
- Account Logins


What We Offer
With a comprehensive suite of qualitative and quantitative capabilities and 55 years of experience in the industry, Sago powers insights through adaptive solutions.
- Recruitment
- Communities
- Methodify® Automated research
- QualBoard® Digital Discussions
- QualMeeting® Digital Interviews
- Global Qualitative
- Global Quantitative
- In-Person Facilities
- Healthcare Solutions
- Research Consulting
- Europe Solutions
- Neuromarketing Tools
- Trial & Jury Consulting
Who We Serve
Form deeper customer connections and make the process of answering your business questions easier. Sago delivers unparalleled access to the audiences you need through adaptive solutions and a consultative approach.
- Consumer Packaged Goods
- Financial Services
- Media Technology
- Medical Device Manufacturing
- Marketing Research
With a 55-year legacy of impact, Sago has proven we have what it takes to be a long-standing industry leader and partner. We continually advance our range of expertise to provide our clients with the highest level of confidence.
- Global Offices
- Partnerships & Certifications
- News & Media
- Researcher Events

Take Your Research to the Next Level with Multi-Video AI Summaries

Steve Schlesinger Inducted Into 2024 Market Research Council Hall of Fame
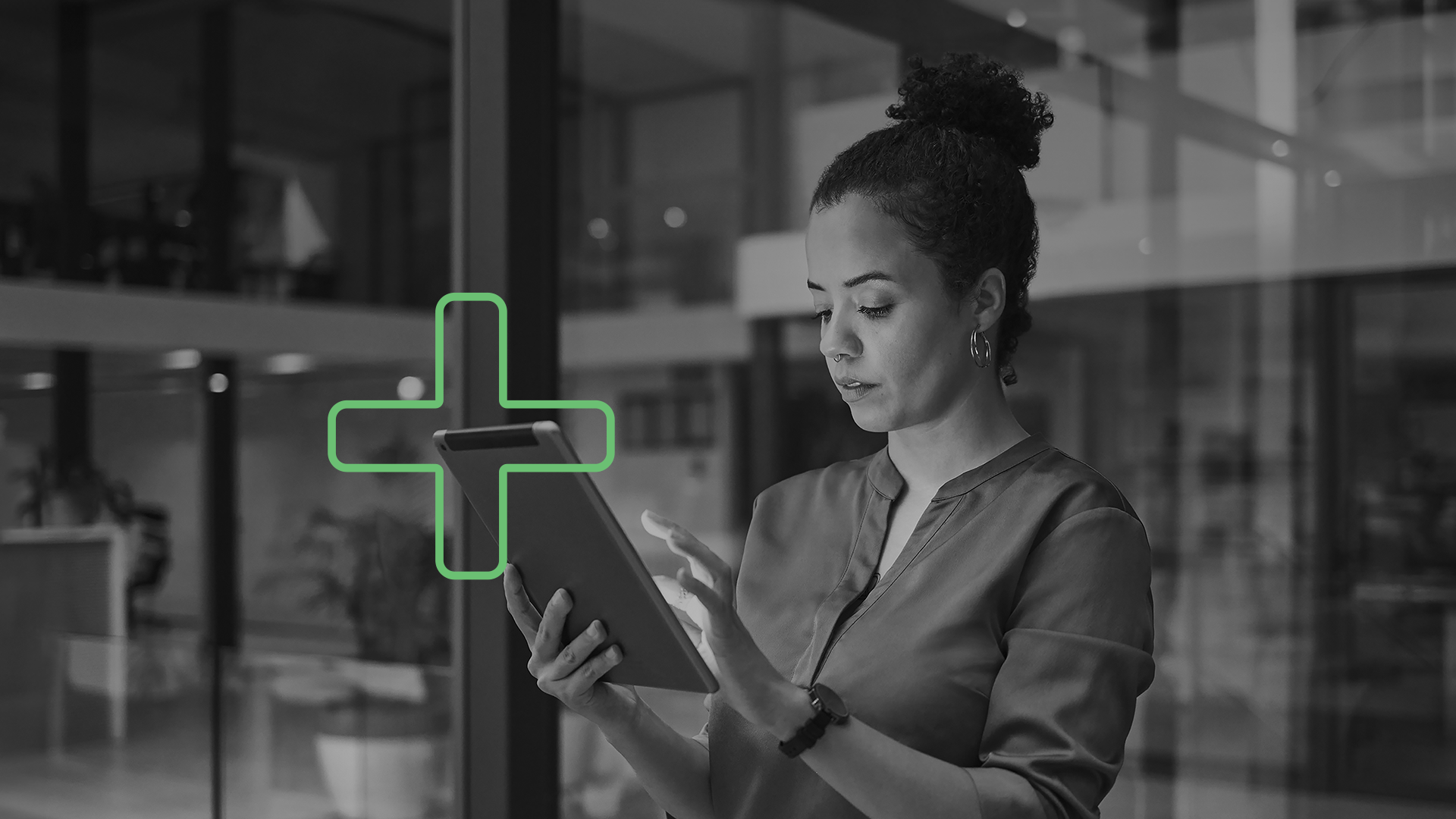
Sago Announces Launch of Sago Health to Elevate Healthcare Research
Drop into your new favorite insights rabbit hole and explore content created by the leading minds in market research.
- Case Studies
- Knowledge Kit

When Europe Hits Pause: The Summer Slowdown and What It Means for Business

The Swing Voters Project, August 2024: Wisconsin
- Partner with us
- Join our panel

Different Types of Sampling Techniques in Qualitative Research
- Resources , Blog

Key Takeaways:
- Sampling techniques in qualitative research include purposive, convenience, snowball, and theoretical sampling.
- Choosing the right sampling technique significantly impacts the accuracy and reliability of the research results.
- It’s crucial to consider the potential impact on the bias, sample diversity, and generalizability when choosing a sampling technique for your qualitative research.
Qualitative research seeks to understand social phenomena from the perspective of those experiencing them. It involves collecting non-numerical data such as interviews, observations, and written documents to gain insights into human experiences, attitudes, and behaviors. While qualitative research can provide rich and nuanced insights, the accuracy and generalizability of findings depend on the quality of the sampling process. Sampling techniques are a critical component of qualitative research as it involves selecting a group of participants who can provide valuable insights into the research questions.
This article explores different types of sampling techniques in qualitative research. First, we’ll provide a comprehensive overview of four standard sampling techniques in qualitative research. and then compare and contrast these techniques to provide guidance on choosing the most appropriate method for a particular study. Additionally, you’ll find best practices for sampling and learn about ethical considerations researchers need to consider in selecting a sample. Overall, this article aims to help researchers conduct effective and high-quality sampling in qualitative research.
In this Article:
- Purposive Sampling
- Convenience Sampling
- Snowball Sampling
- Theoretical Sampling
Factors to Consider When Choosing a Sampling Technique
Practical approaches to sampling: recommended practices, final thoughts, get expert guidance on your sample needs.
Want expert input on the best sampling technique for your qualitative research project? Book a consultation for trusted advice.
Request a consultation
4 Types of Sampling Techniques and Their Applications
Sampling is a crucial aspect of qualitative research as it determines the representativeness and credibility of the data collected. Several sampling techniques are used in qualitative research, each with strengths and weaknesses. In this section, let’s explore four standard sampling techniques in qualitative research: purposive sampling, convenience sampling, snowball sampling, and theoretical sampling. We’ll break down the definition of each technique, when to use it, and its advantages and disadvantages.
1. Purposive Sampling
Purposive sampling, or judgmental sampling, is a non-probability sampling technique in qualitative research that’s commonly used. In purposive sampling, researchers intentionally select participants with specific characteristics or unique experiences related to the research question. The goal is to identify and recruit participants who can provide rich and diverse data to enhance the research findings.
Purposive sampling is used when researchers seek to identify individuals or groups with particular knowledge, skills, or experiences relevant to the research question. For instance, in a study examining the experiences of cancer patients undergoing chemotherapy, purposive sampling may be used to recruit participants who have undergone chemotherapy in the past year. Researchers can better understand the phenomenon under investigation by selecting individuals with relevant backgrounds.
Purposive Sampling: Strengths and Weaknesses
Purposive sampling is a powerful tool for researchers seeking to select participants who can provide valuable insight into their research question. This method is advantageous when studying groups with technical characteristics or experiences where a random selection of participants may yield different results.
One of the main advantages of purposive sampling is the ability to improve the quality and accuracy of data collected by selecting participants most relevant to the research question. This approach also enables researchers to collect data from diverse participants with unique perspectives and experiences related to the research question.
However, researchers should also be aware of potential bias when using purposive sampling. The researcher’s judgment may influence the selection of participants, resulting in a biased sample that does not accurately represent the broader population. Another disadvantage is that purposive sampling may not be representative of the more general population, which limits the generalizability of the findings. To guarantee the accuracy and dependability of data obtained through purposive sampling, researchers must provide a clear and transparent justification of their selection criteria and sampling approach. This entails outlining the specific characteristics or experiences required for participants to be included in the study and explaining the rationale behind these criteria. This level of transparency not only helps readers to evaluate the validity of the findings, but also enhances the replicability of the research.
2. Convenience Sampling
When time and resources are limited, researchers may opt for convenience sampling as a quick and cost-effective way to recruit participants. In this non-probability sampling technique, participants are selected based on their accessibility and willingness to participate rather than their suitability for the research question. Qualitative research often uses this approach to generate various perspectives and experiences.
During the COVID-19 pandemic, convenience sampling was a valuable method for researchers to collect data quickly and efficiently from participants who were easily accessible and willing to participate. For example, in a study examining the experiences of university students during the pandemic, convenience sampling allowed researchers to recruit students who were available and willing to share their experiences quickly. While the pandemic may be over, convenience sampling during this time highlights its value in urgent situations where time and resources are limited.
Convenience Sampling: Strengths and Weaknesses
Convenience sampling offers several advantages to researchers, including its ease of implementation and cost-effectiveness. This technique allows researchers to quickly and efficiently recruit participants without spending time and resources identifying and contacting potential participants. Furthermore, convenience sampling can result in a diverse pool of participants, as individuals from various backgrounds and experiences may be more likely to participate.
While convenience sampling has the advantage of being efficient, researchers need to acknowledge its limitations. One of the primary drawbacks of convenience sampling is that it is susceptible to selection bias. Participants who are more easily accessible may not be representative of the broader population, which can limit the generalizability of the findings. Furthermore, convenience sampling may lead to issues with the reliability of the results, as it may not be possible to replicate the study using the same sample or a similar one.
To mitigate these limitations, researchers should carefully define the population of interest and ensure the sample is drawn from that population. For instance, if a study is investigating the experiences of individuals with a particular medical condition, researchers can recruit participants from specialized clinics or support groups for that condition. Researchers can also use statistical techniques such as stratified sampling or weighting to adjust for potential biases in the sample.
3. Snowball Sampling
Snowball sampling, also called referral sampling, is a unique approach researchers use to recruit participants in qualitative research. The technique involves identifying a few initial participants who meet the eligibility criteria and asking them to refer others they know who also fit the requirements. The sample size grows as referrals are added, creating a chain-like structure.
Snowball sampling enables researchers to reach out to individuals who may be hard to locate through traditional sampling methods, such as members of marginalized or hidden communities. For instance, in a study examining the experiences of undocumented immigrants, snowball sampling may be used to identify and recruit participants through referrals from other undocumented immigrants.
Snowball Sampling: Strengths and Weaknesses
Snowball sampling can produce in-depth and detailed data from participants with common characteristics or experiences. Since referrals are made within a network of individuals who share similarities, researchers can gain deep insights into a specific group’s attitudes, behaviors, and perspectives.
4. Theoretical Sampling
Theoretical sampling is a sophisticated and strategic technique that can help researchers develop more in-depth and nuanced theories from their data. Instead of selecting participants based on convenience or accessibility, researchers using theoretical sampling choose participants based on their potential to contribute to the emerging themes and concepts in the data. This approach allows researchers to refine their research question and theory based on the data they collect rather than forcing their data to fit a preconceived idea.
Theoretical sampling is used when researchers conduct grounded theory research and have developed an initial theory or conceptual framework. In a study examining cancer survivors’ experiences, for example, theoretical sampling may be used to identify and recruit participants who can provide new insights into the coping strategies of survivors.
Theoretical Sampling: Strengths and Weaknesses
One of the significant advantages of theoretical sampling is that it allows researchers to refine their research question and theory based on emerging data. This means the research can be highly targeted and focused, leading to a deeper understanding of the phenomenon being studied. Additionally, theoretical sampling can generate rich and in-depth data, as participants are selected based on their potential to provide new insights into the research question.
Participants are selected based on their perceived ability to offer new perspectives on the research question. This means specific perspectives or experiences may be overrepresented in the sample, leading to an incomplete understanding of the phenomenon being studied. Additionally, theoretical sampling can be time-consuming and resource-intensive, as researchers must continuously analyze the data and recruit new participants.
To mitigate the potential for bias, researchers can take several steps. One way to reduce bias is to use a diverse team of researchers to analyze the data and make participant selection decisions. Having multiple perspectives and backgrounds can help prevent researchers from unconsciously selecting participants who fit their preconceived notions or biases.
Another solution would be to use reflexive sampling. Reflexive sampling involves selecting participants aware of the research process and provides insights into how their biases and experiences may influence their perspectives. By including participants who are reflexive about their subjectivity, researchers can generate more nuanced and self-aware findings.
Choosing the proper sampling technique in qualitative research is one of the most critical decisions a researcher makes when conducting a study. The preferred method can significantly impact the accuracy and reliability of the research results.
For instance, purposive sampling provides a more targeted and specific sample, which helps to answer research questions related to that particular population or phenomenon. However, this approach may also introduce bias by limiting the diversity of the sample.
Conversely, convenience sampling may offer a more diverse sample regarding demographics and backgrounds but may also introduce bias by selecting more willing or available participants.
Snowball sampling may help study hard-to-reach populations, but it can also limit the sample’s diversity as participants are selected based on their connections to existing participants.
Theoretical sampling may offer an opportunity to refine the research question and theory based on emerging data, but it can also be time-consuming and resource-intensive.
Additionally, the choice of sampling technique can impact the generalizability of the research findings. Therefore, it’s crucial to consider the potential impact on the bias, sample diversity, and generalizability when choosing a sampling technique. By doing so, researchers can select the most appropriate method for their research question and ensure the validity and reliability of their findings.
Tips for Selecting Participants
When selecting participants for a qualitative research study, it is crucial to consider the research question and the purpose of the study. In addition, researchers should identify the specific characteristics or criteria they seek in their sample and select participants accordingly.
One helpful tip for selecting participants is to use a pre-screening process to ensure potential participants meet the criteria for inclusion in the study. Another technique is using multiple recruitment methods to ensure the sample is diverse and representative of the studied population.
Ensuring Diversity in Samples
Diversity in the sample is important to ensure the study’s findings apply to a wide range of individuals and situations. One way to ensure diversity is to use stratified sampling, which involves dividing the population into subgroups and selecting participants from each subset. This helps establish that the sample is representative of the larger population.
Maintaining Ethical Considerations
When selecting participants for a qualitative research study, it is essential to ensure ethical considerations are taken into account. Researchers must ensure participants are fully informed about the study and provide their voluntary consent to participate. They must also ensure participants understand their rights and that their confidentiality and privacy will be protected.
A qualitative research study’s success hinges on its sampling technique’s effectiveness. The choice of sampling technique must be guided by the research question, the population being studied, and the purpose of the study. Whether purposive, convenience, snowball, or theoretical sampling, the primary goal is to ensure the validity and reliability of the study’s findings.
By thoughtfully weighing the pros and cons of each sampling technique in qualitative research, researchers can make informed decisions that lead to more reliable and accurate results. In conclusion, carefully selecting a sampling technique is integral to the success of a qualitative research study, and a thorough understanding of the available options can make all the difference in achieving high-quality research outcomes.
If you’re interested in improving your research and sampling methods, Sago offers a variety of solutions. Our qualitative research platforms, such as QualBoard and QualMeeting, can assist you in conducting research studies with precision and efficiency. Our robust global panel and recruitment options help you reach the right people. We also offer qualitative and quantitative research services to meet your research needs. Contact us today to learn more about how we can help improve your research outcomes.
Find the Right Sample for Your Qualitative Research
Trust our team to recruit the participants you need using the appropriate techniques. Book a consultation with our team to get started .
Get in touch

Efficiency Unleashed: Quick Insights with QualBoard’s Multi-video AI Summaries

Enhancing Efficiency with All-in-One Digital Qual
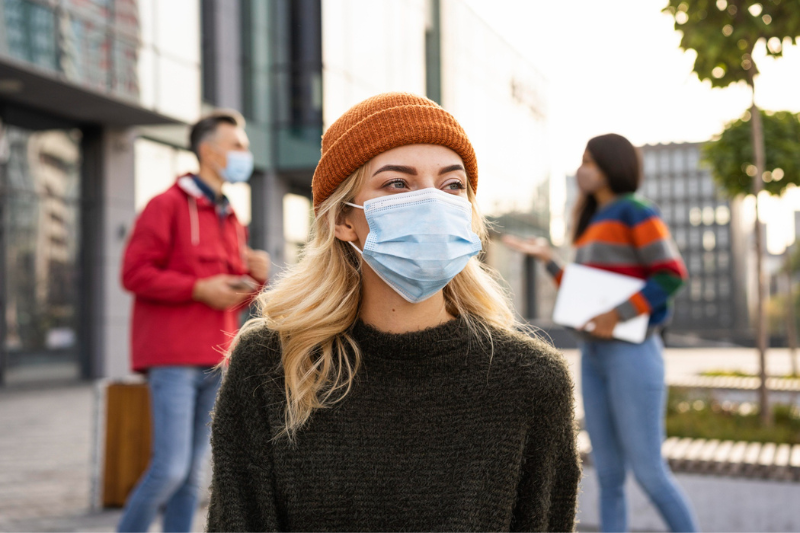
How Connecting with Gen C Can Help Your Brand Grow

The Deciders, July 2024: Former Nikki Haley Voters

OnDemand: Crack the Code: Evolving Panel Expectations

Pioneering the Future of Pediatric Health

The Swing Voter Project, July 2024: Florida

Exploring Travel Trends and Behaviors for Summer 2024
Take a deep dive into your favorite market research topics
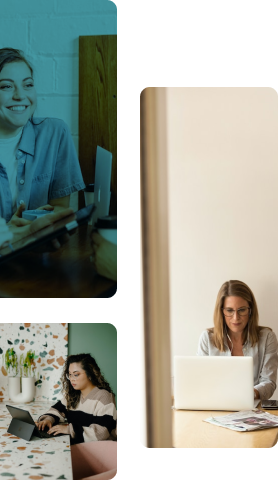
How can we help support you and your research needs?
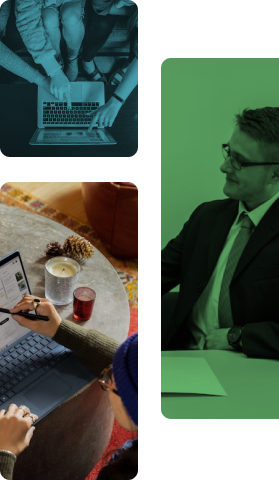
BEFORE YOU GO
Have you considered how to harness AI in your research process? Check out our on-demand webinar for everything you need to know

Have a language expert improve your writing
Run a free plagiarism check in 10 minutes, generate accurate citations for free.
- Knowledge Base
Methodology
- Sampling Methods | Types, Techniques & Examples
Sampling Methods | Types, Techniques & Examples
Published on September 19, 2019 by Shona McCombes . Revised on June 22, 2023.
When you conduct research about a group of people, it’s rarely possible to collect data from every person in that group. Instead, you select a sample . The sample is the group of individuals who will actually participate in the research.
To draw valid conclusions from your results, you have to carefully decide how you will select a sample that is representative of the group as a whole. This is called a sampling method . There are two primary types of sampling methods that you can use in your research:
- Probability sampling involves random selection, allowing you to make strong statistical inferences about the whole group.
- Non-probability sampling involves non-random selection based on convenience or other criteria, allowing you to easily collect data.
You should clearly explain how you selected your sample in the methodology section of your paper or thesis, as well as how you approached minimizing research bias in your work.
Table of contents
Population vs. sample, probability sampling methods, non-probability sampling methods, other interesting articles, frequently asked questions about sampling.
First, you need to understand the difference between a population and a sample , and identify the target population of your research.
- The population is the entire group that you want to draw conclusions about.
- The sample is the specific group of individuals that you will collect data from.
The population can be defined in terms of geographical location, age, income, or many other characteristics.
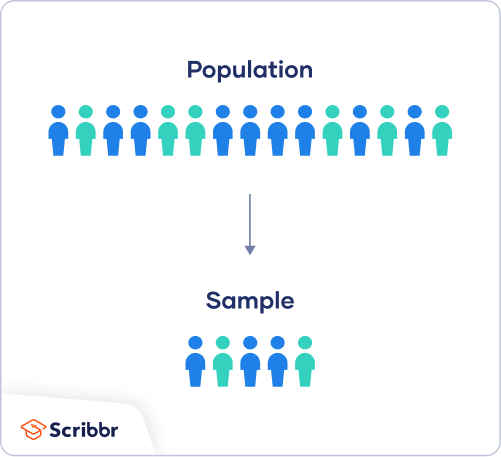
It is important to carefully define your target population according to the purpose and practicalities of your project.
If the population is very large, demographically mixed, and geographically dispersed, it might be difficult to gain access to a representative sample. A lack of a representative sample affects the validity of your results, and can lead to several research biases , particularly sampling bias .
Sampling frame
The sampling frame is the actual list of individuals that the sample will be drawn from. Ideally, it should include the entire target population (and nobody who is not part of that population).
Sample size
The number of individuals you should include in your sample depends on various factors, including the size and variability of the population and your research design. There are different sample size calculators and formulas depending on what you want to achieve with statistical analysis .
Prevent plagiarism. Run a free check.
Probability sampling means that every member of the population has a chance of being selected. It is mainly used in quantitative research . If you want to produce results that are representative of the whole population, probability sampling techniques are the most valid choice.
There are four main types of probability sample.
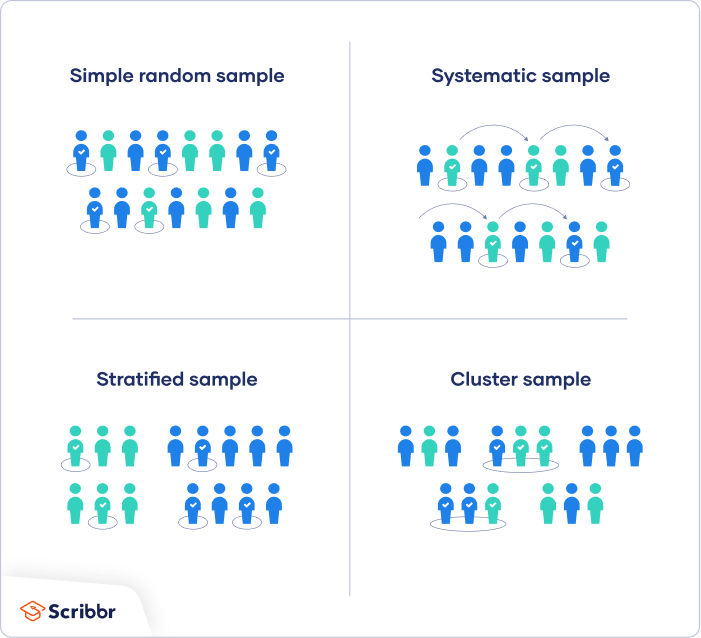
1. Simple random sampling
In a simple random sample, every member of the population has an equal chance of being selected. Your sampling frame should include the whole population.
To conduct this type of sampling, you can use tools like random number generators or other techniques that are based entirely on chance.
2. Systematic sampling
Systematic sampling is similar to simple random sampling, but it is usually slightly easier to conduct. Every member of the population is listed with a number, but instead of randomly generating numbers, individuals are chosen at regular intervals.
If you use this technique, it is important to make sure that there is no hidden pattern in the list that might skew the sample. For example, if the HR database groups employees by team, and team members are listed in order of seniority, there is a risk that your interval might skip over people in junior roles, resulting in a sample that is skewed towards senior employees.
3. Stratified sampling
Stratified sampling involves dividing the population into subpopulations that may differ in important ways. It allows you draw more precise conclusions by ensuring that every subgroup is properly represented in the sample.
To use this sampling method, you divide the population into subgroups (called strata) based on the relevant characteristic (e.g., gender identity, age range, income bracket, job role).
Based on the overall proportions of the population, you calculate how many people should be sampled from each subgroup. Then you use random or systematic sampling to select a sample from each subgroup.
4. Cluster sampling
Cluster sampling also involves dividing the population into subgroups, but each subgroup should have similar characteristics to the whole sample. Instead of sampling individuals from each subgroup, you randomly select entire subgroups.
If it is practically possible, you might include every individual from each sampled cluster. If the clusters themselves are large, you can also sample individuals from within each cluster using one of the techniques above. This is called multistage sampling .
This method is good for dealing with large and dispersed populations, but there is more risk of error in the sample, as there could be substantial differences between clusters. It’s difficult to guarantee that the sampled clusters are really representative of the whole population.
In a non-probability sample, individuals are selected based on non-random criteria, and not every individual has a chance of being included.
This type of sample is easier and cheaper to access, but it has a higher risk of sampling bias . That means the inferences you can make about the population are weaker than with probability samples, and your conclusions may be more limited. If you use a non-probability sample, you should still aim to make it as representative of the population as possible.
Non-probability sampling techniques are often used in exploratory and qualitative research . In these types of research, the aim is not to test a hypothesis about a broad population, but to develop an initial understanding of a small or under-researched population.
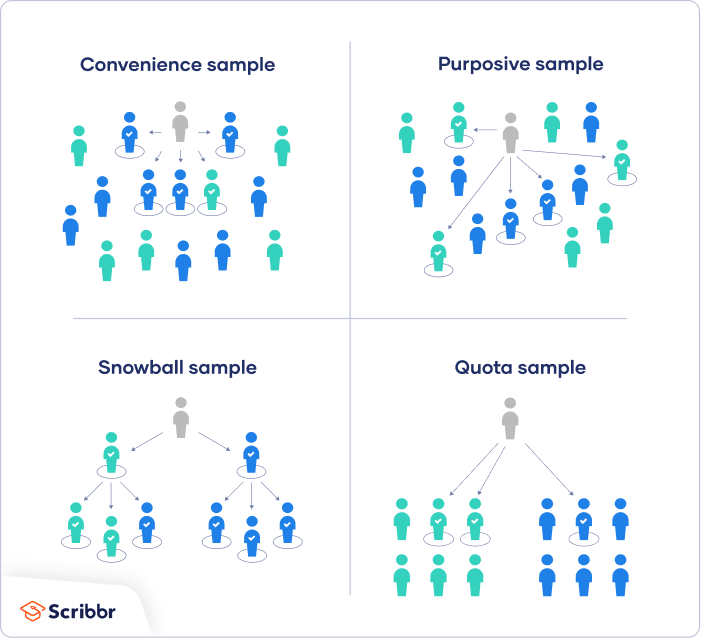
1. Convenience sampling
A convenience sample simply includes the individuals who happen to be most accessible to the researcher.
This is an easy and inexpensive way to gather initial data, but there is no way to tell if the sample is representative of the population, so it can’t produce generalizable results. Convenience samples are at risk for both sampling bias and selection bias .
2. Voluntary response sampling
Similar to a convenience sample, a voluntary response sample is mainly based on ease of access. Instead of the researcher choosing participants and directly contacting them, people volunteer themselves (e.g. by responding to a public online survey).
Voluntary response samples are always at least somewhat biased , as some people will inherently be more likely to volunteer than others, leading to self-selection bias .
3. Purposive sampling
This type of sampling, also known as judgement sampling, involves the researcher using their expertise to select a sample that is most useful to the purposes of the research.
It is often used in qualitative research , where the researcher wants to gain detailed knowledge about a specific phenomenon rather than make statistical inferences, or where the population is very small and specific. An effective purposive sample must have clear criteria and rationale for inclusion. Always make sure to describe your inclusion and exclusion criteria and beware of observer bias affecting your arguments.
4. Snowball sampling
If the population is hard to access, snowball sampling can be used to recruit participants via other participants. The number of people you have access to “snowballs” as you get in contact with more people. The downside here is also representativeness, as you have no way of knowing how representative your sample is due to the reliance on participants recruiting others. This can lead to sampling bias .
5. Quota sampling
Quota sampling relies on the non-random selection of a predetermined number or proportion of units. This is called a quota.
You first divide the population into mutually exclusive subgroups (called strata) and then recruit sample units until you reach your quota. These units share specific characteristics, determined by you prior to forming your strata. The aim of quota sampling is to control what or who makes up your sample.
If you want to know more about statistics , methodology , or research bias , make sure to check out some of our other articles with explanations and examples.
- Student’s t -distribution
- Normal distribution
- Null and Alternative Hypotheses
- Chi square tests
- Confidence interval
- Quartiles & Quantiles
- Cluster sampling
- Stratified sampling
- Data cleansing
- Reproducibility vs Replicability
- Peer review
- Prospective cohort study
Research bias
- Implicit bias
- Cognitive bias
- Placebo effect
- Hawthorne effect
- Hindsight bias
- Affect heuristic
- Social desirability bias
A sample is a subset of individuals from a larger population . Sampling means selecting the group that you will actually collect data from in your research. For example, if you are researching the opinions of students in your university, you could survey a sample of 100 students.
In statistics, sampling allows you to test a hypothesis about the characteristics of a population.
Samples are used to make inferences about populations . Samples are easier to collect data from because they are practical, cost-effective, convenient, and manageable.
Probability sampling means that every member of the target population has a known chance of being included in the sample.
Probability sampling methods include simple random sampling , systematic sampling , stratified sampling , and cluster sampling .
In non-probability sampling , the sample is selected based on non-random criteria, and not every member of the population has a chance of being included.
Common non-probability sampling methods include convenience sampling , voluntary response sampling, purposive sampling , snowball sampling, and quota sampling .
In multistage sampling , or multistage cluster sampling, you draw a sample from a population using smaller and smaller groups at each stage.
This method is often used to collect data from a large, geographically spread group of people in national surveys, for example. You take advantage of hierarchical groupings (e.g., from state to city to neighborhood) to create a sample that’s less expensive and time-consuming to collect data from.
Sampling bias occurs when some members of a population are systematically more likely to be selected in a sample than others.
Cite this Scribbr article
If you want to cite this source, you can copy and paste the citation or click the “Cite this Scribbr article” button to automatically add the citation to our free Citation Generator.
McCombes, S. (2023, June 22). Sampling Methods | Types, Techniques & Examples. Scribbr. Retrieved September 4, 2024, from https://www.scribbr.com/methodology/sampling-methods/
Is this article helpful?
Shona McCombes
Other students also liked, population vs. sample | definitions, differences & examples, simple random sampling | definition, steps & examples, sampling bias and how to avoid it | types & examples, what is your plagiarism score.

Sampling Methods & Strategies 101
Everything you need to know (including examples)
By: Derek Jansen (MBA) | Expert Reviewed By: Kerryn Warren (PhD) | January 2023
If you’re new to research, sooner or later you’re bound to wander into the intimidating world of sampling methods and strategies. If you find yourself on this page, chances are you’re feeling a little overwhelmed or confused. Fear not – in this post we’ll unpack sampling in straightforward language , along with loads of examples .
Overview: Sampling Methods & Strategies
- What is sampling in a research context?
- The two overarching approaches
Simple random sampling
Stratified random sampling, cluster sampling, systematic sampling, purposive sampling, convenience sampling, snowball sampling.
- How to choose the right sampling method
What (exactly) is sampling?
At the simplest level, sampling (within a research context) is the process of selecting a subset of participants from a larger group . For example, if your research involved assessing US consumers’ perceptions about a particular brand of laundry detergent, you wouldn’t be able to collect data from every single person that uses laundry detergent (good luck with that!) – but you could potentially collect data from a smaller subset of this group.
In technical terms, the larger group is referred to as the population , and the subset (the group you’ll actually engage with in your research) is called the sample . Put another way, you can look at the population as a full cake and the sample as a single slice of that cake. In an ideal world, you’d want your sample to be perfectly representative of the population, as that would allow you to generalise your findings to the entire population. In other words, you’d want to cut a perfect cross-sectional slice of cake, such that the slice reflects every layer of the cake in perfect proportion.
Achieving a truly representative sample is, unfortunately, a little trickier than slicing a cake, as there are many practical challenges and obstacles to achieving this in a real-world setting. Thankfully though, you don’t always need to have a perfectly representative sample – it all depends on the specific research aims of each study – so don’t stress yourself out about that just yet!
With the concept of sampling broadly defined, let’s look at the different approaches to sampling to get a better understanding of what it all looks like in practice.
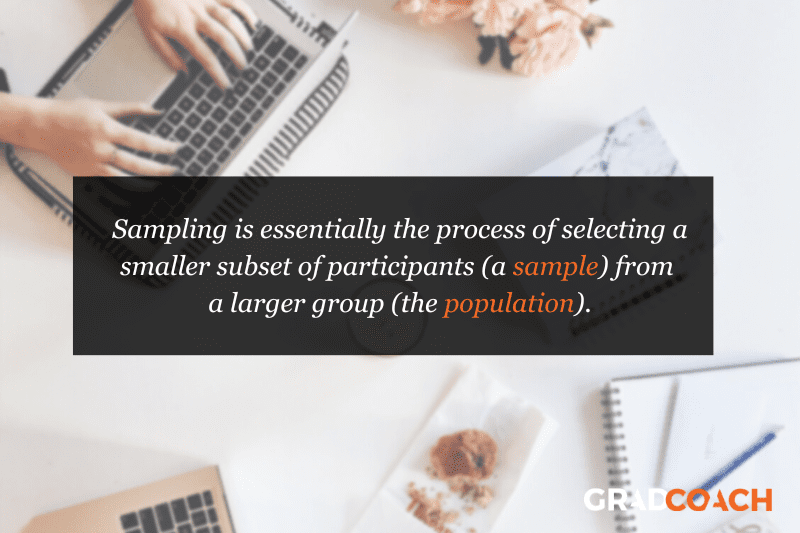
The two overarching sampling approaches
At the highest level, there are two approaches to sampling: probability sampling and non-probability sampling . Within each of these, there are a variety of sampling methods , which we’ll explore a little later.
Probability sampling involves selecting participants (or any unit of interest) on a statistically random basis , which is why it’s also called “random sampling”. In other words, the selection of each individual participant is based on a pre-determined process (not the discretion of the researcher). As a result, this approach achieves a random sample.
Probability-based sampling methods are most commonly used in quantitative research , especially when it’s important to achieve a representative sample that allows the researcher to generalise their findings.
Non-probability sampling , on the other hand, refers to sampling methods in which the selection of participants is not statistically random . In other words, the selection of individual participants is based on the discretion and judgment of the researcher, rather than on a pre-determined process.
Non-probability sampling methods are commonly used in qualitative research , where the richness and depth of the data are more important than the generalisability of the findings.
If that all sounds a little too conceptual and fluffy, don’t worry. Let’s take a look at some actual sampling methods to make it more tangible.
Need a helping hand?
Probability-based sampling methods
First, we’ll look at four common probability-based (random) sampling methods:
Importantly, this is not a comprehensive list of all the probability sampling methods – these are just four of the most common ones. So, if you’re interested in adopting a probability-based sampling approach, be sure to explore all the options.
Simple random sampling involves selecting participants in a completely random fashion , where each participant has an equal chance of being selected. Basically, this sampling method is the equivalent of pulling names out of a hat , except that you can do it digitally. For example, if you had a list of 500 people, you could use a random number generator to draw a list of 50 numbers (each number, reflecting a participant) and then use that dataset as your sample.
Thanks to its simplicity, simple random sampling is easy to implement , and as a consequence, is typically quite cheap and efficient . Given that the selection process is completely random, the results can be generalised fairly reliably. However, this also means it can hide the impact of large subgroups within the data, which can result in minority subgroups having little representation in the results – if any at all. To address this, one needs to take a slightly different approach, which we’ll look at next.
Stratified random sampling is similar to simple random sampling, but it kicks things up a notch. As the name suggests, stratified sampling involves selecting participants randomly , but from within certain pre-defined subgroups (i.e., strata) that share a common trait . For example, you might divide the population into strata based on gender, ethnicity, age range or level of education, and then select randomly from each group.
The benefit of this sampling method is that it gives you more control over the impact of large subgroups (strata) within the population. For example, if a population comprises 80% males and 20% females, you may want to “balance” this skew out by selecting a random sample from an equal number of males and females. This would, of course, reduce the representativeness of the sample, but it would allow you to identify differences between subgroups. So, depending on your research aims, the stratified approach could work well.
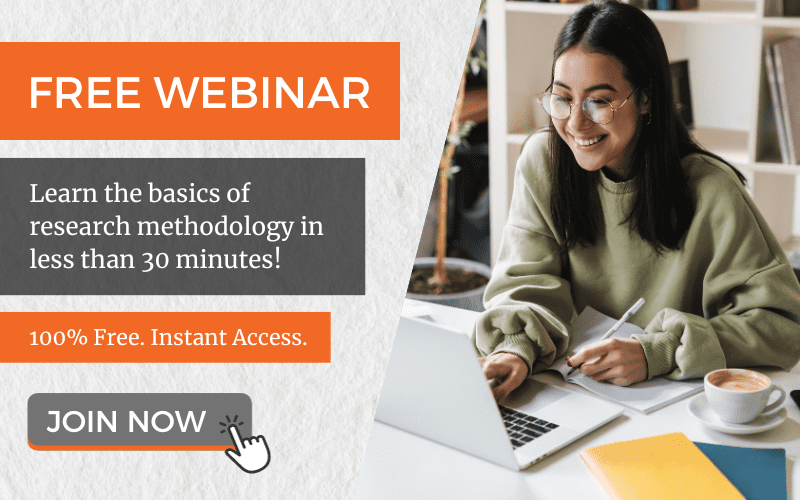
Next on the list is cluster sampling. As the name suggests, this sampling method involves sampling from naturally occurring, mutually exclusive clusters within a population – for example, area codes within a city or cities within a country. Once the clusters are defined, a set of clusters are randomly selected and then a set of participants are randomly selected from each cluster.
Now, you’re probably wondering, “how is cluster sampling different from stratified random sampling?”. Well, let’s look at the previous example where each cluster reflects an area code in a given city.
With cluster sampling, you would collect data from clusters of participants in a handful of area codes (let’s say 5 neighbourhoods). Conversely, with stratified random sampling, you would need to collect data from all over the city (i.e., many more neighbourhoods). You’d still achieve the same sample size either way (let’s say 200 people, for example), but with stratified sampling, you’d need to do a lot more running around, as participants would be scattered across a vast geographic area. As a result, cluster sampling is often the more practical and economical option.
If that all sounds a little mind-bending, you can use the following general rule of thumb. If a population is relatively homogeneous , cluster sampling will often be adequate. Conversely, if a population is quite heterogeneous (i.e., diverse), stratified sampling will generally be more appropriate.
The last probability sampling method we’ll look at is systematic sampling. This method simply involves selecting participants at a set interval , starting from a random point .
For example, if you have a list of students that reflects the population of a university, you could systematically sample that population by selecting participants at an interval of 8 . In other words, you would randomly select a starting point – let’s say student number 40 – followed by student 48, 56, 64, etc.
What’s important with systematic sampling is that the population list you select from needs to be randomly ordered . If there are underlying patterns in the list (for example, if the list is ordered by gender, IQ, age, etc.), this will result in a non-random sample, which would defeat the purpose of adopting this sampling method. Of course, you could safeguard against this by “shuffling” your population list using a random number generator or similar tool.
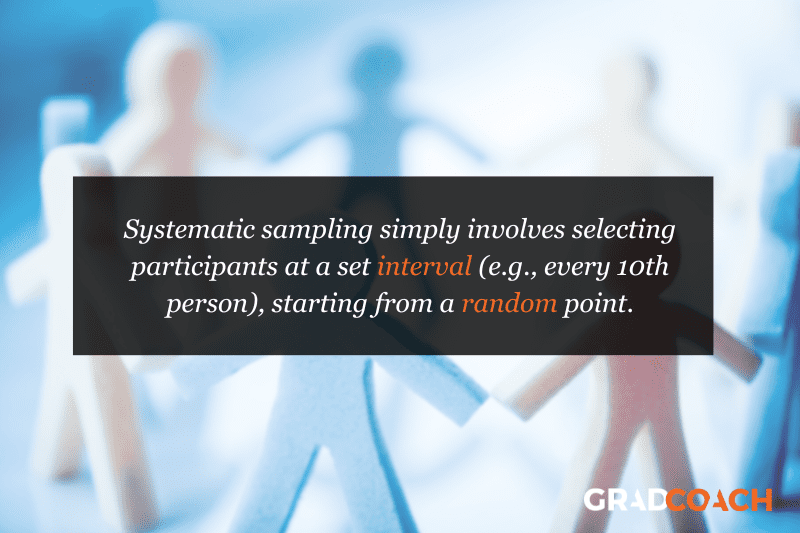
Non-probability-based sampling methods
Right, now that we’ve looked at a few probability-based sampling methods, let’s look at three non-probability methods :
Again, this is not an exhaustive list of all possible sampling methods, so be sure to explore further if you’re interested in adopting a non-probability sampling approach.
First up, we’ve got purposive sampling – also known as judgment , selective or subjective sampling. Again, the name provides some clues, as this method involves the researcher selecting participants using his or her own judgement , based on the purpose of the study (i.e., the research aims).
For example, suppose your research aims were to understand the perceptions of hyper-loyal customers of a particular retail store. In that case, you could use your judgement to engage with frequent shoppers, as well as rare or occasional shoppers, to understand what judgements drive the two behavioural extremes .
Purposive sampling is often used in studies where the aim is to gather information from a small population (especially rare or hard-to-find populations), as it allows the researcher to target specific individuals who have unique knowledge or experience . Naturally, this sampling method is quite prone to researcher bias and judgement error, and it’s unlikely to produce generalisable results, so it’s best suited to studies where the aim is to go deep rather than broad .
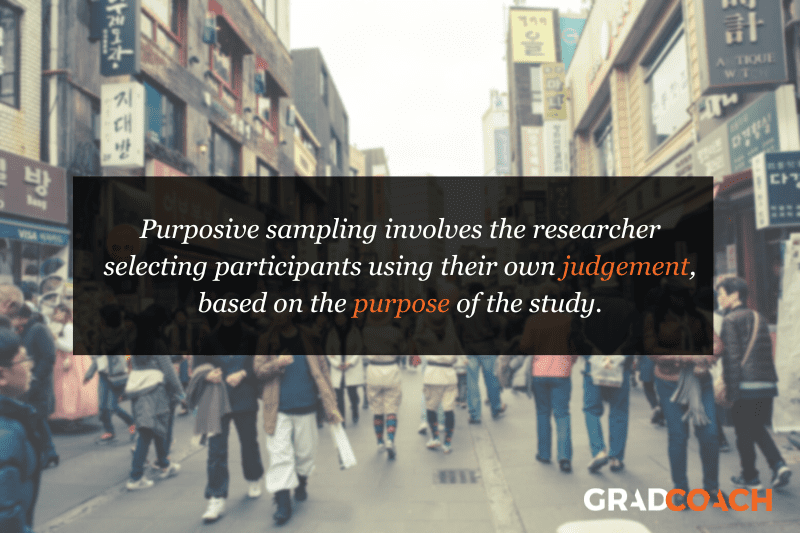
Next up, we have convenience sampling. As the name suggests, with this method, participants are selected based on their availability or accessibility . In other words, the sample is selected based on how convenient it is for the researcher to access it, as opposed to using a defined and objective process.
Naturally, convenience sampling provides a quick and easy way to gather data, as the sample is selected based on the individuals who are readily available or willing to participate. This makes it an attractive option if you’re particularly tight on resources and/or time. However, as you’d expect, this sampling method is unlikely to produce a representative sample and will of course be vulnerable to researcher bias , so it’s important to approach it with caution.
Last but not least, we have the snowball sampling method. This method relies on referrals from initial participants to recruit additional participants. In other words, the initial subjects form the first (small) snowball and each additional subject recruited through referral is added to the snowball, making it larger as it rolls along .
Snowball sampling is often used in research contexts where it’s difficult to identify and access a particular population. For example, people with a rare medical condition or members of an exclusive group. It can also be useful in cases where the research topic is sensitive or taboo and people are unlikely to open up unless they’re referred by someone they trust.
Simply put, snowball sampling is ideal for research that involves reaching hard-to-access populations . But, keep in mind that, once again, it’s a sampling method that’s highly prone to researcher bias and is unlikely to produce a representative sample. So, make sure that it aligns with your research aims and questions before adopting this method.
How to choose a sampling method
Now that we’ve looked at a few popular sampling methods (both probability and non-probability based), the obvious question is, “ how do I choose the right sampling method for my study?”. When selecting a sampling method for your research project, you’ll need to consider two important factors: your research aims and your resources .
As with all research design and methodology choices, your sampling approach needs to be guided by and aligned with your research aims, objectives and research questions – in other words, your golden thread. Specifically, you need to consider whether your research aims are primarily concerned with producing generalisable findings (in which case, you’ll likely opt for a probability-based sampling method) or with achieving rich , deep insights (in which case, a non-probability-based approach could be more practical). Typically, quantitative studies lean toward the former, while qualitative studies aim for the latter, so be sure to consider your broader methodology as well.
The second factor you need to consider is your resources and, more generally, the practical constraints at play. If, for example, you have easy, free access to a large sample at your workplace or university and a healthy budget to help you attract participants, that will open up multiple options in terms of sampling methods. Conversely, if you’re cash-strapped, short on time and don’t have unfettered access to your population of interest, you may be restricted to convenience or referral-based methods.
In short, be ready for trade-offs – you won’t always be able to utilise the “perfect” sampling method for your study, and that’s okay. Much like all the other methodological choices you’ll make as part of your study, you’ll often need to compromise and accept practical trade-offs when it comes to sampling. Don’t let this get you down though – as long as your sampling choice is well explained and justified, and the limitations of your approach are clearly articulated, you’ll be on the right track.
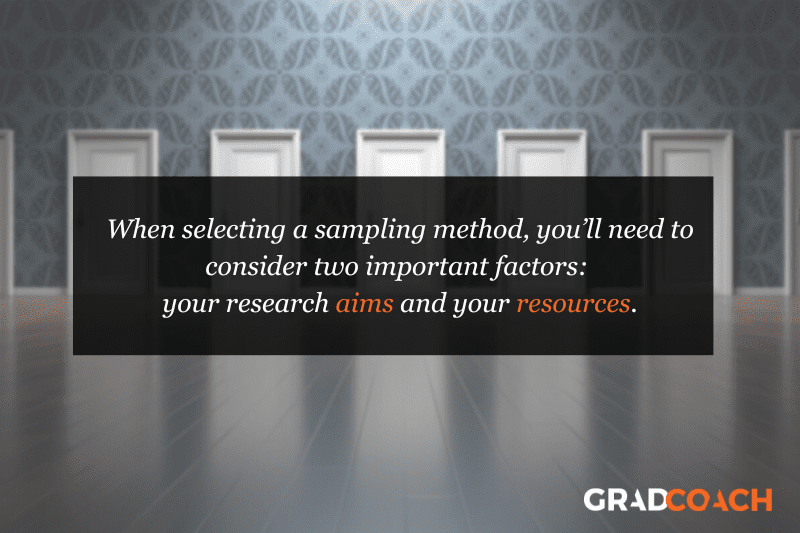
Let’s recap…
In this post, we’ve covered the basics of sampling within the context of a typical research project.
- Sampling refers to the process of defining a subgroup (sample) from the larger group of interest (population).
- The two overarching approaches to sampling are probability sampling (random) and non-probability sampling .
- Common probability-based sampling methods include simple random sampling, stratified random sampling, cluster sampling and systematic sampling.
- Common non-probability-based sampling methods include purposive sampling, convenience sampling and snowball sampling.
- When choosing a sampling method, you need to consider your research aims , objectives and questions, as well as your resources and other practical constraints .
If you’d like to see an example of a sampling strategy in action, be sure to check out our research methodology chapter sample .
Last but not least, if you need hands-on help with your sampling (or any other aspect of your research), take a look at our 1-on-1 coaching service , where we guide you through each step of the research process, at your own pace.
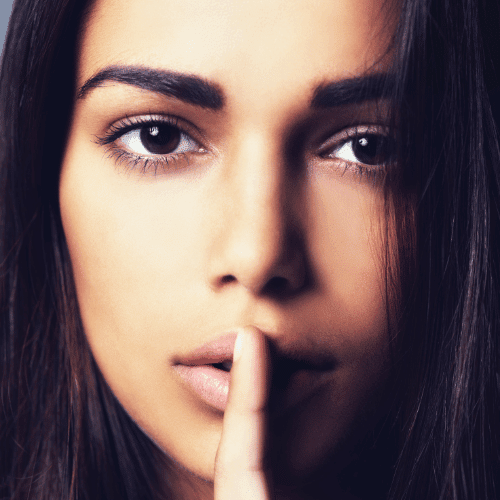
Psst... there’s more!
This post was based on one of our popular Research Bootcamps . If you're working on a research project, you'll definitely want to check this out ...
Excellent and helpful. Best site to get a full understanding of Research methodology. I’m nolonger as “clueless “..😉
Excellent and helpful for junior researcher!
Grad Coach tutorials are excellent – I recommend them to everyone doing research. I will be working with a sample of imprisoned women and now have a much clearer idea concerning sampling. Thank you to all at Grad Coach for generously sharing your expertise with students.
Submit a Comment Cancel reply
Your email address will not be published. Required fields are marked *
Save my name, email, and website in this browser for the next time I comment.
- Print Friendly
Sampling Techniques for Qualitative Research
- First Online: 27 October 2022
Cite this chapter
- Heather Douglas 4
3885 Accesses
7 Citations
This chapter explains how to design suitable sampling strategies for qualitative research. The focus of this chapter is purposive (or theoretical) sampling to produce credible and trustworthy explanations of a phenomenon (a specific aspect of society). A specific research question (RQ) guides the methodology (the study design or approach ). It defines the participants, location, and actions to be used to answer the question. Qualitative studies use specific tools and techniques ( methods ) to sample people, organizations, or whatever is to be examined. The methodology guides the selection of tools and techniques for sampling, data analysis, quality assurance, etc. These all vary according to the purpose and design of the study and the RQ. In this chapter, a fake example is used to demonstrate how to apply your sampling strategy in a developing country.
This is a preview of subscription content, log in via an institution to check access.
Access this chapter
Subscribe and save.
- Get 10 units per month
- Download Article/Chapter or eBook
- 1 Unit = 1 Article or 1 Chapter
- Cancel anytime
- Available as PDF
- Read on any device
- Instant download
- Own it forever
- Available as EPUB and PDF
- Compact, lightweight edition
- Dispatched in 3 to 5 business days
- Free shipping worldwide - see info
- Durable hardcover edition
Tax calculation will be finalised at checkout
Purchases are for personal use only
Institutional subscriptions
Similar content being viewed by others
The role of sampling in mixed methods-research.
Preparation of Qualitative Research
Sampling Errors, Bias, and Objectivity
Douglas, H. (2010). Divergent orientations in social entrepreneurship organisations. In K. Hockerts, J. Robinson, & J. Mair (Eds.), Values and opportunities in social entrepreneurship (pp. 71–95). Palgrave Macmillan.
Chapter Google Scholar
Douglas, H., Eti-Tofinga, B., & Singh, G. (2018a). Contextualising social enterprise in Fiji. Social Enterprise Journal, 14 (2), 208–224. https://doi.org/10.1108/SEJ-05-2017-0032
Article Google Scholar
Douglas, H., Eti-Tofinga, B., & Singh, G. (2018b). Hybrid organisations contributing to wellbeing in small Pacific island countries. Sustainability Accounting, Management and Policy Journal, 9 (4), 490–514. https://doi.org/10.1108/SAMPJ-08-2017-0081
Douglas, H., & Borbasi, S. (2009). Parental perspectives on disability: The story of Sam, Anna, and Marcus. Disabilities: Insights from across fields and around the world, 2 , 201–217.
Google Scholar
Douglas, H. (1999). Community transport in rural Queensland: Using community resources effectively in small communities. Paper presented at the 5th National Rural Health Conference, Adelaide, South Australia, pp. 14–17th March.
Douglas, H. (2006). Action, blastoff, chaos: ABC of successful youth participation. Child, Youth and Environments, 16 (1). Retrieved from http://www.colorado.edu/journals/cye
Douglas, H. (2007). Methodological sampling issues for researching new nonprofit organisations. Paper presented at the 52nd International Council for Small Business (ICSB) 13–15 June, Turku, Finland.
Draper, H., Wilson, S., Flanagan, S., & Ives, J. (2009). Offering payments, reimbursement and incentives to patients and family doctors to encourage participation in research. Family Practice, 26 (3), 231–238. https://doi.org/10.1093/fampra/cmp011
Puamua, P. Q. (1999). Understanding Fijian under-achievement: An integrated perspective. Directions, 21 (2), 100–112.
Download references
Author information
Authors and affiliations.
The University of Queensland, The Royal Society of Queensland, Activation Australia, Brisbane, Australia
Heather Douglas
You can also search for this author in PubMed Google Scholar
Corresponding author
Correspondence to Heather Douglas .
Editor information
Editors and affiliations.
Centre for Family and Child Studies, Research Institute of Humanities and Social Sciences, University of Sharjah, Sharjah, United Arab Emirates
M. Rezaul Islam
Department of Development Studies, University of Dhaka, Dhaka, Bangladesh
Niaz Ahmed Khan
Department of Social Work, School of Humanities, University of Johannesburg, Johannesburg, South Africa
Rajendra Baikady
Rights and permissions
Reprints and permissions
Copyright information
© 2022 The Author(s), under exclusive license to Springer Nature Singapore Pte Ltd.
About this chapter
Douglas, H. (2022). Sampling Techniques for Qualitative Research. In: Islam, M.R., Khan, N.A., Baikady, R. (eds) Principles of Social Research Methodology. Springer, Singapore. https://doi.org/10.1007/978-981-19-5441-2_29
Download citation
DOI : https://doi.org/10.1007/978-981-19-5441-2_29
Published : 27 October 2022
Publisher Name : Springer, Singapore
Print ISBN : 978-981-19-5219-7
Online ISBN : 978-981-19-5441-2
eBook Packages : Social Sciences Social Sciences (R0)
Share this chapter
Anyone you share the following link with will be able to read this content:
Sorry, a shareable link is not currently available for this article.
Provided by the Springer Nature SharedIt content-sharing initiative
- Publish with us
Policies and ethics
- Find a journal
- Track your research

An official website of the United States government
The .gov means it’s official. Federal government websites often end in .gov or .mil. Before sharing sensitive information, make sure you’re on a federal government site.
The site is secure. The https:// ensures that you are connecting to the official website and that any information you provide is encrypted and transmitted securely.
- Publications
- Account settings
- My Bibliography
- Collections
- Citation manager
Save citation to file
Email citation, add to collections.
- Create a new collection
- Add to an existing collection
Add to My Bibliography
Your saved search, create a file for external citation management software, your rss feed.
- Search in PubMed
- Search in NLM Catalog
- Add to Search
Qualitative Sampling Methods
Affiliation.
- 1 14742 School of Nursing, University of Texas Health Science Center, San Antonio, TX, USA.
- PMID: 32813616
- DOI: 10.1177/0890334420949218
Qualitative sampling methods differ from quantitative sampling methods. It is important that one understands those differences, as well as, appropriate qualitative sampling techniques. Appropriate sampling choices enhance the rigor of qualitative research studies. These types of sampling strategies are presented, along with the pros and cons of each. Sample size and data saturation are discussed.
Keywords: breastfeeding; qualitative methods; sampling; sampling methods.
PubMed Disclaimer

Similar articles
- [Saturation sampling in qualitative health research: theoretical contributions]. Fontanella BJ, Ricas J, Turato ER. Fontanella BJ, et al. Cad Saude Publica. 2008 Jan;24(1):17-27. doi: 10.1590/s0102-311x2008000100003. Cad Saude Publica. 2008. PMID: 18209831 Portuguese.
- (I Can't Get No) Saturation: A simulation and guidelines for sample sizes in qualitative research. van Rijnsoever FJ. van Rijnsoever FJ. PLoS One. 2017 Jul 26;12(7):e0181689. doi: 10.1371/journal.pone.0181689. eCollection 2017. PLoS One. 2017. PMID: 28746358 Free PMC article.
- Enhancing rigor in qualitative description: a case study. Milne J, Oberle K. Milne J, et al. J Wound Ostomy Continence Nurs. 2005 Nov-Dec;32(6):413-20. doi: 10.1097/00152192-200511000-00014. J Wound Ostomy Continence Nurs. 2005. PMID: 16301909 Review.
- Sampling Methods. Berndt AE. Berndt AE. J Hum Lact. 2020 May;36(2):224-226. doi: 10.1177/0890334420906850. Epub 2020 Mar 10. J Hum Lact. 2020. PMID: 32155099
- Sampling issues in qualitative research. Higginbottom GM. Higginbottom GM. Nurse Res. 2004;12(1):7-19. doi: 10.7748/nr2004.07.12.1.7.c5927. Nurse Res. 2004. PMID: 15493211 Review.
- Human errors in emergency medical services: a qualitative analysis of contributing factors. Poranen A, Kouvonen A, Nordquist H. Poranen A, et al. Scand J Trauma Resusc Emerg Med. 2024 Aug 30;32(1):78. doi: 10.1186/s13049-024-01253-7. Scand J Trauma Resusc Emerg Med. 2024. PMID: 39215372 Free PMC article.
- Exploring illness perceptions of multimorbidity among community-dwelling older adults: a mixed methods study. Okanlawon Bankole A, Jiwani RB, Avorgbedor F, Wang J, Osokpo OH, Gill SL, Jo Braden C. Okanlawon Bankole A, et al. Aging Health Res. 2023 Dec;3(4):100158. doi: 10.1016/j.ahr.2023.100158. Epub 2023 Sep 16. Aging Health Res. 2023. PMID: 38779434 Free PMC article.
- Virtual reality as a method of cognitive training of processing speed, working memory, and sustained attention in persons with acquired brain injury: a protocol for a randomized controlled trial. Johansen T, Matre M, Løvstad M, Lund A, Martinsen AC, Olsen A, Becker F, Brunborg C, Ponsford J, Spikman J, Neumann D, Tornås S. Johansen T, et al. Trials. 2024 May 22;25(1):340. doi: 10.1186/s13063-024-08178-7. Trials. 2024. PMID: 38778411 Free PMC article.
- Participatory Health Cadre Model to Improve Exclusive Breastfeeding Coverage with King's Conceptual System. Sukmawati E, Wijaya M, Hilmanto D. Sukmawati E, et al. J Multidiscip Healthc. 2024 Apr 24;17:1857-1875. doi: 10.2147/JMDH.S450634. eCollection 2024. J Multidiscip Healthc. 2024. PMID: 38699558 Free PMC article.
- Exploring gestational age, and birth weight assessment in Thatta district, Sindh, Pakistan: Healthcare providers' knowledge, practices, perceived barriers, and the potential of a mobile app for identifying preterm and low birth weight. Tikmani SS, Mårtensson T, Roujani S, Feroz AS, Seyfulayeva A, Mårtensson A, Brown N, Saleem S. Tikmani SS, et al. PLoS One. 2024 Apr 11;19(4):e0299395. doi: 10.1371/journal.pone.0299395. eCollection 2024. PLoS One. 2024. PMID: 38603767 Free PMC article.
- Search in MeSH
LinkOut - more resources
Full text sources.
- Ovid Technologies, Inc.
Miscellaneous
- NCI CPTAC Assay Portal
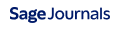
- Citation Manager
NCBI Literature Resources
MeSH PMC Bookshelf Disclaimer
The PubMed wordmark and PubMed logo are registered trademarks of the U.S. Department of Health and Human Services (HHS). Unauthorized use of these marks is strictly prohibited.
Pardon Our Interruption
As you were browsing something about your browser made us think you were a bot. There are a few reasons this might happen:
- You've disabled JavaScript in your web browser.
- You're a power user moving through this website with super-human speed.
- You've disabled cookies in your web browser.
- A third-party browser plugin, such as Ghostery or NoScript, is preventing JavaScript from running. Additional information is available in this support article .
To regain access, please make sure that cookies and JavaScript are enabled before reloading the page.

An official website of the United States government
The .gov means it’s official. Federal government websites often end in .gov or .mil. Before sharing sensitive information, make sure you’re on a federal government site.
The site is secure. The https:// ensures that you are connecting to the official website and that any information you provide is encrypted and transmitted securely.
- Publications
- Account settings
Preview improvements coming to the PMC website in October 2024. Learn More or Try it out now .
- Advanced Search
- Journal List
- Emerg (Tehran)
- v.5(1); 2017

Sampling methods in Clinical Research; an Educational Review
Mohamed elfil.
1 Faculty of Medicine, Alexandria University, Egypt.
Ahmed Negida
2 Faculty of Medicine, Zagazig University, Egypt.
Clinical research usually involves patients with a certain disease or a condition. The generalizability of clinical research findings is based on multiple factors related to the internal and external validity of the research methods. The main methodological issue that influences the generalizability of clinical research findings is the sampling method. In this educational article, we are explaining the different sampling methods in clinical research.
Introduction
In clinical research, we define the population as a group of people who share a common character or a condition, usually the disease. If we are conducting a study on patients with ischemic stroke, it will be difficult to include the whole population of ischemic stroke all over the world. It is difficult to locate the whole population everywhere and to have access to all the population. Therefore, the practical approach in clinical research is to include a part of this population, called “sample population”. The whole population is sometimes called “target population” while the sample population is called “study population. When doing a research study, we should consider the sample to be representative to the target population, as much as possible, with the least possible error and without substitution or incompleteness. The process of selecting a sample population from the target population is called the “sampling method”.
Sampling types
There are two major categories of sampling methods ( figure 1 ): 1; probability sampling methods where all subjects in the target population have equal chances to be selected in the sample [ 1 , 2 ] and 2; non-probability sampling methods where the sample population is selected in a non-systematic process that does not guarantee equal chances for each subject in the target population [ 2 , 3 ]. Samples which were selected using probability sampling methods are more representatives of the target population.

Sampling methods.
Probability sampling method
Simple random sampling
This method is used when the whole population is accessible and the investigators have a list of all subjects in this target population. The list of all subjects in this population is called the “sampling frame”. From this list, we draw a random sample using lottery method or using a computer generated random list [ 4 ].
Stratified random sampling
This method is a modification of the simple random sampling therefore, it requires the condition of sampling frame being available, as well. However, in this method, the whole population is divided into homogeneous strata or subgroups according a demographic factor (e.g. gender, age, religion, socio-economic level, education, or diagnosis etc.). Then, the researchers select draw a random sample from the different strata [ 3 , 4 ]. The advantages of this method are: (1) it allows researchers to obtain an effect size from each strata separately, as if it was a different study. Therefore, the between group differences become apparent, and (2) it allows obtaining samples from minority/under-represented populations. If the researchers used the simple random sampling, the minority population will remain underrepresented in the sample, as well. Simply, because the simple random method usually represents the whole target population. In such case, investigators can better use the stratified random sample to obtain adequate samples from all strata in the population.
Systematic random sampling (Interval sampling)
In this method, the investigators select subjects to be included in the sample based on a systematic rule, using a fixed interval. For example: If the rule is to include the last patient from every 5 patients. We will include patients with these numbers (5, 10, 15, 20, 25, ...etc.). In some situations, it is not necessary to have the sampling frame if there is a specific hospital or center which the patients are visiting regularly. In this case, the researcher can start randomly and then systemically chooses next patients using a fixed interval [ 4 ].
Cluster sampling (Multistage sampling)
It is used when creating a sampling frame is nearly impossible due to the large size of the population. In this method, the population is divided by geographic location into clusters. A list of all clusters is made and investigators draw a random number of clusters to be included. Then, they list all individuals within these clusters, and run another turn of random selection to get a final random sample exactly as simple random sampling. This method is called multistage because the selection passed with two stages: firstly, the selection of eligible clusters, then, the selection of sample from individuals of these clusters. An example for this, if we are conducting a research project on primary school students from Iran. It will be very difficult to get a list of all primary school students all over the country. In this case, a list of primary schools is made and the researcher randomly picks up a number of schools, then pick a random sample from the eligible schools [ 3 ].
Non-probability sampling method
Convenience sampling
Although it is a non-probability sampling method, it is the most applicable and widely used method in clinical research. In this method, the investigators enroll subjects according to their availability and accessibility. Therefore, this method is quick, inexpensive, and convenient. It is called convenient sampling as the researcher selects the sample elements according to their convenient accessibility and proximity [ 3 , 6 ]. For example: assume that we will perform a cohort study on Egyptian patients with Hepatitis C (HCV) virus. The convenience sample here will be confined to the accessible population for the research team. Accessible population are HCV patients attending in Zagazig University Hospital and Cairo University Hospitals. Therefore, within the study period, all patients attending these two hospitals and meet the eligibility criteria will be included in this study.
Judgmental sampling
In this method, the subjects are selected by the choice of the investigators. The researcher assumes specific characteristics for the sample (e.g. male/female ratio = 2/1) and therefore, they judge the sample to be suitable for representing the population. This method is widely criticized due to the likelihood of bias by investigator judgement [ 5 ].
Snow-ball sampling
This method is used when the population cannot be located in a specific place and therefore, it is different to access this population. In this method, the investigator asks each subject to give him access to his colleagues from the same population. This situation is common in social science research, for example, if we running a survey on street children, there will be no list with the homeless children and it will be difficult to locate this population in one place e.g. a school/hospital. Here, the investigators will deliver the survey to one child then, ask him to take them to his colleagues or deliver the surveys to them.
Conflict of interest:
Thank you for visiting nature.com. You are using a browser version with limited support for CSS. To obtain the best experience, we recommend you use a more up to date browser (or turn off compatibility mode in Internet Explorer). In the meantime, to ensure continued support, we are displaying the site without styles and JavaScript.
- View all journals
- Explore content
- About the journal
- Publish with us
- Sign up for alerts
- Open access
- Published: 02 September 2024
Fault diagnosis method for oil-immersed transformers integrated digital twin model
- Haiyan Yao 1 ,
- Xin Zhang 2 ,
- Qiang Guo 1 ,
- Yufeng Miao 1 &
- Shan Guan 3
Scientific Reports volume 14 , Article number: 20355 ( 2024 ) Cite this article
Metrics details
- Electrical and electronic engineering
- Mechanical engineering
To address the problems of low accuracy in fault diagnosis of oil-immersed transformers, poor state perception ability and real-time collaboration during diagnosis feedback, a fault diagnosis method for transformers based on the integration of digital twins is proposed. Firstly, fault sample balance is achieved through Iterative Nearest Neighbor Oversampling (INNOS), Secondly, nine-dimensional ratio features are extracted, and the correlation between dissolved gases in oil and fault types is established. Then, sparse principal component analysis (SPCA) is used for feature fusion and dimensionality reduction. Finally, the Aquila Optimizer (AO) is introduced to optimize the parameters of the Kernel Extreme Learning Machine (KELM), establishing the optimal AO-KELM diagnosis model. The final fault diagnosis accuracy reaches 98.1013%. Combining transformer digital twin models, real-time interaction mapping between physical entities and virtual space is achieved, enabling online diagnosis of transformer faults. Experimental results show that the method proposed in this paper has high diagnostic accuracy and strong stability, providing reference for the intelligent operation and maintenance of transformers.
Similar content being viewed by others
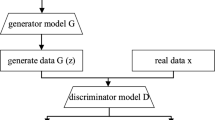
Research on transformer fault diagnosis method based on ACGAN and CGWO-LSSVM
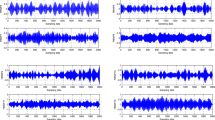
Fault diagnosis of gearbox based on Fourier Bessel EWT and manifold regularization ELM
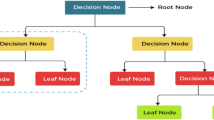
Discernment of transformer oil stray gassing anomalies using machine learning classification techniques
Introduction.
The transformer, as the hub of power systems, its health status directly impacts the stability and reliability of the electrical system's operation. Therefore, the precise management of a transformer's health status is paramount to ensuring the steadfast and secure operation of the power grid 1 .
Presently, the technology of Dissolved Gas Analysis (DGA) is extensively employed in the monitoring and identification of faults within oil-insulated transformers 2 , 3 , primarily encompassing: the IEC triad ratio method 4 , the Rogers quadruple ratio method 5 , and the DUVAL triangle technique 6 . Despite their simplicity of operation, these approaches lack the depth of representation for fault characteristics and are limited by their capabilities, resulting in a blurred and indistinct encoding boundary, thereby leading to a low accuracy rate in fault recognition 7 . With the rapid advancement of artificial intelligence, eminent scholars have integrated machine learning with DGA technology, achieving notable results in the field of transformer fault detection. The literature 8 optimizes the support vector machine parameters through the refinement of the scalar search algorithm, thereby augmenting both the convergence velocity and the diagnostic precision of the methodology. The literature 9 proffers an SE-ELM diagnostic method, whose efficacy was validated through the verification across various datasets. The literature 10 enhances the particle swarm optimization algorithm through the dynamic adjustment of inertial weights and acceleration factors, iteratively optimizing the parameters of XGBoost, thereby augmenting the model's classification acumen. Additionally, methods such as Convolutional Neural Networks 11 , 12 , Long Short-Term Memory Networks 13 , 14 , 15 , LightGBM 16 , and the Capsule Network 17 are extensively employed.
With the advancement of big data and the Internet of Things (IoT) technologies, the Digital Twin (DT) 18 technology has paved a new path for enhancing the efficiency of equipment health management. The core concept is to construct a holographic virtual twin model in the digital realm, utilizing advanced technologies such as intelligent sensing and data transmission, which accurately, comprehensively, and in real-time reflect the evolution of physical devices, achieving intelligent control over entities 19 , 20 , 21 . This technology has been extensively utilized in various sectors including aerospace, manufacturing, and healthcare.
In the field of transformer fault diagnosis, scholars both domestically and internationally have carried out extensive research. Referencing 22 , the study proposed a method for constructing a dual-driving twin model integrating data and models, focusing on 10 kv oil-immersed transformers. This approach enables the synchronization between the actual operating conditions of the transformer and the digital twin center. Referencing 23 , a digital twin fault diagnosis model was constructed based on the mechanism model and data model of transformers. Five characteristic gases extracted from DGA data were selected as input feature vectors for a CNN. Experimental results showed that the 1D-CNN model established in this study responded rapidly, had a short training time, and achieved high accuracy, thus validating the effectiveness of the model. Referencing 24 , a fault diagnosis model based on digital twin was constructed for transformers, taking into account their structural characteristics and operational traits. By optimizing the smoothing factor δ in a probabilistic neural network through differential evolution algorithm, the diagnostic accuracy reached an impressive 96.7%, enabling precise monitoring of the transformer's actual operating state. Reference 25 conducts a statistical analysis of the operating data and state information quantity of power transformers, proposes a framework for a state evaluation system and fault detection system based on GCA-CNN, and verifies with 2000 real data cases that the model has higher accuracy and evaluation and detection effects. The literature 26 establishes a high-fidelity simulation model of transformers to accurately simulate winding currents and the temperatures of different components, which can be used for the identification of early faults. However, the aforementioned research is only focused on a single dissolved gas in oil or vibration signal as the basis for fault diagnosis, but there are many factors affecting transformer faults. In the future, it may be possible to combine multi-source data for comprehensive judgment.
In light of the above context, this paper proposes a fault diagnosis method for oil-immersed transformers that integrates a digital twin model. The main contributions of the paper are divided into several parts. Part 1 mainly elaborates on the research background of the paper and the future research direction. Part 2 establishes a transformer digital twin framework, based on geometric, physical, behavioral, and rule models, to achieve interaction mapping between the virtual entity and the physical entity. Part 3 introduces the methods used in the paper, providing theoretical support for the establishment of an accurate and efficient fault diagnosis model. Part 4 addresses the issue of imbalanced small sample data that can easily lead to misjudgment of minority class samples, deeply explores the correlation between dissolved gases in oil and fault types, and eliminates the 'dimensionality catastrophe' problem, using instance data to obtain diagnostic results. Part 5 discusses and analyzes different sampling methods, different features, and different diagnostic models. Part 6 summarizes the entire paper.
Transformer fault diagnosis model fusing digital twin
Transformer digital twin framework.
This article takes a 400kV oil-immersed transformer as the research object and establishes a transformer digital twin integrated digital twin technology. The constructed digital twin framework mainly includes: physical space, twin body, twin data layer and application service layer 27 , as shown in Fig. 1 .
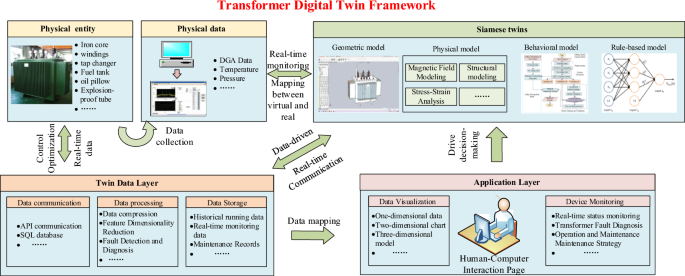
Transformer digital twin framework.
In the process of building a digital twin, the geometric model is the foundation for creating the digital twin model. Three-dimensional software such as UG and SolidWorks are used to comprehensively describe the solid model in terms of geometric dimensions, material properties, and assembly relationships. Based on prior knowledge, physical properties, and operating mechanisms, the geometric model is analyzed and tested for magnetic field, structure, and other modeling aspects, fully reflecting the intrinsic nature and operating mechanism of the transformer. Heterogeneous data from multiple sources, such as dissolved gas in oil and acoustic vibration signals, are collected using state-aware devices. Artificial intelligence algorithms integrated in the behavior model are used for processing and analysis. The derived data generated from simulation calculations are fed back to the mechanism model in real-time. At the same time, simulation data, state-aware data, as well as transformer's full life cycle process data, maintenance records, and computed derived data collectively form the twin database. Through data communication protocols and interfaces, real-time updates and interactive control between the physical entity and the digital twin are achieved, enabling visual description, real-time monitoring, analysis, diagnosis, and intelligent decision-making for the physical transformer. This provides new ideas for improving the safety and reliable operation of power transmission and transformation equipment.
The five-dimensional model of digital twin
The present work is founded on the five-dimensional model proposed by Tao Fei from Beijing Aerospace University 28 , culminating in the creation of a digital twin for transformers, as exemplified by Eq. ( 1 ).
where: PE denotes the physical entity of the transformer, VE represents the virtual entity, SS signifies data, algorithms and models of the digital twin, DD stands for the twinning data of the transformer, and CN symbolizes the interaction and communication among the various components.
The acronym PE stands for transformer physical entity, an ensemble of components including the core, windings, tap-changer, and cooling equipment, it caters to the perception of contact or non-contact by state-sensing devices, embodying the interactive and responsive essence of an objective presence.
The SS represents the process of integrating data and models generated by the digital twin transformer system, thereby facilitating comprehensive monitoring of entities, diagnostic analysis of equipment failures, and predictive maintenance.
VE represents the twin model of the virtual realm, establishing the fundamental groundwork for mapping the virtual to the real. The specific composition is delineated by the formula ( 2 ) shown:
where: Gv represents the geometric model, which uses 3D modeling software to create a comprehensive description of the geometric features of physical entities; Pv represents the physical model, which describes the physical properties and operating mechanisms of electrical equipment; Bv represents the behavior model, which combines artificial intelligence algorithms to create Bv; Rv represents the rule model, which mainly includes expert experience and rule inference based on processed historical data for optimization and deduction.
DD represents twin data, which dynamically stores relevant data of PE/VE/SS, and is an important prerequisite for ensuring intelligent operation and maintenance of transformers. The specific representation is shown in formula ( 3 ):
where: Dp refers to the dynamic factor data collected through the state-aware device; Dv refers to the running parameters in the virtual model; Ds mainly refers to the functional and business service data; Dk includes expert experience, industry rules in the transformer field, and usage guidelines, etc. Df refers to the integrated transformation, interactive fusion, and other derived data of the above-mentioned data.
CN represents the data connection part, which is crucial for ensuring the interaction and updating of the elements in the digital twin model. Through data interfaces, communication protocols, etc., efficient transmission and utilization of data in the digital twin system can be achieved, enabling seamless communication and connectivity among different parts of the model. The interactive relationships of the five dimensions in the digital twin model are shown in Fig. 2 .
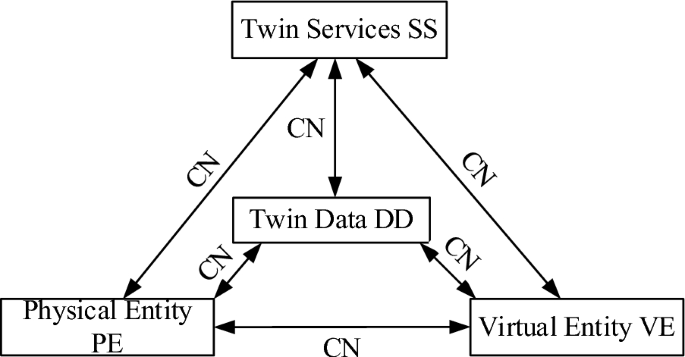
Transformer digital twin five-dimensional model connection relationship.
Transformer fault diagnosis model based on optimized extreme learning machine
Iterative nearest neighbor oversampling algorithm.
The iterative neighborhood oversampling 29 algorithm is a sampling method designed to tackle class imbalance issues, with its principal tenet being the selection of a multitude of class-specific samples as neighbors, and then traversing all k data points within this category, scouring for the most recent unlabeled instance within each label data subset of said category until the dataset balances out or approaches close to it. Here follow the specific steps:
Assume the samples in the dataset for each tag to be \({\text{r}} = \left\{ {r_{1} ,r_{2} , \cdots ,r_{j} , \cdots ,r_{a} } \right\}\) , with \(r_{j} \left( {j = 1,2, \cdots a} \right)\) denoting the number of samples contained within category j . Define the sample set's imbalance factor, utilizing the standard deviation \({\text{var}} \left( r \right)\) to symbolize the dispersal of various types of samples within the dataset, as illustrated in Eq. ( 4 ):
where: \(\mathop r\limits^{ - } = \frac{1}{a}\sum\limits_{j = 1}^{a} {r_{j} }\) .
Based on the philosophy of greedy search, endeavor to identify a multitude of particular sub-samples, with the process detailed in formula ( 5 ):
where: \(x_{j}\) represents the labeled data in category j . If \(x_{\max k}\) is the classification boundary, remove it and select the next nearest neighbor. Then, label it as category j , remove it from the unlabeled data set \(X_{U}\) , add it to the labeled data set \(X_{L}\) , and set \(r_{j} = r_{j} + 1\) . Recalculate the imbalance degree until the preset value is reached, and stop iterating.
Extreme learning machine algorithm
The Kernel Extreme Learning Machine (KELM) 30 is based on a single hidden layer feedforward neural network. It introduces a kernel function on top of the ELM algorithm, which maps low-dimensional data to a high-dimensional feature space, resulting in a model with stronger generalization and robustness. The specific steps are as follows:
Assume we are provided with N samples represented as \(\left\{ {\left( {{\text{x}}_{{\text{i}}} ,t_{i} } \right)} \right\}_{i = 1}^{N}\) , where \(x_{i} = \left[ {x_{i1} ,x_{i2} , \cdots ,x_{in} } \right]^{T} \in R^{n}\) and \(t_{i} = \left[ {t_{i1} ,t_{i2} , \cdots ,t_{im} } \right]^{T} \in R^{n}\) denote the input vector and output function of the model respectively. In the context of a neural network with k hidden layers and an activation function \(g\left( x \right)\) , the number of hidden nodes is L , and the ELM model can be articulated by the formula shown in Eq. ( 6 ):
where: \(\beta_{j} = \left[ {\beta_{j1} ,\beta_{j2} , \cdots ,\beta_{jL} } \right]^{T} \left( {j = 1,2, \cdots ,L} \right)\) denotes the output weight value connecting the j th implicit layer node with the output layer node. Among these, \(H = \left\{ {h_{ij} } \right\}\left( {i = 1,2, \cdots ,N;j = 1,2, \cdots ,L} \right)\) represents the output matrix of the hidden layer, and H denotes the jth column of the input \(x_{1} ,x_{2} , \cdots ,x_{n}\) corresponding to the jth hidden layer node. Within H, the jth row corresponds to the output vector of \(x_{i}\) .
Using the least squares method to obtain the output weight values, as shown in formula ( 7 ):
In the formula, \(H{\prime}\) represents the generalized inverse matrix of the hidden layer output matrix H .
Introducing the kernel function mitigates the issue of randomly generated input weights and bias values, exemplified by the KELM weight output formula ( 8 ):
The KELM output function as expressed in formula ( 9 ):
When \(h\left( x \right)\) remains unknown, the kernel function matrix is represented by formula ( 10 ):
In the equation, \(K\left( {x_{i} ,x_{j} } \right)\) denotes the nuclear function, represented as:
The KELM model's output function expression is delineated in formula ( 12 ):
Sparse principal component analysis
The sparse principal component analysis 31 is a method that builds upon the principal component analysis algorithm by incorporating the LASSO penalty term, thereby enabling the matrix to be sparsely populated. By solving the regression coefficient matrix, it further transforms PCA into an optimization problem aimed at finding the optimal set of coefficients for regression. Compared to traditional PCA, SPCA excels in effectively managing the sparsity within high-dimensional data, yielding results that are more interpretative.
The SPCA algorithm is resolve into two segments: the first entails calculating the principal components via PCA; the second entails enhancing the LASSO penalty term to render the obtained solution sparse. Here follow the specific steps:
Given a \({\text{n}} \times m\) -variant dataset X, the feature decomposition upon normalization treatment, as expounded upon in formula ( 13 ):
In the equation, \(\Lambda \in R^{m \times m}\) represents a diagonal matrix of eigenvalues, arranged in descending order. \(\Lambda \in R^{m \times m}\) is a unitary matrix with column vectors as load vectors.
Select the first k columns of the load matrix \(P \in R^{m \times k}\) , compute the score matrix T , as shown in Eq. ( 14 ):
Projecting T onto X yields a new matrix \(\mathop X\limits^{ \wedge }\) that encompasses information from the corresponding principal component; the difference with X is denoted as E , as illustrated in formula ( 15 ), ( 16 ):
The solution of the SPCA first reverts to the PCA model. The formula ( 15 – 16 ) yields the expression ( 17 ):
Ensure the main component is as near to the original data as possible, that is,it mandates E'sminimalism. Therefore, the principal component seeks resolution through formula ( 18 ):
In the equation, \(\mathop P\limits^{ \wedge }\) is the solution to the minimum value of the principal matrix P .
The vectors sought by PCA are all non-zero; thus, the sparse solution is achieved by incorporating the LASSO penalty term, thereby mitigating the overfitting issue in PCA. The solution formula for sparse principal components, as displayed in formula ( 19 ), is illustrated:
In this equation, matrix A denotes the expected demand matrix to be sought, while matrix B represents the demand matrix expected under the regression problem. A and B represent the \(m \times k\) matrix, \(\mathop A\limits^{ \wedge }\) and \(\mathop B\limits^{ \wedge }\) the matrices to be solved for minimizing values of A and B; they are subject to the constraints \(b_{j} \propto P_{j}\) , \(\lambda\) and \(\lambda_{1,j}\) being the penalty coefficients, and must adhere to \(\lambda > 0\) . The adjusted variance, as expressed in formula ( 20 ), is indicative of:
In the equation, the diagonal matrix interpreting variance is delineated, with \(\mathop P\limits^{ \wedge }\) representing the load matrix following the coefficients. Model contribution lies articulated in formula ( 21 ):
Transformer fault diagnosis model process
This article, established on the premise of transformer fault imbalance within small sample sets, aims at achieving real-time and precise diagnosis through the establishment of a diagnostic model and a determined diagnostic process. The specific diagnostic process is illustrated in Fig. 3 . The article employs the AO-KELM model as the diagnostic model, erecting a diagnostic process that integrates offline model training with online fault identification.
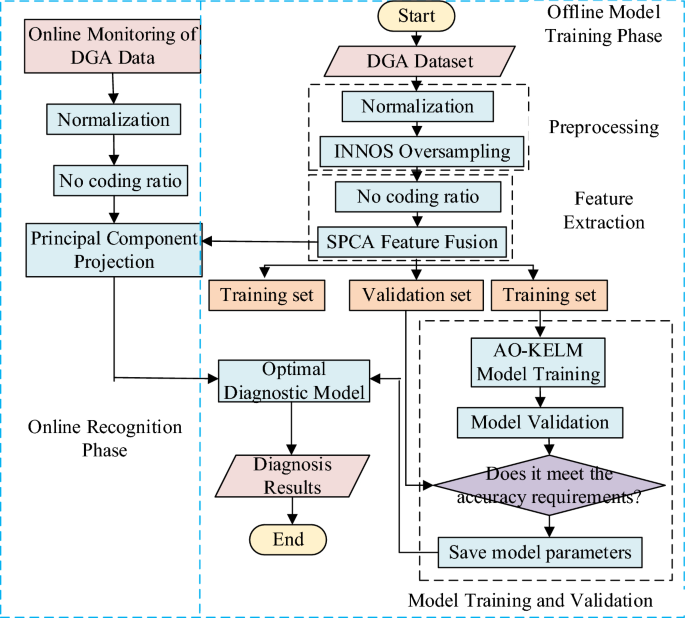
Transformer fault diagnosis model based on optimized kernel extreme learning machine.
⑴ Train the model offline
The article delves into the offline model training segment from three perspectives: data preprocessing, feature extraction, and model recognition.
Step 1: the preprocessing segment encompasses data INNOS's oversampling and normalization treatment. Collect the gathered DGA samples through INNOS for augmenting the minority class samples, followed by normalization treatment.
Step 2: the feature extraction section encompasses the establishment of ratio signature generation and the integration of SPCA for fusion dimensionality reduction. First, construct a multidimensional discriminant signature, delving deeply into the correlation between the ratio of dissolved gas content in oil and the type of fault. Subsequently, employ SPCA for feature fusion to acquire the optimal principal component, thereby removing redundant information, and divide the training set, validation set, and test set proportionally.
Step 3: the model identification segment encompasses the training and validation of the model. Utilizing the AO algorithm to optimize the regularization parameters C and the kernel functions within the KELM model, one verifies the model's accuracy through validation set on each iteration. Should the discrepancy between consecutive training sessions fall beneath 5%, the model training continues; otherwise, the model retraining commences anew until the prerequisite conditions are met. The ultimate establishment of the AO-KELM optimal diagnostic model.
⑵ Online fault diagnosis
Normalize the samples collected in real-time to handle and construct multi-dimensional features, employing an unencoded ratio method to input into an optimal diagnosis model directly following optimal principal component projection, thereby achieving swift recognition of transformer fault. Although the computational time for offline model training is accordingly elevated, it is merely necessary to undergo training once, with the aim of achieving online recognition and diagnosis of transformer faults as data from real-time monitoring continues to be inputted.
Case study analysis
Data source and normalization processing.
Transformer insults are exacerbated by thermal electrochemical action, causing the decomposition of internal insulating materials and the dissolution of various hydrocarbon gases within the insulation oil. Distinct characteristics of gas dissolved in oil under varying fault types exist; research has demonstrated that diagnostic and classification of faults can be achieved through the use of DGA techniques 32 . Consequently, these five gas contents are utilized as a basis for transformer fault diagnosis in this article.
The article selected a comprehensive sample of 337 monitoring data from a particular power supply company, dividing the operating status of transformers into categories such as normal, moderate heat overload, high temperature overload, high energy discharge, low energy discharge, and local discharge, each represented by labels 1 through 6. Each type of fault is augmented with specific characteristic gases including H 2 , CH 4 , C 2 H 4 , C 2 H 6 , and C 2 H 2 ; the exact number of samples for each category is detailed in Table 1 . The data reveals that the majority of samples fall into the category of normal, comprising 35.63% of the total. Low-energy discharge and local discharge types account for 5.55% and 9.78% respectively, with a maximum disparity reaching 5.1:1. Such imbalanced data is prone to misidentifying samples of the minority class as normal, thereby impacting recognition accuracy. Therefore, this paper employs the INNOS algorithm to augment the minority class samples, achieving a balance in sample categories.
To manifest the disparities between data prior to and after sampling, a principal component analysis is conducted upon the sample data from before and after said sampling process. Subsequently, the first two principal components are selected for visualizing the data of various types both before and after said sampling, as illustrated in Fig. 4 . In Fig. 4 , it becomes apparent that the data distribution trends for various types of faults, prior to and after the adoption of the INNOS sampling method, are identical, thereby underscoring the viability of the INNOS sampling approach.
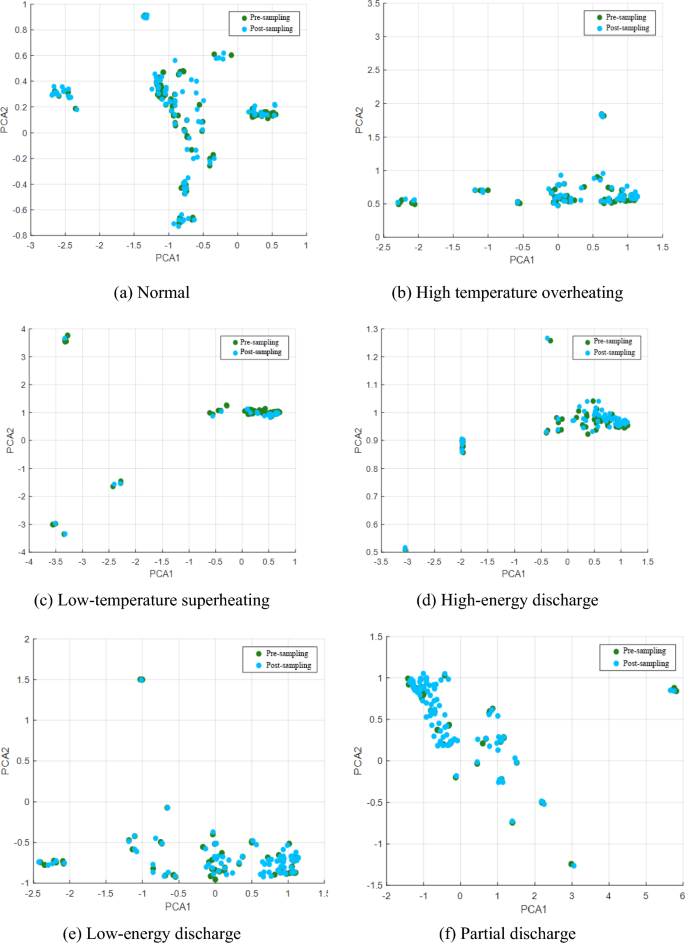
Scatter plot of INNOS samples.
Transformer malfunction signature composition
Considering the substantial disparities among the various volatile gases, a preliminary normalization is required for each gas's abundance, as illustrated in Eq. ( 22 ):
In the equation: \(x_{i}\) and \(x_{{\text{i}}}^{*}\) represent features pre-normalized; \({\text{x}}_{{{\text{i}}\max }}\) and \({\text{x}}_{{{\text{i}}\min }}\) indicate the original minimal and maximum values.
The method of unencoded ratio analysis 33 is but one among numerous techniques widely employed, utilizing the percentage ratio of key gases to either the total gas or the hydrocarbon concentration can profoundly illustrate the interconnectedness between characteristic gases and types of failures. For instance, the ratio of a singular gas to the total hydrocarbon concentration provides a more conclusive indicator of the interplay between diverse fault types; the concentrations of C 2 H 4 and CH 4 can effectively demarcate local discharge from discharge with overheating diagnosis; the percentage composition of C 2 H 2 can determine whether a transformer has experienced thermal failure, among other determinations. The construction of this paper is predicated on the integration of pertinent literature, establishing a nine-dimensional candidate ratio signature for transformer fault diagnosis 31 , as delineated in Table 2 , wherein THC = CH 4 + C 2 H 4 + C 2 H 6 + C 2 H 2 , and ALL = H 2 + CH 4 + C 2 H 4 + C 2 H 6 + C 2 H 2 .
Dimensionality reduction through feature parameter fusion
To avoid the redundancy of fault-related feature information within the samples and to enhance the efficiency and precision of the diagnostic model, the SPCA method was employed for the integration of the derived rational features. The cumulative explicable variance contribution rate of each principal component is depicted in Fig. 5 . It is evident from Fig. 5 that the cumulative variance contribution rate for the first six principal components reaches 90.4419%, indicating that the first five principal components can achieve more than 90% of the ability expressed by all the principal components. Hence, selecting these five principal components as inputs for the transformer fault diagnosis model is warranted.
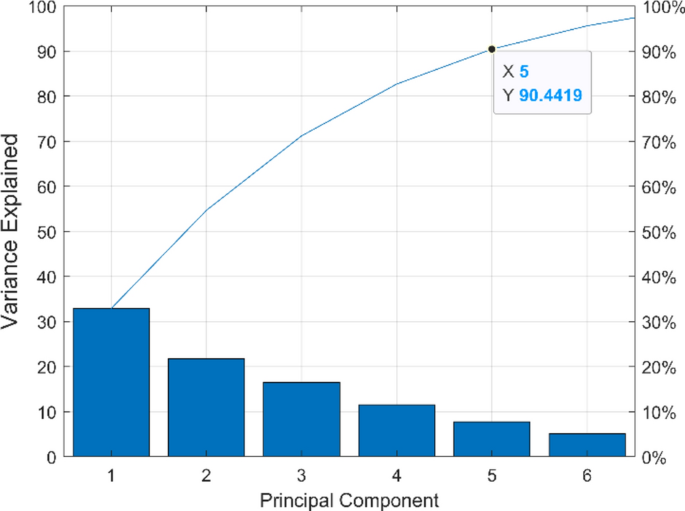
Cumulative variance contribution rate.
Transformer malfunction diagnosis outcomes
The fused features derived from the SPCA extraction are delineated in a ratio of 6:2:2 to be divided into training, testing, and validation datasets. The regularization parameters C within KELM determine the learning capacity of the model and its diagnostic precision; in this paper, we employ the AO optimization algorithm to optimize C, with an introduction of the AO algorithm as delineated in literature 34 , 35 , culminating in the establishment of a diagnostic model based on SPCA-AO-KELM. Figure 6 delineates the confusion matrix diagram of the transformer fault diagnosis. It is evident from Fig. 6 that within the test set of 158 samples, 155 were correctly diagnosed, representing a total correct rate of 98.1013%. The accuracy rates for normal, high-temperature overheating, and low-energy discharge diagnoses are 100%, one case of misjudgment was found in medium–low temperature overheating, high-energy discharge, and partial discharge.
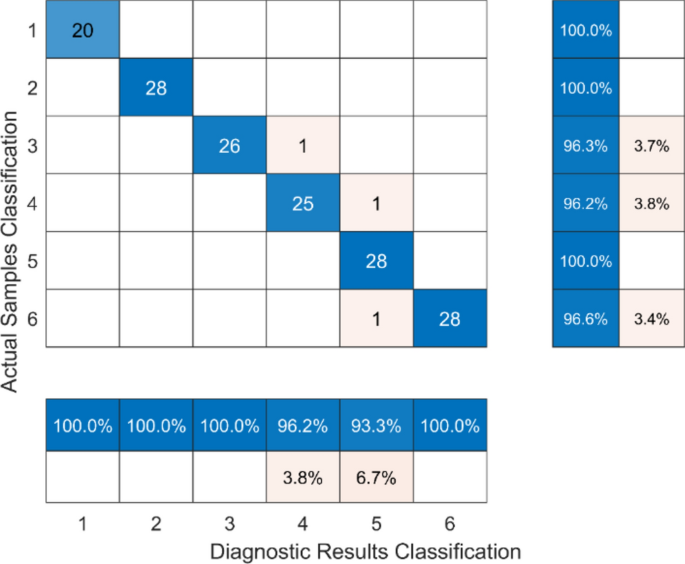
Transformer fault diagnosis results.
However, the precision of diagnostic accuracy alone cannot comprehensively nor efficaciously evaluate the impact of rare class faults on classification performance 36 , 37 . In this paper, we introduce classification model performance evaluation metrics derived from confusion matrices, employing accuracy (R), precision (P), and F1-score as the core components of our evaluation system. The veracity of diagnostic models for identifying various faults is assessed by the accuracy rate, the sensitivity of the model in recognizing a variety of faults is evaluated by the coverage rate, while the F1 score derived from the amalgamation of precision and recall reflects the model's classification performance amidst sample imbalance, with specific formulas denoted in the literature displayed here. The model's precision, recall, and F1-score derived from the computed graph in Fig. 6 respectively stand at 0.9816, 0.9825, and 0.9820, further underscoring the model's high fault detection accuracy and its stable nature.
Results and discussions
Comparison and analysis of different sampling methods.
To verify the effectiveness of the new samples synthesized based on INNOS in improving the accuracy of transformer fault diagnosis, this paper uses unbalanced data set, random oversampling, SMOTE, and ADASYN oversampling algorithms for sample augmentation, and the diagnostic results are shown in Fig. 7 . The red dots in the figure represent the samples that are correctly classified in the test set, while the circles represent the samples of the true class, and the scattered dots represent the samples that are misclassified as other classes. The more scattered sample points, the higher the misclassification rate. In Fig. 7 d, the diagnostic accuracy of the original unbalanced data set without balancing processing is only 88.4058%, indicating that due to the imbalance of data in each fault category, the training of the diagnostic model is insufficient, and it is easy to misclassify minority class samples as majority class samples during classification recognition. After balancing the data set using different sampling methods, the misclassification rate of the samples decreases. The sampling method used in this paper improves the diagnostic accuracy by 7.7967%, 2.5316%, and 1.8987% compared to ADASYN, SMOTE, and random oversampling, respectively, indicating that the INNOS sampling method can effectively solve the problem of low diagnostic accuracy caused by data imbalance.

Diagnostic results under different sampling methods.
Qualitative and quantitative analysis with integrated features
To demonstrate the effectiveness of the SPCA feature fusion method, this study conducted analysis from two perspectives: qualitative observation and quantitative analysis. Firstly, PCA, KPCA, and SPCA were used to extract features from the constructed ratio signs. The cumulative variance contribution rate threshold was set at 90%, and the obtained principal component information is detailed in Table 3 . LASSO penalty term was introduced based on PCA to constrain some loading vectors to zero, resulting in a loss of variance contribution rate. From the data in the table, it can be seen that the contribution rate of SPCA principal components is slightly lower than that of PCA and KPCA, effectively removing redundant information in the ratio features and providing a valid data foundation for subsequent classification and recognition.
Furthermore, for the above feature extraction methods, quantitative calculations were performed. The fused features extracted by the 9-dimensional joint feature, PCA, KPCA, and SPCA were input into the diagnostic model for comparative analysis, as shown in Fig. 8 . From Fig. 8 a–d, it can be observed that the diagnostic accuracy is significantly improved after feature extraction. Figure 8 a has a higher accuracy compared to Fig. 8 b and c, which validates the superiority of the SPCA feature extraction method.
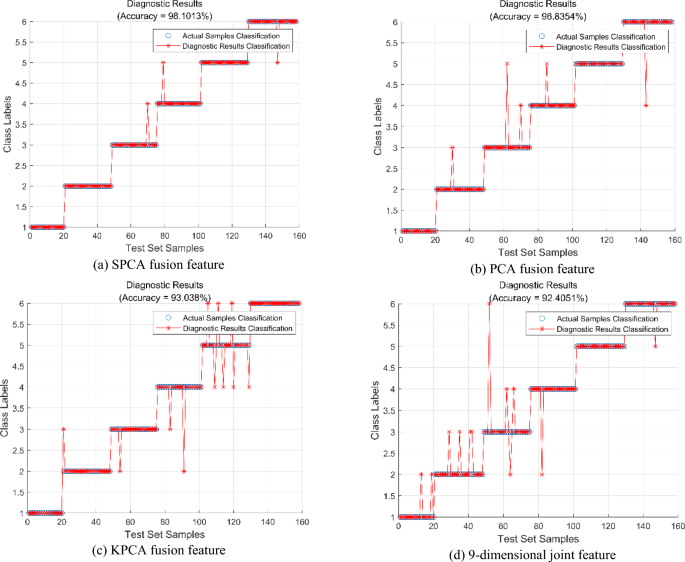
Diagnostic outcomes under various characteristics.
Analysis of contrastive diagnostic models
To explore the diagnostic performance of the models, three diagnostic models, ELM, KELM, and AO-ELM, were constructed for horizontal comparison. The diagnostic results are shown in Table 4 . From the perspective of a single model, the introduction of a kernel function improved the diagnostic accuracy and evaluation indicators of ELM. From the perspective of optimization algorithms, the diagnostic capability of fault recognition was effectively improved after parameter optimization using the AO algorithm.
On the other hand, the extracted integration features are respectively inputted into the POA-SVM model proposed in Literature 38 , the SSA-ELM model suggested in Literature 39 , and the PSO-BiLSTM model introduced in Literature 40 for longitudinal comparison. To circumvent the chances of chance, each model is subjected to ten-fold cross-validation, as manifested in Table 5 . It is evident from Table 5 that, under conditions where the input features remain identical, the AO-KELM outperforms both the POA-SVM and POA-SVM by elevating the average accuracy by 3.23% and 2.64%, respectively, while the PSO-BiLSTM lags behind with a mere 1.8% increase in accuracy. This clearly signifies the robust stability of the AO-KELM model and its formidable classification capabilities.
The paper introduces an oil-immersed transformer fault diagnosis method that integrates digital twin models, providing validation through case studies, leading to the conclusions below:
Build a twin mechanism model based on geometric, physical, rule, and behavior models, use real-time data to drive the fusion of data and mechanism models, complete real-time mapping between physical entities and virtual entities, and use visualization technology to express the twin in multiple dimensions, achieve intelligent diagnosis, health monitoring, and optimization decision-making for the transformer entity.
Proposed a transformer fault diagnosis model based on optimized kernel extreme learning machine, which solves the problem of misjudgment of minority class samples caused by unbalanced small samples, effectively extracts fusion features, establishes the optimal AO-KELM classifier, and achieves an accuracy of 98.1013%. By comparing with different diagnostic models, the classification performance and stability of the proposed method are verified.
Data availability
The datasets generated and/or analysed during the currentstudy are not publicly availabledue [REASON WHY DATA ARENOT PUBLlC] but are availablefrom the corresponding authoron reasonable request. E-mail:[email protected].
Tightiz, L. et al. An intelligent system based on optimized ANFIS and association rules for power transformer fault diagnosis. ISA Trans. 103 , 63–74 (2020).
Article PubMed Google Scholar
Zhang, Y. et al. Fault diagnosis of transformer using artificial intelligence: A review. Front. Energy Res. 10 , 1006474 (2022).
Article Google Scholar
Wani, S. A. et al. Advances in DGA based condition monitoring of transformers: A review. Renew. Sustain. Energy Rev. 149 , 111347 (2021).
Article CAS Google Scholar
Malik, H. & Mishra, S. Application of gene expression programming (GEP) in power transformers fault diagnosis using DGA. IEEE Trans. Ind. Appl. 52 (6), 4556–4565 (2016).
Lin, J., Ma, J. & Zhu, J. Hierarchical federated learning for power transformer fault diagnosis. IEEE Trans. Instrum. Meas. 71 , 1–11 (2022).
Google Scholar
Duval, M. A review of faults detectable by gas-in-oil analysis in transformers. IEEE Electr. Insul. Mag. 18 (3), 8–17 (2002).
Li, P. & Hu, G. M. Transformer fault diagnosis based on data enhanced one-dimensional improved convolutional neural network. Power Syst. Technol. 47 (07), 2957–2967 (2023).
Zhou, X. H. et al. Transformer fault diagnosis based on SVM optimized by the improved bald eagle search algorithm. Power Syst. Prot. Control 51 (08), 118–126 (2023).
Chen, H. C., Zhang, Y. & Chen, M. Transformer dissolved gas analysis for highly-imbalanced dataset using multi-class sequential ensembled ELM. IEEE Trans. Dielectr. Electr. Insulat. https://doi.org/10.1109/TDEI.2023.3280436 (2023).
Gong, Z. W. Y. et al. Fault diagnosis method of transformer based on improved particle swarm optimization XGBoost. High Volt. Appar. 59 (08), 61–69 (2023).
Xu, H. R. & Wang, Z. Y. Condition evaluation and fault diagnosis of power transformer based on GAN-CNN. J. Electrotechnol. Electr. Eng. Manag. 6 (3), 8–16 (2023).
Wang, Z. & Xu, H. GCA-CNN based transformer digital twin model construction and fault diagnosis and condition evaluation analysis. Acad. J. Comput. Inf. Sci. 6 (6), 100–107 (2023).
MathSciNet Google Scholar
Wang, L., Littler, T. & Liu, X. Dynamic incipient fault forecasting for power transformers using an LSTM model. IEEE Trans. Dielectr. Electr. Insulat. https://doi.org/10.1109/TDEI.2023.3253463 (2023).
Ding, Y. et al. A novel time–frequency Transformer based on self-attention mechanism and its application in fault diagnosis of rolling bearings. Mech. Syst. Signal Process. 168 , 108616 (2022).
Zheng, Q. et al. A real-time transformer discharge pattern recognition method based on CNN-LSTM driven by few-shot learning. Electr. Power Syst. Res. 219 , 109241 (2023).
Yan, P. et al. Transformer fault diagnosis research based on LIF technology and IAO optimization of LightGBM. Anal. Methods 15 (3), 261–274 (2023).
Yang, D. C. et al. Fault diagnosis of transformer based on capsule network. High Volt. Eng. 47 (02), 415–425 (2021).
Grieves, M. & Vickers, J. Digital twin: Mitigating unpredictable, undesirable emergent behavior in complex systems. In Transdisciplinary Prespectives on Complex Systems (eds Kahlen, F.-J. et al.) 85–113 (Springer International Publishing, 2017).
Chapter Google Scholar
Bai, X. Z. et al. Selection method of feature derived from dissolved gas in oil for transformers fault diagnosis. High Volt. Eng. 49 (09), 3873–3886 (2023).
Liu, Y. P. et al. Application prospect and key technology of digital twin in power transmission and transformation equipment. High Volt. Eng. 48 (05), 1621–1633 (2022).
Yang, F. et al. Application and implementation method of digital twin in electric equipment. High Volt. Eng. 47 (05), 1505–1521 (2021).
Jiang, L. et al. Research on transformer fault diagnosis method based on digital twin. J. Syst. Simulat. https://doi.org/10.16182/j.issn1004731x.joss.23-1402 (2024).
Yan, Z. J. & Yang, Y. F. Fault diagnosis of transformers based on CNN and digital twin. Comput. Digit. Eng. 51 (11), 2758–2762 (2023).
Wang, Y. & Zhang, T. H. Fault diagnosis of transformers based on optimal probabilistic neural network based on digital twin. Mod. Mach. Tool Autom. Manuf. Techn. 11 , 20–23 (2020).
Moutis, P. & Alizadeh-Mousavi, O. Digital twin of distribution power transformer for real-time monitoring of medium voltage from low voltage measurements. IEEE Trans. Power Deliv. 36 (4), 1952–1963 (2020).
Zhang, L. J. et al. Study on electrothermal characteristics of oil-immersed power transformers in early stage of interturn faults. Proc. CSEE 43 (15), 6124–6136 (2023).
Tao, F. et al. Five-dimension digital twin model and its ten applications. Comput. Integr. Manuf. Syst. 25 (01), 1–18 (2019).
Li, S. W. et al. Application of data feature selection and classification in mechanical fault diagnosis. J. Vibrat. Shock 39 (02), 218–222 (2020).
CAS Google Scholar
Han, X. et al. A novel power transformer fault diagnosis model based on Harris-Hawks-optimization algorithm optimized kernel extreme learning machine. J. Electr. Eng. Technol. 17 (3), 1993–2001 (2022).
Kong, D. M. et al. Research on oil identification method based on three-dimensional fluorescence spectroscopy combined with sparse principal component analysis and support vector machine. Spectroscopy Spectral Anal. 41 (11), 3474–3479 (2021).
Kim, S. W. et al. New methods of DGA diagnosis using IEC TC 10 and related databases part l: Application of gas-ratio combinations. IEEE Trans. Dielectr. Electr. Insulat. 20 (2), 685–690 (2013).
Guo, R. Y., Peng, M. M. & Cao, Z. Q. Fault diagnosis of power transformer based on SE-DenseNet. Adv. Technol. Electr. Eng. Energy 40 (01), 61–69 (2021).
Wang, K. et al. New features derived from dissolved gas Analysis for fault diagnosis of power transformers. Proc. CSEE 36 (23), 6570–6578+6625 (2016).
Li, G. L. et al. Thermal error model of spindle for precision CNC machine tool based on AO-CNN. J. Xi’an Jiaotong Univ. 56 (08), 51–61 (2022).
Zhang, C. S. et al. improved aquila optimization based on multi-strategy integration. Acta Electron. Sin. 51 (05), 1245–1255 (2023).
Wang, Y. et al. Transformer fault diagnosis fused with synthetic minority over-sampling balanced multi-classification data based on improved extreme learning machine. Power Syst. Technol. 47 (09), 3799–3807 (2023).
Tang, J. et al. Oversampling and cost⁃sensitive algorithm for transformer fault diagnosis with unbalanced samples. High Volt. Apparatus 59 (06), 93–102 (2023).
Liu, D. D. et al. POA-SVM transformer fault diagnosis based on ADASYN balanced data set. Power Syst. Clean Energy 39 (08), 36–44 (2023).
Wang, Y. et al. Transformer DGA fault diagnosis method based on DBN-SSAELM. Power Syst. Prot. Control 51 (04), 32–42 (2023).
Fan, Q. C., Yu, F. & Xuan, M. Power transformer fault diagnosis based on optimized Bi-LSTM model. Comput. Simul. 39 (11), 136–140 (2022).
Download references
Acknowledgements
Project supported by Jilin Provincial Development and Reform Commission innovation capacity construction fund (2020C022-6).
Author information
Authors and affiliations.
Hangzhou Electric Power Equipment Manufacturing Co. Ltd Yuhang Qunli Complete Sets Electricity Manufacturing Branch Electric, Hangzhou, 311000, China
Haiyan Yao, Qiang Guo & Yufeng Miao
Hangzhou Electric Power Equipment Manufacturing Co. Ltd., Hangzhou, 311000, China
Northeast Electric Power University School of Mechanic Engineering, Jilin, 132012, China
You can also search for this author in PubMed Google Scholar
Contributions
Haiyan Y designed the experiments and contributedmaterials/analysis tools; Xin Zhang analyzed the data and its visualization; Qiang Guo and Yufeng Miao M guided the data analysis; Shan Guan wrote the paper; All authors have reviewed the manuscript.
Corresponding author
Correspondence to Shan Guan .
Ethics declarations
Competing interests.
The authors declare no competing interests.
Additional information
Publisher's note.
Springer Nature remains neutral with regard to jurisdictional claims in published maps and institutional affiliations.
Rights and permissions
Open Access This article is licensed under a Creative Commons Attribution-NonCommercial-NoDerivatives 4.0 International License, which permits any non-commercial use, sharing, distribution and reproduction in any medium or format, as long as you give appropriate credit to the original author(s) and the source, provide a link to the Creative Commons licence, and indicate if you modified the licensed material. You do not have permission under this licence to share adapted material derived from this article or parts of it. The images or other third party material in this article are included in the article’s Creative Commons licence, unless indicated otherwise in a credit line to the material. If material is not included in the article’s Creative Commons licence and your intended use is not permitted by statutory regulation or exceeds the permitted use, you will need to obtain permission directly from the copyright holder. To view a copy of this licence, visit http://creativecommons.org/licenses/by-nc-nd/4.0/ .
Reprints and permissions
About this article
Cite this article.
Yao, H., Zhang, X., Guo, Q. et al. Fault diagnosis method for oil-immersed transformers integrated digital twin model. Sci Rep 14 , 20355 (2024). https://doi.org/10.1038/s41598-024-71107-w
Download citation
Received : 20 May 2024
Accepted : 26 August 2024
Published : 02 September 2024
DOI : https://doi.org/10.1038/s41598-024-71107-w
Share this article
Anyone you share the following link with will be able to read this content:
Sorry, a shareable link is not currently available for this article.
Provided by the Springer Nature SharedIt content-sharing initiative
- Transformer fault diagnosis
- Digital twin
- Imbalanced small sample
By submitting a comment you agree to abide by our Terms and Community Guidelines . If you find something abusive or that does not comply with our terms or guidelines please flag it as inappropriate.
Quick links
- Explore articles by subject
- Guide to authors
- Editorial policies
Sign up for the Nature Briefing newsletter — what matters in science, free to your inbox daily.

This paper is in the following e-collection/theme issue:
Published on 3.9.2024 in Vol 26 (2024)
This is a member publication of University of Oxford (Jisc)
Value of Engagement in Digital Health Technology Research: Evidence Across 6 Unique Cohort Studies
Authors of this article:

Original Paper
- Sarah M Goodday 1, 2 , MSc, PhD ;
- Emma Karlin 1 , MSc ;
- Alexa Brooks 1 , MS, RD ;
- Carol Chapman 3 , MPH ;
- Christiana Harry 1 , MPH ;
- Nelly Lugo 1 , BS ;
- Shannon Peabody 1 , BA ;
- Shazia Rangwala 4 , MPH ;
- Ella Swanson 1 , BS ;
- Jonell Tempero 1 , BS, MS ;
- Robin Yang 1 , MS ;
- Daniel R Karlin 1, 5, 6 , MA, MD ;
- Ron Rabinowicz 7, 8 , MD ;
- David Malkin 7, 9 , MD ;
- Simon Travis 10 , Prof Dr ;
- Alissa Walsh 10 , MD ;
- Robert P Hirten 11 , MD ;
- Bruce E Sands 11 , MS, MD ;
- Chetan Bettegowda 12 , MD, PhD ;
- Matthias Holdhoff 13 , MD, PhD ;
- Jessica Wollett 12 , MS ;
- Kelly Szajna 12 , BSc, RN ;
- Kallan Dirmeyer 12 , BS ;
- Anna Dodd 14 , MS ;
- Shawn Hutchinson 14 , MS ;
- Stephanie Ramotar 14 , BSc ;
- Robert C Grant 14 , MD, PhD ;
- Adrien Boch 15 , MA ;
- Mackenzie Wildman 16 , PhD ;
- Stephen H Friend 2, 4 , MD, PhD
1 4YouandMe, Seattle, WA, United States
2 Department of Psychiatry, University of Oxford, Oxford, United Kingdom
3 Crohn's & Colitis Foundation, New York, NY, United States
4 Section of Urology and Renal Transplantation, Virginia Mason Francisan Health, Seattle, WA, United States
5 MindMed Inc, New York, NY, United States
6 Tufts University School of Medicine, Boston, MA, United States
7 Department of Paediatrics, University of Toronto, Toronto, ON, Canada
8 Department of Pediatric Hematology/Oncology, Schneider Children's Medical Center of Israel, Petach-Tikva, Israel
9 Department of Pediatrics, University of Toronto, Toronto, ON, Canada
10 Gasteroentology Unit, Oxford University Hospitals NHS Foundation Trust and Biomedical Research Centre, Oxford, United Kingdom
11 The Dr. Henry D. Janowitz Division of Gastroenterology, Icahn School of Medicine at Mount Sinai, New York, NY, United States
12 Department of Neurosurgery, Johns Hopkins University School of Medicine, Baltimore, MD, United States
13 The Sidney Kimmel Comprehensive Cancer Center at Johns Hopkins, Baltimore, MD, United States
14 Princess Margaret Cancer Center, University Health Network, Toronto, ON, Canada
15 Evidation Health Inc, Santa Mateo, CA, United States
16 Sage Bionetworks, Seattle, WA, United States
Corresponding Author:
Sarah M Goodday, MSc, PhD
2901 3rd Ave
Seattle, WA, 98121
United States
Phone: 1 (206) 928 8243
Email: [email protected]
Background: Wearable digital health technologies and mobile apps (personal digital health technologies [DHTs]) hold great promise for transforming health research and care. However, engagement in personal DHT research is poor.
Objective: The objective of this paper is to describe how participant engagement techniques and different study designs affect participant adherence, retention, and overall engagement in research involving personal DHTs.
Methods: Quantitative and qualitative analysis of engagement factors are reported across 6 unique personal DHT research studies that adopted aspects of a participant-centric design. Study populations included (1) frontline health care workers; (2) a conception, pregnant, and postpartum population; (3) individuals with Crohn disease; (4) individuals with pancreatic cancer; (5) individuals with central nervous system tumors; and (6) families with a Li-Fraumeni syndrome affected member. All included studies involved the use of a study smartphone app that collected both daily and intermittent passive and active tasks, as well as using multiple wearable devices including smartwatches, smart rings, and smart scales. All studies included a variety of participant-centric engagement strategies centered on working with participants as co-designers and regular check-in phone calls to provide support over study participation. Overall retention, probability of staying in the study, and median adherence to study activities are reported.
Results: The median proportion of participants retained in the study across the 6 studies was 77.2% (IQR 72.6%-88%). The probability of staying in the study stayed above 80% for all studies during the first month of study participation and stayed above 50% for the entire active study period across all studies. Median adherence to study activities varied by study population. Severely ill cancer populations and postpartum mothers showed the lowest adherence to personal DHT research tasks, largely the result of physical, mental, and situational barriers. Except for the cancer and postpartum populations, median adherences for the Oura smart ring, Garmin, and Apple smartwatches were over 80% and 90%, respectively. Median adherence to the scheduled check-in calls was high across all but one cohort (50%, IQR 20%-75%: low-engagement cohort). Median adherence to study-related activities in this low-engagement cohort was lower than in all other included studies.
Conclusions: Participant-centric engagement strategies aid in participant retention and maintain good adherence in some populations. Primary barriers to engagement were participant burden (task fatigue and inconvenience), physical, mental, and situational barriers (unable to complete tasks), and low perceived benefit (lack of understanding of the value of personal DHTs). More population-specific tailoring of personal DHT designs is needed so that these new tools can be perceived as personally valuable to the end user.
Introduction
Wearable digital health technologies (DHTs) [ 1 , 2 ] and mobile apps facilitate the remote, real-world assessment of health including objective signs of disease that are typically confined to health care visits and health care provider interpretation. These specific categories of DHTs, herein referred to as “personal DHTs,” hold promise for transforming health research through the new ability to capture high-resolution, high-frequency, in-the-moment health-related multimodal information in decentralized ways. Through the provision of personal DHTs in clinical care, individuals could be better empowered to navigate their health outside the health care system with greater accessibility, agency, and accuracy than currently possible [ 1 , 2 ]. One of the largest challenges in the future of digital health that involves the use of personal DHTs is end-user engagement. While direct comparisons of engagement in personal DHT research are challenging due to the heterogeneous reporting of retention and adherence factors, and a lack of consensus on a definition of “engagement” [ 3 - 6 ], accumulating evidence supports that so far engagement in the use of personal DHTs has been poor. Specifically, retention in personal DHT research studies and the use of health-related apps is low across diverse populations and applications [ 7 - 9 ]. Further, there is evidence of attrition biases in personal DHT research resulting in insufficient representation of minority populations [ 7 ]. In addition to poor retention, personal DHT research studies have low adherence to completing active app-based tasks resulting in large amounts of missing data. This missing data problem results in challenges in artificial intelligence models from insufficient volumes of data to follow individual patterns, and limits app-based context “label” data. This “label” data is crucial for validating passively collected information from personal DHTs, particularly given the early state of the field and as the utility of certain approaches such as knowledge graphs and large language models emerge.
Several personal DHTs health research studies have started to surface [ 7 - 12 ], resulting in the identification of barriers to engagement. These barriers include technical problems with the technology and in collecting the data, usability, privacy concerns, and digital literacy. Many of these barriers point to a need to retain a human element in the research process, and to include an aspect of co-designing with end users. Emerging personal DHT research studies that show better engagement retain some form of “human-in-the-loop” (regular contact with research staff) and co-design or end-user approach [ 11 - 15 ]. Among these studies, retention rates of 80% and higher have been observed, while average adherence to wearable device use and daily app surveys have been shown to be >90% and 70%, respectively [ 11 - 15 ].
The promise of digital health rests on the assumption that end users can be engaged in the long-term use of personal DHTs for health monitoring, yet this remains to be seen among most existing research applications. There have been increasing international calls for the inclusion of patients in the design and conduct of health research [ 16 - 18 ], and this seems particularly relevant for digital health research where the patient is the end user of these new remote tools. In this paper, we report on engagement across 6 unique personal DHT health research studies that adopted different aspects of a participant-centric design, but each with distinct population and design features. The objective is to describe how participant engagement techniques and different personal DHT designs affect participant adherence, retention, and overall engagement in personal DHT health research.
Study Design
In total, 6 personal DHT research studies are included in this quantitative and qualitative analysis of engagement that span diverse populations including a frontline health care population (the stress and recovery in frontline health care workers study) [ 11 ]; a conception, pregnancy, and postpartum population (Better Understanding the Metamorphosis of Pregnancy [BUMP] study) [ 19 ]; and populations with different diseases including Crohn disease (stress in Crohn: forecasting symptom transitions study), Li-Fraumeni syndrome (stress and LFS: a feasibility study of wearable technologies to detect stress in families with LFS), and patients with pancreatic and central nervous system (CNS) tumors (help enable real-time observations [HERO] in pancreatic [PANC] and CNS tumors studies) [ 20 ].
All of these studies were conducted by 4YouandMe—a US-based nonprofit (charitable) organization. 4YouandMe specializes in open-source research into the application of personal DHTs for health and wellness [ 20 ]. 4YouandMe has a particular focus on leveraging personal DHTs to empower the patient in navigating their unique disease or life transitional period. These 6 studies were included in this analysis as they reflect all of the completed studies by 4YouandMe at the time of this analysis. Characteristics of these studies can be found in Table 1 and additional methodological detail can be found in Multimedia Appendix 1 . All studies involved the use of a bespoke study smartphone app built by 4YouandMe and the use of the Oura smart ring, the Garmin smartwatch, the Apple smartwatch, an Empatica smartwatch, and the Bodyport Cardiac Scale. Details of these devices can be found in Multimedia Appendix 2 ).
Study and population | Sample size | Age (years), median (IQR) | Active study time (months) | Recruitment | Devices | Average (SD) app daily burden | Compensation | Engagement strategy | |
Frontline health care workers | 365 | 33.0 (28.0-42.0) | 4-6 | Remote: Social media and health care organization newsletters | 5 (1.8) minutes | None (participants completing the study kept the wearable devices) | |||
Patients with Crohn disease | 195 (MSSM , N=139; Oxford, N=56) | MSSM (median 29, IQR 24-37), Oxford (median 39, IQR 32-50) | 6-9 | In-clinic: through inflammatory bowel disease clinics | 7.7 (1.0) minutes | Yes, participants could keep the ring or receive compensation based on points accumulated | |||
Patients with CNS tumors | 12 | 52 (43-56) | 7 | In-clinic: through cancer specialty clinics | 5.3 (2.1) minutes | None (participants completing the study kept the wearable devices) | |||
Patients with pancreatic cancer | 26 | 57 (53-65) | 1 to 14 months | In-clinic: through cancer specialty clinics | 3.1 (1.9) minutes | None (participants completing the study kept the wearable devices) | |||
Affected and unaffected family members of a proband with LFS | 49 | 39.0 (7.9-68.0) | 6 | In-clinic: through cancer specialty clinics | 2.3 (0.9) minutes | None | |||
Pregnant individuals (up to 15 weeks) | 524 | 33.0 (30-36) | Up to 12 months | Remote: through patient-provider portals, social media, and community health clinics | 5.0 (2.3) minutes | Yes, participants received compensation based on study points accumulated | |||
Individuals actively attempting to get pregnant | 273 | 34.0 (31-36) | Up to 6 months | Remote: through patient-provider portals, social media, and community health clinics | 3.8 (2.0) minutes | Yes, participants could keep the ring or receive compensation |
a MSSM: Mount Sinai School of Medicine.
b HERO-CNS: help enable real-time observations—central nervous system.
c CNS: central nervous system.
d HERO-PANC: help enable real-time observations—pancreatic cancer.
e n=24, 2 unknown.
f Until withdrawal, progression, death, or study completion (October 31, 2022).
g LFS: Li-Fraumeni syndrome.
h BUMP: Better Understanding the Metamorphosis of Pregnancy.
i BUMP-C: Better Understanding the Metamorphosis of Pregnancy—Conception.
Ethical Considerations
All included studies were approved by the local institutional research ethics boards (REB) at their local sites ( Multimedia Appendix 1 ): stress and recovery in frontline health care workers study (institutional review board [IRB], Advarra [4UCOVID1901, Pro00043205]), BUMP study (IRB Advarra Pro00047893), stress in Crohn (Oxford site: Hampshire-A IRAS ID: 269286, Mount Sinai School of Medicine [MSSM] site: IRB of MSSM: GCO 19-1543 | IRB-19-02298), stress and LFS (Sick Kids: REB: 1000072240), HERO-CNS (John Hopkins Medicine IRB IRB00253818), and HERO-PANC (University Hospital Network REB: 20-5211).
Statistical Analysis
Definitions of adherence in digital health research studies are heterogeneous [ 3 - 6 ]. Consistent criteria for adherence across all included studies were attempted. While many different wearable features could be used as the basis for the use of the device, features that were most reliably monitored were selected. For studies using the Oura smart ring, daily adherence was defined as at least one sleep data event present for the prior night. The Oura ring was only expected to be worn at night for many of the included studies, which is why sleep data were used as the indicator for adherence. For studies using the Garmin smartwatch, daily adherence was defined as step data present for that day. For the Empatica smartwatch, daily adherence was defined as at least one data event (worn properly in a day). Adherence to the Bodyport Cardiac Scale was defined as the proportion of days where a weight event was present divided by the total number of expected follow-up days. Adherence to in-app task completion was defined as the proportion of tasks completed when prompted in the app divided by the total number of tasks that should have been completed over study follow-up. For example, all included studies had a daily survey. In a study with a minimum of 4 months of follow-up expected from participants, the total number of expected daily surveys is approximately 120. For a weekly app survey, the total number of expected surveys for a 4-month study follow-up would be 16. Adherence to biweekly check-in calls was defined as the proportion of calls completed divided by the total number of expected calls over study follow-up. Medians and ranges are described since the adherence distributions were nonnormally distributed. All adherence estimations were performed only among retained participants.
Differences in adherence and retention by sociodemographic characteristics were estimated using χ 2 , Fisher exact, Mann-Whitney U , and ANOVA tests where appropriate among studies that have sufficient sample sizes (stress and recovery, BUMP, and stress in Crohn). Survival probabilities using the Kaplan-Meier approach were calculated to display the probability of retention over the course of each included study. Retention (total proportion of participants completing the study among all enrolled) is also reported. Additional information on how retention was calculated for each unique study can be found in Multimedia Appendix 3 .
Description of Included Studies
Study design characteristics of all studies are described in Table 1 . All studies included the use of at least one wearable device plus a study app that involved daily, as well as intermittent surveys (daily question prompts, validated questionnaires) and active tasks (cognitive active or physical function tasks [eg, walk tests], video diaries). In all included studies, participants were required to use their own Android or iPhone smartphone for study activities. Recruitment mechanisms differed across studies with some including remote recruitment through digital advertisements on social media, professional organizations and newsletters, and patient portals (stress and recovery, and BUMP), while others recruited patients in-person through specialty clinics (stress in Crohn, HERO studies, and stress and LFS). The daily burden of app active tasks across studies ranged from 2 to 7 minutes. Study follow-up periods across studies ranged from 4 to 18 months. Across all studies except the stress and LFS study, participants were offered to keep some of the study wearable devices (most often the ring and the watch). Further, 2 studies included the option for modest financial compensation (BUMP and stress in Crohn).
All studies included an engagement strategy that centered around a biweekly phone check-in with a consistent engagement specialist that served the purpose of supporting participants, helping them with onboarding, resolving potential technological problems, and discussing and collecting study experience feedback. Additionally, all included studies implemented different strategies that focused on working with participants as co-designers. These strategies included making app changes that were driven by direct participant feedback during active follow-up, offering a “your data” section in the app that allowed participants to track key symptoms over time, hosting optional investigator-participant Zoom calls where participants could meet the study team, receive study updates, preliminary results, and could offer more feedback, and inviting participants to contribute to and be listed as coauthors on published work.
Adherence by Study Population
Median adherence in engagement phone check-in calls, wearable device use, daily app survey completion, and in-app active tasks can be found in Table 2 . Median adherence varied across study populations. The stress in Crohn–MSSM site had a lower adherence on the engagement check-in calls (50%) compared to other studies, many of which had 100% adherence on these calls ( Table 2 ). This study site is herein referred to as the low-engagement cohort. In this low-engagement cohort, median adherence to completing daily app surveys, to wearing the Empatica smartwatch, and to using the Bodyport Cardiac Scale were lower than all other study cohorts that included these studies’ activities (except the BUMP-postpartum cohort). Further, median adherence to using the Oura smart ring was lower in the low-engagement cohort compared to other cohorts except for the postpartum and severely ill cancer populations.
The HERO studies included the most severely ill participants including patients with active diagnoses of CNS and pancreatic tumors. Some HERO participants were undergoing chemotherapy, some had therapy-related complications, some had infections, and some had progressive, life-threatening tumor growth. While the total number of participants in these studies was low, these studies showed low adherence on the daily survey (<55%) and wearable device use (<65% HERO-CNS only). Interestingly, HERO-PANC participants exhibited high wearable device use median adherence (83.3%, IQR 51%-93.2%, Oura and 95.5%, IQR 75.2%-99.2%, Garmin), despite the health status of this population. Further, median adherence to in-app cognitive active tasks was higher among the HERO studies compared to most other studies. Engagement check-in call adherence was also high in the HERO studies. Among the BUMP postpartum cohort, there was consistently lower adherence on all study tasks except for the engagement check-in calls compared to other studies, particularly in comparison to the BUMP prenatal cohort. Specifically, median adherence to the Oura ring, Garmin smartwatch use, and the Bodyport Cardiac Scale in the BUMP-prenatal cohort compared to the BUMP postpartum cohort dropped from 87.2% (IQR 68.7%-96.7%) to 55% (IQR 5.5%-83.7%), 96.7% (IQR 82.9%-100%) to 62.5% (IQR 12.3%-96.4%), and 74.7% (IQR 52%-87.3%) to 33.1% (IQR 8.9%-67.7%), respectively ( Table 2 ).
Stress and recovery | BUMP-C | BUMP | BUMP-POST | SINC -MSSM | SINC-Oxford | HERO-CNS | HERO-PANC | Stress in LFS | |
Participants, n | 297 | 98 | 379 | 379 | 117 | 54 | 7 | 19 | 45 |
ES check-ins, median (IQR) | 75.0 (57.1-87.5) | 100.0 (87.9-100.0) | 100.0 (88.4-100.0) | 100.0 (100.0-100.0) | 50.0 (20.0-75.0) | 100.0 (90.9-100.0) | 85.7 (78.1-88.2) | 100.0 (100.0-100.0) | 60.0 (40.0-80.0) |
Oura ring, median (IQR) | 97.0 (86.0-100.0) | 90.6 (76.3-97.7) | 87.2 (68.7-96.7) | 55.0 (5.5-83.7) | 80.5 (37.1-92.4) | 98.9 (94.0-99.6) | 42.3 (32.0-58.2) | 83.3 (51.0-93.2) | — |
Garmin watch, median (IQR) | — | — | 96.7 (82.9-100.0) | 62.4 (12.3-96.4) | — | — | 63.3 (54.7-64.3) | 95.5 (75.2-99.2) | — |
Apple watch, median (IQR) | — | — | 98.1 (87.7-100.0) | 79.8 (32.4-96.3) | — | — | — | — | — |
Empatica watch, median (IQR) | — | — | — | — | 26.0 (6.2-64.1) | 72.5 (37.1-96.8) | — | — | 86.8 (66.7-95.6) |
Bodyport scale, median (IQR) | — | — | 74.7 (52.0-87.3) | 33.1 (8.9-67.7) | 38.5 (17.1-64.7) | — | — | 79.5 (52.7-88.4) | — |
Daily survey, median (IQR) | 75.4 (57.2-88.2) | 42.4 (24.6-69.7) | 60.1 (34.4-81.7) | 18.4 (1.0-47.6) | 27.9 (10.4-51.9) | 70.3 (41.9-84.0) | 53.3 (47.8-71.5) | 49.1 (20.2-83.4) | 62.5 (40.96-82.59) |
Reaction rime, median (IQR) | 88.9 (75.0-100.0) | 43.4 (24.3-72.8) | — | — | 30.4 (9.7-50.6) | 69.5 (46.6-89.3) | 59.0 (50.0-66.7) | 62.5 (20.9-86.6) | — |
Trail making, median (IQR) | 88.9 (71.1-100.0) | 46.5 (24.0-73.7) | — | — | 28.7 (9.4-50.0) | 71.6 (45.0-87.3) | 61.5 (52.1-76.5) | 38.1 (4.2-76.2) | 57.7 (36.8-72.0) |
EBT , median (IQR) | — | 30.1 (16.2-54.1) | 44.6 (22.6-73.9) | 6.5 (0.0-33.3) | 23.1 (9.1-44.4) | 32.1 (0.0-58.6) | — | — | — |
N-Back, median (IQR) | — | — | 51.4 (24.9-76.4) | 8.3 (0.0-44.4) | — | — | — | — | — |
Gait task, median (IQR) | — | — | 25.0 (0.0-60.0) | 0.0 (0.0-0.0) | — | — | 24.5 (18.8-62.8) | 36.0 (2.2-74.0) | — |
Walk test, median (IQR) | — | — | 14.3 (0.0-40.0) | 0.0 (0.0-0.0) | — | — | 23.1 (13.9-60.4) | 25.0 (7.8-49.5) | — |
Video diary, median (IQR) | — | 4.3 (0.0-27.7) | 8.3 (0.0-50.0) | 0.0 (0.0-0.0) | 5.6 (0.0-22.2) | 9.4 (0.0-35.1) | 25.0 (8.7-77.1) | 0.0 (0.0-37.5) | — |
a BUMP-C: Better Understanding the Metamorphosis of Pregnancy—Conception.
b BUMP: Better Understanding the Metamorphosis of Pregnancy.
c BUMP-POST: Better Understanding the Metamorphosis of Pregnancy—Postpartum.
d SINC: stress in Crohn.
e MSSM: Mount Sinai School of Medicine.
f HERO-CNS: help enable real-time observations—central nervous system.
g HERO-PANC: help enable real-time observations—pancreatic cancer.
h LFS: Li-Fraumeni syndrome.
i ES: engagement specialist.
j Not available.
k EBT: emotional bias test.
Adherence by Study Activity
There were differences in adherence rates across different study activities. Adherence to wearable device use was consistently higher across studies compared to in-app activities, which is not surprising given the passive nature of these devices. Excluding the postpartum and HERO-CNS study, median adherence to Oura ring use was >80% across all studies, and as high as 99% (IQR 94.9%-99.6%; stress in Crohn-Oxford site; Table 2 ). There were also differences in adherence across specific wearable devices. Garmin and Apple smartwatch adherence was >95% in BUMP pregnant individuals and HERO-PANC participants, while median adherence for the Empatica Watch was lower among the studies that used this device (stress in Crohn-Oxford, 72.5%, IQR 37.1%-96.8%; stress in Crohn-MSSM, low-engagement cohort, 26%, IQR 6.2%-64.1%; and stress in LFS, 86.8%, IQR 0.7%-0.9%). Median adherence to the Bodyport Cardiac Scale was 74.7% (IQR 52%-87.3%) among BUMP pregnant individuals and 79.5% (IQR 52.7%-88.4%) in HERO-PANC participants ( Table 2 ). Excluding the postpartum and HERO study populations and the low-engagement cohort, in-app daily survey adherence was >60% for all studies ( Table 2 ). Finally, adherence to in-app active tasks was lower in general compared to other activities such as wearable device use or in-app surveys. Tasks that involved walking (gait and walk task) or speaking (video diaries) showed lower adherence compared to other active tasks (eg, cognitive and emotional bias tasks; Table 2 ).
Adherence by Study Recruitment and Engagement Strategy
There did not appear to be any meaningful difference in median adherence rates across study activities by study recruitment methods (in-clinic vs remote) or follow-up time. Further, 2 studies that included modest financial compensation in addition to engagement strategies showed higher adherence rates compared to some of the other studies (ie, BUMP and stress in Crohn), but the impact of compensation is difficult to disentangle from other study characteristics such as population differences, and these studies did not show superior adherence rates compared to the stress and recovery study that did not offer financial compensation.
The median proportion of participants retained in the study across the 6 studies was 77.2% (IQR 72.6%-88%; Table 3 ). The probability of staying in the study stayed above 80% for all studies during the first month of study participation and stayed above 50% for the entire active study period across all studies ( Multimedia Appendix 4 ).
Study | Proportion retained at study completion, retained/enrolled (%) |
Stress and recovery | 297/365 (81.4) |
BUMP-C | 134/187 (72.7) |
BUMP | 379/524 (72.3) |
Stress in Crohn-MSSM | 117/139 (84.2) |
Stress in Crohn-Oxford | 54/56 (96.4) |
HERO-CNS | 7/12 (58.3) |
HERO-PANC | 19/26 (73.1) |
Stress and LFS | 45/49 (91.8) |
b Only includes participants who were enrolled in the Better Understanding the Metamorphosis of Pregnancy—Conception-specific app.
c BUMP: Better Understanding the Metamorphosis of Pregnancy.
d MSSM: Mount Sinai School of Medicine.
e HERO-CNS: help enable real-time observations—central nervous system.
f HERO-PANC: help enable real-time observations—pancreatic cancer.
g Help enable real-time observations—pancreatic cancer has unique factors to consider when interpreting the proportion retained until study completion, since the study aimed to monitor patients until they developed progressive disease or died, or the study end date (October 31, 2022; see Multimedia Appendix 3 ).
Adherence and Retention by Participant Sociodemographic Characteristics
Median adherence for the Oura smart ring, a smartwatch (Garmin, Apple, and Empatica), and the Bodyport Cardiac Scale was lower among younger participants compared to older participants across most studies ( Multimedia Appendix 5 ). Specifically, Oura smart ring adherence was significantly lower in those aged 18-25 years compared to those aged ≥26 years in the BUMP study ( P =.03) and stress in Crohn-MSSM studies ( P =.02), and was lower in the BUMP-C and stress and recover studies, but this difference was not statistically significant at P =.59 and P =.08, respectively. Median adherence for Apple smartwatch use was significantly lower in those aged 18-25 years compared to those aged ≥26 years in the BUMP study ( P =.02), while median adherence for Garmin smartwatch use was lower but not statistically significant ( P =.06). Median adherence for the Bodyport Cardiac Scale was significantly lower in those aged 18-25 years compared to those aged ≥26 years in BUMP ( P <.005) and stress in Crohn-MSSM ( P <.006).
In the BUMP study, Black or African American ethnicity had significantly higher median adherence to completing the in-app daily survey compared to other race or ethnicity groups ( P =.01). This trend was observed in the stress and recovery study ( P =.07) and the stress in Crohn-MSSM study ( P =.24), although the difference was not statistically significant. In contrast, median adherence to Oura smart ring, smartwatch, and Bodyport Cardiac Scale use was lower among Black or African American individuals compared to other race or ethnicity groups, although these differences were not statistically significant ( Multimedia Appendix 5 ).
Retention did not significantly differ by age group or gender ( Multimedia Appendix 6 ).
Retention likelihood was significantly different by race or ethnicity groups in BUMP-C ( P <.001) and BUMP ( P= .001). Specifically, participants of White ethnicity were more likely to stay in the study in both BUMP-C and BUMP, while participants reporting their race or ethnicity as either unknown or not reporting this item were less likely to be retained ( Multimedia Appendix 6 ).
Barriers to Engagement (Qualitative Synthesis of Participant Feedback)
Figure 1 describes key themes that impacted participant retention, adherence, and overall engagement that cut across all included studies. These themes include participant burden and forgetfulness, digital literacy, physical and mental barriers, personal and altruistic benefits, and privacy and confidentiality. Qualitative feedback from participants, research staff, and investigators across these 5 themes is summarized in Multimedia Appendix 7 . The top three barriers to engagement in active study tasks were (1) participant burden and in particular fatigue with the repetitiveness of tasks; (2) physical or mental and situational barriers that prevented the ability to complete tasks; and (3) personal and altruistic benefit, namely the perception that the use of the personal DHTs was not personally useful for a health benefit or a lack of understanding as to why and how certain features (eg, heart rate variability) could be useful to track for health benefit. Qualitative feedback from participants in the 2 cohorts demonstrating lower adherence (HERO-PANC and BUMP post partum) suggested that while participants were highly engaged, they were either too ill, distracted, or tired to complete many of the study activities while navigating a serious illness or the early postpartum period.

Principal Findings
Evidence across 6 unique and diverse studies involving the longitudinal use of personal DHTs supports that participant-centric engagement strategies aid in participant retention and maintaining good adherence in some populations. These strategies centered around (1) human contact with an engagement specialist as often as every 2 weeks, (2) investigator-participant meetings during active study follow-up, (3) offering returned symptom data in the app, (4) inviting participants to contribute as coauthors in published work, and (5) real-time modifications to the study app based on participant feedback.
In the majority of included studies, the probability of staying in the study stayed above 90% for the first month and stayed above 50% for active study periods for all studies. Lower retention or adherence was observed among studies that included a severely ill cancer population and a postpartum population. Barriers to participation in these cohorts were largely the result of physical and situational roadblocks. Excluding studies of a severely ill and postpartum population and the low-engagement cohort in the stress in Crohn study, adherence to Oura smart ring and Garmin smartwatch use was 80% and as high as 99% in some cohorts, while adherence to the Bodyport Cardiac Scale was 75% in a pregnant population. This supports that different populations can successfully be engaged in the use of active app assessments and wearable devices in the long term with adequate support.
Retention and adherence rates observed in these studies are higher than typically reported by other personal DHT research studies [ 7 - 9 , 12 , 13 , 21 ]. For example, a review of 8 large app-based DHT research studies in the United States reported that the probability of staying in the study dropped to or below 50% after the first 4 weeks of participation for all included studies [ 7 ]. Further, across the 8 included studies in this review, >50% of participants did not engage with the app for at least 7 days. Another large app-based study in the United States, the Warfighter Analytics Using Smartphones for Health study that collected daily active and passive app data reported a median retention of 45.2% (38/84 days), while the probability of staying in the study hit 50% at approximately 5.5 weeks [ 10 ]. A large app-based study in the United Kingdom (cloudy with a chance of pain study) involving daily active app assessments reported that 64% of participants fell into the low engagement or no engagement categories after baseline [ 12 ]. The RADAR study [ 14 ], a multinational study involving active and passive assessments from an app, and a Fitbit reported comparable retention results among participants with major depression to those reported here. This study reported a retention rate of 54.6% for 43 weeks of study participation; however, the probability of staying in the study stayed above 75% for the first several months of participation (~6 months). While the active app assessments in this study only included assessments every 2 weeks as opposed to daily assessments, this study additionally included aspects of a participant-centric design, which may have contributed to the higher reported retention [ 15 ].
Taken together, in comparison to other published personal DHT research studies, the 6 studies included in this paper reflect higher levels of engagement. Importantly, the included studies in this analysis involved high burden designs in comparison to other studies that request, for example, weekly or biweekly active tasks of participants [ 14 ] or only involve the use of a smartwatch. Specifically, across the included studies here, participants were expected to complete on average 4.6 (SD 1.62) minutes a day of app activities in addition to continuously using multiple wearable devices.
While different variations of participant-centric strategies were used across the 6 included studies, a key common feature was a biweekly check-in call with an engagement specialist. These calls served the purpose of providing support and building rapport with participants, working through onboarding and technological issues with study devices, tracking adherence, and receiving study-related feedback from participants. Numerous challenges arise in the conduct of remote, personal DHT research, and without frequent check-in and semiregular data monitoring by research staff, knowledge of these issues is a black box. The most significant drop in retention in personal DHT research studies tends to be during the first few weeks of participation [ 7 ]. These early onboarding weeks are crucial in working with participants to ensure they can get into a rhythm of participation. The passive sensing nature of personal DHTs has much potential to inform new objective measures of health, however, are not always intuitively understood as personally important for unique diseases (eg, heart rate variability or phone screen time). Personal DHT studies allow for “light touch” research approaches that enable data collection without traditional research coordinator contact, but this may come with a cost that inadvertently creates a less engaging study environment for participants and limits the opportunity to help participants understand the value in their participation. Of the included 6 studies, 1 cohort had much lower engagement on the check-in calls (50% adherence) compared to other included studies and, in turn, consistently demonstrated lower adherence to study-related activities. Still, even with extensive engagement designs, populations that had physical, mental, and situational barriers to study task completion (ie, severely ill, postpartum mothers) showed lower adherence to wearable device use and active smartphone tasks compared to other study populations. Top reported barriers to engagement included participant burden, physical, mental, and situational barriers, and low perceived value of personal DHTs for health care. These engagement barriers have been reported in previous literature [ 8 , 9 ] relating to DHT research and in the use of DHT interventions. However, the conveyed importance of the perceived value of the approach among participants in the current analysis is noteworthy. Given the foreign nature of personal DHTs for many individuals, particularly older populations, further work is needed to co-design and educate end users on the potential value of self-monitoring unique health-related data.
Irrespective of the engagement approach, adherence to in-app surveys and tasks was lower than wearable device use, which is not surprising given the higher burden related to in-app activities. The self-reported information captured from frequent or momentary in-app assessments is extremely valuable as context information. This context information or “label” data is useful for validating objectively captured information, yet remains the most difficult to capture in sufficient detail. Further, certain in-app activity adherences were consistently lower than others. Namely, activities that required the user to be active (walk in a straight line or complete a video diary) were low across studies. Still, adherence to daily in-app surveys was >60% for all studies excluding the postpartum and HERO study populations.
Limitations
This quantitative and qualitative analysis compared observational data across different digital health studies. However, no true comparison cohort that did not include engagement strategies was included. Therefore, the inferred casualty of participant check-ins with engagement specialists on retention and adherence rates cannot be not concluded. We are formally testing whether the biweekly check-in significantly increases adherence and retention in an ongoing study with an appropriate comparison arm without check-in support (NCT05753605). One of the included studies (stress and recovery) was conducted during the early 2020 COVID-19 pandemic. There is some evidence that engagement in research was higher during the early pandemic time periods [ 22 ]. It cannot be ruled out that the higher observed retention and adherence in this study compared to others was not due to this potential time period bias. The stress in the Crohn-Oxford site included a population of patients some of whom were already engaged in the use of web-based monitoring of symptoms. In turn, this could have contributed to the high retention and higher adherence observed at this site compared to the other stress in the Crohn-MSSM site. The results presented on barriers to engagement were primarily qualitative and collected from conversations with participants, research staff, and investigators across studies.
Conclusions
Globally, mobile apps are used for a variety of purposes in everyday life, while the use of smartwatches for activity monitoring is gaining increasing popularity. However, the use of these tools for health remains a challenge. These findings support that human support via phone and other participant-centric engagement strategies centered on giving back to participants and working with them as co-designers can support sufficient retention and adherence in personal DHT research across diverse populations. This has implications for the utility and potential necessity of a digital support worker in digital health care, as highlighted by others [ 23 ]. A power of personal DHTs is enabling the patient to be in control of their health through self-monitoring, but this new role comes with a responsibility. This important shift in role from doctor to patient outlines how crucial it is to include patients in the early design phase of personal DHT health research. Further work is needed to inform app designs that support habitual forming activities around task completion so that app-related activities become a part of participants’ daily routine and are perceived as personally valuable.
Acknowledgments
The stress and recovery study was supported in part by the Bill & Melinda Gates Foundation (INV-016651). The stress in Crohn study was funded by the Leona M. and Harry B. Helmsley Charitable Trust (1911-03376). The help enable real-time observation (HERO)–central nervus system study was funded by the Mark Foundation for Cancer Research through an ASPIRE award (19-024-ASP). The HERO–pancreatic cancer study was funded by the Mark Foundation for Cancer Research through an ASPIRE award (19-024-ASP), Pancreatic Cancer Canada, the Princess Margaret Cancer Foundation, and 4YouandMe. The Better Understanding the Metamorphosis of Pregnancy (BUMP) study was funded by 4YouandMe and Sema4 along with supplemental in-kind contributions from coalition partners (Evidation Health, Vector Institute, Cambridge Cognition, and Bodyport). The stress and LFS study was funded by in-kind contributions from 4YouandMe, SickKids Hospital, and the Vector Institute.
Conflicts of Interest
CB is a consultant for Depuy Synthes, Bionaut Labs, Galectin Therapeutics, Haystack Oncology, and Privo Technologies. CB is a cofounder of Belay Diagnostics and OrisDx. DRK is an officer, employee, and shareholder of MindMed; a consultant at Tempus, Nightware, and Limitless; and board member of Sonara. RPH is an advisory board member at Bristol Meyers Squibb. MH is an advisory board member for Servier, AnHeart, and Bayer; steering committee member for Novartis; honoraria from Novartis; data safety monitoring committee member for Advarra and Parexel. RG received a graduate scholarship from Pfizer and provided consulting or advisory roles for Astrazeneca, Tempus, Eisai, Incyte, Knight Therapeutics, Guardant Health, and Ipsen. The others declare no conflicts of interest.
Study descriptions.
Study wearable devices.
Retention calculations.
Probability of retaining in the study across studies.
Median adherence to study activities stratified by sociodemographic characteristics.
Sociodemographic differences in participants who were retained versus not retained.
Qualitative feedback from participants, research staff, and investigators surrounding barriers to engagement in digital health research, summarized across 6 unique studies.
- Friend SH, Ginsburg GS, Picard RW. Wearable digital health technology. N Engl J Med. 2023;389(22):2100-2101. [ CrossRef ] [ Medline ]
- Goodday SM, Geddes JR, Friend SH. Disrupting the power balance between doctors and patients in the digital era. Lancet Digit Health. 2021;3(3):e142-e143. [ FREE Full text ] [ CrossRef ] [ Medline ]
- Ng MM, Firth J, Minen M, Torous J. User engagement in mental health apps: a review of measurement, reporting, and validity. Psychiatr Serv. 2019;70(7):538-544. [ FREE Full text ] [ CrossRef ] [ Medline ]
- White KM, Williamson C, Bergou N, Oetzmann C, de Angel V, Matcham F, et al. A systematic review of engagement reporting in remote measurement studies for health symptom tracking. NPJ Digit Med. 2022;5(1):82. [ FREE Full text ] [ CrossRef ] [ Medline ]
- Daniore P, Nittas V, von Wyl V. Enrollment and retention of participants in remote digital health studies: scoping review and framework proposal. J Med Internet Res. 2022;24(9):e39910. [ FREE Full text ] [ CrossRef ] [ Medline ]
- Chan A, Chan D, Lee H, Ng CC, Yeo AHL. Reporting adherence, validity and physical activity measures of wearable activity trackers in medical research: a systematic review. Int J Med Inform. 2022;160:104696. [ FREE Full text ] [ CrossRef ] [ Medline ]
- Pratap A, Neto EC, Snyder P, Stepnowsky C, Elhadad N, Grant D, et al. Indicators of retention in remote digital health studies: a cross-study evaluation of 100,000 participants. NPJ Digit Med. 2020;3:21. [ FREE Full text ] [ CrossRef ] [ Medline ]
- Simblett S, Matcham F, Siddi S, Bulgari V, Barattieri di San Pietro C, Hortas López J, et al. RADAR-CNS Consortium. Barriers to and facilitators of engagement with mHealth technology for remote measurement and management of depression: qualitative analysis. JMIR mHealth uHealth. 2019;7(1):e11325. [ FREE Full text ] [ CrossRef ] [ Medline ]
- O'Connor S, Hanlon P, O'Donnell CA, Garcia S, Glanville J, Mair F. Understanding factors affecting patient and public engagement and recruitment to digital health interventions: a systematic review of qualitative studies. BMC Med Inform Decis Mak. 2016;16(1):120. [ FREE Full text ] [ CrossRef ] [ Medline ]
- Li SX, Halabi R, Selvarajan R, Woerner M, Fillipo IG, Banerjee S, et al. Recruitment and retention in remote research: learnings from a large, decentralized real-world study. JMIR Form Res. 2022;6(11):e40765. [ FREE Full text ] [ CrossRef ] [ Medline ]
- Goodday SM, Karlin E, Alfarano A, Brooks A, Chapman C, Desille R, Stress And Recovery Participants, et al. An alternative to the light touch digital health remote study: the stress and recovery in frontline COVID-19 health care workers study. JMIR Form Res. 2021;5(12):e32165. [ FREE Full text ] [ CrossRef ] [ Medline ]
- Druce KL, McBeth J, van der Veer SN, Selby DA, Vidgen B, Georgatzis K, et al. Recruitment and ongoing engagement in a UK smartphone study examining the association between weather and pain: cohort study. JMIR mHealth uHealth. 2017;5(11):e168. [ FREE Full text ] [ CrossRef ] [ Medline ]
- Reade S, Spencer K, Sergeant JC, Sperrin M, Schultz DM, Ainsworth J, et al. Cloudy with a chance of pain: engagement and subsequent attrition of daily data entry in a smartphone pilot study tracking weather, disease severity, and physical activity in patients with rheumatoid arthritis. JMIR mHealth uHealth. 2017;5(3):e37. [ FREE Full text ] [ CrossRef ] [ Medline ]
- Matcham F, Leightley D, Siddi S, Lamers F, White KM, Annas P, et al. RADAR-CNS consortium. Remote Assessment of Disease and Relapse in Major Depressive Disorder (RADAR-MDD): recruitment, retention, and data availability in a longitudinal remote measurement study. BMC Psychiatry. 2022;22(1):136. [ FREE Full text ] [ CrossRef ] [ Medline ]
- Zhang Y, Pratap A, Folarin AA, Sun S, Cummins N, Matcham F, et al. Long-term participant retention and engagement patterns in an app and wearable-based multinational remote digital depression study. NPJ Digit Med. Feb 17, 2023;6(1):25. [ CrossRef ] [ Medline ]
- How we engage the public. Wellcome. URL: https://wellcome.org/what-we-do/public-engagement [accessed 2024-02-02]
- Canada's strategy for patient-oriented research. Canadian Institutes of Health Research. URL: https://cihr-irsc.gc.ca/e/44000.html [accessed 2024-02-02]
- NIHR launches new centre for engagement and dissemination. National Institute of Health and Care Research. URL: https://www.nihr.ac.uk/news/nihr-launches-new-centre-for-engagement-and-dissemination/24576 [accessed 2024-02-02]
- Goodday SM, Karlin E, Brooks A, Chapman C, Karlin DR, Foschini L, et al. Better Understanding of the Metamorphosis of Pregnancy (BUMP): protocol for a digital feasibility study in women from preconception to postpartum. NPJ Digit Med. Mar 30, 2022;5(1):40. [ CrossRef ] [ Medline ]
- 4YouandMe. URL: http://4youandme.org [accessed 2023-06-07]
- Nowell WB, Curtis JR, Zhao H, Xie F, Stradford L, Curtis D, et al. Participant engagement and adherence to providing smartwatch and patient-reported outcome data: digital tracking of rheumatoid arthritis longitudinally (DIGITAL) real-world study. JMIR Hum Factors. 2023;10:e44034. [ FREE Full text ] [ CrossRef ] [ Medline ]
- Vargo D, Zhu L, Benwell B, Yan Z. Digital technology use during COVID-19 pandemic: a rapid review. Human Behav and Emerg Tech. Dec 28, 2020;3(1):13-24. [ CrossRef ]
- Perret S, Alon N, Carpenter-Song E, Myrick K, Thompson K, Li S, et al. Standardising the role of a digital navigator in behavioural health: a systematic review. Lancet Digit Health. 2023;5(12):e925-e932. [ FREE Full text ] [ CrossRef ] [ Medline ]
Abbreviations
Better Understanding the Metamorphosis of Pregnancy |
central nervous system |
digital health technology |
help enable real-time observation |
institutional review board |
Li-Fraumeni syndrome |
Mount Sinai School of Medicine |
pancreatic cancer |
research ethics board |
Edited by G Eysenbach, T de Azevedo Cardoso; submitted 06.03.24; peer-reviewed by C Godoy Jr; comments to author 05.04.24; revised version received 12.04.24; accepted 29.05.24; published 03.09.24.
©Sarah M Goodday, Emma Karlin, Alexa Brooks, Carol Chapman, Christiana Harry, Nelly Lugo, Shannon Peabody, Shazia Rangwala, Ella Swanson, Jonell Tempero, Robin Yang, Daniel R Karlin, Ron Rabinowicz, David Malkin, Simon Travis, Alissa Walsh, Robert P Hirten, Bruce E Sands, Chetan Bettegowda, Matthias Holdhoff, Jessica Wollett, Kelly Szajna, Kallan Dirmeyer, Anna Dodd, Shawn Hutchinson, Stephanie Ramotar, Robert C Grant, Adrien Boch, Mackenzie Wildman, Stephen H Friend. Originally published in the Journal of Medical Internet Research (https://www.jmir.org), 03.09.2024.
This is an open-access article distributed under the terms of the Creative Commons Attribution License (https://creativecommons.org/licenses/by/4.0/), which permits unrestricted use, distribution, and reproduction in any medium, provided the original work, first published in the Journal of Medical Internet Research (ISSN 1438-8871), is properly cited. The complete bibliographic information, a link to the original publication on https://www.jmir.org/, as well as this copyright and license information must be included.
The University of Chicago The Law School
Abrams environmental law clinic—significant achievements for 2023-24, protecting our great lakes, rivers, and shorelines.
The Abrams Clinic represents Friends of the Chicago River and the Sierra Club in their efforts to hold Trump Tower in downtown Chicago accountable for withdrawing water illegally from the Chicago River. To cool the building, Trump Tower draws water at high volumes, similar to industrial factories or power plants, but Trump Tower operated for more than a decade without ever conducting the legally required studies to determine the impact of those operations on aquatic life or without installing sufficient equipment to protect aquatic life consistent with federal regulations. After the Clinic sent a notice of intent to sue Trump Tower, the State of Illinois filed its own case in the summer of 2018, and the Clinic moved successfully to intervene in that case. In 2023-24, motions practice and discovery continued. Working with co-counsel at Northwestern University’s Pritzker Law School’s Environmental Advocacy Center, the Clinic moved to amend its complaint to include Trump Tower’s systematic underreporting each month of the volume of water that it intakes from and discharges to the Chicago River. The Clinic and co-counsel addressed Trump Tower’s motion to dismiss some of our clients’ claims, and we filed a motion for summary judgment on our claim that Trump Tower has committed a public nuisance. We also worked closely with our expert, Dr. Peter Henderson, on a supplemental disclosure and on defending an additional deposition of him. In summer 2024, the Clinic is defending its motion for summary judgment and challenging Trump Tower’s own motion for summary judgment. The Clinic is also preparing for trial, which could take place as early as fall 2024.
Since 2016, the Abrams Clinic has worked with the Chicago chapter of the Surfrider Foundation to protect water quality along the Lake Michigan shoreline in northwest Indiana, where its members surf. In April 2017, the U. S. Steel plant in Portage, Indiana, spilled approximately 300 pounds of hexavalent chromium into Lake Michigan. In January 2018, the Abrams Clinic filed a suit on behalf of Surfrider against U. S. Steel, alleging multiple violations of U. S. Steel’s discharge permits; the City of Chicago filed suit shortly after. When the US government and the State of Indiana filed their own, separate case, the Clinic filed extensive comments on the proposed consent decree. In August 2021, the court entered a revised consent decree which included provisions advocated for by Surfrider and the City of Chicago, namely a water sampling project that alerts beachgoers as to Lake Michigan’s water quality conditions, better notifications in case of future spills, and improvements to U. S. Steel’s operations and maintenance plans. In the 2023-24 academic year, the Clinic successfully litigated its claims for attorneys’ fees as a substantially prevailing party. Significantly, the court’s order adopted the “Fitzpatrick matrix,” used by the US Attorney’s Office for the District of Columbia to determine appropriate hourly rates for civil litigants, endorsed Chicago legal market rates as the appropriate rates for complex environmental litigation in Northwest Indiana, and allowed for partially reconstructed time records. The Clinic’s work, which has received significant media attention, helped to spawn other litigation to address pollution by other industrial facilities in Northwest Indiana and other enforcement against U. S. Steel by the State of Indiana.
In Winter Quarter 2024, Clinic students worked closely with Dr. John Ikerd, an agricultural economist and emeritus professor at the University of Missouri, to file an amicus brief in Food & Water Watch v. U.S. Environmental Protection Agency . In that case pending before the Ninth Circuit, Food & Water Watch argues that US EPA is illegally allowing Concentrated Animal Feeding Operations, more commonly known as factory farms, to pollute waterways significantly more than is allowable under the Clean Water Act. In the brief for Dr. Ikerd and co-amici Austin Frerick, Crawford Stewardship Project, Family Farm Defenders, Farm Aid, Missouri Rural Crisis Center, National Family Farm Coalition, National Sustainable Agriculture Coalition, and Western Organization of Resource Councils, we argued that EPA’s refusal to regulate CAFOs effectively is an unwarranted application of “agricultural exceptionalism” to industrial agriculture and that EPA effectively distorts the animal production market by allowing CAFOs to externalize their pollution costs and diminishing the ability of family farms to compete. Attorneys for the litigants will argue the case in September 2024.
Energy and Climate
Energy justice.
The Abrams Clinic supported grassroots organizations advocating for energy justice in low-income communities and Black, Indigenous, and People of Color (BIPOC) communities in Michigan. With the Clinic’s representation, these organizations intervened in cases before the Michigan Public Service Commission (MPSC), which regulates investor-owned utilities. Students conducted discovery, drafted written testimony, cross-examined utility executives, participated in settlement discussions, and filed briefs for these projects. The Clinic’s representation has elevated the concerns of these community organizations and forced both the utilities and regulators to consider issues of equity to an unprecedented degree. This year, on behalf of Soulardarity (Highland Park, MI), We Want Green, Too (Detroit, MI), and Urban Core Collective (Grand Rapids, MI), Clinic students engaged in eight contested cases before the MPSC against DTE Electric, DTE Gas, and Consumers Energy, as well as provided support for our clients’ advocacy in other non-contested MPSC proceedings.
The Clinic started this past fall with wins in three cases. First, the Clinic’s clients settled with DTE Electric in its Integrated Resource Plan case. The settlement included an agreement to close the second dirtiest coal power plant in Michigan three years early, $30 million from DTE’s shareholders to assist low-income customers in paying their bills, and $8 million from DTE’s shareholders toward a community fund that assists low-income customers with installing energy efficiency improvements, renewable energy, and battery technology. Second, in DTE Electric’s 2023 request for a rate hike (a “rate case”), the Commission required DTE Electric to develop a more robust environmental justice analysis and rejected the Company’s second attempt to waive consumer protections through a proposed electric utility prepayment program with a questionable history of success during its pilot run. The final Commission order and the administrative law judge’s proposal for final decision cited the Clinic’s testimony and briefs. Third, in Consumers Electric’s 2023 rate case, the Commission rejected the Company’s request for a higher ratepayer-funded return on its investments and required the Company to create a process that will enable intervenors to obtain accurate GIS data. The Clinic intends to use this data to map the disparate impact of infrastructure investment in low-income and BIPOC communities.
In the winter, the Clinic filed public comments regarding DTE Electric and Consumers Energy’s “distribution grid plans” (DGP) as well as supported interventions in two additional cases: Consumers Energy’s voluntary green pricing (VGP) case and the Clinic’s first case against the gas utility DTE Gas. Beginning with the DGP comments, the Clinic first addressed Consumers’s 2023 Electric Distribution Infrastructure Investment Plan (EDIIP), which detailed current distribution system health and the utility’s approximately $7 billion capital project planning ($2 billion of which went unaccounted for in the EDIIP) over 2023–2028. The Clinic then commented on DTE Electric’s 2023 DGP, which outlined the utility’s opaque project prioritization and planned more than $9 billion in capital investments and associated maintenance over 2024–2028. The comments targeted four areas of deficiencies in both the EDIIP and DGP: (1) inadequate consideration of distributed energy resources (DERs) as providing grid reliability, resiliency, and energy transition benefits; (2) flawed environmental justice analysis, particularly with respect to the collection of performance metrics and the narrow implementation of the Michigan Environmental Justice Screen Tool; (3) inequitable investment patterns across census tracts, with emphasis on DTE Electric’s skewed prioritization for retaining its old circuits rather than upgrading those circuits; and (4) failing to engage with community feedback.
For the VGP case against Consumers, the Clinic supported the filing of both an initial brief and reply brief requesting that the Commission reject the Company’s flawed proposal for a “community solar” program. In a prior case, the Clinic advocated for the development of a community solar program that would provide low-income, BIPOC communities with access to clean energy. As a result of our efforts, the Commission approved a settlement agreement requiring the Company “to evaluate and provide a strawman recommendation on community solar in its Voluntary Green Pricing Program.” However, the Company’s subsequent proposal in its VGP case violated the Commission’s order because it (1) was not consistent with the applicable law, MCL 460.1061; (2) was not a true community solar program; (3) lacked essential details; (4) failed to compensate subscribers sufficiently; (5) included overpriced and inflexible subscriptions; (6) excessively limited capacity; and (7) failed to provide a clear pathway for certain participants to transition into other VGP programs. For these reasons, the Clinic argued that the Commission should reject the Company’s proposal.
In DTE Gas’s current rate case, the Clinic worked with four witnesses to develop testimony that would rebut DTE Gas’s request for a rate hike on its customers. The testimony advocated for a pathway to a just energy transition that avoids dumping the costs of stranded gas assets on the low-income and BIPOC communities that are likely to be the last to electrify. Instead, the testimony proposed that the gas and electric utilities undertake integrated planning that would prioritize electric infrastructure over gas infrastructure investment to ensure that DTE Gas does not over-invest in gas infrastructure that will be rendered obsolete in the coming decades. The Clinic also worked with one expert witness to develop an analysis of DTE Gas’s unaffordable bills and inequitable shutoff, deposit, and collections practices. Lastly, the Clinic offered testimony on behalf of and from community members who would be directly impacted by the Company’s rate hike and lack of affordable and quality service. Clinic students have spent the summer drafting an approximately one-hundred-page brief making these arguments formally. We expect the Commission’s decision this fall.
Finally, both DTE Electric and Consumers Energy have filed additional requests for rate increases after the conclusion of their respective rate cases filed in 2023. On behalf of our Clients, the Clinic has intervened in these cases, and clinic students have already reviewed thousands of pages of documents and started to develop arguments and strategies to protect low-income and BIPOC communities from the utility’s ceaseless efforts to increase the cost of energy.
Corporate Climate Greenwashing
The Abrams Environmental Law Clinic worked with a leading international nonprofit dedicated to using the law to protect the environment to research corporate climate greenwashing, focusing on consumer protection, green financing, and securities liability. Clinic students spent the year examining an innovative state law, drafted a fifty-page guide to the statute and relevant cases, and examined how the law would apply to a variety of potential cases. Students then presented their findings in a case study and oral presentation to members of ClientEarth, including the organization’s North American head and members of its European team. The project helped identify the strengths and weaknesses of potential new strategies for increasing corporate accountability in the fight against climate change.
Land Contamination, Lead, and Hazardous Waste
The Abrams Clinic continues to represent East Chicago, Indiana, residents who live or lived on or adjacent to the USS Lead Superfund site. This year, the Clinic worked closely with the East Chicago/Calumet Coalition Community Advisory Group (CAG) to advance the CAG’s advocacy beyond the Superfund site and the adjacent Dupont RCRA site. Through multiple forms of advocacy, the clinics challenged the poor performance and permit modification and renewal attempts of Tradebe Treatment and Recycling, LLC (Tradebe), a hazardous waste storage and recycling facility in the community. Clinic students sent letters to US EPA and Indiana Department of Environmental Management officials about how IDEM has failed to assess meaningful penalties against Tradebe for repeated violations of the law and how IDEM has allowed Tradebe to continue to threaten public and worker health and safety by not improving its operations. Students also drafted substantial comments for the CAG on the US EPA’s Lead and Copper Rule improvements, the Suppliers’ Park proposed cleanup, and Sims Metal’s proposed air permit revisions. The Clinic has also continued working with the CAG, environmental experts, and regulators since US EPA awarded $200,000 to the CAG for community air monitoring. The Clinic and its clients also joined comments drafted by other environmental organizations about poor operations and loose regulatory oversight of several industrial facilities in the area.
Endangered Species
The Abrams Clinic represented the Center for Biological Diversity (CBD) and the Hoosier Environmental Council (HEC) in litigation regarding the US Fish and Wildlife Service’s (Service) failure to list the Kirtland’s snake as threatened or endangered under the Endangered Species Act. The Kirtland’s snake is a small, secretive, non-venomous snake historically located across the Midwest and the Ohio River Valley. Development and climate change have undermined large portions of the snake’s habitat, and populations are declining. Accordingly, the Clinic sued the Service in the US District Court for the District of Columbia last summer over the Service’s denial of CBD’s request to have the Kirtland’s snake protected. This spring, the Clinic was able to reach a settlement with the Service that requires the Service to reconsider its listing decision for the Kirtland’s snake and to pay attorney fees.
The Clinic also represented CBD in preparation for litigation regarding the Service’s failure to list another species as threatened or endangered. Threats from land development and climate change have devastated this species as well, and the species has already been extirpated from two of the sixteen US states in its range. As such, the Clinic worked this winter and spring to prepare a notice of intent (NOI) to sue the Service. The Team poured over hundreds of FOIA documents and dug into the Service’s supporting documentation to create strong arguments against the Service in the imminent litigation. The Clinic will send the NOI and file a complaint in the next few months.
Students and Faculty
Twenty-four law school students from the classes of 2024 and 2025 participated in the Clinic, performing complex legal research, reviewing documents obtained through discovery, drafting legal research memos and briefs, conferring with clients, conducting cross-examination, participating in settlement conferences, and arguing motions. Students secured nine clerkships, five were heading to private practice after graduation, and two are pursuing public interest work. Sam Heppell joined the Clinic from civil rights private practice, bringing the Clinic to its full complement of three attorneys.
- Open access
- Published: 31 August 2024
The association between dietary phytochemical index and bacterial vaginosis risk: secondary analysis of case-control study
- Aynaz Khademian ORCID: orcid.org/0000-0001-9098-3730 1 ,
- Morvarid Noormohammadi ORCID: orcid.org/0000-0002-1971-8982 2 , 3 ,
- Mozhgan Hafizi Moori 4 ,
- Maede Makhtoomi 5 , 6 ,
- Sedighe Esmaeilzadeh 7 ,
- Mehran Nouri ORCID: orcid.org/0000-0002-7031-3542 7 &
- Ghazaleh Eslamian ORCID: orcid.org/0000-0002-8960-5123 8
Journal of Health, Population and Nutrition volume 43 , Article number: 135 ( 2024 ) Cite this article
Metrics details
Introduction
By studying the dietary habits of patients with bacterial vaginosis (BV) and the controls, we aim to find out whether the dietary intakes of phytochemicals could reduce the odds of BV. To the best of our knowledge, no study has ever examined the matter before. Therefore, we decided to conduct this secondary analysis of case-control study to examine the association between dietary phytochemicals and BV.
This case-control study was conducted at the gynecological clinic of Imam Hossein Hospital using a convenience sampling method from November 2020 to June 2021. To diagnose BV, all participants underwent examination by a gynecologist, assessing the presence of 3 or 4 criteria from the Amsel criteria. A validated semi-quantitative food frequency questionnaire was used. The phytochemical index was determined using McCarty’s method. To assess the association between dietary phytochemical intake and the odds of BV, binary logistic regression was utilized.
After adjusting for potential confounders, the association between phytochemical index and BV remained significant (odds ratio (OR) = 0.349, 95% confidence interval (CI): 0.176–0.695, p -value = 0.003). Furthermore, each unit increase in fat intake was associated with higher odds of BV (OR = 1.008, 95% CI: 1.002–1.014, p -value = 0.006), and a positive family history of BV continued to show significantly increased odds of BV (OR = 3.442, 95% CI: 2.068–5.728, p -value < 0.001).
In summary, the findings of this study indicate that increased consumption of dietary phytochemicals is associated with a reduced risk of BV among Iranian women of reproductive age. Additional research, especially longitudinal dietary studies, is required to explore the potential impact of dietary modifications on BV.
The vagina hosts a wide variety of bacteria which are dynamic and ever-changing. However, under normal circumstances, lactobacilli make up the majority of them. This optimum proportion is believed to fight off infections by producing lactic acid and maintaining an acidic environment. Disruption of the said proportion, perhaps through the increase in the amount of various anaerobic bacteria, is called bacterial vaginosis (BV) [ 1 ] and is believed to cause several complications for women of childbearing age [ 2 ]. The dysbiosis of the vaginal microbiota might pave the way for the contraction of sexually transmitted infections, urogenital infections, and pelvic inflammatory disease [ 3 ]. Moreover, up to 40% of preterm births are thought to be caused by some sort of vaginal or intrauterine infection [ 4 ]. Ethnical differences, sexual activities, vaginal douching, and fluctuations in estrogen levels are deemed to be the main risk factors. However, despite affecting 1 in every 3 women, many aspects of the disease, especially the dietary aspects, remain unknown and therefore there is still a call for further research.
Phytochemicals are organic compounds produced by plants, mainly to serve protective purposes and, therefore considered secondary metabolites [ 5 ]. So far, thousands of phytochemicals have been discovered, extracted, and carefully studied and are believed to exert many beneficial effects [ 6 ]. Some of the significant phytochemicals are alkaloids, saponins, tannins, flavonoids, and steroids which have also been shown to stimulate strong antioxidant, antimicrobial, antiallergic, and antiviral activities [ 7 , 8 ]. Their antibacterial properties have especially been of notice. Despite being less potent than antibiotics, research has shown phytochemical supplementation in adjunction to antibiotics could strengthen the efficacy of antibiotic therapy, especially when they are beginning to fall short against bacteria due to their increased development of resistance [ 9 ]. Through a recently devised index, we can now estimate the approximate dietary intake of phytochemicals. Observational studies examining the benefits of dietary phytochemicals have shown their efficacy in reducing the risk of cancer, heart disease, and neurodegenerative diseases [ 10 , 11 , 12 ].
By studying the dietary habits of patients with BV and the controls, we aim to find out whether the dietary intakes of phytochemicals could reduce the odds of BV. To the best of our knowledge, no study has ever examined the matter before. Therefore, we decided to conduct this secondary analysis of case-control study to examine the association between dietary phytochemicals and BV.
Method and materials
Study population.
This secondary analysis of case-control study was conducted at the gynecological clinic of Imam Hossein Hospital using a convenience sampling method from November 2020 to June 2021. The sample size was determined based on the study by Fahim et al. [ 13 ]. We enrolled 151 women with BV in the case group and 143 healthy women in the control group. This study was a secondary analysis and detailed information on the sample size calculation and other inclusion criteria can be found in previous studies [ 14 , 15 , 16 , 17 , 18 ]. To diagnose BV, all participants underwent examination by a gynecologist, assessing the presence of 3 or 4 criteria from the Amsel criteria, which include: a homogeneous and watery vaginal discharge, vaginal pH greater than 4.5, presence of 20% clue cells observed during saline microscopy, and a fishy odor detected after adding 10% potassium hydroxide to the discharge slide [ 19 , 20 ].
Eligible participants met the following inclusion criteria: willingness to participate and signing the consent form, aged between 15 and 45 years, not pregnant, not in menopause, and not using antibiotics, probiotics, hormonal contraceptives, vaginal douches, or immunosuppressive medications. They also did not have systemic illnesses, autoimmune diseases, chronic infections, diet-related chronic diseases like diabetes and cardiovascular disease, or any uterine cavity issues such as fibroids, polyps, or hysterectomy. The only difference in inclusion criteria between the case and control groups was the presence of a BV diagnosis for the case group and the absence of ongoing or previous BV or BV treatment for the control group.
Participants in both groups were excluded if they did not complete 60% or more of the food frequency questionnaire (FFQ), if their reported energy intake deviated beyond ± 3 standard deviations (SD) from the average energy intake, or if they expressed unwillingness to continue participating in the study.
A checklist was employed to gather data on participants’ age, family history of BV, polycystic ovary syndrome, and pregnancy, pregnancy history, menstrual cycle, education level, occupational status, smoking habits, number of sexual partners, and monthly family income. Questions regarding alcohol and opium use were omitted due to specific religious and cultural beliefs among Iranians. Anthropometric assessments included weight measurement using a reliable scale with a precision of 100 g, height measurement in the standing position without shoes with 1 mm accuracy, and waist circumference (WC) measurement to assess central adiposity using a measuring tape accurate to the nearest 1 mm, conducted by a trained examiner. Body Mass Index (BMI) was calculated by dividing weight in kilograms by the square of height in meters. Physical activity levels were assessed using the International Physical Activity Questionnaire (IPAQ), the validity and reliability of which have been previously established in studies conducted in Iran [ 21 ].
Dietary intake assessment
A validated semi-quantitative FFQ consisting of 168 food items [ 22 ], each with a standard and commonly used serving size in Iran, was used to estimate the participants’ dietary intake over the year preceding the interview. During the interview, participants were informed about the average size of each food item. They then reported how often they consumed each item, specifying the frequency on a daily, weekly, or monthly basis. The reported values for each food were converted to grams using a household scale guide. The average daily intakes of energy and nutrients were calculated using the Iranian food composition Table [ 23 ] and the USDA food composition Table [ 24 ].
Phytochemical index
The phytochemical index was determined using McCarty’s method [ 25 ] as follows: [phytochemical index = (daily energy from phytochemicals- rich foods (kcal) / total daily energy intake (kcal)) × 100]. First, the energy intake from each phytochemical-rich food item was calculated based on their total gram intake. Then, the total energy intake from all phytochemical-rich foods was determined. These foods included whole grains, legumes, nuts, olives and olive oil, soy products, seeds, tea, coffee, and spices. Natural vegetable and fruit juices, along with tomato sauces, were included in the vegetable and fruit groups because of their high phytochemical content. However, potatoes and pickled vegetables were excluded from the vegetable groups due to their low phytochemical content [ 26 , 27 ]. The total phytochemical index intake was then classified as either below or above the mean intake.
Statistical analysis
All statistical analyses were conducted using SPSS (Statistical Package for the Social Sciences, version 23, Chicago, IL, United States). The Chi-square and Kruskal-Wallis tests were used to compare categorical and non-parametric baseline variables between tertiles of the phytochemical index in both the case and control groups. Continuous variables were presented as medians (with 25th-75th confidence intervals), and categorical variables as percentages. To assess the association between dietary phytochemical intake and the odds of bacterial vaginosis (BV), binary logistic regression was utilized in both crude and adjusted models (using the Backward LR method for multivariate analysis), calculating odds ratios (OR) with 95% confidence intervals (CI). The second model adjusted for potential confounders, which were selected based on a p -value < 0.25 in the univariate analysis (adjusted for age (years), BMI (kg/m 2 ), fat intake (g/day), and familial history of BV (no/yes)).
Table 1 shows significant differences in age ( p -value = 0.032), BMI ( p -value = 0.002), pregnancy history ( p -value = 0.002), pregnancy number ( p -value = 0.003), and menstrual cycle ( p -value = 0.010) across phytochemical index tertiles within the case group. Additionally, all nutrient and food group intakes differed significantly across phytochemical index tertiles in both case and control groups ( p -value < 0.001), except for seeds ( p -value = 0.066) and legumes ( p -value = 0.174) in the case group, and seeds ( p -value = 0.178) in the control group. Other sources of phytochemical index did not show significant differences ( p -value = 0.100) in the case group.
Table 2 presents the results of both univariate and multivariate regression models assessing the relationship between phytochemical index and other variables with the risk of BV. In the univariate analysis, compared to the lowest tertile of phytochemical index, the highest tertile showed significantly lower odds of BV (OR = 0.514, 95% CI: 0.290–0.909, p -value = 0.022). Additionally, a significantly higher odds of BV was observed in individuals with a positive family history of BV compared to the reference group (OR = 3.595, 95% CI: 2.190–5.900, p -value < 0.001).
After adjusting for potential confounders (variables with p -value < 0.25 in univariate analysis), the association between phytochemical index and BV remained significant (OR = 0.349, 95% CI: 0.176–0.695, p -value = 0.003). Furthermore, each unit increase in fat intake was associated with higher odds of BV (OR = 1.008, 95% CI: 1.002–1.014, p -value = 0.006), and a positive history of BV continued to show significantly increased odds of BV (OR = 3.442, 95% CI: 2.068–5.728, p -value < 0.001).
In the current study, by investigating the dietary habits of patients with BV and controls, we found that a higher intake of dietary phytochemicals is associated with a lower risk of BV. The association remained significant even after adjustment for potential cofounders.
Research investigating the association between diet and BV is scarce. In a study on 208 Iranian women with BV, it was reported that participants who were supplemented daily with vitamin D responded better to the treatment compared to the placebo group [ 28 ]. A case-control study reported that the serum level of 25-hydroxy vitamin D was significantly lower in participants with BV compared to healthy participants [ 29 ]. It has been suggested that sufficient vitamin D could protect women against BV through the production of some antimicrobial peptides that exist in the lysosomes of macrophages and neutrophils [ 30 ]. Furthermore, It has also been shown that subclinical iron deficiency in early pregnancy might lead to BV [ 31 ]. Iron deficiency may weaken the host response against vaginal bacterial colonization [ 32 ]. Administration of probiotic supplements has also been proven efficient in treating BV patients [ 33 ]. Since probiotics produce beneficial metabolites, their impact goes beyond the well-known benefits to the intestines [ 34 , 35 ]. They could lower cholesterol levels [ 36 ] and improve the absorption of magnesium and calcium [ 37 ], all of which are said to help reduce inflammation [ 38 , 39 ].
Research abounds investigating the antimicrobial effects of phytochemicals and they were shown to be significantly efficient against a broad spectrum of bacteria. Among them, Flavonols, Flavonols, and phenolic acids are of significance. They were shown to be able to overcome the development of resistance in bacterial pathogens and fight off bacterial infections [ 40 ]. For instance, a certain flavonoid was reported to reverse the β-lactam antibiotic resistance of S. aureus [ 41 ]. Moreover, Zhao et al. investigated a specific phytochemical in green tea and found that it may inhibit the enzyme β-lactamase that blocks the effects of antibiotics such as cefotaxime and imipenem [ 42 ]. The proposed mechanisms through which they exert their impacts are as follows; they interact with the cytoplasmic membrane, alterations in the bacterial cell wall and cell membrane, reduce the pH values, suppress biofilm formation, and reduce the extracellular polysaccharide activity [ 43 , 44 ].
However, all the mentioned studies have been conducted on a handful of phytochemicals in an in-vitro setting. Despite their accuracy, the process takes a lot of time and resources. Hence, there was still a need to investigate the matter in a wide population. Then came the phytochemical index, which measures the phytochemical content in food composition databases. Subsequently, more population-based studies have emerged to investigate the effects of these compounds on chronic diseases. Most of which, yielded positive results [ 45 ]. For once, Kim et al. showed that high consumption of phytochemical-rich foods is associated with lower inflammation [ 46 ]. A case-control study indicated that higher consumption of phytochemicals is related to lower risk of pre-diabetes [ 47 ]. Another case-control study revealed a reverse association between the consumption of phytochemicals and the risk of breast cancer [ 48 ]. Finally, a meta-analysis of nine cross-sectional studies revealed that a high consumption of phytochemicals is associated with a reduced risk of overweight and obesity [ 49 ].
To the best of our knowledge, this is the first study to investigate the association between dietary-derived phytochemicals and BV which could provide further understanding of these compounds. The mentioned method for the calculation and evaluation of the dietary phytochemical index has been performed on another Iranian population study, so has been validated [ 50 ]. Though the method by which our results were generated is certainly more time and cost-efficient than in vitro studies, some limitations should be noted. First, since FFQ is a memory-dependent assessment tool, the chances of recall bias in reporting dietary intake are high. Moreover, FFQ lacks detailed information on how the food is prepared and is limited to a fixed list of foods, so it may not properly capture the eating patterns of the studied population. Second, the case-control nature of our study was another limitation, as it prevented us from inferring causality. Third, phytochemicals abound in plant foods, such as vegetables, fruit, whole grains, nuts, and legumes [ 51 ]. So the consumption of phytochemical-rich plant foods provides other beneficial nutrients such as fiber, B vitamins, folate, and Vitamin E [ 52 , 53 , 54 ]. Hence, pinning down the reported results only on phytochemicals may not be completely accurate, although we tried to nullify the effects of these nutrients by controlling for them. Finally, there might be a risk of selection bias as our subjects were enrolled from a hospital, thus, the study result might not be attributable to society as many patients may be undiagnosed or might resort to home remedies and not be hospitalized.
In summary, the findings of this study indicate that increased consumption of dietary phytochemicals is associated with a reduced risk of BV among Iranian women of reproductive age. Hence, regular intake of dietary phytochemicals could be introduced as a potentially effective approach in the prevention and management of BV. Additional research, especially longitudinal dietary studies, is required to explore the potential impact of dietary modifications on BV.
Data availability
The datasets used and/or analyzed during the current study are available from the corresponding author on reasonable request.
Brogden KA. Polymicrobial diseases of animals and humans. Polymicrobial Dis. 2002:1–20.
Hogan VK, Culhane JF, Hitti J, Rauh VA, McCollum KF, Agnew KJ. Relative performance of three methods for diagnosing bacterial vaginosis during pregnancy. Matern Child Health J. 2007;11:532–9.
Article PubMed Google Scholar
Mastromarino P, Vitali B, Mosca L. Bacterial vaginosis: a review on clinical trials with probiotics. New microbiológica. 2013;36(3):229–38.
PubMed Google Scholar
Goldenberg RL, Culhane JF, Iams JD, Romero R. Epidemiology and causes of preterm birth. Lancet. 2008;371(9606):75–84.
Article PubMed PubMed Central Google Scholar
Martel J, Ojcius DM, Ko Y-F, Ke P-Y, Wu C-Y, Peng H-H, Young JD. Hormetic effects of phytochemicals on health and longevity. Trends Endocrinol Metabolism. 2019;30(6):335–46.
Article CAS Google Scholar
Leitzmann C. Characteristics and health benefits of phytochemicals. Forsch Komplementmed. 2016;23(2):69–74.
Jaeger R, Cuny E. Terpenoids with special pharmacological significance: a review. Nat Prod Commun. 2016;11(9):1934578X1601100946.
CAS Google Scholar
Sharma BR, Kumar V, Gat Y, Kumar N, Parashar A, Pinakin DJ. Microbial maceration: a sustainable approach for phytochemical extraction. 3 Biotech. 2018;8:1–13.
Article Google Scholar
Patra AK. An overview of antimicrobial properties of different classes of phytochemicals. Diet Phytochemicals Microbes. 2012:1–32.
Park K. The role of Dietary Phytochemicals: evidence from Epidemiological studies. Nutrients. 2023;15(6).
Commenges D, Scotet V, Renaud S, Jacqmin-Gadda H, Barberger-Gateau P, Dartigues JF. Intake of flavonoids and risk of dementia. Eur J Epidemiol. 2000;16(4):357–63.
Article PubMed CAS Google Scholar
Khalesi S, Irwin C, Schubert M. Flaxseed consumption may reduce blood pressure: a systematic review and meta-analysis of controlled trials. J Nutr. 2015;145(4):758–65.
Fahim NK, Negida A, Fahim AK. Sample size calculation guide - part 3: how to calculate the sample size for an independent case-control study. Adv J Emerg Med. 2019;3(2):e20.
PubMed PubMed Central Google Scholar
Noormohammadi M, Eslamian G, Kazemi SN, Rashidkhani B. Dietary acid load, alternative healthy eating index score, and bacterial vaginosis: is there any association? A case-control study. BMC Infect Dis. 2022;22(1):803.
Article PubMed PubMed Central CAS Google Scholar
Noormohammadi M, Eslamian G, Kazemi SN, Rashidkhani B. Association between dietary patterns and bacterial vaginosis: a case–control study. Sci Rep. 2022;12(1):12199.
Noormohammadi M, Eslamian G, Kazemi SN, Rashidkhani B. Is there any association between adherence to the Mediterranean Diet and Dietary Total antioxidant capacity with bacterial vaginosis? Results from a case–control study. BMC Womens Health. 2022;22(1):244.
Noormohammadi M, Eslamian G, Kazemi SN, Rashidkhani B, Malek S. Association of Dietary Glycemic Index, Glycemic load, insulin index, and insulin load with bacterial vaginosis in Iranian women: a case-control study. Infect Dis Obstet Gynecol. 2022;2022:1225544.
Noormohammadi M, Eslamian G, Kazemi SN, Rashidkhani B, Omidifar F. Association between consumption of ultra-processed foods and bacterial vaginosis: a case-control study. Iran J Obstet Gynecol Infertility. 2022;24(12):67–76.
Google Scholar
Delaney ML, Onderdonk AB. Nugent score related to vaginal culture in pregnant women11The Microbiology and Prematurity Study Group consists of the following: Robin Ross, PhD, Mei-Ling Lee, PhD, Andrea M. DuBois, BS, Wendy Osterling, BS, and David G. Aiello, BS, Channing Laboratory, Brigham and Women’s Hospital, Boston MA; and Ruth Tuomala, MD, Ellice Lieberman, MD, Amy Cohen, BA, Dorothy Pender, RN, and, Steele L. MT(ASCP), Department of Obstetrics and Gynecology, Brigham and Women’s Hospital, Boston, MA. Obstetrics & Gynecology. 2001;98(1):79–84.
Tuddenham S, Ghanem KG, Caulfield LE, Rovner AJ, Robinson C, Shivakoti R, et al. Associations between dietary micronutrient intake and molecular-bacterial vaginosis. Reproductive Health. 2019;16(1):151.
Moghaddam MHB, Aghdam F, Asghari Jafarabadi M, Allahverdipour H, Nikookheslat S, Safarpour S. The Iranian version of International Physical Activity Questionnaire (IPAQ) in Iran: content and construct validity, factor structure, internal consistency and Stability. World Appl Sci J. 2012;18:1073–80.
Mirmiran P, Hosseini Esfahani F, Mehrabi Y, Hedayati M, Azizi F. Reliability and relative validity of an FFQ for nutrients in the Tehran lipid and glucose study. Public Health Nutr. 2010;13(5):654–62.
Azar M, Sarkisian E. Food composition table of Iran. Tehran: National Nutrition and Food Research Institute, Shaheed Beheshti University. 1980;65.
Hotz C, Abdelrahman L, Sison C, Moursi M, Loechl C. A food composition table for Central and Eastern Uganda. Volume 2. Washington, DC: International Food Policy Research Institute and International Center for Tropical Agriculture; 2012.
McCarty MF. Proposal for a dietary phytochemical index. Med Hypotheses. 2004;63(5):813–7.
Bentyaghoob S, Dehghani F, Alimohammadi A, Shateri Z, Kahrizsangi MA, Nejad ET, et al. Oxidative balance score and dietary phytochemical index can reduce the risk of colorectal cancer in Iranian population. BMC Gastroenterol. 2023;23(1):183.
Shirani M, Far ZG, Bagheri M, Nouri M. The association between non-enzymatic dietary total antioxidant capacity and phytochemical index with semen parameters: a cross-sectional study in Isfahan infertile men. J Nutr Food Secur. 2022.
Taheri M, Baheiraei A, Foroushani AR, Nikmanesh B, Modarres M. Treatment of vitamin D deficiency is an effective method in the elimination of asymptomatic bacterial vaginosis: a placebo-controlled randomized clinical trial. Indian J Med Res. 2015;141(6):799–806.
Dunlop AL, Taylor RN, Tangpricha V, Fortunato S, Menon R. Maternal vitamin D, folate, and polyunsaturated fatty acid status and bacterial vaginosis during pregnancy. Infect Dis Obstet Gynecol. 2011;2011:216217.
Bodnar LM, Krohn MA, Simhan HN. Maternal vitamin D deficiency is associated with bacterial vaginosis in the first trimester of pregnancy. J Nutr. 2009;139(6):1157–61.
Verstraelen H, Delanghe J, Roelens K, Blot S, Claeys G, Temmerman M. Subclinical iron deficiency is a strong predictor of bacterial vaginosis in early pregnancy. BMC Infect Dis. 2005;5:55.
Bendich A. Micronutrients in women’s health and immune function. Nutrition. 2001;17(10):858–67.
Thoma ME, Klebanoff MA, Rovner AJ, Nansel TR, Neggers Y, Andrews WW, Schwebke JR. Bacterial vaginosis is Associated with Variation in Dietary Indices1,2. J Nutr. 2011;141(9):1698–704.
Chen Z, Liang W, Liang J, Dou J, Guo F, Zhang D, et al. Probiotics: functional food ingredients with the potential to reduce hypertension. Front Cell Infect Microbiol. 2023;13:1220877.
Eslamparast T, Eghtesad S, Hekmatdoost A, Poustchi H. Probiotics and nonalcoholic fatty liver disease. Middle East J Dig Dis. 2013;5(3):129–36.
Momin ES, Khan AA, Kashyap T, Pervaiz MA, Akram A, Mannan V, et al. The effects of Probiotics on cholesterol levels in patients with metabolic syndrome: a systematic review. Cureus. 2023;15(4):e37567.
Sheridan PO, Bindels LB, Saulnier DM, Reid G, Nova E, Holmgren K, et al. Can prebiotics and probiotics improve therapeutic outcomes for undernourished individuals? Gut Microbes. 2014;5(1):74–82.
Klein GL. The role of calcium in inflammation-Associated Bone Resorption. Biomolecules. 2018;8(3).
Veronese N, Pizzol D, Smith L, Dominguez LJ, Barbagallo M. Effect of Magnesium supplementation on inflammatory parameters: a Meta-analysis of Randomized controlled trials. Nutrients. 2022;14(3).
Khare T, Anand U, Dey A, Assaraf YG, Chen ZS, Liu Z, Kumar V. Exploring phytochemicals for combating Antibiotic Resistance in Microbial pathogens. Front Pharmacol. 2021;12:720726.
Siriwong S, Teethaisong Y, Thumanu K, Dunkhunthod B, Eumkeb G. The synergy and mode of action of quercetin plus Amoxicillin against Amoxicillin-resistant Staphylococcus epidermidis. BMC Pharmacol Toxicol. 2016;17(1):39.
Zhao WH, Hu ZQ, Hara Y, Shimamura T. Inhibition of penicillinase by epigallocatechin gallate resulting in restoration of antibacterial activity of penicillin against penicillinase-producing Staphylococcus aureus. Antimicrob Agents Chemother. 2002;46(7):2266–8.
Bazzaz BSF, Khameneh B, Ostad MRZ, Hosseinzadeh H. In vitro evaluation of antibacterial activity of verbascoside, lemon verbena extract and caffeine in combination with gentamicin against drug-resistant Staphylococcus aureus and Escherichia coli clinical isolates. Avicenna J Phytomedicine. 2018;8(3):246.
Miklasińska-Majdanik M, Kępa M, Wojtyczka RD, Idzik D, Wąsik TJ. Phenolic compounds diminish antibiotic resistance of Staphylococcus aureus clinical strains. Int J Environ Res Public Health. 2018;15(10):2321.
Wei C, Liu L, Liu R, Dai W, Cui W, Li D. Association between the phytochemical index and overweight/obesity: a meta-analysis. Nutrients. 2022;14(7):1429.
Kim C, Park K. Association between Phytochemical Index and inflammation in Korean adults. Antioxid (Basel). 2022;11(2).
Abshirini M, Mahaki B, Bagheri F, Siassi F, Koohdani F, Sotoudeh G. Higher intake of Phytochemical-Rich Foods is inversely related to prediabetes: a case-control study. Int J Prev Med. 2018;9:64.
Ghoreishy SM, Aminianfar A, Benisi-Kohansal S, Azadbakht L, Esmaillzadeh A. Association between dietary phytochemical index and breast cancer: a case-control study. Breast Cancer. 2021;28(6):1283–91.
Wei C, Liu L, Liu R, Dai W, Cui W, Li D. Association between the Phytochemical Index and Overweight/Obesity: a Meta-analysis. Nutrients. 2022;14(7).
Amirkhizi F, Ghoreishy SM, Hamedi-Shahraki S, Asghari S. Higher dietary phytochemical index is associated with lower odds of knee osteoarthritis. Sci Rep. 2022;12(1):9059.
Liu RH. Potential synergy of phytochemicals in cancer prevention: mechanism of action. J Nutr. 2004;134(12):S3479–85.
Liu RH. Health-promoting components of fruits and vegetables in the diet. Adv Nutr. 2013;4(3):S384–92.
Hu FB. Plant-based foods and prevention of cardiovascular disease: an overview. Am J Clin Nutr. 2003;78(3):S544–51.
Borneo R, León AE. Whole grain cereals: functional components and health benefits. Food Funct. 2012;3(2):110–9.
Download references
Acknowledgements
We thank the Deputy of Shahid Beheshti University of Medical Sciences, Tehran, Iran.
Author information
Authors and affiliations.
Department of Microbiology, School of Medicine, Babol University of Medical Sciences, Babol, Iran
Aynaz Khademian
Department of Nutrition, School of Public Health, Iran University of Medical Sciences, Tehran, Iran
Morvarid Noormohammadi
Student Research Committee, Faculty of Public Health Branch, Iran University of Medical Sciences, Tehran, Iran
Department of Midwifery, Faculty of Nursing and Midwifery, Ahvaz Branch, Islamic Azad University, Ahvaz, Iran
Mozhgan Hafizi Moori
Students’ Research Committee, Shiraz University of Medical Sciences, Shiraz, Iran
Maede Makhtoomi
Department of Community Nutrition, School of Nutrition and Food Sciences, Shiraz University of Medical Sciences, Shiraz, Iran
Infertility and Reproductive Health Research Center, Health Research Institute, Babol University of Medical Sciences, Babol, Iran
Sedighe Esmaeilzadeh & Mehran Nouri
Department of Cellular and Molecular Nutrition, Faculty of Nutrition and Food Technology, National Nutrition and Food Technology Research Institute, Shahid Beheshti University of Medical Sciences, Tehran, Iran
Ghazaleh Eslamian
You can also search for this author in PubMed Google Scholar
Contributions
A.K., M.N., M.H.M., M.M. and M.N.; Contributed to writing the first draft. M.N. and G.E.; Contributed to all data and statistical analysis and interpretation of data. S.E., M.N. and G.E.; Contributed to the research concept, supervised the work, and revised the manuscript. All authors read and approved the final manuscript.
Corresponding authors
Correspondence to Mehran Nouri or Ghazaleh Eslamian .
Ethics declarations
Ethics approval and consent to participate.
This study was conducted in accordance with the ethical standards of the declaration of Helsinki and was approved by the Ethics Committee of Shahid Beheshti University of Medical Sciences (IR.SBMU.NNFTRI.REC.1399.054). All participants read and signed the informed consent form.
Competing interests
The authors declare no competing interests.
Conflict of interest
Additional information, publisher’s note.
Springer Nature remains neutral with regard to jurisdictional claims in published maps and institutional affiliations.
Rights and permissions
Open Access This article is licensed under a Creative Commons Attribution-NonCommercial-NoDerivatives 4.0 International License, which permits any non-commercial use, sharing, distribution and reproduction in any medium or format, as long as you give appropriate credit to the original author(s) and the source, provide a link to the Creative Commons licence, and indicate if you modified the licensed material. You do not have permission under this licence to share adapted material derived from this article or parts of it. The images or other third party material in this article are included in the article’s Creative Commons licence, unless indicated otherwise in a credit line to the material. If material is not included in the article’s Creative Commons licence and your intended use is not permitted by statutory regulation or exceeds the permitted use, you will need to obtain permission directly from the copyright holder. To view a copy of this licence, visit http://creativecommons.org/licenses/by-nc-nd/4.0/ .
Reprints and permissions
About this article
Cite this article.
Khademian, A., Noormohammadi, M., Moori, M.H. et al. The association between dietary phytochemical index and bacterial vaginosis risk: secondary analysis of case-control study. J Health Popul Nutr 43 , 135 (2024). https://doi.org/10.1186/s41043-024-00631-2
Download citation
Received : 05 July 2024
Accepted : 20 August 2024
Published : 31 August 2024
DOI : https://doi.org/10.1186/s41043-024-00631-2
Share this article
Anyone you share the following link with will be able to read this content:
Sorry, a shareable link is not currently available for this article.
Provided by the Springer Nature SharedIt content-sharing initiative
- Phytochemicals
- Dietary phytochemicals
- Phytonutrients
- Bacterial vaginosis
- Bacterial vaginitis
Journal of Health, Population and Nutrition
ISSN: 2072-1315
- General enquiries: [email protected]

IMAGES
VIDEO
COMMENTS
The data collection plan needs to be broadly defined and open during data collection. Sampling strategies should be chosen in such a way that they yield rich information and are consistent with the methodological approach used. Data saturation determines sample size and is different for each study. The most commonly used data collection methods are participant observation, face-to-face in ...
Sampling can be divided in a number of different ways. At a basic level, with the exception of total population sampling you will often see the divide between random sampling of a representative population and non-random sampling. Clearly, for many more quantitative- minded researchers, non-random sampling is the second-choice approach as it creates potential issues of 'bias'. However, in ...
Sampling justification and logic Any senior researcher, or seasoned mentor, has a practiced response to the 'how many' question. Mine tends to start with a reminder about the different philosophical assumptions undergirding qualitative and quantitative research projects ( Staller, 2013 ). As Abrams (2010) points out, this difference leads to "major differences in sampling goals and ...
Purposeful sampling is widely used in qualitative research for the identification and selection of information-rich cases related to the phenomenon of interest. Although there are several different purposeful sampling strategies, criterion sampling appears ...
Purposive sampling has a long developmental history and there are as many views that it is simple and straightforward as there are about its complexity. The reason for purposive sampling is the better matching of the sample to the aims and objectives of the research, thus improving the rigour of the study and trustworthiness of the data and ...
Abstract. Knowledge of sampling methods is essential to design quality research. Critical questions are provided to help researchers choose a sampling method. This article reviews probability and non-probability sampling methods, lists and defines specific sampling techniques, and provides pros and cons for consideration.
Understand the pros and cons of different sampling techniques and how to choose the right one for your qualitative research project.
Purposive Sampling. Purposive (or purposeful) sampling is a non-probability technique used to deliberately select the best sources of data to meet the purpose of the study. Purposive sampling is sometimes referred to as theoretical or selective or specific sampling. Theoretical sampling is used in qualitative research when a study is designed ...
This innovative book critically evaluates widely used sampling strategies, identifying key theoretical assumptions and considering how empirical and theoretical claims are made from these diverse methods. Nick Emmel presents a groundbreaking reworking of sampling and choosing cases in qualitative research. Drawing on international case studies ...
Sampling in case study research involves decisions that the researchers make regarding sampling strategies, the number of case studies, and the definition of the unit of analysis. It is central to theory-building and ... Mills, A. J., Durepos, G., & Wiebe, E. (2010).
Probability sampling methods Probability sampling means that every member of the population has a chance of being selected. It is mainly used in quantitative research. If you want to produce results that are representative of the whole population, probability sampling techniques are the most valid choice. There are four main types of probability sample. 1. Simple random sampling In a simple ...
Results Presenting individual case studies has highlighted how purposive sampling can be integrated into varying contexts dependent on study design. The sampling strategies clearly situate each study in terms of trustworthiness for data collection and analysis. The selected approach to purposive sampling used in each case aligns to the research methodology, aims and objectives, thus addressing ...
Learn about different sampling methods in qualitative research from this PDF chapter. Find and cite relevant research on ResearchGate.
Learn about the most popular sampling methods and strategies, including probability and non-probability-based methods, including examples.
Qualitative studies use specific tools and techniques (methods) to sample people, organizations, or whatever is to be examined. The methodology guides the selection of tools and techniques for sampling, data analysis, quality assurance, etc. These all vary according to the purpose and design of the study and the RQ.
Sampling is a critical element of research design. Different methods can be used for sample selection to ensure that members of the study population reflect both the source and target populations, including probability and non-probability sampling. Power and sample size are used to determine the number of subjects needed to answer the research ...
Qualitative sampling methods differ from quantitative sampling methods. It is important that one understands those differences, as well as, appropriate qualitative sampling techniques. Appropriate sampling choices enhance the rigor of qualitative research studies. These types of sampling strategies are presented, along with the pros and cons of ...
This paper will examine the available methods in sampling participants for qualitative study. Specifically, the paper will discuss the sampling frame suitable for case study, such as single-case (holistic and embedded), multi-case, and a snowball or network sampling procedure. Discussion will also involve challenges anticipated for each ...
Introduction Considerations of sampling are fundamental to any empirical study. However, in studies based on qualitative research interviews, sampling issues are rarely discussed. Possible reasons include a lack of universal 'rules of thumb' governing sampling considerations and the diversity of approaches to qualitative inquiry.
Furthermore, as there are different types of sampling techniques/methods, researcher needs to understand the differences to select the proper sampling method for the research.
Specifically, the paper will discuss the sampling frame suitable for case study, such as single-case (holistic and embedded), multi-case, and a snowball or network sampling procedure.
What are sampling procedures? Sampling in qualitative research has a different meaning than it does in quantitative research. Qualitative sampling, you are looking to find a group of individuals or a culture or a social organization in which you can get rich description of the load experience of either the question under inquiry or the culture or social organization under inquiry.
In 2013, Roshanian et al. 28 adopted the Latin hypercube sampling (LHS) method and assumed that the uncertain variables were normally distributed; this method was used to select the sample values ...
The main methodological issue that influences the generalizability of clinical research findings is the sampling method. In this educational article, we are explaining the different sampling methods in clinical research. Key Words: Research design, sampling studies, evidence-based medicine, population surveillance, education Go to:
Sampling distributions are the principal means for structuring and measuring sample-to-sample variability, but students do not readily distinguish between sample and sampling distributions (Case & Jacobbe, Citation 2018; Chance et al., Citation 2004; van Dijke-Droogers et al., Citation 2021 a). This difficulty suggests the need for coherent and ...
Abstract A case study is one of the most commonly used methodologies of social research. This article attempts to look into the various dimensions of a case study research strategy, the different epistemological strands which determine the particular case study type and approach adopted in the field, discusses the factors which can enhance the effectiveness of a case study research, and the ...
Case study analysis. ... The sampling method used in this paper improves the diagnostic accuracy by 7.7967%, 2.5316%, and 1.8987% compared to ADASYN, SMOTE, and random oversampling, respectively ...
Background: Wearable digital health technologies and mobile apps (personal digital health technologies [DHTs]) hold great promise for transforming health research and care. However, engagement in personal DHT research is poor. Objective: The objective of this paper is to describe how participant engagement techniques and different study designs affect participant adherence, retention, and ...
Students then presented their findings in a case study and oral presentation to members of ClientEarth, including the organization's North American head and members of its European team. The project helped identify the strengths and weaknesses of potential new strategies for increasing corporate accountability in the fight against climate change.
Additional research, especially longitudinal dietary studies, is required to explore the potential impact of dietary modifications on BV. ... This case-control study was conducted at the gynecological clinic of Imam Hossein Hospital using a convenience sampling method from November 2020 to June 2021. To diagnose BV, all participants underwent ...