| | | | | - Tips & Tricks
- Website & Apps
- ChatGPT Blogs
- ChatGPT News
- ChatGPT Tutorial
What is Speech Recognition?Speech recognition or speech-to-text recognition , is the capacity of a machine or program to recognize spoken words and transform them into text. Speech Recognition is an important feature in several applications used such as home automation, artificial intelligence, etc. In this article, we are going to explore how speech recognition software work, speech recognition algorithms, and the role of NLP. See examples of how this technology is used in everyday life and various industries, making interactions with devices smarter and more intuitive. Speech Recognition , also known as automatic speech recognition ( ASR ), computer speech recognition, or speech-to-text, focuses on enabling computers to understand and interpret human speech. Speech recognition involves converting spoken language into text or executing commands based on the recognized words. This technology relies on sophisticated algorithms and machine learning models to process and understand human speech in real-time , despite the variations in accents , pitch , speed , and slang . Key Features of Speech Recognition- Accuracy and Speed: They can process speech in real-time or near real-time, providing quick responses to user inputs.
- Natural Language Understanding (NLU): NLU enables systems to handle complex commands and queries , making technology more intuitive and user-friendly .
- Multi-Language Support: Support for multiple languages and dialects , allowing users from different linguistic backgrounds to interact with technology in their native language.
- Background Noise Handling: This feature is crucial for voice-activated systems used in public or outdoor settings.
Speech Recognition AlgorithmsSpeech recognition technology relies on complex algorithms to translate spoken language into text or commands that computers can understand and act upon. Here are the algorithms and approaches used in speech recognition: 1. Hidden Markov Models (HMM)Hidden Markov Models have been the backbone of speech recognition for many years. They model speech as a sequence of states, with each state representing a phoneme (basic unit of sound) or group of phonemes. HMMs are used to estimate the probability of a given sequence of sounds, making it possible to determine the most likely words spoken. Usage : Although newer methods have surpassed HMM in performance, it remains a fundamental concept in speech recognition, often used in combination with other techniques. 2. Natural language processing (NLP)NLP is the area of artificial intelligence which focuses on the interaction between humans and machines through language through speech and text. Many mobile devices incorporate speech recognition into their systems to conduct voice search. Example such as : Siri or provide more accessibility around texting. 3. Deep Neural Networks (DNN)DNNs have improved speech recognition’s accuracy a lot. These networks can learn hierarchical representations of data, making them particularly effective at modeling complex patterns like those found in human speech. DNNs are used both for acoustic modeling , to better understand the sound of speech , and for language modeling, to predict the likelihood of certain word sequences. 4. End-to-End Deep LearningNow, the trend has shifted towards end-to-end deep learning models , which can directly map speech inputs to text outputs without the need for intermediate phonetic representations. These models, often based on advanced RNNs , Transformers, or Attention Mechanisms , can learn more complex patterns and dependencies in the speech signal. What is Automatic Speech Recognition?Automatic Speech Recognition (ASR) is a technology that enables computers to understand and transcribe spoken language into text. It works by analyzing audio input, such as spoken words, and converting them into written text , typically in real-time. ASR systems use algorithms and machine learning techniques to recognize and interpret speech patterns , phonemes, and language models to accurately transcribe spoken words. This technology is widely used in various applications, including virtual assistants , voice-controlled devices , dictation software , customer service automation , and language translation services . What is Dragon speech recognition software?Dragon speech recognition software is a program developed by Nuance Communications that allows users to dictate text and control their computer using voice commands. It transcribes spoken words into written text in real-time , enabling hands-free operation of computers and devices. Dragon software is widely used for various purposes, including dictating documents , composing emails , navigating the web , and controlling applications . It also features advanced capabilities such as voice commands for editing and formatting text , as well as custom vocabulary and voice profiles for improved accuracy and personalization. What is a normal speech recognition threshold?The normal speech recognition threshold refers to the level of sound, typically measured in decibels (dB) , at which a person can accurately recognize speech. In quiet environments, this threshold is typically around 0 to 10 dB for individuals with normal hearing. However, in noisy environments or for individuals with hearing impairments , the threshold may be higher, meaning they require a louder volume to accurately recognize speech . Uses of Speech Recognition- Virtual Assistants: These are like digital helpers that understand what you say. They can do things like set reminders, search the internet, and control smart home devices, all without you having to touch anything. Examples include Siri , Alexa , and Google Assistant .
- Accessibility Tools: Speech recognition makes technology easier to use for people with disabilities. Features like voice control on phones and computers help them interact with devices more easily. There are also special apps for people with disabilities.
- Automotive Systems: In cars, you can use your voice to control things like navigation and music. This helps drivers stay focused and safe on the road. Examples include voice-activated navigation systems in cars.
- Healthcare: Doctors use speech recognition to quickly write down notes about patients, so they have more time to spend with them. There are also voice-controlled bots that help with patient care. For example, doctors use dictation tools to write down patient information quickly.
- Customer Service: Speech recognition is used to direct customer calls to the right place or provide automated help. This makes things run smoother and keeps customers happy. Examples include call centers that you can talk to and customer service bots .
- Education and E-Learning: Speech recognition helps people learn languages by giving them feedback on their pronunciation. It also transcribes lectures, making them easier to understand. Examples include language learning apps and lecture transcribing services.
- Security and Authentication: Voice recognition, combined with biometrics , keeps things secure by making sure it’s really you accessing your stuff. This is used in banking and for secure facilities. For example, some banks use your voice to make sure it’s really you logging in.
- Entertainment and Media: Voice recognition helps you find stuff to watch or listen to by just talking. This makes it easier to use things like TV and music services . There are also games you can play using just your voice.
Speech recognition is a powerful technology that lets computers understand and process human speech. It’s used everywhere, from asking your smartphone for directions to controlling your smart home devices with just your voice. This tech makes life easier by helping with tasks without needing to type or press buttons, making gadgets like virtual assistants more helpful. It’s also super important for making tech accessible to everyone, including those who might have a hard time using keyboards or screens. As we keep finding new ways to use speech recognition, it’s becoming a big part of our daily tech life, showing just how much we can do when we talk to our devices. What is Speech Recognition?- FAQsWhat are examples of speech recognition. Note Taking/Writing: An example of speech recognition technology in use is speech-to-text platforms such as Speechmatics or Google’s speech-to-text engine. In addition, many voice assistants offer speech-to-text translation. Is speech recognition secure?Security concerns related to speech recognition primarily involve the privacy and protection of audio data collected and processed by speech recognition systems. Ensuring secure data transmission, storage, and processing is essential to address these concerns. Is speech recognition and voice recognition same?No, speech recognition and voice recognition are different. Speech recognition converts spoken words into text using NLP, focusing on the content of speech. Voice recognition, however, identifies the speaker based on vocal characteristics, emphasizing security and personalization without interpreting the speech’s content. What is speech recognition in AI?Speech recognition is the process of converting sound signals to text transcriptions. Steps involved in conversion of a sound wave to text transcription in a speech recognition system are: Recording: Audio is recorded using a voice recorder. Sampling: Continuous audio wave is converted to discrete values. What are the type of Speech Recognition?Dictation Systems: Convert speech to text. Voice Command Systems: Execute spoken commands. Speaker-Dependent Systems: Trained for specific users. Speaker-Independent Systems: Work for any user. Continuous Speech Recognition: Allows natural, flowing speech. Discrete Speech Recognition: Requires pauses between words. NLP-Integrated Systems: Understand context and meaning How accurate is speech recognition technology?The accuracy of speech recognition technology can vary depending on factors such as the quality of audio input , language complexity , and the specific application or system being used. Advances in machine learning and deep learning have improved accuracy significantly in recent years. Please Login to comment...Similar reads. - Computer Networks
- Computer Subject
- tech-updates
- How to Delete Discord Servers: Step by Step Guide
- Google increases YouTube Premium price in India: Check our the latest plans
- California Lawmakers Pass Bill to Limit AI Replicas
- Best 10 IPTV Service Providers in Germany
- 15 Most Important Aptitude Topics For Placements [2024]
Improve your Coding Skills with Practice What kind of Experience do you want to share?- ABBREVIATIONS
- BIOGRAPHIES
- CALCULATORS
- CONVERSIONS
- DEFINITIONS
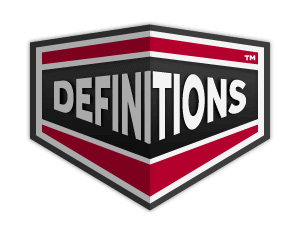 Vocabulary What does speech mean?Definitions for speech spitʃ speech, this dictionary definitions page includes all the possible meanings, example usage and translations of the word speech ., princeton's wordnet rate this definition: 3.8 / 4 votes. address, speech noun the act of delivering a formal spoken communication to an audience "he listened to an address on minor Roman poets" speech, speech communication, spoken communication, spoken language, language, voice communication, oral communication noun (language) communication by word of mouth "his speech was garbled"; "he uttered harsh language"; "he recorded the spoken language of the streets" something spoken "he could hear them uttering merry speeches" the exchange of spoken words "they were perfectly comfortable together without speech" manner of speaking, speech, delivery noun your characteristic style or manner of expressing yourself orally "his manner of speaking was quite abrupt"; "her speech was barren of southernisms"; "I detected a slight accent in his speech" lecture, speech, talking to noun a lengthy rebuke "a good lecture was my father's idea of discipline"; "the teacher gave him a talking to" actor's line, speech, words noun words making up the dialogue of a play "the actor forgot his speech" language, speech noun the mental faculty or power of vocal communication "language sets homo sapiens apart from all other animals" Wiktionary Rate this definition: 0.0 / 0 votesThe faculty of speech; the ability to speak or to use vocalizations to communicate. A session of speaking; a long oral message given publicly usually by one person. The candidate made some ambitious promises in his campaign speech. Etymology: From speche, from spæc, spræc, from sprēkijō, from spereg-. Cognate with spraak, Sprache, sprog. More at speak. Samuel Johnson's Dictionary Rate this definition: 0.0 / 0 votesSpeech noun Etymology: from speak. There is none comparable to the variety of instructive expressions by speech, wherewith a man alone is endowed, for the communication of his thoughts. William Holder , on Speech. Though our ideas are first acquired by various sensations and reflections, yet we convey them to each other by the means of certain sounds, or written marks, which we call words; and a great part of our knowledge is both obtained and communicated by these means, which are called speech. Isaac Watts. In speech be eight parts. Accidence. The acts of God to human ears Cannot without process of speech be told. John Milton. There is neither speech nor language, but their voices are heard among them. Ps. Common Prayer. A plague upon your epileptick visage! Smile you my speeches as I were a fool. William Shakespeare , K. Lear. The duke did of me demand What was the speech among the Londoners, Concerning the French journey. William Shakespeare. Speech of a man’s self ought to be seldom. Francis Bacon , Essays. The constant design of these orators, in all their speeches, was to drive some one particular point. Jonathan Swift. I, with leave of speech implor’d, reply’d. John Milton. Wikipedia Rate this definition: 0.0 / 0 votesSpeech is a human vocal communication using language. Each language uses phonetic combinations of vowel and consonant sounds that form the sound of its words (that is, all English words sound different from all French words, even if they are the same word, e.g., "role" or "hotel"), and using those words in their semantic character as words in the lexicon of a language according to the syntactic constraints that govern lexical words' function in a sentence. In speaking, speakers perform many different intentional speech acts, e.g., informing, declaring, asking, persuading, directing, and can use enunciation, intonation, degrees of loudness, tempo, and other non-representational or paralinguistic aspects of vocalization to convey meaning. In their speech, speakers also unintentionally communicate many aspects of their social position such as sex, age, place of origin (through accent), physical states (alertness and sleepiness, vigor or weakness, health or illness), psychological states (emotions or moods), physico-psychological states (sobriety or drunkenness, normal consciousness and trance states), education or experience, and the like. Although people ordinarily use speech in dealing with other persons (or animals), when people swear they do not always mean to communicate anything to anyone, and sometimes in expressing urgent emotions or desires they use speech as a quasi-magical cause, as when they encourage a player in a game to do or warn them not to do something. There are also many situations in which people engage in solitary speech. People talk to themselves sometimes in acts that are a development of what some psychologists (e.g., Lev Vygotsky) have maintained is the use of silent speech in an interior monologue to vivify and organize cognition, sometimes in the momentary adoption of a dual persona as self addressing self as though addressing another person. Solo speech can be used to memorize or to test one's memorization of things, and in prayer or in meditation (e.g., the use of a mantra). Researchers study many different aspects of speech: speech production and speech perception of the sounds used in a language, speech repetition, speech errors, the ability to map heard spoken words onto the vocalizations needed to recreate them, which plays a key role in children's enlargement of their vocabulary, and what different areas of the human brain, such as Broca's area and Wernicke's area, underlie speech. Speech is the subject of study for linguistics, cognitive science, communication studies, psychology, computer science, speech pathology, otolaryngology, and acoustics. Speech compares with written language, which may differ in its vocabulary, syntax, and phonetics from the spoken language, a situation called diglossia. The evolutionary origins of speech are unknown and subject to much debate and speculation. While animals also communicate using vocalizations, and trained apes such as Washoe and Kanzi can use simple sign language, no animals' vocalizations are articulated phonemically and syntactically, and do not constitute speech. ChatGPT Rate this definition: 0.0 / 0 votesSpeech is a form of human communication using spoken language. It involves the vocal production of sounds, usually in a structured and conventional way, to convey a particular meaning or message. This process includes articulation, voice production, fluency, and language skills involving vocabulary, grammar, and syntax. Webster Dictionary Rate this definition: 3.0 / 1 votethe faculty of uttering articulate sounds or words; the faculty of expressing thoughts by words or articulate sounds; the power of speaking he act of speaking; that which is spoken; words, as expressing ideas; language; conversation a particular language, as distinct from others; a tongue; a dialect talk; mention; common saying formal discourse in public; oration; harangue ny declaration of thoughts to make a speech; to harangue Wikidata Rate this definition: 5.0 / 1 voteSpeech is the vocalized form of human communication. It is based upon the syntactic combination of lexicals and names that are drawn from very large vocabularies. Each spoken word is created out of the phonetic combination of a limited set of vowel and consonant speech sound units. These vocabularies, the syntax which structures them, and their set of speech sound units differ, creating the existence of many thousands of different types of mutually unintelligible human languages. Most human speakers are able to communicate in two or more of them. The vocal abilities that enable humans to produce speech also provide humans with the ability to sing. A gestural form of human communication exists for the deaf in the form of sign language. Speech in some cultures has become the basis of a written language, often one that differs in its vocabulary, syntax and phonetics from its associated spoken one, a situation called diglossia. Speech in addition to its use in communication, it is suggested by some psychologists such as Vygotsky is internally used by mental processes to enhance and organize cognition in the form of an interior monologue. Chambers 20th Century Dictionary Rate this definition: 5.0 / 1 votespēch, n. that which is spoken: language: the power of speaking: manner of speech, oration: any declaration of thoughts: mention: colloquy: conference.— ns. Speech′-craft , the science of language: the gift of speech; Speech′-crī′er , one who hawked the broadsides containing the dying speeches of persons executed, once common; Speech′-day , the public day at the close of a school year.— adj. Speech′ful , loquacious.— ns. Speechificā′tion , the act of making harangues; Speech′ifīer .— v.i. Speech′ify , to make speeches, harangue (implying contempt).— adj. Speech′less , destitute or deprived of the power of speech.— adv. Speech′lessly .— ns. Speech′lessness ; Speech′-māk′er , one accustomed to speak in public; Speech′-māk′ing , a formal speaking before an assembly; Speech′-read′ing , the art of following spoken words by observing the speaker's lips, as taught to deaf-mutes. [A.S. spǽc , sprǽc ; Ger. sprache .] U.S. National Library of Medicine Rate this definition: 0.0 / 0 votesCommunication through a system of conventional vocal symbols. Editors Contribution Rate this definition: 0.0 / 0 votesThe faculty or act of speaking. His speech and language developed at such a fast pace to his peers. Submitted by MaryC on January 12, 2020 To express how we feel using words and language. They decided a wedding speech was not necessary as they chose for everyone to come to the wedding and enjoy themselves, have fun, feel the love and unity and dance the night away. Submitted by MaryC on April 9, 2020 Suggested Resources Rate this definition: 0.0 / 0 votesSong lyrics by speech -- Explore a large variety of song lyrics performed by speech on the Lyrics.com website. Surnames Frequency by Census Records Rate this definition: 0.0 / 0 votesAccording to the U.S. Census Bureau, Speech is ranked #91625 in terms of the most common surnames in America. The Speech surname appeared 201 times in the 2010 census and if you were to sample 100,000 people in the United States, approximately 0 would have the surname Speech . 85.5% or 172 total occurrences were Black . 8.4% or 17 total occurrences were White . 3.9% or 8 total occurrences were of Hispanic origin. Matched Categories- Auditory Communication
- Expressive Style
British National CorpusSpoken Corpus Frequency Rank popularity for the word 'speech' in Spoken Corpus Frequency: #1308 Written Corpus Frequency Rank popularity for the word 'speech' in Written Corpus Frequency: #2059 Nouns Frequency Rank popularity for the word 'speech' in Nouns Frequency: #532 Usage in printed sources From: [["1507","1"],["1520","1"],["1524","3"],["1527","1"],["1563","18"],["1564","7"],["1568","2"],["1572","8"],["1575","1"],["1579","5"],["1581","6"],["1582","18"],["1587","20"],["1588","1"],["1590","16"],["1592","6"],["1593","3"],["1595","3"],["1598","9"],["1603","4"],["1605","1"],["1606","3"],["1607","13"],["1610","1"],["1611","3"],["1612","2"],["1619","2"],["1620","33"],["1621","5"],["1623","10"],["1624","3"],["1625","11"],["1626","1"],["1629","4"],["1630","9"],["1631","28"],["1635","23"],["1637","21"],["1638","4"],["1640","1"],["1641","6"],["1642","17"],["1643","10"],["1644","48"],["1645","14"],["1646","2"],["1647","12"],["1648","35"],["1649","4"],["1651","49"],["1652","12"],["1653","25"],["1655","5"],["1656","87"],["1657","13"],["1658","41"],["1659","13"],["1660","9"],["1661","13"],["1662","10"],["1663","4"],["1664","1"],["1665","3"],["1666","2"],["1667","29"],["1668","61"],["1669","25"],["1670","39"],["1671","9"],["1672","7"],["1673","25"],["1674","6"],["1675","35"],["1676","209"],["1677","26"],["1678","74"],["1679","23"],["1680","49"],["1681","49"],["1682","89"],["1683","56"],["1684","18"],["1685","182"],["1686","23"],["1687","8"],["1688","28"],["1689","8"],["1690","12"],["1692","9"],["1693","23"],["1694","16"],["1695","1"],["1696","1"],["1697","2"],["1698","34"],["1699","30"],["1700","26"],["1701","39"],["1702","12"],["1703","43"],["1704","19"],["1705","16"],["1706","55"],["1707","34"],["1708","30"],["1709","12"],["1710","28"],["1711","8"],["1712","40"],["1713","25"],["1714","36"],["1715","17"],["1716","162"],["1717","62"],["1718","33"],["1719","93"],["1720","75"],["1721","102"],["1722","66"],["1723","530"],["1724","283"],["1725","115"],["1726","85"],["1727","137"],["1728","101"],["1729","99"],["1730","177"],["1731","88"],["1732","113"],["1733","112"],["1734","74"],["1735","48"],["1736","79"],["1737","200"],["1738","164"],["1739","74"],["1740","117"],["1741","405"],["1742","105"],["1743","195"],["1744","487"],["1745","108"],["1746","204"],["1747","824"],["1748","389"],["1749","273"],["1750","521"],["1751","515"],["1752","392"],["1753","789"],["1754","923"],["1755","876"],["1756","325"],["1757","350"],["1758","1104"],["1759","2351"],["1760","829"],["1761","835"],["1762","749"],["1763","1892"],["1764","951"],["1765","1026"],["1766","1364"],["1767","686"],["1768","1207"],["1769","732"],["1770","1125"],["1771","690"],["1772","665"],["1773","975"],["1774","1031"],["1775","1947"],["1776","1361"],["1777","773"],["1778","1031"],["1779","1296"],["1780","922"],["1781","864"],["1782","1238"],["1783","975"],["1784","1292"],["1785","1663"],["1786","1454"],["1787","1865"],["1788","2378"],["1789","1994"],["1790","1990"],["1791","1921"],["1792","2654"],["1793","2182"],["1794","2105"],["1795","2712"],["1796","3632"],["1797","2399"],["1798","2991"],["1799","2776"],["1800","4236"],["1801","6942"],["1802","7040"],["1803","5630"],["1804","6226"],["1805","8374"],["1806","7128"],["1807","8506"],["1808","7781"],["1809","8308"],["1810","11118"],["1811","11044"],["1812","10550"],["1813","7575"],["1814","9499"],["1815","10554"],["1816","12234"],["1817","11821"],["1818","11808"],["1819","10323"],["1820","15192"],["1821","11140"],["1822","17057"],["1823","16792"],["1824","17983"],["1825","20132"],["1826","14512"],["1827","14892"],["1828","16539"],["1829","16130"],["1830","18320"],["1831","16718"],["1832","16891"],["1833","20059"],["1834","15155"],["1835","19706"],["1836","26533"],["1837","18234"],["1838","23694"],["1839","23594"],["1840","26089"],["1841","26757"],["1842","22580"],["1843","24696"],["1844","28065"],["1845","27506"],["1846","32479"],["1847","27321"],["1848","30731"],["1849","30821"],["1850","30968"],["1851","34626"],["1852","33608"],["1853","50791"],["1854","49724"],["1855","39645"],["1856","44171"],["1857","38634"],["1858","36877"],["1859","37512"],["1860","48911"],["1861","31764"],["1862","27251"],["1863","29193"],["1864","35193"],["1865","36147"],["1866","40542"],["1867","38848"],["1868","40389"],["1869","39496"],["1870","39794"],["1871","37099"],["1872","39856"],["1873","35715"],["1874","47704"],["1875","54118"],["1876","55948"],["1877","51427"],["1878","45003"],["1879","50110"],["1880","68891"],["1881","65396"],["1882","62298"],["1883","78421"],["1884","67763"],["1885","67016"],["1886","54176"],["1887","61529"],["1888","60125"],["1889","60801"],["1890","63681"],["1891","65047"],["1892","82074"],["1893","71219"],["1894","70572"],["1895","71055"],["1896","80626"],["1897","73160"],["1898","86751"],["1899","108656"],["1900","111867"],["1901","96703"],["1902","98241"],["1903","96647"],["1904","100995"],["1905","98656"],["1906","105624"],["1907","104734"],["1908","109555"],["1909","94914"],["1910","98847"],["1911","97218"],["1912","100714"],["1913","98467"],["1914","88043"],["1915","81797"],["1916","88570"],["1917","86142"],["1918","73075"],["1919","83381"],["1920","102853"],["1921","87705"],["1922","97928"],["1923","87595"],["1924","77621"],["1925","86268"],["1926","75320"],["1927","93265"],["1928","98415"],["1929","95147"],["1930","89376"],["1931","92321"],["1932","89659"],["1933","73810"],["1934","81161"],["1935","98760"],["1936","95033"],["1937","99423"],["1938","104607"],["1939","111607"],["1940","89578"],["1941","87382"],["1942","80999"],["1943","72438"],["1944","63555"],["1945","65740"],["1946","99194"],["1947","128027"],["1948","127280"],["1949","129839"],["1950","130970"],["1951","129830"],["1952","140254"],["1953","135601"],["1954","136245"],["1955","135426"],["1956","144724"],["1957","164731"],["1958","167365"],["1959","155146"],["1960","198431"],["1961","204266"],["1962","227773"],["1963","262218"],["1964","251954"],["1965","284006"],["1966","296823"],["1967","296341"],["1968","330703"],["1969","338014"],["1970","362961"],["1971","342179"],["1972","368280"],["1973","325039"],["1974","301974"],["1975","317847"],["1976","338004"],["1977","341041"],["1978","335964"],["1979","343888"],["1980","343948"],["1981","350879"],["1982","355099"],["1983","382659"],["1984","387854"],["1985","409539"],["1986","432477"],["1987","468344"],["1988","453332"],["1989","473135"],["1990","518764"],["1991","508480"],["1992","552839"],["1993","535924"],["1994","593924"],["1995","598596"],["1996","664239"],["1997","687756"],["1998","671306"],["1999","755735"],["2000","826761"],["2001","788880"],["2002","859811"],["2003","902167"],["2004","1008059"],["2005","983465"],["2006","1054782"],["2007","1116060"],["2008","1382800"]] Anagrams for speech »How to pronounce speech. Alex US English David US English Mark US English Daniel British Libby British Mia British Karen Australian Hayley Australian Natasha Australian Veena Indian Priya Indian Neerja Indian Zira US English Oliver British Wendy British Fred US English Tessa South African How to say speech in sign language?Chaldean Numerology The numerical value of speech in Chaldean Numerology is: 2 Pythagorean Numerology The numerical value of speech in Pythagorean Numerology is: 2 Examples of speech in a SentenceKierkegaard : People demand freedom of speech as a compensation for the freedom of thought which they seldom use. Republican governors across America are leading the charge in defending liberty and securing unmatched economic prosperity in our states, the Biden administration is governing from the far-left, ignoring the problems of working-class Americans while pushing an agenda that stifles free speech , free thought, and economic freedom. The American people have had enough, but there is an alternative and that's what I look forward to sharing on Tuesday evening. Julia Louis-Dreyfus : These last few nights have been going so well weve decided to add a fifth night where we will just play Michelle Obamas speech on a loop. Jacob Frey : So while our thriving city is open to everyone, I will continue to stand alongside people in Minneapolis and reject speech and behavior that make any of our residents less safe. Katie Sanders : I am using my right of free speech to voice the opinion that is not being heard, i expected that a petition or something of the sort would go around. I am not upset that it is happening because they have that right, but I would be upset if I were to lose my job becauseI love it and have not had any problems with any residents before this. The death threats I have been receiving are not okay and that should be universally accepted. As for the WCU Faculty Senate, the point is being proven about opposing views because they are saying our opinion is nonsense and proceeded to create t-shirts for sale. After being called out for their post, they immediately deleted it. Popularity rank by frequency of use- ^ Princeton's WordNet http://wordnetweb.princeton.edu/perl/webwn?s=speech
- ^ Wiktionary https://en.wiktionary.org/wiki/Speech
- ^ Samuel Johnson's Dictionary https://johnsonsdictionaryonline.com/views/search.php?term=speech
- ^ Wikipedia https://en.wikipedia.org/wiki/Speech
- ^ ChatGPT https://chat.openai.com
- ^ Webster Dictionary https://www.merriam-webster.com/dictionary/speech
- ^ Wikidata https://www.wikidata.org/w/index.php?search=speech
- ^ Chambers 20th Century Dictionary https://www.gutenberg.org/files/37683/37683-h/37683-h.htm#:~:text=speech
- ^ Surnames Frequency by Census Records https://www.census.gov/topics/population/genealogy/data/2010_surnames.html
- ^ Usage in printed sources https://books.google.com/ngrams/graph?content=speech
Word of the DayWould you like us to send you a free new word definition delivered to your inbox daily. Please enter your email address: CitationUse the citation below to add this definition to your bibliography:. Style: MLA Chicago APA "speech." Definitions.net. STANDS4 LLC, 2024. Web. 2 Sep. 2024. < https://www.definitions.net/definition/speech >.  Discuss these speech definitions with the community: Report CommentWe're doing our best to make sure our content is useful, accurate and safe. If by any chance you spot an inappropriate comment while navigating through our website please use this form to let us know, and we'll take care of it shortly. You need to be logged in to favorite .Create a new account. Your name: * Required Your email address: * Required Pick a user name: * Required Username: * Required Password: * Required Forgot your password? Retrieve it Are we missing a good definition for speech ? Don't keep it to yourself...Image credit, the web's largest resource for, definitions & translations, a member of the stands4 network, image or illustration of. .ogv/256px--Real-time_MRI_-_Speaking_(English).ogv.jpg) Free, no signup required :Add to chrome, add to firefox, browse definitions.net, are you a words master, the act of carrying something, Nearby & related entries:. - speculum noun
- spedding, james
- speech acoustics
- speech act noun
- speech articulation tests
- speech assessment methods phonetic alphabet
- speech balloon
Alternative searches for speech :- Search for speech on Amazon
  |
IMAGES
VIDEO
COMMENTS
In the field of sociolinguistics, social network describes the structure of a particular speech community.Social networks are composed of a "web of ties" (Lesley Milroy) between individuals, and the structure of a network will vary depending on the types of connections it is composed of.Social network theory (as used by sociolinguists) posits that social networks, and the interactions between ...
Speech community is a term in sociolinguistics and linguistic anthropology used to describe a group of people who share the same language, speech characteristics, and ways of interpreting communication. Speech communities may be large regions like an urban area with a common, distinct accent (think of Boston with its dropped r's) or small units like families and friends (think of a nickname ...
A speech community is a group of people who share a set of linguistic norms and expectations regarding the use of language. [ 1] It is a concept mostly associated with sociolinguistics and anthropological linguistics . Exactly how to define speech community is debated in the literature. Definitions of speech community tend to involve varying ...
The speech community (SpCom), a core concept in empirical linguistics, is the. intersection of many principal problems in sociolinguistic theory and method. I trace its history of development and ...
In this study, we delineate the neural substrates underlying the planning processes relevant for rapid turn-taking by measuring cortical activity while participants engage in structured ...
The meaning of SPEECH COMMUNITY is a group of people sharing characteristic patterns of vocabulary, grammar, and pronunciation.
A communication network refers to an interconnected system that enables the exchange and flow of information among individuals, teams, and departments. The communication network within an organization consists of various components such as hierarchies, departments, teams, and individuals, each with specific roles and responsibilities.
The concept of the speech community is one of the important concepts in sociolinguistics. In this video I have discussed what is speech communit...
A community: a group of people with a shared set of activities, practices, beliefs, and social structures. A speech community: a group of people who share similar ideas, uses, and norms of language. The kind of group that sociolinguists attempt to study is called Speech Community.
The meaning of NETWORK is a fabric or structure of cords or wires that cross at regular intervals and are knotted or secured at the crossings. How to use network in a sentence. ... Share the Definition of network on Twitter Twitter. Kids Definition. network. 1 of 2 noun. net· work ˈnet-ˌwərk . 1
The algorithm ( netts) constructs networks from a speech transcript that represent the content of what the speaker said. The idea here is that the nodes in the network show the entities that the speaker mentioned, like a cat, a house, etc. (usually nouns). And the edges of the network show the relationships between the entities.
Speech recognition, also known as automatic speech recognition (ASR), computer speech recognition or speech-to-text, is a capability that enables a program to process human speech into a written format. While speech recognition is commonly confused with voice recognition, speech recognition focuses on the translation of speech from a verbal ...
Every neural network consists of layers of nodes, or artificial neurons—an input layer, one or more hidden layers, and an output layer. Each node connects to others, and has its own associated weight and threshold. If the output of any individual node is above the specified threshold value, that node is activated, sending data to the next ...
Communication. Communication refers to the process of exchanging information, including emotions and thoughts (Bishop and al., 2016), with others using speaking, writing, signs, facial expressions and body language. Communication thus incorporates speech and language, but also prosody (linguistic and emotional).
Speech recognition is an interdisciplinary subfield of computer science and computational linguistics that develops methodologies and technologies that enable the recognition and translation of spoken language into text by computers. It is also known as automatic speech recognition (ASR), computer speech recognition or speech-to-text (STT).It incorporates knowledge and research in the computer ...
The meaning of NETWORKING is the exchange of information or services among individuals, groups, or institutions; specifically : the cultivation of productive relationships for employment or business. How to use networking in a sentence.
A speech analyst is a specialist responsible for interpreting the data generated by speech analytics tools. Their primary tasks include analyzing call recordings, identifying trends and providing actionable insights to help businesses improve customer service, ensure compliance and optimize operational efficiency.
speech network translation in English - English Reverso dictionary, see also 'curtain speech, direct speech, free speech, indirect speech', examples, definition, conjugation
The Underground Railroad was a network of people and safe houses, not a railroad with tracks and trains. synonyms: organization, system similar words: association, league, order: definition 2: a group of radio or television stations, or a company that controls such a group.
Learn the definition of 'speech network'. Check out the pronunciation, synonyms and grammar. ... Using said short call number, the user establishes a link with the speech service network server and utilizes the designation of the service in the speech service network server (VOXP) to identify and start the service. patents-wipo.
Automatic Speech Recognition (ASR) is a technology that enables computers to understand and transcribe spoken language into text. It works by analyzing audio input, such as spoken words, and converting them into written text, typically in real-time. ASR systems use algorithms and machine learning techniques to recognize and interpret speech ...
Free Speech TV (FSTV) is an American progressive news and opinion network.It was launched in 1995 and is owned and operated by Public Communicators Incorporated, a 501(c)(3) non-profit, tax-exempt organization founded in 1974. Distributed principally by Dish Network, DirecTV, and the network's live stream at freespeech.org and on Roku, Free Speech TV has run commercial free since 1995 with ...
Definition of speech in the Definitions.net dictionary. Meaning of speech. What does speech mean? Information and translations of speech in the most comprehensive dictionary definitions resource on the web. Login . The STANDS4 Network. ... A Member Of The STANDS4 Network.
Vice President Kamala Harris on Thursday offered her most expansive explanation to date on why she's changed some of her positions on fracking and immigration, telling CNN's Dana Bash her ...