invasive procedures, is available in most of the hospitals now. These systems enable a degree of precision and accuracy far greater than the procedures done manually. The less invasive the surgery, the less trauma it will occur and less blood loss, less anxiety of the patients.
Improved radiology
The first computed tomography scanners were introduced in 1971. The first magnetic resonance imaging (MRI) scan of the human body took place in 1977. By the early 2000s, cardiac MRI, body MRI, and fetal imaging, became routine. The search continues for new algorithms to detect specific diseases as well as to analyze the results of scans [ 9 ]. All those are the contribution of the technology of AI.
Virtual presence
The virtual presence technology can enable a distant diagnosis of the diseases. The patient does not have to leave his/her bed but using a remote presence robot, doctors can check the patients without actually being there. Health professionals can move around and interact almost as effectively as if they were present. This allows specialists to assist patients who are unable to travel.
S OME CAUTIONS TO BE REMINDED
Despite all the positive promises that AI provides, human experts, however, are still essential and necessary to design, program, and operate the AI from any unpredictable error from occurring. Beth Kindig, a San Francisco-based technology analyst with more than a decade of experience in analyzing private and public technology companies, published a free newsletter indicating that although AI has a potential promise for better medical diagnosis, human experts are still needed to avoid the misclassification of unknown diseases because AI is not omnipotent to solve all problems for human kinds. There are times when AI meets an impasse, and to carry on its mission, it may just proceed indiscriminately, ending in creating more problems. Thus vigilant watch of AI's function cannot be neglected. This reminder is known as physician-in-the-loop [ 13 ].
The question of an ethical AI consequently was brought up by Elizabeth Gibney in her article published in Nature to caution any bias and possible societal harm [ 14 ]. The Neural Information processing Systems (NeurIPS) conference in Vancouver Canada in 2020 brought up the ethical controversies of the application of AI technology, such as in predictive policing or facial recognition, that due to bias algorithms can result in hurting the vulnerable population [ 14 ]. For instance, the NeurIPS can be programmed to target certain race or decree as the probable suspect of crime or trouble makers.
T HE CHALLENGE OF ARTIFICIAL INTELLIGENCE TO BIOETHICS
Artificial intelligence ethics must be developed.
Bioethics is a discipline that focuses on the relationship among living beings. Bioethics accentuates the good and the right in biospheres and can be categorized into at least three areas, the bioethics in health settings that is the relationship between physicians and patients, the bioethics in social settings that is the relationship among humankind and the bioethics in environmental settings that is the relationship between man and nature including animal ethics, land ethics, ecological ethics…etc. All these are concerned about relationships within and among natural existences.
As AI arises, human has a new challenge in terms of establishing a relationship toward something that is not natural in its own right. Bioethics normally discusses the relationship within natural existences, either humankind or his environment, that are parts of natural phenomena. But now men have to deal with something that is human-made, artificial and unnatural, namely AI. Human has created many things yet never has human had to think of how to ethically relate to his own creation. AI by itself is without feeling or personality. AI engineers have realized the importance of giving the AI ability to discern so that it will avoid any deviated activities causing unintended harm. From this perspective, we understand that AI can have a negative impact on humans and society; thus, a bioethics of AI becomes important to make sure that AI will not take off on its own by deviating from its originally designated purpose.
Stephen Hawking warned early in 2014 that the development of full AI could spell the end of the human race. He said that once humans develop AI, it may take off on its own and redesign itself at an ever-increasing rate [ 15 ]. Humans, who are limited by slow biological evolution, could not compete and would be superseded. In his book Superintelligence, Nick Bostrom gives an argument that AI will pose a threat to humankind. He argues that sufficiently intelligent AI can exhibit convergent behavior such as acquiring resources or protecting itself from being shut down, and it might harm humanity [ 16 ].
The question is–do we have to think of bioethics for the human's own created product that bears no bio-vitality? Can a machine have a mind, consciousness, and mental state in exactly the same sense that human beings do? Can a machine be sentient and thus deserve certain rights? Can a machine intentionally cause harm? Regulations must be contemplated as a bioethical mandate for AI production.
Studies have shown that AI can reflect the very prejudices humans have tried to overcome. As AI becomes “truly ubiquitous,” it has a tremendous potential to positively impact all manner of life, from industry to employment to health care and even security. Addressing the risks associated with the technology, Janosch Delcker, Politico Europe's AI correspondent, said: “I don't think AI will ever be free of bias, at least not as long as we stick to machine learning as we know it today,”…. “What's crucially important, I believe, is to recognize that those biases exist and that policymakers try to mitigate them” [ 17 ]. The High-Level Expert Group on AI of the European Union presented Ethics Guidelines for Trustworthy AI in 2019 that suggested AI systems must be accountable, explainable, and unbiased. Three emphases are given:
- Lawful-respecting all applicable laws and regulations
- Ethical-respecting ethical principles and values
- Robust-being adaptive, reliable, fair, and trustworthy from a technical perspective while taking into account its social environment [ 18 ].
Seven requirements are recommended [ 18 ]:
- AI should not trample on human autonomy. People should not be manipulated or coerced by AI systems, and humans should be able to intervene or oversee every decision that the software makes
- AI should be secure and accurate. It should not be easily compromised by external attacks, and it should be reasonably reliable
- Personal data collected by AI systems should be secure and private. It should not be accessible to just anyone, and it should not be easily stolen
- Data and algorithms used to create an AI system should be accessible, and the decisions made by the software should be “understood and traced by human beings.” In other words, operators should be able to explain the decisions their AI systems make
- Services provided by AI should be available to all, regardless of age, gender, race, or other characteristics. Similarly, systems should not be biased along these lines
- AI systems should be sustainable (i.e., they should be ecologically responsible) and “enhance positive social change”
- AI systems should be auditable and covered by existing protections for corporate whistleblowers. The negative impacts of systems should be acknowledged and reported in advance.
From these guidelines, we can suggest that future AI must be equipped with human sensibility or “AI humanities.” To accomplish this, AI researchers, manufacturers, and all industries must bear in mind that technology is to serve not to manipulate humans and his society. Bostrom and Judkowsky listed responsibility, transparency, auditability, incorruptibility, and predictability [ 19 ] as criteria for the computerized society to think about.
S UGGESTED PRINCIPLES FOR ARTIFICIAL INTELLIGENCE BIOETHICS
Nathan Strout, a reporter at Space and Intelligence System at Easter University, USA, reported just recently that the intelligence community is developing its own AI ethics. The Pentagon made announced in February 2020 that it is in the process of adopting principles for using AI as the guidelines for the department to follow while developing new AI tools and AI-enabled technologies. Ben Huebner, chief of the Office of Director of National Intelligence's Civil Liberties, Privacy, and Transparency Office, said that “We're going to need to ensure that we have transparency and accountability in these structures as we use them. They have to be secure and resilient” [ 20 ]. Two themes have been suggested for the AI community to think more about: Explainability and interpretability. Explainability is the concept of understanding how the analytic works, while interpretability is being able to understand a particular result produced by an analytic [ 20 ].
All the principles suggested by scholars for AI bioethics are well-brought-up. I gather from different bioethical principles in all the related fields of bioethics to suggest four principles here for consideration to guide the future development of the AI technology. We however must bear in mind that the main attention should still be placed on human because AI after all has been designed and manufactured by human. AI proceeds to its work according to its algorithm. AI itself cannot empathize nor have the ability to discern good from evil and may commit mistakes in processes. All the ethical quality of AI depends on the human designers; therefore, it is an AI bioethics and at the same time, a trans-bioethics that abridge human and material worlds. Here are the principles:
- Beneficence: Beneficence means doing good, and here it refers to the purpose and functions of AI should benefit the whole human life, society and universe. Any AI that will perform any destructive work on bio-universe, including all life forms, must be avoided and forbidden. The AI scientists must understand that reason of developing this technology has no other purpose but to benefit human society as a whole not for any individual personal gain. It should be altruistic, not egocentric in nature
- Value-upholding: This refers to AI's congruence to social values, in other words, universal values that govern the order of the natural world must be observed. AI cannot elevate to the height above social and moral norms and must be bias-free. The scientific and technological developments must be for the enhancement of human well-being that is the chief value AI must hold dearly as it progresses further
- Lucidity: AI must be transparent without hiding any secret agenda. It has to be easily comprehensible, detectable, incorruptible, and perceivable. AI technology should be made available for public auditing, testing and review, and subject to accountability standards … In high-stakes settings like diagnosing cancer from radiologic images, an algorithm that can't “explain its work” may pose an unacceptable risk. Thus, explainability and interpretability are absolutely required
- Accountability: AI designers and developers must bear in mind they carry a heavy responsibility on their shoulders of the outcome and impact of AI on whole human society and the universe. They must be accountable for whatever they manufacture and create.
C ONCLUSION
AI is here to stay in our world and we must try to enforce the AI bioethics of beneficence, value upholding, lucidity and accountability. Since AI is without a soul as it is, its bioethics must be transcendental to bridge the shortcoming of AI's inability to empathize. AI is a reality of the world. We must take note of what Joseph Weizenbaum, a pioneer of AI, said that we must not let computers make important decisions for us because AI as a machine will never possess human qualities such as compassion and wisdom to morally discern and judge [ 10 ]. Bioethics is not a matter of calculation but a process of conscientization. Although AI designers can up-load all information, data, and programmed to AI to function as a human being, it is still a machine and a tool. AI will always remain as AI without having authentic human feelings and the capacity to commiserate. Therefore, AI technology must be progressed with extreme caution. As Von der Leyen said in White Paper on AI – A European approach to excellence and trust : “AI must serve people, and therefore, AI must always comply with people's rights…. High-risk AI. That potentially interferes with people's rights has to be tested and certified before it reaches our single market” [ 21 ].
Financial support and sponsorship
Conflicts of interest.
There are no conflicts of interest.
R EFERENCES
AI Has Lost Its Magic
That’s how you know it’s taking over.
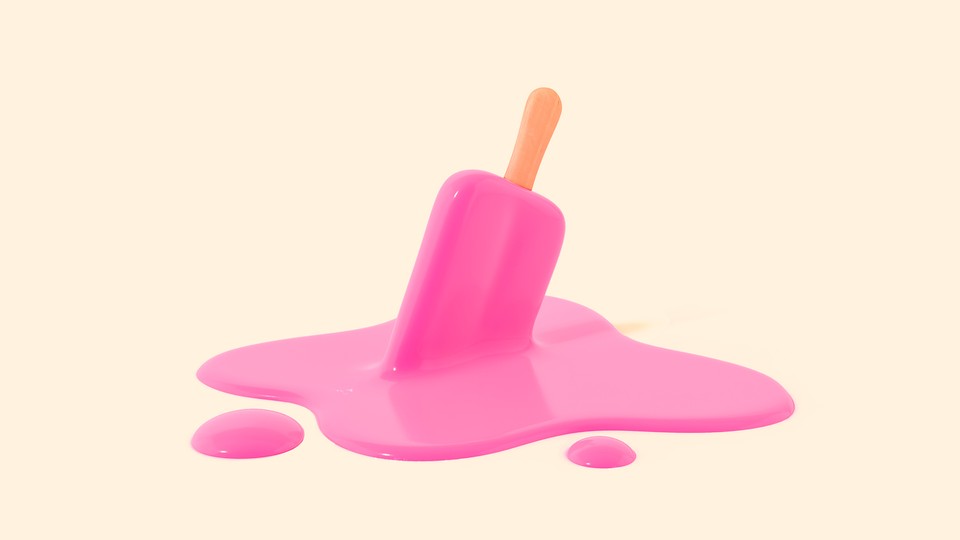
I frequently ask ChatGPT to write poems in the style of the American modernist poet Hart Crane. It does an admirable job of delivering. But the other day, when I instructed the software to give the Crane treatment to a plate of ice-cream sandwiches, I felt bored before I even saw the answer. “The oozing cream, like time, escapes our grasp, / Each moment slipping with a silent gasp.” This was fine. It was competent. I read the poem, Slacked part of it to a colleague, and closed the window. Whatever.
A year and a half has passed since generative AI captured the public imagination and my own. For many months, the fees I paid to ChatGPT and Midjourney felt like money better spent than the cost of my Netflix subscription, even just for entertainment. I’d sit on the couch and generate cheeseburger kaiju while Bridgerton played, unwatched, before me. But now that time is over. The torpor that I felt in asking for Hart Crane’s ode to an ice-cream sandwich seemed to mark the end point of a brief, glorious phase in the history of technology. Generative AI appeared as if from nowhere, bringing magic, both light and dark. If the curtain on that show has now been drawn, it’s not because AI turned out to be a flop. Just the opposite: The tools that it enables have only slipped into the background, from where they will exert their greatest influence.
Looking back at my ChatGPT history, I used to ask for Hart Crane–ice-cream stuff all the time. An Emily Dickinson poem about Sizzler (“In Sizzler’s embrace, we find our space / Where simple joys and flavors interlace”). Edna St. Vincent Millay on Beverly Hills, 90210 (“In sun-kissed land where palm trees sway / Jeans of stone-wash in a bygone day”). Biz Markie and then Eazy-E verses about the (real!) Snoop Dogg cereal Frosted Drizzlerz. A blurb about Rainbow Brite in the style of the philosopher Jacques Derrida. I asked for these things, at first, just to see what each model was capable of doing, to explore how it worked. I found that AI had the uncanny ability to blend concepts both precisely and creatively.
Read: The AI Mona Lisa explains everything
Last autumn, I wrote in The Atlantic that, at its best, generative AI could be used as a tool to supercharge your imagination . I’d been using DALL-E to give a real-ish form to almost any notion that popped into my head. One weekend, I spent most of a family outing stealing moments to build out the fictional, 120-year history of a pear-flavored French soft drink called P’Poire. Then there was Trotter, a cigarette made by and for pigs. I’ve spent so many hours on these sideline pranks that the products now feel real to me. They are real, at least in the way that any fiction—Popeye, Harry Potter—can be real.
But slowly, invisibly, the work of really using AI took over. While researching a story about lemon-lime flavor , I asked ChatGPT to give me an overview of the U.S. market for beverages with this ingredient, but had to do my own research to confirm the facts. In the course of working out new programs of study for my university department, I had the software assess and devise possible names. Neither task produced a fraction of the delight that I’d once derived from just a single AI-generated phrase, “jeans of stone-wash.” But at least the latter gave me what I needed at the time: a workable mediocrity.
I still found some opportunities to supercharge my imagination, but those became less frequent over time. In their place, I assigned AI the mule-worthy burden of mere tasks . Faced with the question of which wait-listed students to admit into an overenrolled computer-science class , I used ChatGPT to apply the relevant and complicated criteria. (If a parent or my provost is reading this, I did not send any student’s actual name or personal data to OpenAI.) In need of a project website on short order, I had the service create one far more quickly than I could have by hand. When I wanted to analyze the full corpus of Wordle solutions for a recent story on the New York Times games library, I asked for help from OpenAI’s Data Analyst. Nobody had promised me any of this, so having something that kind of worked felt like a gift.
The more imaginative uses of AI were always bound to buckle under this actual utility. A year ago, university professors like me were already fretting over the technology’s practical consequences, and we spent many weeks debating whether and how universities could control the use of large language models in assignments. Indeed, for students, generative AI seemed obviously and immediately productive: Right away, it could help them write college essays and do homework . (Teachers found lots of ways to use it, too.) The applications seemed to grow and grow. In November, OpenAI CEO Sam Altman said the ChatGPT service had 100 million weekly users. In January, the job-ratings website Glassdoor put out a survey finding that 62 percent of professionals, including 77 percent of those in marketing, were using ChatGPT at work. And last month, Pew Research Center reported that almost half of American adults believe they interact with AI, in one form or another, several times a week at least.
Read: Things get strange when AI starts training itself
The rapid adoption was in part a function of AI’s novelty—without initial interest, nothing can catch on. But that user growth could be sustained only by the technology’s transition into something unexciting. Inventions become important not when they offer a glimpse of some possible future—as, say, the Apple Vision Pro does right now—but when they’re able to recede into the background, to become mundane. Of course you have a smartphone. Of course you have a refrigerator, a television, a microwave, an automobile. These technologies are not—which is to say, they are no longer —delightful.
Not all inventions lose their shimmer right away, but the ones that change the world won’t take long to seem humdrum. I already miss the feeling of enchantment that came from making new Hart Crane poems or pear-soft-drink ad campaigns. I miss the joy of seeing any imaginable idea brought instantly to life. But whatever nostalgia one might have for the early days of ChatGPT and DALL-E will be no less fleeting in the end. First the magic fades, then the nostalgia. This is what happens to a technology that’s taking over. This is a measure of its power.
📕 Studying HQ
Comprehensive argumentative essay paper on artificial intelligence, rachel r.n..
- February 22, 2024
What You'll Learn
Unraveling the Promise and Peril of Artificial Intelligence
Artificial Intelligence (AI) stands as a hallmark of human innovation, promising to revolutionize industries, economies, and even the fabric of society itself. With its ability to mimic cognitive functions, AI has penetrated various spheres of human existence, from healthcare to finance, transportation to entertainment. However, this technological marvel is not without its controversies and ethical dilemmas. This essay delves into the multifaceted landscape of artificial intelligence, exploring its potential, challenges, and implications for humanity.(Comprehensive Argumentative Essay Paper on Artificial Intelligence)
AI holds the promise of unlocking unprecedented levels of efficiency and productivity across industries . In healthcare, AI-driven diagnostic tools can analyze vast amounts of medical data to detect diseases with higher accuracy and speed than human physicians. Moreover, AI-powered robotic surgeries enable minimally invasive procedures, reducing patient recovery times and risks. In manufacturing, AI-driven automation streamlines production processes, leading to cost savings and higher output. Self-driving cars, a pinnacle of AI innovation, promise safer roads and greater mobility for individuals, while also potentially reducing traffic congestion and emissions.(Comprehensive Argumentative Essay Paper on Artificial Intelligence)
Furthermore, AI has revolutionized the way we interact with technology, enhancing user experiences through natural language processing and personalized recommendations. Virtual assistants like Siri and Alexa have become ubiquitous, simplifying tasks and providing timely information at our fingertips. AI-driven recommendation algorithms power platforms like Netflix and Spotify, catering to individual preferences and shaping our consumption habits.(Comprehensive Argumentative Essay Paper on Artificial Intelligence)
Despite its transformative potential, AI also raises significant concerns regarding privacy , security, and the displacement of human labor. The proliferation of AI-powered surveillance systems raises alarms about encroachments on personal privacy and civil liberties. Facial recognition technology, for instance, poses risks of mass surveillance and wrongful identifications. Moreover, the reliance on AI for critical decision-making, such as in criminal justice or financial markets, raises questions about accountability and transparency. Biases embedded in AI algorithms can perpetuate social inequalities and discrimination, amplifying existing societal injustices.(Comprehensive Argumentative Essay Paper on Artificial Intelligence)
Furthermore, the widespread adoption of AI-driven automation threatens to disrupt labor markets, leading to job displacement and widening economic disparities. Low-skilled workers are particularly vulnerable to being replaced by AI-powered systems, exacerbating socio-economic inequalities. Moreover, the concentration of AI capabilities in the hands of a few powerful corporations raises concerns about monopolistic practices and the concentration of wealth and power.(Comprehensive Argumentative Essay Paper on Artificial Intelligence)
The ethical implications of AI extend beyond its practical applications to f undamental questions about the nature of intelligence, consciousness, and autonomy. As AI systems become increasingly sophisticated, they blur the lines between machine and human cognition, raising questions about the moral status of AI entities. Should AI systems be granted rights and responsibilities akin to human beings? Can AI possess consciousness and subjective experiences? These philosophical inquiries challenge our understanding of personhood and moral agency in the age of artificial intelligence.(Comprehensive Argumentative Essay Paper on Artificial Intelligence)
Furthermore, the development and deployment of AI raise profound ethical dilemmas regarding accountability and control. Who should be held responsible when AI systems malfunction or make erroneous decisions with significant consequences? How can we ensure that AI aligns with human values and ethical principles? These questions underscore the importance of ethical frameworks and regulatory mechanisms to govern the development and use of AI technology responsibly.(Comprehensive Argumentative Essay Paper on Artificial Intelligence)
In conclusion, artificial intelligence holds immense promise as a transformative force for human society, offering solutions to complex problems and augmenting human capabilities. However, its rapid advancement also poses significant challenges and ethical dilemmas that demand careful consideration. As we navigate the evolving landscape of AI, it is imperative to strike a balance between innovation and responsibility, ensuring that AI serves the collective good while upholding fundamental human values and rights. Only through thoughtful reflection, ethical deliberation, and inclusive governance can we harness the full potential of artificial intelligence for the betterment of humanity.(Comprehensive Argumentative Essay Paper on Artificial Intelligence)
Owe, A., & Baum, S. D. (2021). Moral consideration of nonhumans in the ethics of artificial intelligence. AI and Ethics , 1 (4), 517-528. https://scholar.google.com/citations?user=lJxa2TEAAAAJ&hl=en&oi=sra
Heinrichs, B. (2022). Discrimination in the age of artificial intelligence. AI & society , 1-12. https://link.springer.com/article/10.1007/s00146-021-01192-2
Start by filling this short order form order.studyinghq.com
And then follow the progressive flow.
Having an issue, chat with us here
Cathy, CS.
New Concept ? Let a subject expert write your paper for You
Have a subject expert write for you now, have a subject expert finish your paper for you, edit my paper for me, have an expert write your dissertation's chapter, popular topics.
Business StudyingHq Essay Topics and Ideas How to Guides Samples
- Nursing Solutions
- Study Guides
- Free Study Database for Essays
- Privacy Policy
- Writing Service
- Discounts / Offers
Study Hub:
- Studying Blog
- Topic Ideas
- How to Guides
- Business Studying
- Nursing Studying
- Literature and English Studying
Writing Tools
- Citation Generator
- Topic Generator
- Paraphrasing Tool
- Conclusion Maker
- Research Title Generator
- Thesis Statement Generator
- Summarizing Tool
- Terms and Conditions
- Confidentiality Policy
- Cookies Policy
- Refund and Revision Policy
Our samples and other types of content are meant for research and reference purposes only. We are strongly against plagiarism and academic dishonesty.
Contact Us:
📞 +15512677917
2012-2024 © studyinghq.com. All rights reserved
- Skip to content
- Skip to main menu
- Skip to more DW sites
Will artificial intelligence ever rival human thinking?
The narrowness of AI will someday be replaced by artificial general intelligence. But will it have the capability to rival human intelligence and creativity?
Some of the world’s most advanced artificial intelligence (AI) systems, at least the ones the public hear about, are famous for beating human players at chess or poker . Other algorithms are known for their ability to learn how to recognize cats or their inability to recognize people with darker skin.
But are current AI systems anything more than toys? Sure, their ability to play games or identify animals is impressive, but does this help toward creating useful AI systems? To answer this, we need to take a step back and question what the goals of AI are.
AI tries to predict the future by analyzing the past
The fundamental idea behind AI is simple: To analyze patterns from the past to make accurate predictions about the future.
This idea underlies every algorithm, from Google showing you adverts of what it predicts you want to buy, to predicting whether an image of a face is you or your neighbor. AI is also being used to predict whether patients have cancer or not from analyzing medical records and scans.
Pluribus , the poker playing bot, was able to beat the world’s top poker players in 2019 by being able to predict it could out-bluff the humans.
Making predictions requires incredible amounts of data and the power to process it quickly. Pluribus, for example, filters data from billions of card games in a matter of milliseconds. It stiches patterns together to predict the best possible hand to play, always looking back at its data history to achieve the task at hand, never wondering what it means to look forward.
Pluribus, AlphaGo, Amazon Rekognition ― there are many algorithms out there that are incredibly effective at their job, some so good they can beat human experts.
All these examples are proof of how powerful AI can be at making predictions. The question is which task you want it to be good at.
Human intelligence is general, artificial intelligence narrow
AI systems can really only do one task. Pluribus, for example, is so task-specific that it can’t even play another card game like blackjack, let alone drive a car or plan world domination.
This is very much unlike human intelligence. One of our key features is that we can generalize. We become highly skilled at different skills throughout life ― learning everything from how to walk, how to play card games or how to write articles. We might specialize in a few of those skills, even making a career out of some, but we’re still capable of learning and performing other tasks in our lives.
What’s more, we can also transfer skills, using knowledge of one thing to acquire skills in another. AI systems fundamentally don’t work this way. They learn through endless repetition, or at least until the energy bill gets too high, improving prediction accuracy through trillions of iterations and sheer weight of calculations.
If developers want AI to be as versatile as human intelligence, then AI needs to start being able to have more generalizable and transferable intelligence.
Artificial general intelligence
And the narrowness of AI is changing. What’s set to revolutionize computing is artificial general intelligence (AGI). Much like humans, AGIs will be able to do several tasks at once, each one of them at an expert level.
AGIs like this haven’t been developed yet, but according to Irina Higgins, research scientist at Google subsidiary DeepMind, we’re not far off.
Higgins told DW that ʺ10-15 years ago people thought AGI was a crazy pipe dream. They thought it was 1,500 years away, maybe never. But it’s happening in our lifetime.ʺ
The modest plans are to use AGI to help us answer the really big problems in science, like space exploration or curing cancer.
But the more you read about the potential of AGI, the more the narrative becomes more science fiction than science ― think silicon, plastic and metal beings calling themselves humans or super-computers running city-wide bureaucracies.
Transformative AI is broadening artificial intelligence
While AGI leans more towards science fiction, developments in the field of transformative AI belong firmly in the nonfiction category.
"Even though AI is very, very task specific, people are broadening the tasks a computer can do," Eng Lim Goh, Chief Technology Officer at Hewlett Packard Enterprise, told DW.
One of the first transformative AI systems already in use are Large Language Models (LLMs).
"LLMs started by autocorrecting misspelt words in texts. Then they were trained to autocomplete sentences. And now, because they’ve processed so much text data, they can have a conversation with you," he said, referring to chatbots.
The capabilities of LLMs have been broadened further from there. Now the systems are able to provide responses not just to text but also to images.
"But keep in mind that these systems are still very narrow when you compare it to someone’s job. LLMs can’t understand human meaning of texts and images. They can’t creatively use texts and images like humans can," Goh said.
Some readers’ minds might now be wandering to AI ‘art’ – algorithms like DALL-E 2 that generate images based on input texts.
But is this art? Is this evidence that machines can create? It’s open for philosophical debate, but according to many observers, AI does not create art but merely imitates it.
To mis-quote Ludwig Wittgenstein, "my words have meaning, your AI’s do not."
Edited by: Carla Bleiker
Explore more
Dall-e 2 ai image creator, related topics.

Oxford University Press's Academic Insights for the Thinking World

Is humanity a passing phase in evolution of intelligence and civilisation?

Living Computers: Replicators, Information Processing, and the Evolution of Life
- By Alvis Brazma
- April 2 nd 2024
“The History of every major Galactic Civilization tends to pass through three distinct and recognizable phases, those of Survival, Inquiry and Sophistication…”
Douglas Adams, The Hitchhiker’s Guide to The Galaxy (1979)
“I think it’s quite conceivable that humanity is just a passing phase in the evolution of intelligence.”
Geoffrey Hinton (2023)
In light of the recent spectacular developments in artificial intelligence (AI), questions are now being asked about whether AI could present a danger to humanity. Can AI take over from us? Is humanity a passing phase in the evolution of intelligence and civilisation? Let’s look at these questions from the long-term evolutionary perspective.
Life has existed on Earth for more than three billion years, humanity for less than 0.01% of this time, and civilisation for even less. A billion years from now, our Sun will start expanding and the Earth will soon become too hot for life. Thus, evolutionarily, life on our planet is already reaching old age, while human civilisation has just been born. Can AI help our civilisation to outlast the habitable Solar system and, possibly, life itself, as we know it presently?
Defining life is not easy, but few will disagree that an essential feature of life is its ability to process information. Every animal brain does this, every living cell does this, and even more fundamentally, evolution is continuously processing information residing in the entire collection of genomes on Earth, via the genetic algorithm of Darwin’s survival of the fittest. There is no life without information.
It can be argued that until very recently on the evolutionary timescale, i.e. until human language evolved, most information that existed on Earth and was durable enough to last for more than a generation, was recorded in DNA or in some other polymer molecules. The emergence of human language changed this; with language, information started accumulating in other media, such as clay tablets, paper, or computer memory chips. Most likely, information is now growing faster in the world’s libraries and computer clouds than in the DNA of all genomes of all species.
We can refer to this “new” information as cultural information as opposed to the genetic information of DNA. Cultural information is the basis of a civilisation; genetic information is the basis of life underpinning it. Thus, if genetic information got too damaged, life, cultural information, and civilisation itself would disappear soon. But could this change in the future? There is no civilisation without cultural information, but can there be a civilisation without genetic information? Can our civilisation outlast the Solar system in the form of AI? Or will genetic information always be needed to underpin any civilisation?
For now, AI exists only as information in computer hardware, built and maintained by humans. For AI to exist autonomously, it would need to “break out” of the “information world” of bits and bytes into the physical world of atoms and molecules. AI would need robots maintaining and repairing the hardware on which it is run, recycling the materials from which this hardware is built, and mining for replacement ones. Moreover, this artificial robot/computer “ecosystem” would not only have to maintain itself, but as the environment changes, would also have to change and adapt.
Life, as we know it, has been evolving for billions of years. It has evolved to process information and materials by zillions of nano-scale molecular “machines” all working in parallel, competing as well as backing each other up, maintaining themselves and the ecosystem supporting them. The total complexity of this machinery, also called the biosphere, is mindboggling. In DNA, one bit of information takes less than 50 atoms. Given the atomic nature of physical matter, every part in life’s machinery is as miniature as possible in principle. Can AI achieve such a complexity, robustness, and adaptability by alternative means and without DNA?
Although this is hard to imagine, cultural evolution has produced tools not known to biological evolution. We can now record information as electron density distribution in a silicon crystal at 3 nm scale. Information can be processed much faster in a computer chip than in a living cell. Human brains contain about 10 11 neurons each, which probably is close to the limit how many neurons a single biological brain can contain. Though this is more than computer hardware currently offers to AI, for future AI systems, this is not a limit. Moreover, humans have to communicate information among each other via the bottleneck of language; computers do not have such a limitation.
Where does this all leave us? Will the first two phases in the evolution of life—information mostly confined to DNA, and then information “breaking out” of the DNA harness but still underpinned by information in DNA, be followed by the third phase? Will information and its processing outside living organisms become robust enough to survive and thrive without the underpinning DNA? Will our civilisation be able to outlast the Solar system, and if so, will this happen with or without DNA?
To get to that point, our civilisation first needs to survive its infancy. For now, AI cannot exist without humans. For now, AI can only take over from us if we help it to do so. And indeed, among all the envisioned threats of AI, the most realistic one seems to be deception and spread of misinformation. In other words, corrupting information. Stopping this trend is our biggest near-term challenge.
Feature image by Daniel Falcão via Unsplash .
Alvis Brazma is a Senior Scientist at the European Molecular Biology Laboratory (EMBL) - European Bioinformatics Institute (EBI), Cambridge, UK. He has worked in the discipline of bioinformatics-the science looking at biology from the perspective of information-from its very earliest days. He has published over 150 scientific papers on a wide range of subjects, from computer science to biology (and the links between the two), including in the highest impact journals such as Nature and Science, and has been cited almost 50,000 times.
- Mathematics
- Science & Medicine
Our Privacy Policy sets out how Oxford University Press handles your personal information, and your rights to object to your personal information being used for marketing to you or being processed as part of our business activities.
We will only use your personal information to register you for OUPblog articles.
Or subscribe to articles in the subject area by email or RSS
Related posts:

Recent Comments
There are currently no comments.
Leave a Comment
Your email address will not be published. Required fields are marked *
Save my name, email, and website in this browser for the next time I comment.
April 10, 2024
UC Berkeley's only nonpartisan political magazine
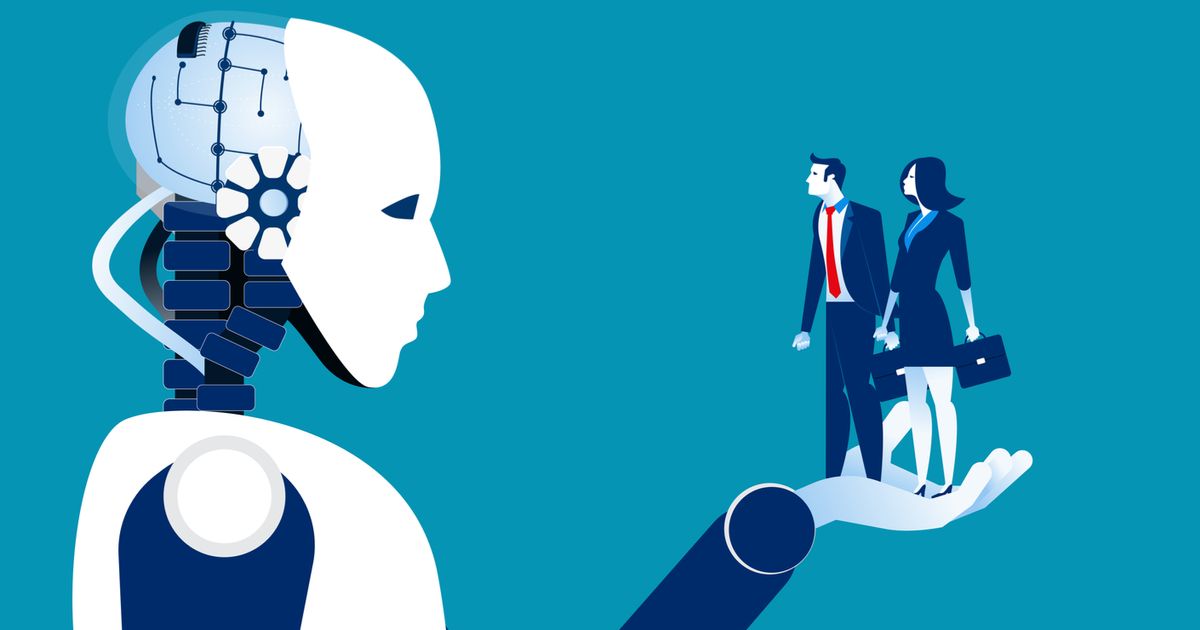
Artificial Intelligence and the Loss of Humanity
The term “artificial intelligence,” or AI, has become a buzzword in recent years. Optimists see AI as the panacea to society’s most fundamental problems, from crime to corruption to inequality, while pessimists fear that AI will overtake human intelligence and crown itself king of the world. Underlying these two seemingly antithetical views is the assumption that AI is better and smarter than humanity and will ultimately replace humanity in making decisions.
It is easy to buy into the hype of omnipotent artificial intelligence these days, as venture capitalists dump billions of dollars into tech start-ups and government technocrats boast of how AI helps them streamline municipal governance . But the hype is just hype: AI is simply not as smart as we think. The true threat of AI to humanity lies not in the power of AI itself but in the ways people are already beginning to use it to chip away at our humanity.
AI outperforms humans, but only in low-level tasks.
Artificial intelligence is a field in computer science that seeks to have computers perform certain tasks by simulating human intelligence. Although the founding fathers of AI in the 1950s and 1960s experimented with manually codifying knowledge into computer systems, most of today’s AI application is carried out via a statistical approach through machine learning, thanks to the proliferation of big data and computational power in recent years. However, today’s AI is still limited to the performance of specialized tasks, such as classifying images, recognizing patterns and generating sentences.
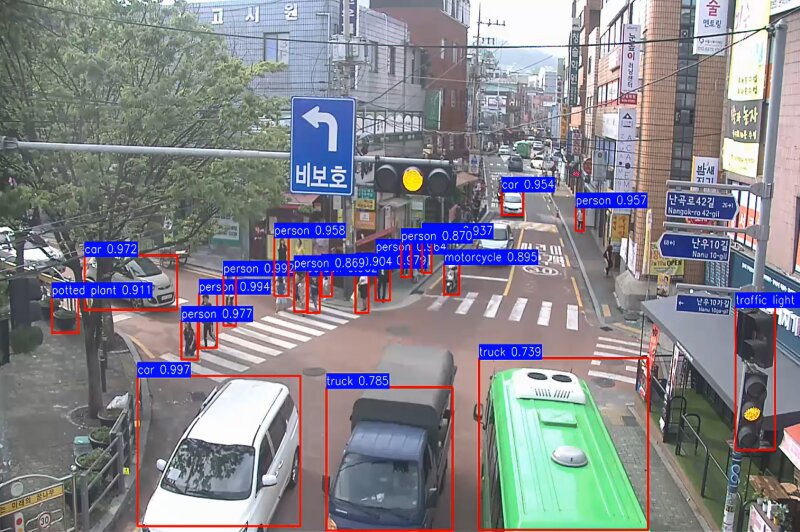
Although a specialized AI might outperform humans in its specific function, it does not understand the logic and principles of its actions. An AI that classifies images, for example, might label images of cats and dogs more accurately than a human, but it never knows how a cat is similar to and different from a dog. Similarly, a natural language processing (NLP) AI can train a model that projects English words onto vectors, but it does not comprehend the etymology and context of each individual word. AI performs tasks mechanically without understanding the content of the tasks, which means that it is certainly not able to outsmart its human masters in a dystopian manner and will not reach such a level for a long time, if ever.
AI does not dehumanize humans — humans do.
AI does not understand humanity, but the epistemological wall between AI and humanity is further complicated by the fact that humans do not understand AI, either. A typical AI model easily contains hundreds of thousands of parameters, whose weights are fine-tuned according to some mathematical principles in order to minimize “loss,” a rough estimate of how wrong the model is. The design of the loss function and its minimization process are often more art than science. We do not know what the weights in the model mean or how the model predicts one result rather than another. Without an explainable framework, decision-making driven by AI is a black box , unaccountable and even inhumane.
This is more than just a theoretical concern. This year in China, local authorities rolled out the so-called “health code,” a QR code assigned to each individual using an AI-powered risk assessment algorithm indicating their risk of contracting and spreading COVID-19. There have been numerous pieces of news coverage about citizens who found their health codes suddenly turning from green (low-risk) to red (high risk) for no reason. They became “digital refugees” as they were immediately banned from entering public venues, including grocery stores, which require green codes. Nobody knows how the risk assessment algorithm works under the hood, yet, in this trying time of coronavirus, it is determining people’s day-to-day lives.
AI applications can intervene in human agency.
Artificial intelligence is also transforming the medical industry. Predictive algorithms are now powering brain-computer interfaces (BCIs) that can read signals from the brain and even write in signals if necessary. For example, a BCI can identify a seizure and act to suppress the symptom, a potentially life-saving application of AI. But BCIs also create problems concerning agency. Who is controlling one’s brain — the user or the machine?
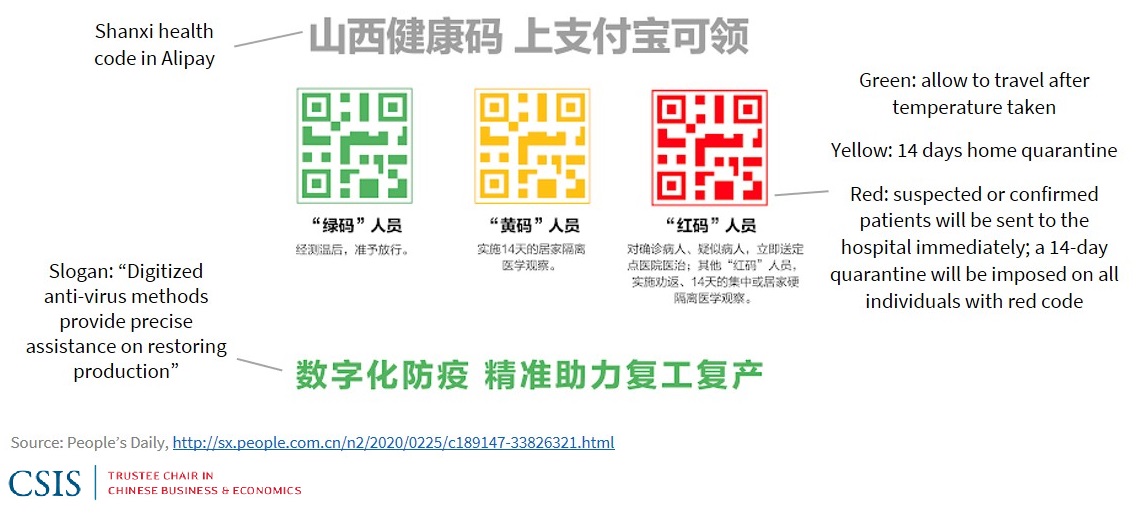
One need not plug their brain into some electronic device to face this issue of agency. The newsfeed of our social medias is constantly using artificial intelligence to push us content based on patterns from our views, likes, moves of the mouse and number of seconds we spend scrolling through a page. We are passive consumers in a deluge of information tailored to our tastes, no longer having to actively reach out to find information — because that information finds us.
AI knows nothing about culture and values.
Feeding an AI system requires data, the representation of information. Some information, such as gender, age and temperature, can be easily coded and quantified. However, there is no way to uniformly quantify complex emotions, beliefs, cultures, norms and values. Because AI systems cannot process these concepts, the best they can do is to seek to maximize benefits and minimize losses for people according to mathematical principles. This utilitarian logic, though, often contravenes what we would consider noble from a moral standpoint — prioritizing the weak over the strong, safeguarding the rights of the minority despite giving up greater overall welfare and seeking truth and justice rather than telling lies.
The fact that AI does not understand culture or values does not imply that AI is value-neutral. Rather, any AI designed by humans is implicitly value-laden. It is consciously or unconsciously imbued with the belief system of its designer. Biases in AI can come from the representativeness of the historical data, the ways in which data scientists clean and interpret the data, which categorizing buckets the model is designed to output, the choice of loss function and other design features. A more aggressive company culture, for example, might favor maximizing recall in AI, or the proportion of positives identified as positive, while a more prudent culture would encourage maximizing precision, the proportion of labelled positives that are actually positive. While such a distinction might seem trivial, in a medical setting, it can become an issue of life and death: do we try to distribute as much of a treatment as possible despite its side effects, or do we act more prudently to limit the distribution of the treatment to minimize side effects, even if many people will never get the treatment? Within a single AI model, these two goals can never be achieved simultaneously because they are mathematically opposed to each other. People have to make a choice when designing an AI system, and the choice they make will inevitably reflect the values of the designers.
Take responsibility, now.
AI may or may not outsmart human beings one day — we simply do not know. What we do know is that AI is already changing power dynamics and interpersonal relations today. Government institutions and corporations run the risk of treating atomized individuals as miniscule data points to be aggregated and tapped by AI programs, devoid of personal idiosyncrasies, specialized needs, or unconditional moral worth. This dehumanization is further amplified by the winner-takes-all logic of AI platform economies that creates mighty monopolies, resulting in a situation in which even the smallest decisions made by these companies have the power to erode human agency and autonomy. In order to mitigate the side effects of AI applications, academia, civil society, regulators and corporations must join forces in ensuring that human-centric AI will empower humanity and make our world a better place.
Featured image source: Odyssey
Published in Multimedia
- artifical intelligence
- Artificial Intelligence
- machine learning
Xiantao studies Sociology and Data Science at UC Berkeley. He writes on Hong Kong, U.S.-China relations, and technology.
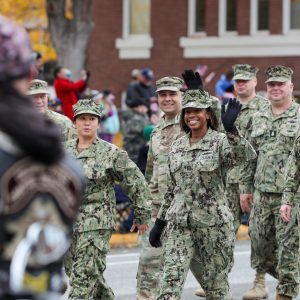
Comments are closed.
Free Essay – Artificial intelligence (Al) and human intelligence
Free essay – artificial intelligence (ai) and human intelligence.
Significant progress in AI has been achieved in recent years, especially with the development of machine learning and deep learning algorithms. By virtue of these developments, AI is now capable of activities formerly associated solely with human intellect, such as pattern recognition, natural language comprehension, and even the production of works of art. Though AI has made great strides, it has a long way to go before it can compete with human intellect in terms of complexity and adaptability.
Artificial intelligence (AI) is not likely to replace human intelligence for several reasons. Biological processes support human intellect, whereas algorithms and mathematical models form the basis of AI and are no less potent, but are fundamentally different. To yet, artificial intelligence has been unable to replicate the whole range of human intellect, which includes not just logical reasoning but also emotions, intuition, and original thought. More importantly, human intellect is formed over the course of a lifetime of events and learning, which is difficult for an AI system to mimic.
But it’s also impossible to deny that AI might one day be smarter than humans at some tasks. An example is the ability of AI to analyse large volumes of data considerably more quickly and correctly than a person. Because of this, AI is extremely helpful in areas like data analysis, where it can spot patterns and trends that a human being would have no hope of spotting. The speed and accuracy with which AI can complete such activities greatly outpaces that of any human.
Assuming that intelligence is a zero-sum game, however, the concept of AI replacing human intellect is problematic. Perhaps a more fruitful perspective would be to regard AI not as a competitor to human intellect but as a means to expand and improve upon it. Our strengths as humans lie in areas where AI has yet to make significant inroads, such as strategic thinking, creativity, and emotional intelligence. By working together in this way, AI and human intellect may thrive.
Finally, while AI has made tremendous strides and may one day be smarter than humans, it is still far from replacing us completely. Given the unique characteristics of AI and the potential for it to complement human intellect rather than replace it, it seems likely that the two will coexist and mutually enrich one another in the future. Keeping these in mind as we advance AI research and development is essential to guaranteeing that the technology will be used for the benefit of humanity.
You can also check other Research here:
- Accounting Research Project
- Adult Education
- Agricultural Science
- Banking & Finance
- Biblical Theology & CRS
- Biblical Theology and CRS
- Biology Education
- Business Administration
- Computer Engineering Project
- Computer Science 2
- Criminology Research Project
- Early Childhood Education
- Economic Education
- Education Research Project
- Educational Administration and Planning Research Project
- English Education
- Entrepreneurship
- Environmental Sciences Research Project
- Guidance and Counselling Research Project
- History Education
- Human Kinetics and Health Education
- Maritime and Transportation
- Marketing Research Project 2
- Mass Communication
- Mathematics Education
- Medical Biochemistry Project
- Organizational Behaviour
32 Other Projects pdf doc
- Political Science
- Public Administration
- Public Health Research Project
- More Research Project
- Transportation Management
Free Essay – Artificial intelligence (Al) and human intelligence?
Related Posts
Is pop culture really discouraging students from completing their academic programme, debate: private school is better than public schools, full project – an evaluation of effective management of teachers’ job-related stress in some selected primary schools.
WhatsApp us
Is Artificial Intelligence Replacing Jobs? Here's The Truth
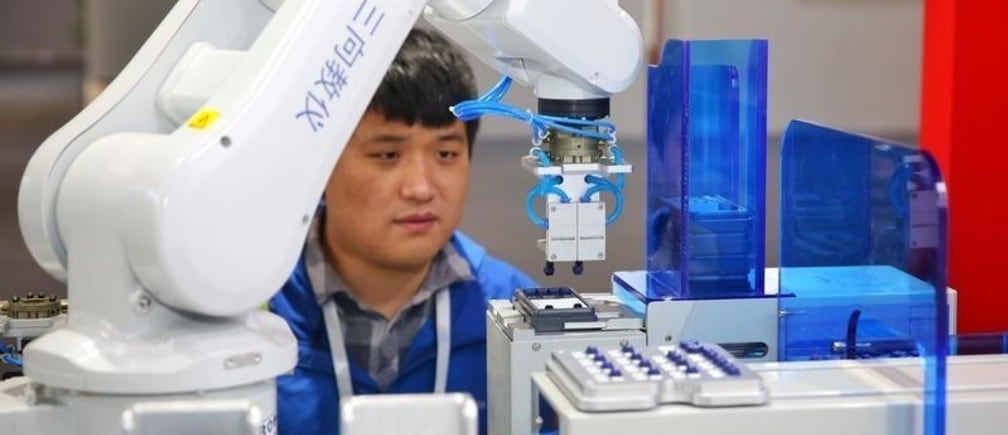
A robotic arm assembles an electronic calculator at the Convention on the Exchange of Overseas Talents in Guangzhou Image: REUTERS/Stringer
.chakra .wef-1c7l3mo{-webkit-transition:all 0.15s ease-out;transition:all 0.15s ease-out;cursor:pointer;-webkit-text-decoration:none;text-decoration:none;outline:none;color:inherit;}.chakra .wef-1c7l3mo:hover,.chakra .wef-1c7l3mo[data-hover]{-webkit-text-decoration:underline;text-decoration:underline;}.chakra .wef-1c7l3mo:focus,.chakra .wef-1c7l3mo[data-focus]{box-shadow:0 0 0 3px rgba(168,203,251,0.5);} John Hawksworth
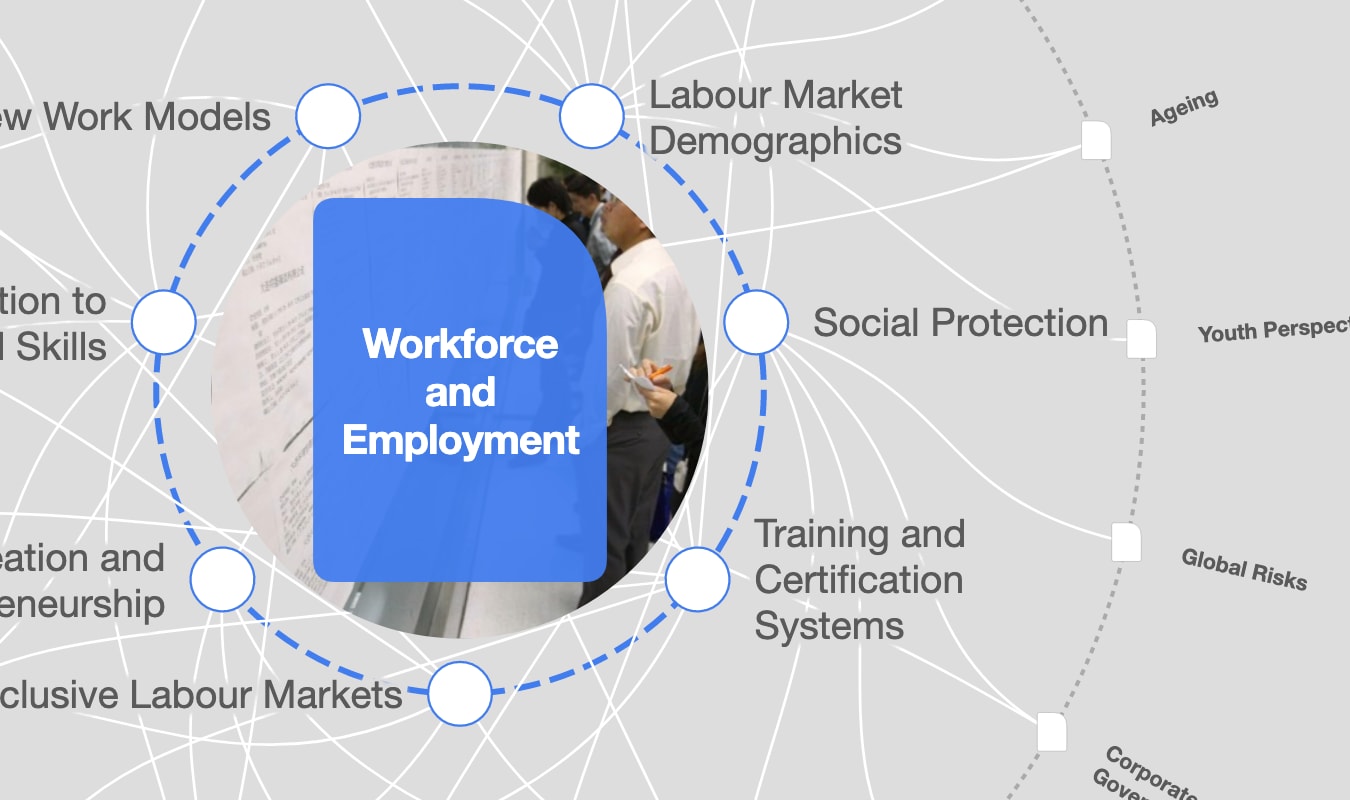
.chakra .wef-9dduvl{margin-top:16px;margin-bottom:16px;line-height:1.388;font-size:1.25rem;}@media screen and (min-width:56.5rem){.chakra .wef-9dduvl{font-size:1.125rem;}} Explore and monitor how .chakra .wef-15eoq1r{margin-top:16px;margin-bottom:16px;line-height:1.388;font-size:1.25rem;color:#F7DB5E;}@media screen and (min-width:56.5rem){.chakra .wef-15eoq1r{font-size:1.125rem;}} Future of Work is affecting economies, industries and global issues
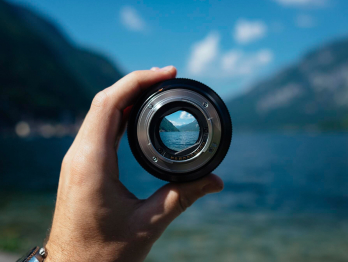
.chakra .wef-1nk5u5d{margin-top:16px;margin-bottom:16px;line-height:1.388;color:#2846F8;font-size:1.25rem;}@media screen and (min-width:56.5rem){.chakra .wef-1nk5u5d{font-size:1.125rem;}} Get involved with our crowdsourced digital platform to deliver impact at scale
Stay up to date:, future of work.
Automation is nothing new – machines have been replacing human workers at a gradual rate ever since the Industrial Revolution. This happened first in agriculture and skilled crafts like hand weaving, then in mass manufacturing and, in more recent decades, in many clerical tasks.
As the extra income generated by these technological advances has been recycled into the economy, new demand for human labour has been generated and there have, generally, still been plenty of jobs to go round.
But a new generation of smart machines, fuelled by rapid advances in artificial intelligence (AI) and robotics, could potentially replace a large proportion of existing human jobs . While some new jobs would be created as in the past, the concern is there may not be enough of these to go round, particularly as the cost of smart machines falls over time and their capabilities increase.
Is artificial intelligence replacing jobs?
There is an element of truth in this argument, and indeed our own past research suggests that up to 30% of existing jobs across the OECD could be at potential risk of automation by the mid-2030s .
But this is not the whole truth for two main reasons, which we explore in detail in recent research published for the UK and a new report on China which will launch at the World Economic Forum’s meeting in Tianjin in September 2018 .
Firstly, just because a job has the technical potential to be automated does not mean this will definitely happen. There is a variety of economic, political, regulatory and organizational factors that could block or at least significantly delay automation. Based on our probabilistic risk analysis, our central estimate is that only around 20% of existing UK jobs may actually be displaced by AI and related technologies over the 20 years to 2037, rising to around 26% in China owing to the higher potential for automation there particularly in manufacturing and agriculture. We refer to this as the ‘displacement effect’.
Secondly, and more importantly, AI and related technologies will also boost economic growth and so create many additional job opportunities, just as other past waves of technological innovation have done from steam engines to computers. In particular, AI systems and robots will boost productivity, reduce costs and improve the quality and range of products that companies can produce.
Successful firms will boost profits as a result, much of which will be reinvested either in those companies or in other businesses by shareholders receiving dividends and realising capital gains. To stay competitive, firms will ultimately have to pass most of these benefits on to consumers in the form of lower (quality-adjusted) prices, which will have the effect of increasing real income levels. This means that households can buy more with their money and, as a result, firms will need to hire additional workers to respond to the extra demand. We refer to this as the income effect, which offsets the displacement effect on jobs.
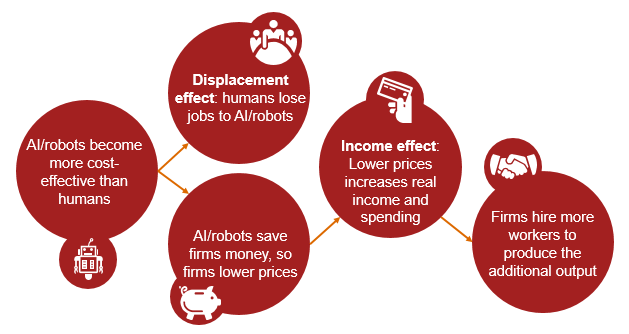
Our new research put some numbers on these job displacement and income effects for the UK, which we have found from past research is fairly typical of OECD economies as a whole; and China, the largest of the emerging economies.
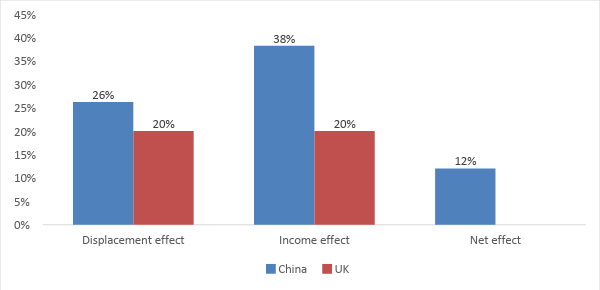
For the UK, the estimated net impact on jobs is broadly neutral, with around 7 million jobs (20%) projected to be displaced in our central scenario but a similar number of new jobs being created. More detailed analysis suggests significant net job gains in sectors like healthcare, where demand will rise due to an ageing population but where there are also limits to the scope for automation because of the continued need for a human touch. Significant job displacement in areas like manufacturing and, as driverless vehicles roll out across the economy, transport and logistics will offset these gains.
For China, there is an estimated negative net impact on agricultural employment, continuing a long-standing trend, more than offset by large increases in construction and services. As for the UK, healthcare will be one area with considerable potential for net job gains given China’s rapidly ageing population.
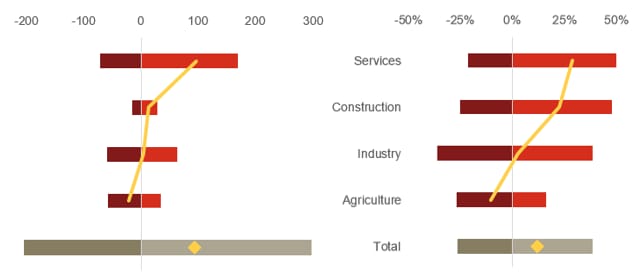
One result that might seem surprising is that the impact on jobs in China’s industrial sector is estimated to be broadly neutral. This reflects the fact that while there will be considerable scope for further automation in Chinese manufacturing as wages there rise, we also estimate that China will take the lead in manufacturing the AI-enhanced products (robots, driverless vehicles, drones etc) that will come out of this Fourth Industrial Revolution.
More generally, the huge boost to the Chinese economy from AI and related technologies, which we estimate could be more than 20% of GDP by 2030, will raise real incomes across the economy. This will create new demand for goods and services that will require additional human workers to produce, particularly in areas that are harder to automate.
No room for complacency – the challenge for government and business
While our estimates suggest that fears of mass technological unemployment are probably unfounded, this is not a recipe for complacency. As with past industrial revolutions, this latest one will bring considerable disruption to both labour markets and existing business models.
In China, we could see around 200 million existing jobs displaced over the next two decades, which will require workers to move to industry sectors and places where new jobs will be created. Of course, China has seen even larger movements of workers from the farms to the cities since the early 1980s, but the process will not be easy. Given China’s ageing population, an increase in immigration may be required to meet the demand for additional workers.
Have you read?
Two reasons computers won't destroy all the jobs, how new technologies can create huge numbers of meaningful jobs.
Both government and business have a role in maximizing the benefits from AI and related technologies while minimizing the costs. The latter will require increased investment in retraining workers for new careers, boosting their digital skills but also reframing the education system to focus on human skills that are less easy to automate: creativity, co-operation, personal communication, and managerial and entrepreneurial skills. Businesses too have a role to play in encouraging a culture of lifelong learning amongst their workers.
For government, AI will boost economic growth and, therefore, tax revenues. This should enable social safety nets, including state health and social care systems, to be strengthened for those who find it difficult to adjust to the new technologies. Such measures will be important if the huge potential benefits of AI and related technologies are to spread as widely as possible across society.
Read the full report: The net impact of AI and related technologies on jobs in China
Don't miss any update on this topic
Create a free account and access your personalized content collection with our latest publications and analyses.
License and Republishing
World Economic Forum articles may be republished in accordance with the Creative Commons Attribution-NonCommercial-NoDerivatives 4.0 International Public License, and in accordance with our Terms of Use.
The views expressed in this article are those of the author alone and not the World Economic Forum.
Related topics:
The agenda .chakra .wef-n7bacu{margin-top:16px;margin-bottom:16px;line-height:1.388;font-weight:400;} weekly.
A weekly update of the most important issues driving the global agenda
.chakra .wef-1dtnjt5{display:-webkit-box;display:-webkit-flex;display:-ms-flexbox;display:flex;-webkit-align-items:center;-webkit-box-align:center;-ms-flex-align:center;align-items:center;-webkit-flex-wrap:wrap;-ms-flex-wrap:wrap;flex-wrap:wrap;} More on Future of Work .chakra .wef-17xejub{-webkit-flex:1;-ms-flex:1;flex:1;justify-self:stretch;-webkit-align-self:stretch;-ms-flex-item-align:stretch;align-self:stretch;} .chakra .wef-nr1rr4{display:-webkit-inline-box;display:-webkit-inline-flex;display:-ms-inline-flexbox;display:inline-flex;white-space:normal;vertical-align:middle;text-transform:uppercase;font-size:0.75rem;border-radius:0.25rem;font-weight:700;-webkit-align-items:center;-webkit-box-align:center;-ms-flex-align:center;align-items:center;line-height:1.2;-webkit-letter-spacing:1.25px;-moz-letter-spacing:1.25px;-ms-letter-spacing:1.25px;letter-spacing:1.25px;background:none;padding:0px;color:#B3B3B3;-webkit-box-decoration-break:clone;box-decoration-break:clone;-webkit-box-decoration-break:clone;}@media screen and (min-width:37.5rem){.chakra .wef-nr1rr4{font-size:0.875rem;}}@media screen and (min-width:56.5rem){.chakra .wef-nr1rr4{font-size:1rem;}} See all
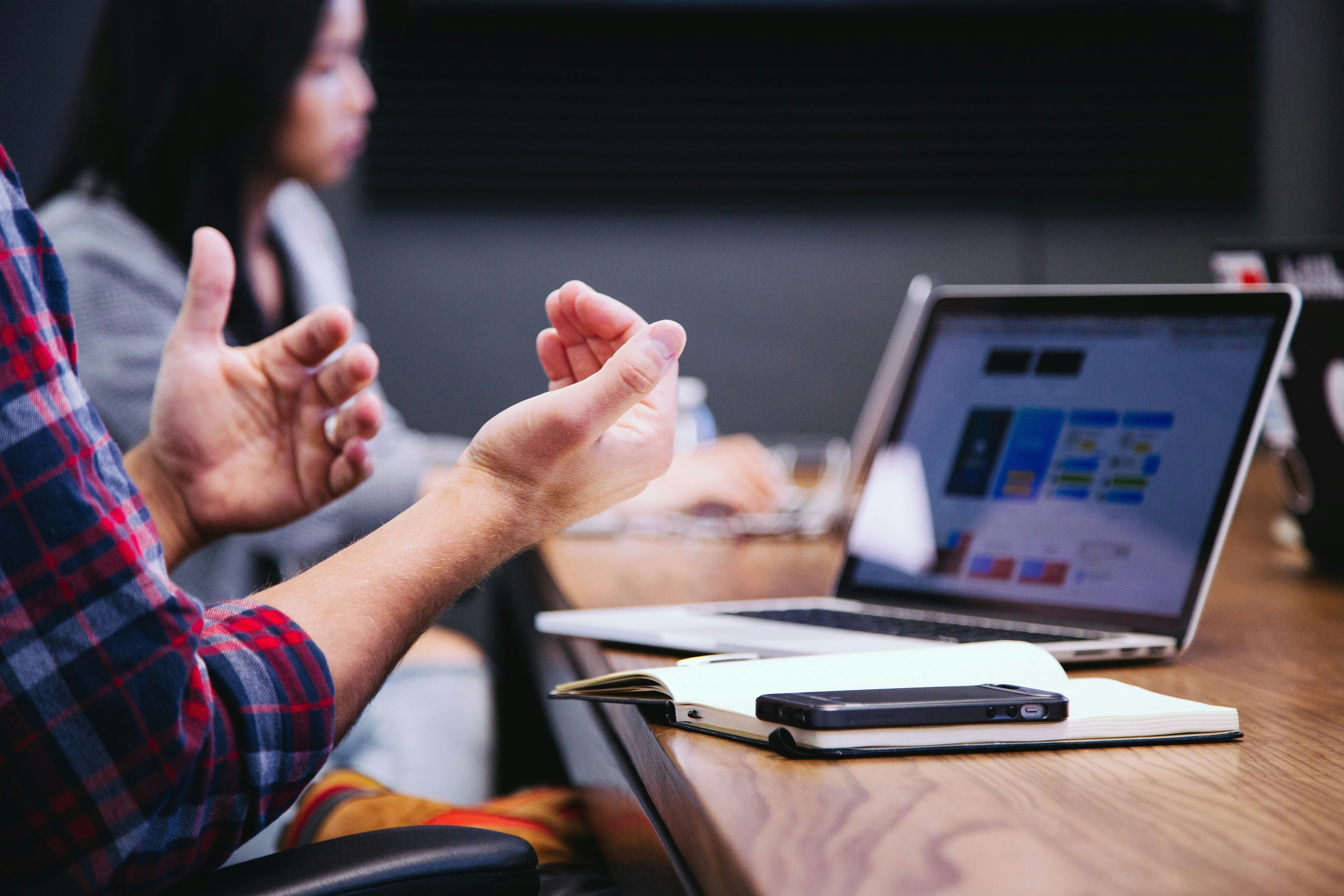
Green job vacancies are on the rise – but workers with green skills are in short supply
Andrea Willige
February 29, 2024
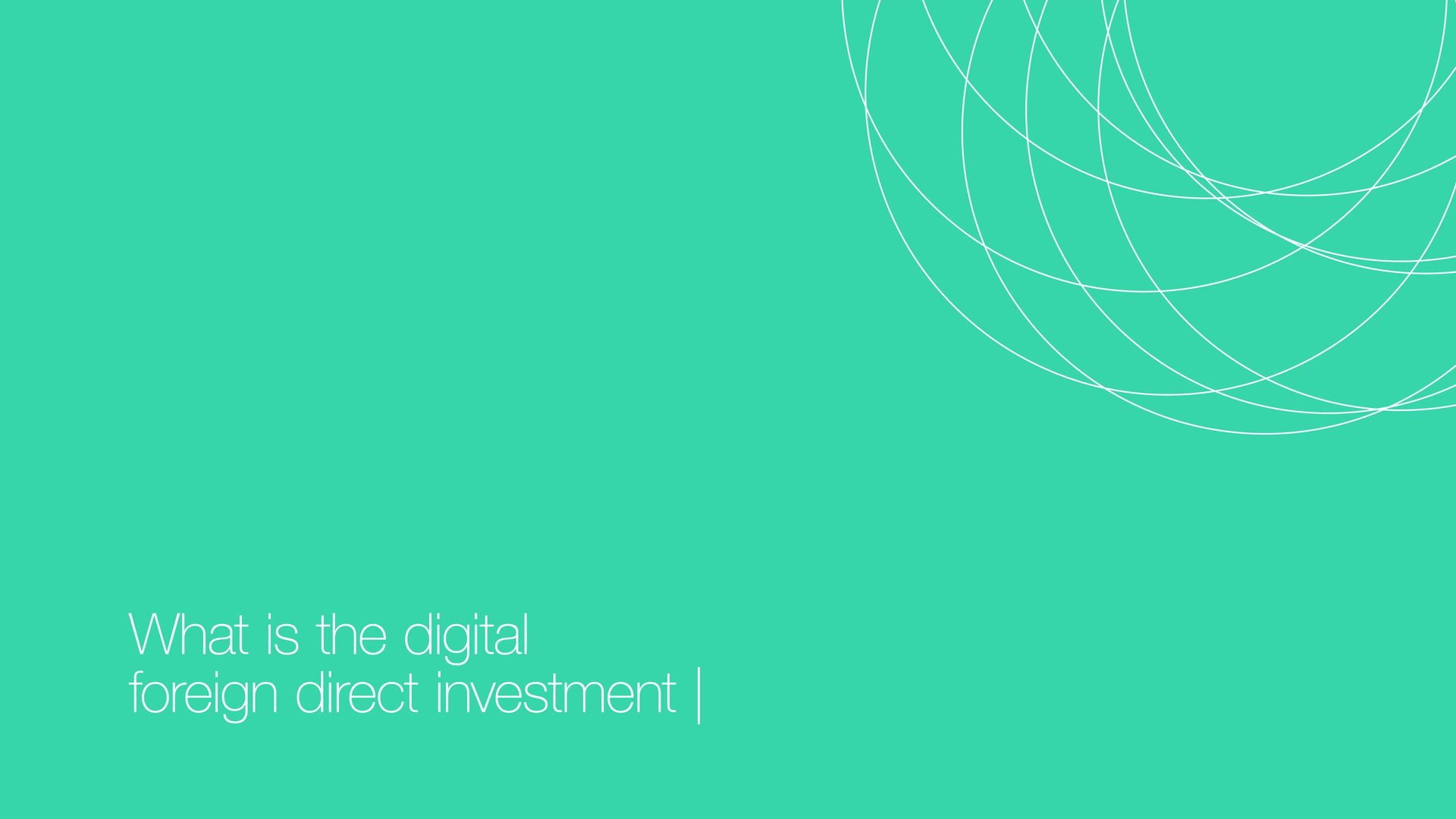
Digital Cooperation Organization - Deemah Al Yahya
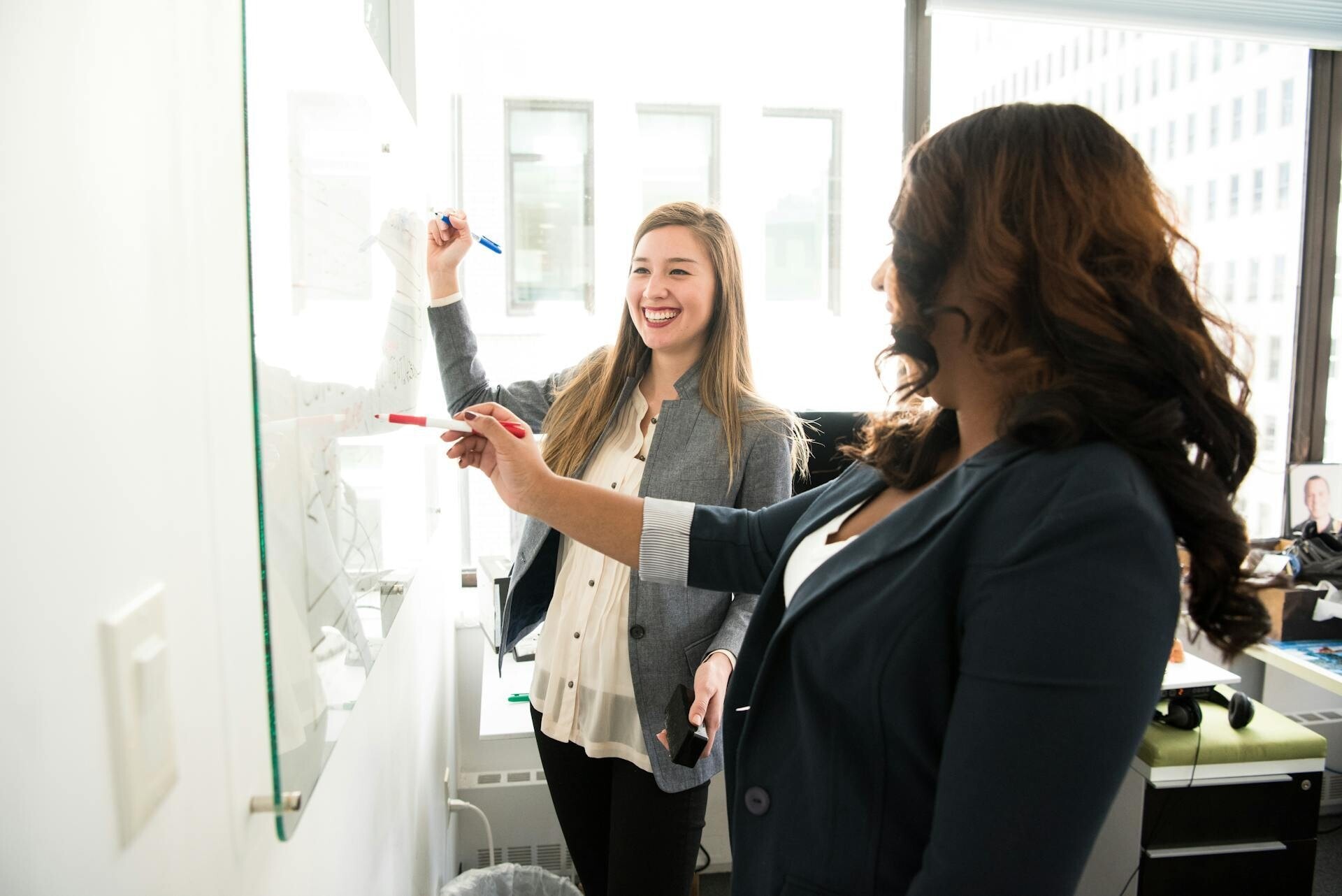
Why clear job descriptions matter for gender equality
Kara Baskin
February 22, 2024
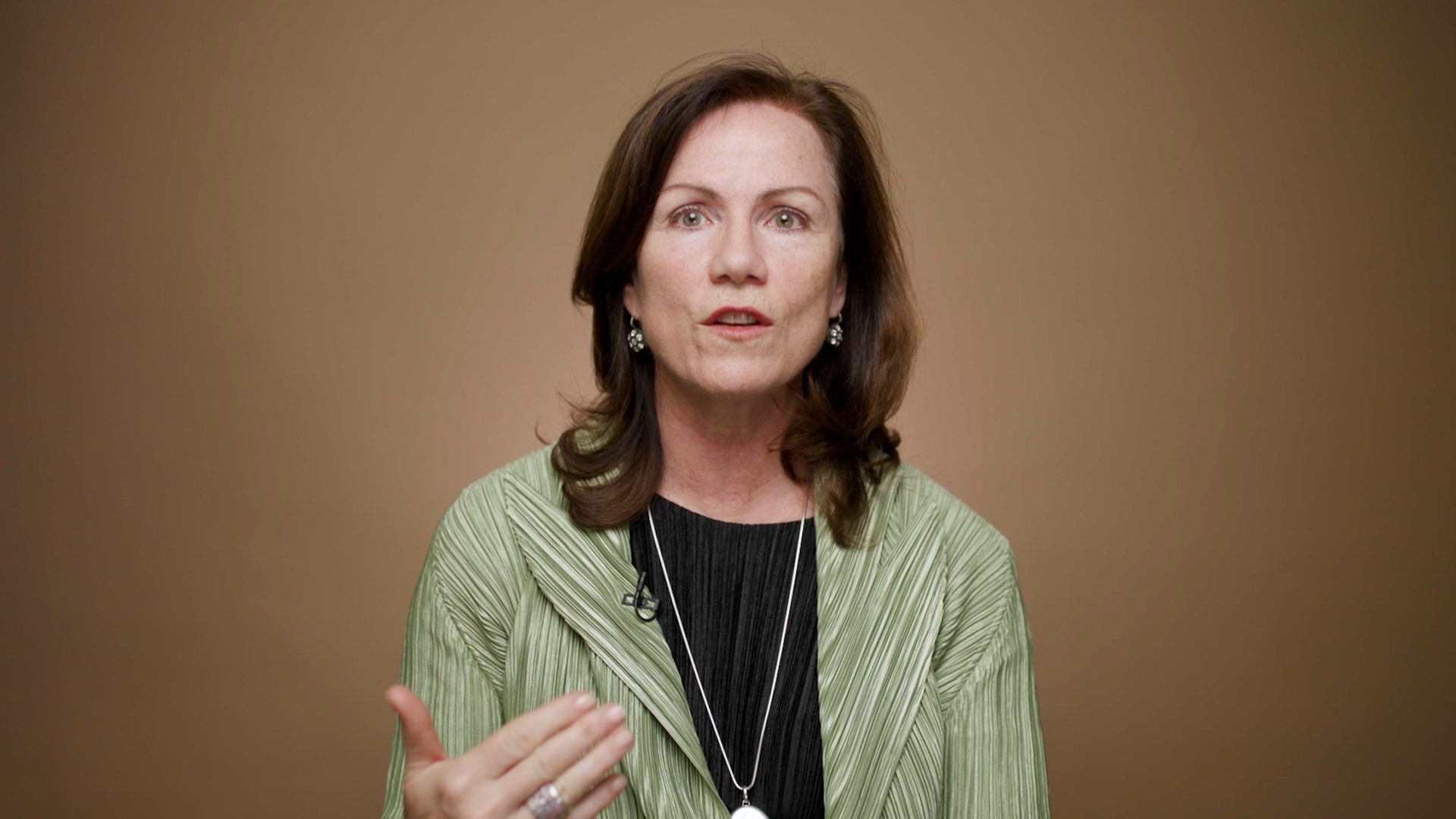
Improve staff well-being and your workplace will run better, says this CEO
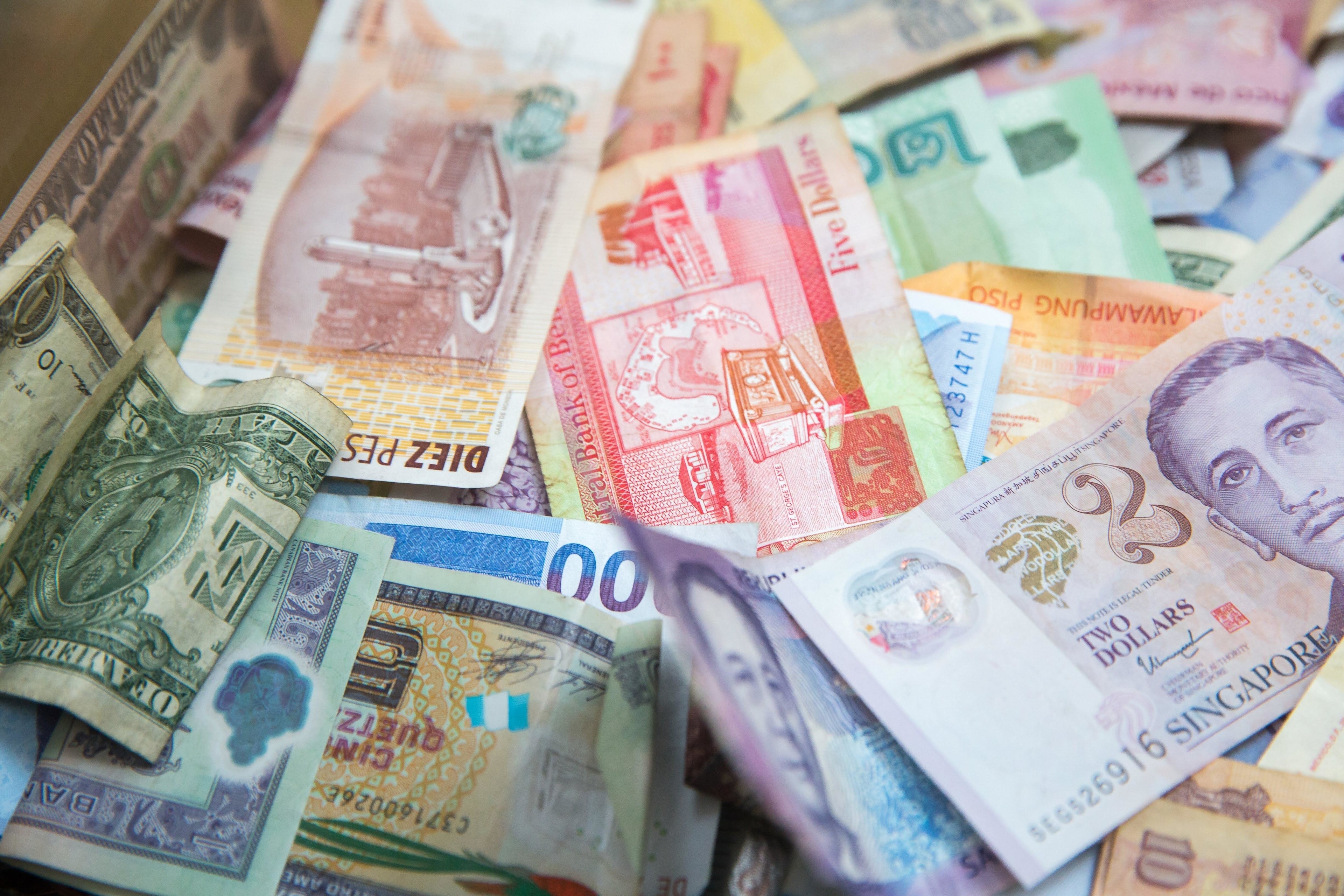
Explainer: What is a recession?
Stephen Hall and Rebecca Geldard
February 19, 2024
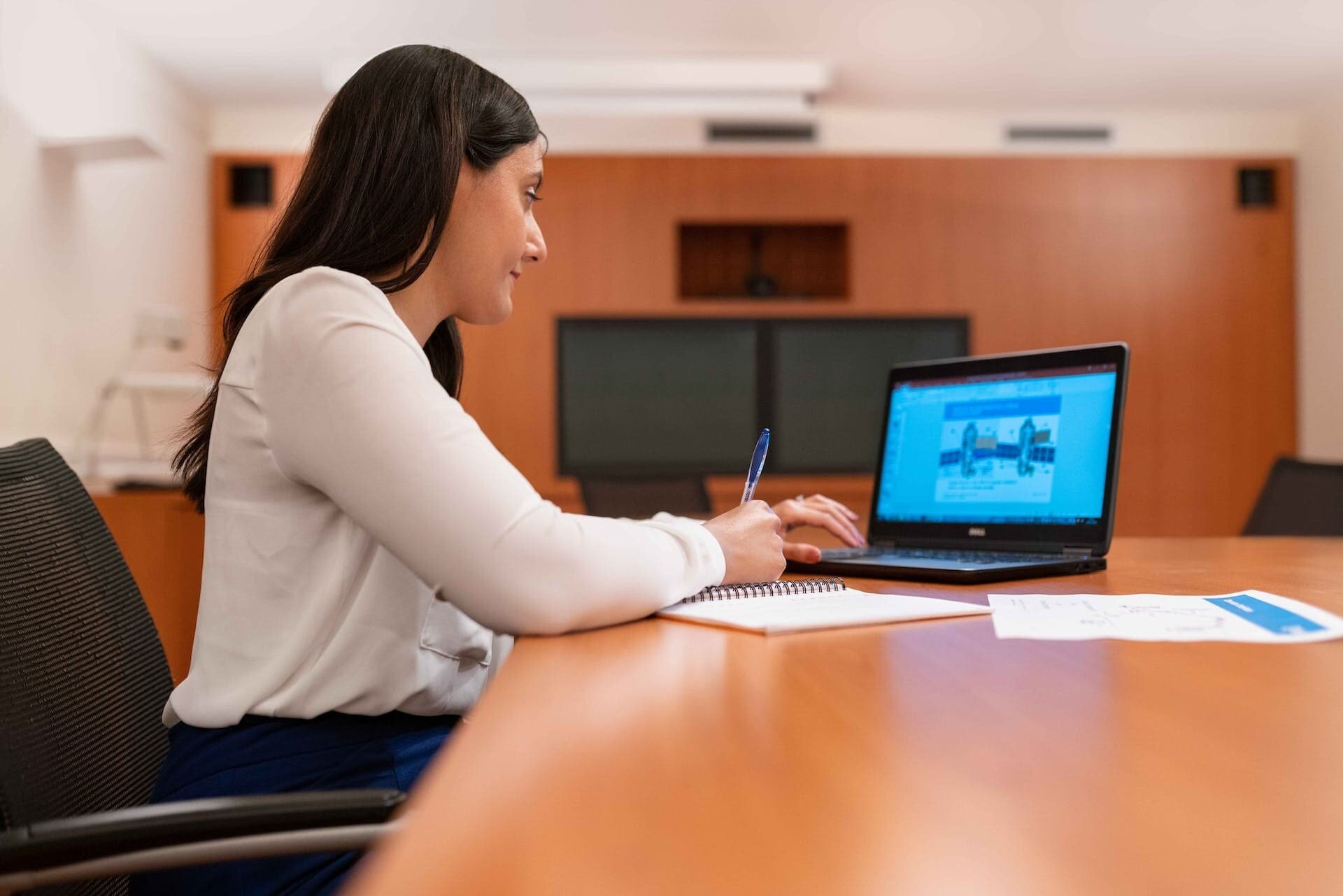
Is your organization ignoring workplace bullying? Here's why it matters
Jason Walker and Deborah Circo
February 12, 2024
Artificial Intelligence Essay for Students and Children
500+ words essay on artificial intelligence.
Artificial Intelligence refers to the intelligence of machines. This is in contrast to the natural intelligence of humans and animals. With Artificial Intelligence, machines perform functions such as learning, planning, reasoning and problem-solving. Most noteworthy, Artificial Intelligence is the simulation of human intelligence by machines. It is probably the fastest-growing development in the World of technology and innovation . Furthermore, many experts believe AI could solve major challenges and crisis situations.

Types of Artificial Intelligence
First of all, the categorization of Artificial Intelligence is into four types. Arend Hintze came up with this categorization. The categories are as follows:
Type 1: Reactive machines – These machines can react to situations. A famous example can be Deep Blue, the IBM chess program. Most noteworthy, the chess program won against Garry Kasparov , the popular chess legend. Furthermore, such machines lack memory. These machines certainly cannot use past experiences to inform future ones. It analyses all possible alternatives and chooses the best one.
Type 2: Limited memory – These AI systems are capable of using past experiences to inform future ones. A good example can be self-driving cars. Such cars have decision making systems . The car makes actions like changing lanes. Most noteworthy, these actions come from observations. There is no permanent storage of these observations.
Type 3: Theory of mind – This refers to understand others. Above all, this means to understand that others have their beliefs, intentions, desires, and opinions. However, this type of AI does not exist yet.
Type 4: Self-awareness – This is the highest and most sophisticated level of Artificial Intelligence. Such systems have a sense of self. Furthermore, they have awareness, consciousness, and emotions. Obviously, such type of technology does not yet exist. This technology would certainly be a revolution .
Get the huge list of more than 500 Essay Topics and Ideas
Applications of Artificial Intelligence
First of all, AI has significant use in healthcare. Companies are trying to develop technologies for quick diagnosis. Artificial Intelligence would efficiently operate on patients without human supervision. Such technological surgeries are already taking place. Another excellent healthcare technology is IBM Watson.
Artificial Intelligence in business would significantly save time and effort. There is an application of robotic automation to human business tasks. Furthermore, Machine learning algorithms help in better serving customers. Chatbots provide immediate response and service to customers.

AI can greatly increase the rate of work in manufacturing. Manufacture of a huge number of products can take place with AI. Furthermore, the entire production process can take place without human intervention. Hence, a lot of time and effort is saved.
Artificial Intelligence has applications in various other fields. These fields can be military , law , video games , government, finance, automotive, audit, art, etc. Hence, it’s clear that AI has a massive amount of different applications.
To sum it up, Artificial Intelligence looks all set to be the future of the World. Experts believe AI would certainly become a part and parcel of human life soon. AI would completely change the way we view our World. With Artificial Intelligence, the future seems intriguing and exciting.
{ “@context”: “https://schema.org”, “@type”: “FAQPage”, “mainEntity”: [{ “@type”: “Question”, “name”: “Give an example of AI reactive machines?”, “acceptedAnswer”: { “@type”: “Answer”, “text”: “Reactive machines react to situations. An example of it is the Deep Blue, the IBM chess program, This program defeated the popular chess player Garry Kasparov.” } }, { “@type”: “Question”, “name”: “How do chatbots help in business?”, “acceptedAnswer”: { “@type”: “Answer”, “text”:”Chatbots help in business by assisting customers. Above all, they do this by providing immediate response and service to customers.”} }] }
Customize your course in 30 seconds
Which class are you in.

- Travelling Essay
- Picnic Essay
- Our Country Essay
- My Parents Essay
- Essay on Favourite Personality
- Essay on Memorable Day of My Life
- Essay on Knowledge is Power
- Essay on Gurpurab
- Essay on My Favourite Season
- Essay on Types of Sports
Leave a Reply Cancel reply
Your email address will not be published. Required fields are marked *
Download the App

AI Index Report
The AI Index Report tracks, collates, distills, and visualizes data related to artificial intelligence. Our mission is to provide unbiased, rigorously vetted, broadly sourced data in order for policymakers, researchers, executives, journalists, and the general public to develop a more thorough and nuanced understanding of the complex field of AI. The report aims to be the world’s most credible and authoritative source for data and insights about AI.
Read the 2023 AI Index Report
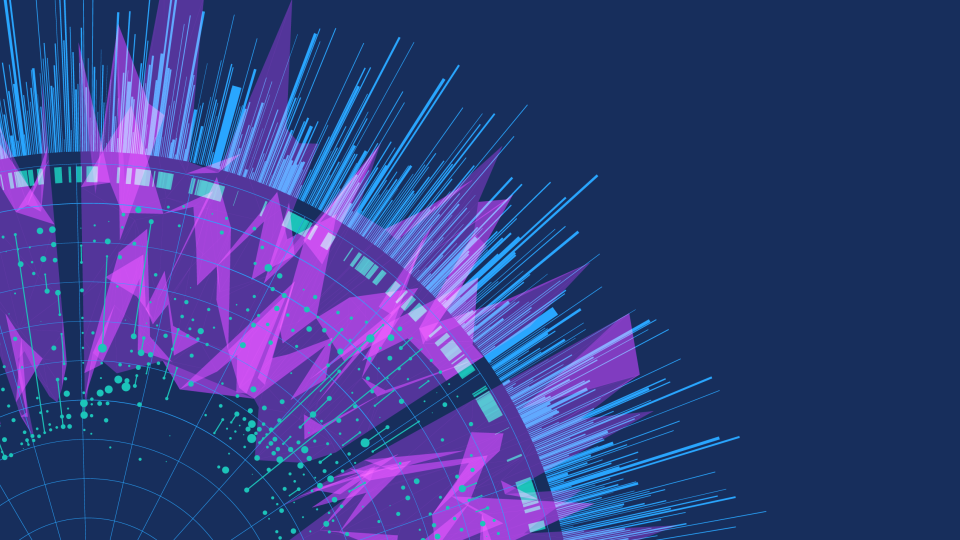
Coming Soon: 2024 AI Index Report!
The 2024 AI Index Report will be out April 15! Sign up for our mailing list to receive it in your inbox.
Steering Committee Co-Directors
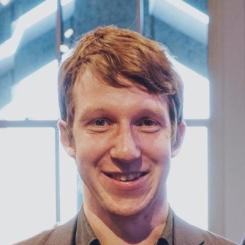
Ray Perrault
Steering committee members.
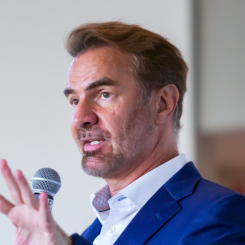
Erik Brynjolfsson
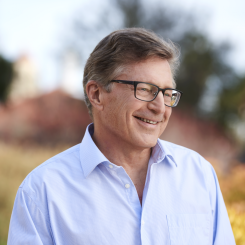
John Etchemendy
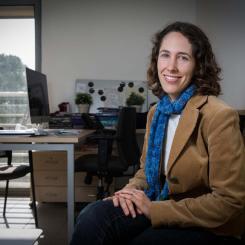
Katrina Ligett
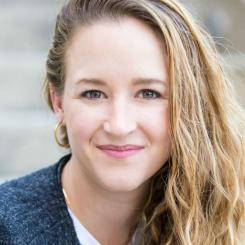
Terah Lyons
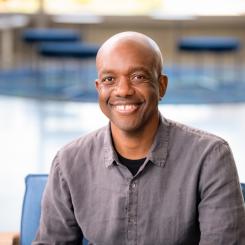
James Manyika
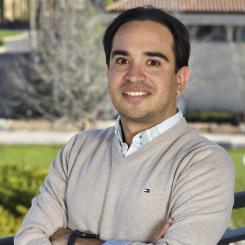
Juan Carlos Niebles
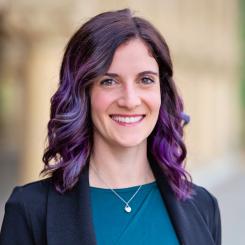
Vanessa Parli
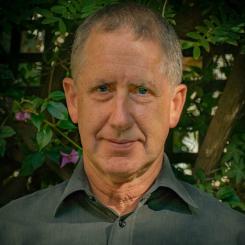
Yoav Shoham
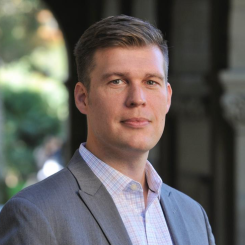
Russell Wald
Staff members.
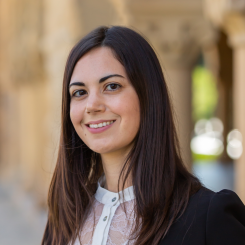
Loredana Fattorini
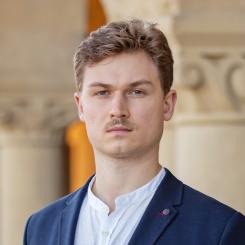
Nestor Maslej
Letter from the co-directors.
AI has moved into its era of deployment; throughout 2022 and the beginning of 2023, new large-scale AI models have been released every month. These models, such as ChatGPT, Stable Diffusion, Whisper, and DALL-E 2, are capable of an increasingly broad range of tasks, from text manipulation and analysis, to image generation, to unprecedentedly good speech recognition. These systems demonstrate capabilities in question answering, and the generation of text, image, and code unimagined a decade ago, and they outperform the state of the art on many benchmarks, old and new. However, they are prone to hallucination, routinely biased, and can be tricked into serving nefarious aims, highlighting the complicated ethical challenges associated with their deployment.
Although 2022 was the first year in a decade where private AI investment decreased, AI is still a topic of great interest to policymakers, industry leaders, researchers, and the public. Policymakers are talking about AI more than ever before. Industry leaders that have integrated AI into their businesses are seeing tangible cost and revenue benefits. The number of AI publications and collaborations continues to increase. And the public is forming sharper opinions about AI and which elements they like or dislike.
AI will continue to improve and, as such, become a greater part of all our lives. Given the increased presence of this technology and its potential for massive disruption, we should all begin thinking more critically about how exactly we want AI to be developed and deployed. We should also ask questions about who is deploying it—as our analysis shows, AI is increasingly defined by the actions of a small set of private sector actors, rather than a broader range of societal actors. This year’s AI Index paints a picture of where we are so far with AI, in order to highlight what might await us in the future.
- Jack Clark and Ray Perrault
Our Supporting Partners
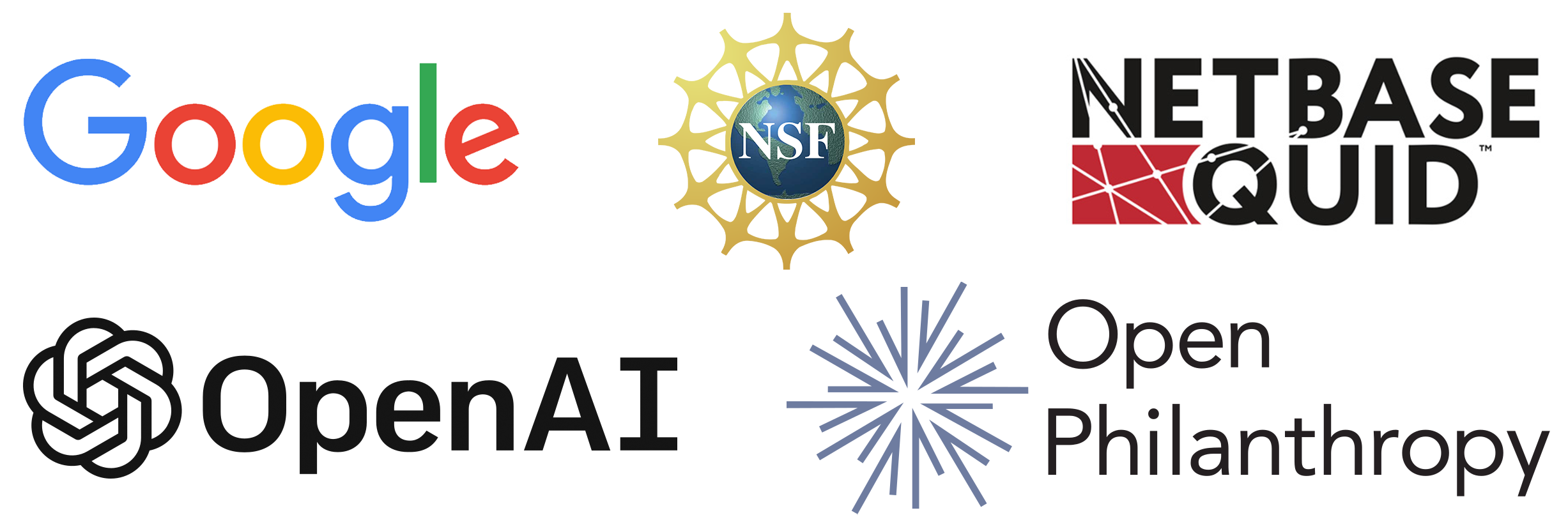
Analytics & Research Partners

Stay up to date on the AI Index by subscribing to the Stanford HAI newsletter.
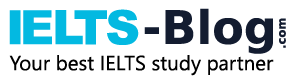
- Writing Correction
- Online Prep Platform
- Online Course
- Speaking Assessment
- Ace The IELTS
- Target Band 7
- Practice Tests Downloads
- IELTS Success Formula
- Essays Band 9 IELTS Writing Task 2 samples – IELTS Band 9 essays
- Essays Band 8 IELTS Writing – samples of IELTS essays of Band 8
- Essays Band 7 IELTS Writing – samples of IELTS essays of Band 7
- Essays Band 6 IELTS Writing – samples of IELTS essays of Band 6
- Essays Band 5 IELTS Writing – samples of IELTS essays of Band 5
- Reports Band 9 IELTS Writing – samples of IELTS reports of Band 9 (Academic Writing Task 1)
- Reports Band 8 IELTS Writing – samples of IELTS reports of Band 8
- Reports Band 7 IELTS Writing – samples of IELTS reports of Band 7
- Letters Band 9 IELTS Writing Task 1 – samples of IELTS letters of Band 9
- Letters Band 8 IELTS Writing – samples of IELTS letters of Band 8
- Letters Band 7 IELTS Writing – samples of IELTS letters of Band 7
- Speaking Samples
- Tests Samples
- 2023, 2024 IELTS questions
- 2022 IELTS questions
- 2021 IELTS questions
- 2020 IELTS questions
- High Scorer’s Advice IELTS high achievers share their secrets
- IELTS Results Competition
- IELTS-Blog App
IELTS essay, topic: Artificial Intelligence will take over the role of teachers (agree/disagree)
- IELTS Essays - Band 9
This is a model response to a Writing Task 2 topic from High Scorer’s Choice IELTS Practice Tests book series (reprinted with permission). This answer is close to IELTS Band 9.
Set 6 Academic book, Practice Test 26
Writing Task 2
You should spend about 40 minutes on this task.
Write about the following topic:
Some people feel that with the rise of artificial intelligence, computers and robots will take over the roles of teachers. To what extent do you agree or disagree with this statement?
Give reasons for your answer and include any relevant examples from your knowledge or experience.
You should write at least 250 words.
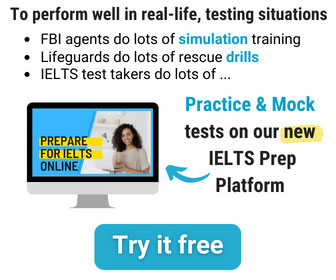
Sample Band 9 Essay
With ever increasing technological advances, computers and robots are replacing human roles in different areas of society. This trend can also be seen in education, where interactive programs can enhance the educational experience for children and young adults. Whether, however, this revolution can also take over the role of the teacher completely is debatable, and I oppose this idea as it is unlikely to serve students well.
The roles of computers and robots can be seen in many areas of the workplace. Classic examples are car factories, where a lot of the repetitive precision jobs done on assembly lines have been performed by robots for many years, and medicine, where diagnosis, and treatment, including operations, have also been assisted by computers for a long time. According to the media, it won’t also be long until we have cars that are driven automatically.
It has long been discussed whether robots and computers can do this in education. It is well known that the complexity of programs can now adapt to so many situations that something can already be set up that has the required knowledge of the teacher, along with the ability to predict and answer all questions that might be asked by students. In fact, due to the nature of computers, the knowledge levels can far exceed a teacher’s and have more breadth, as a computer can have equal knowledge in all the subjects that are taught in school, as opposed to a single teacher’s specialisation. It seems very likely, therefore, that computers and robots should be able to deliver the lessons that teachers can, including various ways of differentiating and presenting materials to suit varying abilities and ages of students.
Where I am not convinced is in the pastoral role of teachers. Part of teaching is managing behaviour and showing empathy with students, so that they feel cared for and important. Even if a robot or computer can be programmed to imitate these actions, students will likely respond in a different way when they know an interaction is part of an algorithm rather than based on human emotion.
Therefore, although I feel that computers should be able to perform a lot of the roles of teachers in the future, they should be used as educational tools to assist teachers and not to replace them. In this way, students would receive the benefits of both ways of instruction.
Go here for more IELTS Band 9 Essays
Related posts:
- IELTS essay, topic: Celebrities can be poor role models for teenagers (agree/disagree) This essay topic was seen in a recent IELTS test...
- IELTS essay, topic: Having a salaried job is better than being self-employed (agree/disagree) This is a model response to a Writing Task 2...
- IELTS essay, topic: Individuals should be responsible for funding their own retirement (agree/disagree) This is a model response to a Writing Task 2...
- IELTS essay, topic: Only people over 18 years old should be allowed to use social media (agree/disagree) This is a model response to a Writing Task 2...
- IELTS essay, topic: Women, not men, should stay at home to care for children (agree/disagree) This is a model response to a Writing Task 2...
Leave a Reply
Your email address will not be published. Required fields are marked *
Save my name, email, and website in this browser for the next time I comment.

How Tech Giants Cut Corners to Harvest Data for A.I.
OpenAI, Google and Meta ignored corporate policies, altered their own rules and discussed skirting copyright law as they sought online information to train their newest artificial intelligence systems.
Researchers at OpenAI’s office in San Francisco developed a tool to transcribe YouTube videos to amass conversational text for A.I. development. Credit... Jason Henry for The New York Times
Supported by
- Share full article

By Cade Metz , Cecilia Kang , Sheera Frenkel , Stuart A. Thompson and Nico Grant
Reporting from San Francisco, Washington and New York
- Published April 6, 2024 Updated April 8, 2024
In late 2021, OpenAI faced a supply problem.
The artificial intelligence lab had exhausted every reservoir of reputable English-language text on the internet as it developed its latest A.I. system. It needed more data to train the next version of its technology — lots more.
Listen to this article with reporter commentary
Open this article in the New York Times Audio app on iOS.
So OpenAI researchers created a speech recognition tool called Whisper. It could transcribe the audio from YouTube videos, yielding new conversational text that would make an A.I. system smarter.
Some OpenAI employees discussed how such a move might go against YouTube’s rules, three people with knowledge of the conversations said. YouTube, which is owned by Google, prohibits use of its videos for applications that are “independent” of the video platform.
Ultimately, an OpenAI team transcribed more than one million hours of YouTube videos, the people said. The team included Greg Brockman, OpenAI’s president, who personally helped collect the videos, two of the people said. The texts were then fed into a system called GPT-4 , which was widely considered one of the world’s most powerful A.I. models and was the basis of the latest version of the ChatGPT chatbot.
The race to lead A.I. has become a desperate hunt for the digital data needed to advance the technology. To obtain that data, tech companies including OpenAI, Google and Meta have cut corners, ignored corporate policies and debated bending the law, according to an examination by The New York Times.
At Meta, which owns Facebook and Instagram, managers, lawyers and engineers last year discussed buying the publishing house Simon & Schuster to procure long works, according to recordings of internal meetings obtained by The Times. They also conferred on gathering copyrighted data from across the internet, even if that meant facing lawsuits. Negotiating licenses with publishers, artists, musicians and the news industry would take too long, they said.
Like OpenAI, Google transcribed YouTube videos to harvest text for its A.I. models, five people with knowledge of the company’s practices said. That potentially violated the copyrights to the videos, which belong to their creators.
Last year, Google also broadened its terms of service. One motivation for the change, according to members of the company’s privacy team and an internal message viewed by The Times, was to allow Google to be able to tap publicly available Google Docs, restaurant reviews on Google Maps and other online material for more of its A.I. products.
The companies’ actions illustrate how online information — news stories, fictional works, message board posts, Wikipedia articles, computer programs, photos, podcasts and movie clips — has increasingly become the lifeblood of the booming A.I. industry. Creating innovative systems depends on having enough data to teach the technologies to instantly produce text, images, sounds and videos that resemble what a human creates.
The volume of data is crucial. Leading chatbot systems have learned from pools of digital text spanning as many as three trillion words, or roughly twice the number of words stored in Oxford University’s Bodleian Library, which has collected manuscripts since 1602. The most prized data, A.I. researchers said, is high-quality information, such as published books and articles, which have been carefully written and edited by professionals.
For years, the internet — with sites like Wikipedia and Reddit — was a seemingly endless source of data. But as A.I. advanced, tech companies sought more repositories. Google and Meta, which have billions of users who produce search queries and social media posts every day, were largely limited by privacy laws and their own policies from drawing on much of that content for A.I.
Their situation is urgent. Tech companies could run through the high-quality data on the internet as soon as 2026, according to Epoch, a research institute. The companies are using the data faster than it is being produced.
“The only practical way for these tools to exist is if they can be trained on massive amounts of data without having to license that data,” Sy Damle, a lawyer who represents Andreessen Horowitz, a Silicon Valley venture capital firm, said of A.I. models last year in a public discussion about copyright law. “The data needed is so massive that even collective licensing really can’t work.”
Tech companies are so hungry for new data that some are developing “synthetic” information. This is not organic data created by humans, but text, images and code that A.I. models produce — in other words, the systems learn from what they themselves generate.
OpenAI said each of its A.I. models “has a unique data set that we curate to help their understanding of the world and remain globally competitive in research.” Google said that its A.I. models “are trained on some YouTube content,” which was allowed under agreements with YouTube creators, and that the company did not use data from office apps outside of an experimental program. Meta said it had “made aggressive investments” to integrate A.I. into its services and had billions of publicly shared images and videos from Instagram and Facebook for training its models.
For creators, the growing use of their works by A.I. companies has prompted lawsuits over copyright and licensing. The Times sued OpenAI and Microsoft last year for using copyrighted news articles without permission to train A.I. chatbots. OpenAI and Microsoft have said using the articles was “fair use,” or allowed under copyright law, because they transformed the works for a different purpose.
More than 10,000 trade groups, authors, companies and others submitted comments last year about the use of creative works by A.I. models to the Copyright Office , a federal agency that is preparing guidance on how copyright law applies in the A.I. era.
Justine Bateman, a filmmaker, former actress and author of two books, told the Copyright Office that A.I. models were taking content — including her writing and films — without permission or payment.
“This is the largest theft in the United States, period,” she said in an interview.
‘Scale Is All You Need’

In January 2020, Jared Kaplan, a theoretical physicist at Johns Hopkins University, published a groundbreaking paper on A.I. that stoked the appetite for online data.
His conclusion was unequivocal: The more data there was to train a large language model — the technology that drives online chatbots — the better it would perform. Just as a student learns more by reading more books, large language models can better pinpoint patterns in text and be more accurate with more information.
“Everyone was very surprised that these trends — these scaling laws as we call them — were basically as precise as what you see in astronomy or physics,” said Dr. Kaplan, who published the paper with nine OpenAI researchers. (He now works at the A.I. start-up Anthropic.)
“Scale is all you need” soon became a rallying cry for A.I.
Researchers have long used large public databases of digital information to develop A.I., including Wikipedia and Common Crawl, a database of more than 250 billion web pages collected since 2007. Researchers often “cleaned” the data by removing hate speech and other unwanted text before using it to train A.I. models.
In 2020, data sets were tiny by today’s standards. One database containing 30,000 photographs from the photo website Flickr was considered a vital resource at the time.
After Dr. Kaplan’s paper, that amount of data was no longer enough. It became all about “just making things really big,” said Brandon Duderstadt, the chief executive of Nomic, an A.I. company in New York.
Before 2020, most A.I. models used relatively little training data.
Mr. Kaplan’s paper, released in 2020, led to a new era defined by GPT-3, a large language model, where researchers began including more data in their models …
… much, much more data.
When OpenAI unveiled GPT-3 in November 2020, it was trained on the largest amount of data to date — about 300 billion “tokens,” which are essentially words or pieces of words. After learning from that data, the system generated text with astounding accuracy, writing blog posts, poetry and its own computer programs.
In 2022, DeepMind, an A.I. lab owned by Google, went further. It tested 400 A.I. models and varied the amount of training data and other factors. The top-performing models used even more data than Dr. Kaplan had predicted in his paper. One model, Chinchilla, was trained on 1.4 trillion tokens.
It was soon overtaken. Last year, researchers from China released an A.I. model, Skywork , which was trained on 3.2 trillion tokens from English and Chinese texts. Google also unveiled an A.I. system, PaLM 2 , which topped 3.6 trillion tokens .
Transcribing YouTube
In May, Sam Altman , the chief executive of OpenAI, acknowledged that A.I. companies would use up all viable data on the internet.
“That will run out,” he said in a speech at a tech conference.
Mr. Altman had seen the phenomenon up close. At OpenAI, researchers had gathered data for years, cleaned it and fed it into a vast pool of text to train the company’s language models. They had mined the computer code repository GitHub, vacuumed up databases of chess moves and drawn on data describing high school tests and homework assignments from the website Quizlet.
By late 2021, those supplies were depleted, said eight people with knowledge of the company, who were not authorized to speak publicly.
OpenAI was desperate for more data to develop its next-generation A.I. model, GPT-4. So employees discussed transcribing podcasts, audiobooks and YouTube videos, the people said. They talked about creating data from scratch with A.I. systems. They also considered buying start-ups that had collected large amounts of digital data.
OpenAI eventually made Whisper, the speech recognition tool, to transcribe YouTube videos and podcasts, six people said. But YouTube prohibits people from not only using its videos for “independent” applications, but also accessing its videos by “any automated means (such as robots, botnets or scrapers).”
OpenAI employees knew they were wading into a legal gray area, the people said, but believed that training A.I. with the videos was fair use. Mr. Brockman, OpenAI’s president, was listed in a research paper as a creator of Whisper. He personally helped gather YouTube videos and fed them into the technology, two people said.
Mr. Brockman referred requests for comment to OpenAI, which said it uses “numerous sources” of data.
Last year, OpenAI released GPT-4, which drew on the more than one million hours of YouTube videos that Whisper had transcribed. Mr. Brockman led the team that developed GPT-4.
Some Google employees were aware that OpenAI had harvested YouTube videos for data, two people with knowledge of the companies said. But they didn’t stop OpenAI because Google had also used transcripts of YouTube videos to train its A.I. models, the people said. That practice may have violated the copyrights of YouTube creators. So if Google made a fuss about OpenAI, there might be a public outcry against its own methods, the people said.
Matt Bryant, a Google spokesman, said the company had no knowledge of OpenAI’s practices and prohibited “unauthorized scraping or downloading of YouTube content.” Google takes action when it has a clear legal or technical basis to do so, he said.
Google’s rules allowed it to tap YouTube user data to develop new features for the video platform. But it was unclear whether Google could use YouTube data to build a commercial service beyond the video platform, such as a chatbot.
Geoffrey Lottenberg, an intellectual property lawyer with the law firm Berger Singerman, said Google’s language about what it could and could not do with YouTube video transcripts was vague.
“Whether the data could be used for a new commercial service is open to interpretation and could be litigated,” he said.
In late 2022, after OpenAI released ChatGPT and set off an industrywide race to catch up , Google researchers and engineers discussed tapping other user data. Billions of words sat in people’s Google Docs and other free Google apps. But the company’s privacy restrictions limited how they could use the data, three people with knowledge of Google’s practices said.
In June, Google’s legal department asked the privacy team to draft language to broaden what the company could use consumer data for, according to two members of the privacy team and an internal message viewed by The Times.
The employees were told Google wanted to use people’s publicly available content in Google Docs, Google Sheets and related apps for an array of A.I. products. The employees said they didn’t know if the company had previously trained A.I. on such data.
At the time, Google’s privacy policy said the company could use publicly available information only to “help train Google’s language models and build features like Google Translate.”
The privacy team wrote new terms so Google could tap the data for its “A.I. models and build products and features like Google Translate, Bard and Cloud AI capabilities,” which was a wider collection of A.I. technologies.
“What is the end goal here?” one member of the privacy team asked in an internal message. “How broad are we going?”
The team was told specifically to release the new terms on the Fourth of July weekend, when people were typically focused on the holiday, the employees said. The revised policy debuted on July 1, at the start of the long weekend.
How Google Can Use Your Data
Here are the changes Google made to its privacy policy last year for its free consumer apps.

Google uses information to improve our services and to develop new products, features and technologies that benefit our users and the public. For example, we use publicly available information to help train Google’s language AI models and build products and features like Google Translate , Bard, and Cloud AI capabilities .

In August, two privacy team members said, they pressed managers on whether Google could start using data from free consumer versions of Google Docs, Google Sheets and Google Slides. They were not given clear answers, they said.
Mr. Bryant said that the privacy policy changes had been made for clarity and that Google did not use information from Google Docs or related apps to train language models “without explicit permission” from users, referring to a voluntary program that allows users to test experimental features.
“We did not start training on additional types of data based on this language change,” he said.
The Debate at Meta
Mark Zuckerberg, Meta’s chief executive, had invested in A.I. for years — but suddenly found himself behind when OpenAI released ChatGPT in 2022. He immediately pushed to match and exceed ChatGPT , calling executives and engineers at all hours of the night to push them to develop a rival chatbot, said three current and former employees, who were not authorized to discuss confidential conversations.
But by early last year, Meta had hit the same hurdle as its rivals: not enough data.
Ahmad Al-Dahle, Meta’s vice president of generative A.I., told executives that his team had used almost every available English-language book, essay, poem and news article on the internet to develop a model, according to recordings of internal meetings, which were shared by an employee.
Meta could not match ChatGPT unless it got more data, Mr. Al-Dahle told colleagues. In March and April 2023, some of the company’s business development leaders, engineers and lawyers met nearly daily to tackle the problem.
Some debated paying $10 a book for the full licensing rights to new titles. They discussed buying Simon & Schuster, which publishes authors like Stephen King, according to the recordings.
They also talked about how they had summarized books, essays and other works from the internet without permission and discussed sucking up more, even if that meant facing lawsuits. One lawyer warned of “ethical” concerns around taking intellectual property from artists but was met with silence, according to the recordings.
Mr. Zuckerberg demanded a solution, employees said.
“The capability that Mark is looking for in the product is just something that we currently aren’t able to deliver,” one engineer said.
While Meta operates giant social networks, it didn’t have troves of user posts at its disposal, two employees said. Many Facebook users had deleted their earlier posts, and the platform wasn’t where people wrote essay-type content, they said.
Meta was also limited by privacy changes it introduced after a 2018 scandal over sharing its users’ data with Cambridge Analytica, a voter-profiling company.
Mr. Zuckerberg said in a recent investor call that the billions of publicly shared videos and photos on Facebook and Instagram are “greater than the Common Crawl data set.”
During their recorded discussions, Meta executives talked about how they had hired contractors in Africa to aggregate summaries of fiction and nonfiction. The summaries included copyrighted content “because we have no way of not collecting that,” a manager said in one meeting.
Meta’s executives said OpenAI seemed to have used copyrighted material without permission. It would take Meta too long to negotiate licenses with publishers, artists, musicians and the news industry, they said, according to the recordings.
“The only thing that’s holding us back from being as good as ChatGPT is literally just data volume,” Nick Grudin, a vice president of global partnership and content, said in one meeting.
OpenAI appeared to be taking copyrighted material and Meta could follow this “market precedent,” he added.
Meta’s executives agreed to lean on a 2015 court decision involving the Authors Guild versus Google , according to the recordings. In that case, Google was permitted to scan, digitize and catalog books in an online database after arguing that it had reproduced only snippets of the works online and had transformed the originals, which made it fair use.
Using data to train A.I. systems, Meta’s lawyers said in their meetings, should similarly be fair use.
At least two employees raised concerns about using intellectual property and not paying authors and other artists fairly or at all, according to the recordings. One employee recounted a separate discussion about copyrighted data with senior executives including Chris Cox, Meta’s chief product officer, and said no one in that meeting considered the ethics of using people’s creative works.
‘Synthetic’ Data
OpenAI’s Mr. Altman had a plan to deal with the looming data shortage.
Companies like his, he said at the May conference, would eventually train their A.I. on text generated by A.I. — otherwise known as synthetic data.
Since an A.I. model can produce humanlike text, Mr. Altman and others have argued, the systems can create additional data to develop better versions of themselves. This would help developers build increasingly powerful technology and reduce their dependence on copyrighted data.
“As long as you can get over the synthetic data event horizon, where the model is smart enough to make good synthetic data, everything will be fine,” Mr. Altman said.
A.I. researchers have explored synthetic data for years. But building an A.I system that can train itself is easier said than done. A.I. models that learn from their own outputs can get caught in a loop where they reinforce their own quirks, mistakes and limitations.
“The data these systems need is like a path through the jungle,” said Jeff Clune, a former OpenAI researcher who now teaches computer science at the University of British Columbia. “If they only train on synthetic data, they can get lost in the jungle.”
To combat this, OpenAI and others are investigating how two different A.I. models might work together to generate synthetic data that is more useful and reliable. One system produces the data, while a second judges the information to separate the good from the bad. Researchers are divided on whether this method will work.
A.I. executives are barreling ahead nonetheless.
“It should be all right,” Mr. Altman said at the conference.
Read by Cade Metz
Audio produced by Patricia Sulbarán .
An earlier version of this article misstated the publisher of J.K. Rowling’s books. Her works have been published by Scholastic, Little, Brown and others. They were not published by Simon & Schuster.
How we handle corrections
Cade Metz writes about artificial intelligence, driverless cars, robotics, virtual reality and other emerging areas of technology. More about Cade Metz
Cecilia Kang reports on technology and regulatory policy and is based in Washington D.C. She has written about technology for over two decades. More about Cecilia Kang
Sheera Frenkel is a reporter based in the San Francisco Bay Area, covering the ways technology impacts everyday lives with a focus on social media companies, including Facebook, Instagram, Twitter, TikTok, YouTube, Telegram and WhatsApp. More about Sheera Frenkel
Stuart A. Thompson writes about how false and misleading information spreads online and how it affects people around the world. He focuses on misinformation, disinformation and other misleading content. More about Stuart A. Thompson
Nico Grant is a technology reporter covering Google from San Francisco. Previously, he spent five years at Bloomberg News, where he focused on Google and cloud computing. More about Nico Grant
Explore Our Coverage of Artificial Intelligence
News and Analysis
U.S. clinics are starting to offer patients a new service: having their mammograms read not just by a radiologist, but also by an A.I. model .
OpenAI unveiled Voice Engine , an A.I. technology that can recreate a person’s voice from a 15-second recording.
Amazon said it had added $2.75 billion to its investment in Anthropic , an A.I. start-up that competes with companies like OpenAI and Google.
The Age of A.I.
Teen girls are confronting an epidemic of deepfake nudes in schools across the United States, as middle and high school students have used A.I. to fabricate explicit images of female classmates.
A.I. is peering into restaurant garbage pails and crunching grocery-store data to try to figure out how to send less uneaten food into dumpsters.
David Autor, an M.I.T. economist and tech skeptic, argues that A.I. is fundamentally different from past waves of computerization.
Economists doubt that A.I. is already visible in productivity data . Big companies, however, talk often about adopting it to improve efficiency.
The Caribbean island Anguilla made $32 million last year, more than 10& of its G.D.P., from companies registering web addresses that end in .ai .
When it comes to the A.I. that powers chatbots, China trails the United States. But when it comes to producing the scientists behind a new generation of humanoid technologies, China is pulling ahead .
Advertisement

IMAGES
VIDEO
COMMENTS
Human intelligence lies in the basis of such developments and represents the collective knowledge gained from the analysis of experiences people live through. In turn, AI is an outcome of this progression, which allows humanity to put this data in a digital form that possesses some autonomous qualities. As a result, AI also contains limitations ...
July 18, 2023. Artificial general intelligence (AGI) is difficult to precisely define but refers to a superintelligent AI recognizable from science fiction. AGI may still be far off, but the ...
In an economy where data is changing how companies create value — and compete — experts predict that using artificial intelligence (AI) at a larger scale will add as much as $15.7 trillion to ...
In other words, it's the point where AI can tackle any intellectual task a human can. AGI isn't here yet; current AI models are held back by a lack of certain human traits such as true ...
Some 979 technology pioneers, innovators, developers, business and policy leaders, researchers and activists answered this question in a canvassing of experts conducted in the summer of 2018. The experts predicted networked artificial intelligence will amplify human effectiveness but also threaten human autonomy, agency and capabilities.
When thinking about the future of artificial intelligence, I find it helpful to consider two different concepts in particular: human-level AI, and transformative AI. 2 The first concept highlights the AI's capabilities and anchors them to a familiar benchmark, while transformative AI emphasizes the impact that this technology would have on ...
The 2021 report is the second in a series that will be released every five years until 2116. Titled "Gathering Strength, Gathering Storms," the report explores the various ways AI is increasingly touching people's lives in settings that range from movie recommendations and voice assistants to autonomous driving and automated medical ...
Abstract: Today, artificial intelligence (AI) is capable of learning from its experience through the element of its Machine Learning (ML) in conjunction with Deep Learning (DL) component and using ...
Artificial Intelligence or AI is a simulation of human intelligence applied to a computer system or other machine device so that the device has a way of thinking like humans ( J. E. Korteling et ...
AI is one of the most debated subjects of today and there seems little common understanding concerning the differences and similarities of human intelligence and artificial intelligence. Discussions on many relevant topics, such as trustworthiness, explainability, and ethics are characterized by implicit anthropocentric and anthropomorphistic conceptions and, for instance, the pursuit of human ...
Essence. The purpose of human intelligence is to combine a range of cognitive activities in order to adapt to new circumstances. The goal of artificial intelligence (AI) is to create computers that are able to behave like humans and complete jobs that humans would normally do. Functionality. People make use of the memory, processing ...
Like many people, the experts seemed to have been surprised by the rapid AI progress of the last year and have updated their forecasts accordingly—when AI Impacts ran the same survey in 2022 ...
Humanity would cease to exist, predicted the essay's author, with the emergence of superintelligence, or AI, that surpasses human-level intelligence in a broad array of areas. Tallinn, an ...
Bioethics is not a matter of calculation but a process of conscientization. Although AI designers can up-load all information, data, and programmed to AI to function as a human being, it is still a machine and a tool. AI will always remain as AI without having authentic human feelings and the capacity to commiserate.
More From Artificial Intelligence. ... it could help them write college essays and do homework. ... This is what happens to a technology that's taking over. This is a measure of its power.
Unraveling the Promise and Peril of Artificial Intelligence Comprehensive Argumentative Essay Paper on Artificial Intelligence. Artificial Intelligence (AI) stands as a hallmark of human innovation, promising to revolutionize industries, economies, and even the fabric of society itself. With its ability to mimic cognitive functions, AI has penetrated various spheres of human existence, from ...
Human intelligence is general, artificial intelligence narrow. ... This artifical intelligence robot probably isn't going to take over the world anytime soonImage: AP
In light of the recent spectacular developments in artificial intelligence (AI), questions are now being asked about whether AI could present a danger to humanity. ... Human brains contain about 10 11 neurons each, which probably is close to the limit how many neurons a single biological brain can contain. Though this is more than computer ...
The term "artificial intelligence," or AI, has become a buzzword in recent years. Optimists see AI as the panacea to society's most fundamental problems, from crime to corruption to inequality, while pessimists fear that AI will overtake human intelligence and crown itself king of the world. Underlying these two seemingly antithetical views is the assumption
Free Essay - Artificial intelligence (AI) and human intelligence Significant progress in AI has been achieved in recent years, especially with the development of machine learning and deep learning algorithms. By virtue of these developments, AI is now capable of activities formerly associated solely with human intellect, such as pattern recognition, natural language comprehension, and even ...
Stuart Russell is a distinguished professor of computer science at UC Berkeley and director of the Center for Human-Compatible Artificial Intelligence. Stuart Russell: Intelligence gives you power over the world, and if you are more intelligent — all other things being equal — you're going to have more power. And so if we build AI systems ...
Based on our probabilistic risk analysis, our central estimate is that only around 20% of existing UK jobs may actually be displaced by AI and related technologies over the 20 years to 2037, rising to around 26% in China owing to the higher potential for automation there particularly in manufacturing and agriculture.
With AI taking over routine and analytical tasks, humans will find greater value in roles that require creativity, strategy, and emotional intelligence, fields where AI cannot easily tread.
Artificial Intelligence refers to the intelligence of machines. This is in contrast to the natural intelligence of humans and animals. With Artificial Intelligence, machines perform functions such as learning, planning, reasoning and problem-solving. Most noteworthy, Artificial Intelligence is the simulation of human intelligence by machines.
The AI Index Report tracks, collates, distills, and visualizes data related to artificial intelligence. Our mission is to provide unbiased, rigorously vetted, broadly sourced data in order for policymakers, researchers, executives, journalists, and the general public to develop a more thorough and nuanced understanding of the complex field of AI.
Artificial intelligence—the creation of software and hardware able to simulate human smarts—isn't new. Crucial core technologies for today's AI were first conceived in the 1970s and '80s ...
Sample Band 9 Essay. With ever increasing technological advances, computers and robots are replacing human roles in different areas of society. This trend can also be seen in education, where interactive programs can enhance the educational experience for children and young adults.
Artificial intelligence (AI) is increasingly reshaping service by performing various tasks, constituting a major source of innovation, yet threatening human jobs. We develop a theory of AI job ...
In late 2021, OpenAI faced a supply problem. The artificial intelligence lab had exhausted every reservoir of reputable English-language text on the internet as it developed its latest A.I. system.