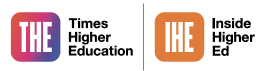

How to develop a graphical framework to chart your research
Graphic representations or frameworks can be powerful tools to explain research processes and outcomes. David Waller explains how researchers can develop effective visual models to chart their work

David Waller
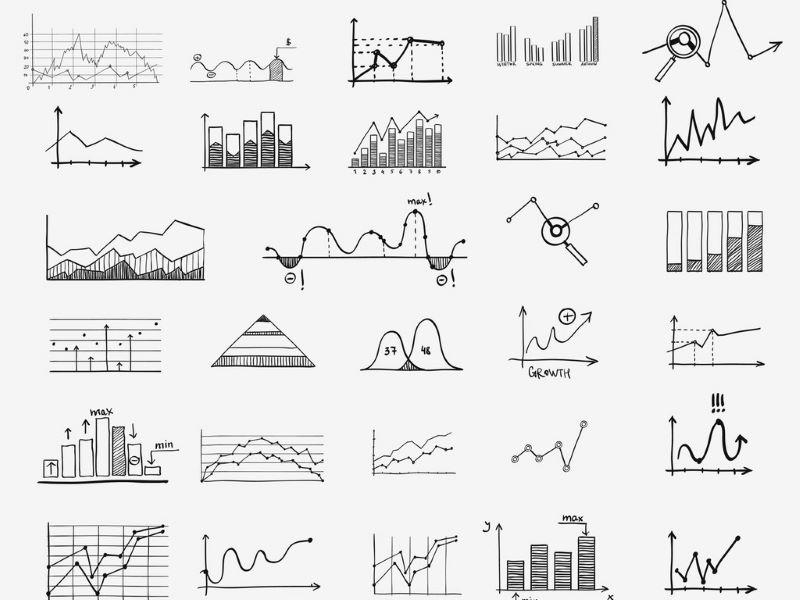
You may also like
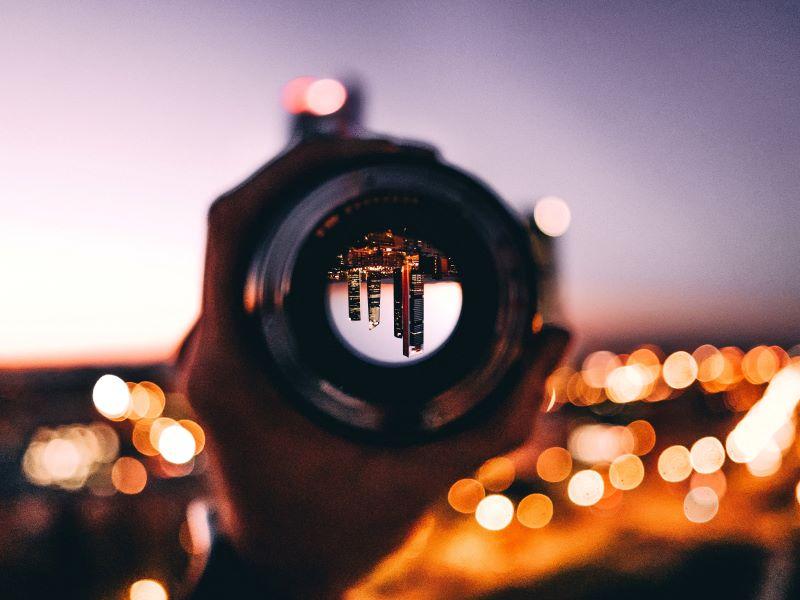
Popular resources
.css-1txxx8u{overflow:hidden;max-height:81px;text-indent:0px;} Change is coming, whether higher education likes it or not
Ai and assessment redesign: a four-step process, upgrade your teaching: four instant improvement tips, the cruel optimism of research careers: how to support contract workers, create an onboarding programme for neurodivergent students.
While undertaking a study, researchers can uncover insights, connections and findings that are extremely valuable to anyone likely to read their eventual paper. Thus, it is important for the researcher to clearly present and explain the ideas and potential relationships. One important way of presenting findings and relationships is by developing a graphical conceptual framework.
A graphical conceptual framework is a visual model that assists readers by illustrating how concepts, constructs, themes or processes work. It is an image designed to help the viewer understand how various factors interrelate and affect outcomes, such as a chart, graph or map.
These are commonly used in research to show outcomes but also to create, develop, test, support and criticise various ideas and models. The use of a conceptual framework can vary depending on whether it is being used for qualitative or quantitative research.
- Using literature reviews to strengthen research: tips for PhDs and supervisors
- Get your research out there: 7 strategies for high-impact science communication
- Understanding peer review: what it is, how it works and why it is important
There are many forms that a graphical conceptual framework can take, which can depend on the topic, the type of research or findings, and what can best present the story.
Below are examples of frameworks based on qualitative and quantitative research.
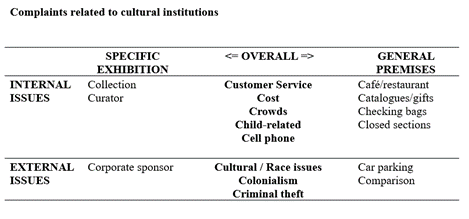
As shown by the table below, in qualitative research the conceptual framework is developed at the end of the study to illustrate the factors or issues presented in the qualitative data. It is designed to assist in theory building and the visual understanding of the exploratory findings. It can also be used to develop a framework in preparation for testing the proposition using quantitative research.
In quantitative research a conceptual framework can be used to synthesise the literature and theoretical concepts at the beginning of the study to present a model that will be tested in the statistical analysis of the research.
Introduction | Introduction |
Background/lit review | Background/lit review |
Methodology |
|
Findings | Methodology |
Discussion | Findings |
| Discussion |
Conclusion | Conclusion |
It is important to understand that the role of a conceptual framework differs depending on the type of research that is being undertaken.
So how should you go about creating a conceptual framework? After undertaking some studies where I have developed conceptual frameworks, here is a simple model based on “Six Rs”: Review, Reflect, Relationships, Reflect, Review, and Repeat.
Process for developing conceptual frameworks:
Review: literature/themes/theory.
Reflect: what are the main concepts/issues?
Relationships: what are their relationships?
Reflect: does the diagram represent it sufficiently?
Review: check it with theory, colleagues, stakeholders, etc.
Repeat: review and revise it to see if something better occurs.
This is not an easy process. It is important to begin by reviewing what has been presented in previous studies in the literature or in practice. This provides a solid background to the proposed model as it can show how it relates to accepted theoretical concepts or practical examples, and helps make sure that it is grounded in logical sense.
It can start with pen and paper, but after reviewing you should reflect to consider if the proposed framework takes into account the main concepts and issues, and the potential relationships that have been presented on the topic in previous works.
It may take a few versions before you are happy with the final framework, so it is worth continuing to reflect on the model and review its worth by reassessing it to determine if the model is consistent with the literature and theories. It can also be useful to discuss the idea with colleagues or to present preliminary ideas at a conference or workshop – be open to changes.
Even after you come up with a potential model it is good to repeat the process to review the framework and be prepared to revise it as this can help in refining the model. Over time you may develop a number of models with each one superseding the previous one.
A concern is that some students hold on to the framework they first thought of and worry that developing or changing it will be seen as a weakness in their research. However, a revised and refined model can be an important factor in justifying the value of the research.
Plenty of possibilities and theoretical topics could be considered to enhance the model. Whether it ultimately supports the theoretical constructs of the research will be dependent on what occurs when it is tested. As social psychologist, Kurt Lewin, famously said “ There's nothing so practical as good theory ”.
The final result after doing your reviewing and reflecting should be a clear graphical presentation that will help the reader understand what the research is about as well as where it is heading.
It doesn’t need to be complex. A simple diagram or table can clarify the nature of a process and help in its analysis, which can be important for the researcher when communicating to their audience. As the saying goes: “ A picture is worth 1000 words ”. The same goes for a good conceptual framework, when explaining a research process or findings.
David Waller is an associate professor at the University of Technology Sydney .
If you found this interesting and want advice and insight from academics and university staff delivered direct to your inbox each week, sign up for the THE Campus newsletter .
Change is coming, whether higher education likes it or not
The iscanner app supports the academic community in information sharing and management, support students with caring responsibilities in he, step up to support students with disabilities, emotions and learning: what role do emotions play in how and why students learn, the secret to timely, relevant, inclusive communication with students.
Register for free
and unlock a host of features on the THE site
- Business Essentials
- Leadership & Management
- Credential of Leadership, Impact, and Management in Business (CLIMB)
- Entrepreneurship & Innovation
- Digital Transformation
- Finance & Accounting
- Business in Society
- For Organizations
- Support Portal
- Media Coverage
- Founding Donors
- Leadership Team
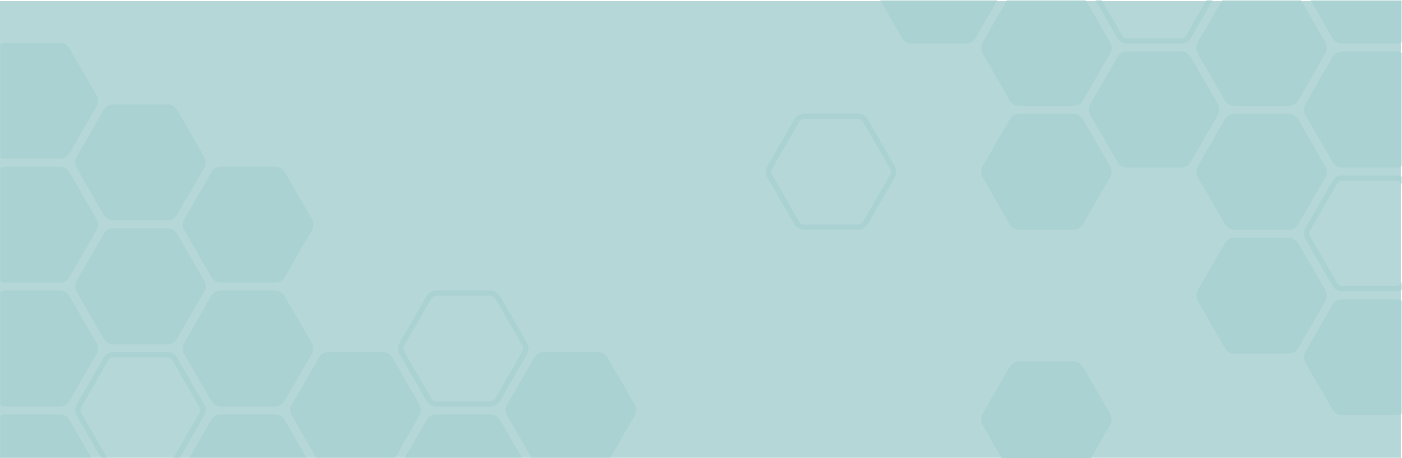
- Harvard Business School →
- HBS Online →
- Business Insights →
Business Insights
Harvard Business School Online's Business Insights Blog provides the career insights you need to achieve your goals and gain confidence in your business skills.
- Career Development
- Communication
- Decision-Making
- Earning Your MBA
- Negotiation
- News & Events
- Productivity
- Staff Spotlight
- Student Profiles
- Work-Life Balance
- AI Essentials for Business
- Alternative Investments
- Business Analytics
- Business Strategy
- Business and Climate Change
- Creating Brand Value
- Design Thinking and Innovation
- Digital Marketing Strategy
- Disruptive Strategy
- Economics for Managers
- Entrepreneurship Essentials
- Financial Accounting
- Global Business
- Launching Tech Ventures
- Leadership Principles
- Leadership, Ethics, and Corporate Accountability
- Leading Change and Organizational Renewal
- Leading with Finance
- Management Essentials
- Negotiation Mastery
- Organizational Leadership
- Power and Influence for Positive Impact
- Strategy Execution
- Sustainable Business Strategy
- Sustainable Investing
- Winning with Digital Platforms
17 Data Visualization Techniques All Professionals Should Know
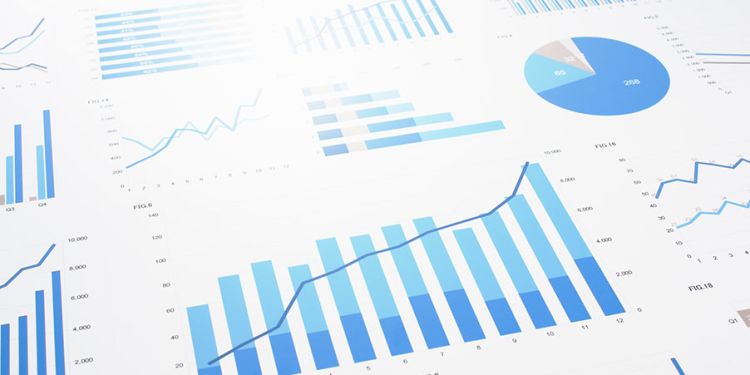
- 17 Sep 2019
There’s a growing demand for business analytics and data expertise in the workforce. But you don’t need to be a professional analyst to benefit from data-related skills.
Becoming skilled at common data visualization techniques can help you reap the rewards of data-driven decision-making , including increased confidence and potential cost savings. Learning how to effectively visualize data could be the first step toward using data analytics and data science to your advantage to add value to your organization.
Several data visualization techniques can help you become more effective in your role. Here are 17 essential data visualization techniques all professionals should know, as well as tips to help you effectively present your data.
Access your free e-book today.
What Is Data Visualization?
Data visualization is the process of creating graphical representations of information. This process helps the presenter communicate data in a way that’s easy for the viewer to interpret and draw conclusions.
There are many different techniques and tools you can leverage to visualize data, so you want to know which ones to use and when. Here are some of the most important data visualization techniques all professionals should know.
Data Visualization Techniques
The type of data visualization technique you leverage will vary based on the type of data you’re working with, in addition to the story you’re telling with your data .
Here are some important data visualization techniques to know:
- Gantt Chart
- Box and Whisker Plot
- Waterfall Chart
- Scatter Plot
- Pictogram Chart
- Highlight Table
- Bullet Graph
- Choropleth Map
- Network Diagram
- Correlation Matrices
1. Pie Chart

Pie charts are one of the most common and basic data visualization techniques, used across a wide range of applications. Pie charts are ideal for illustrating proportions, or part-to-whole comparisons.
Because pie charts are relatively simple and easy to read, they’re best suited for audiences who might be unfamiliar with the information or are only interested in the key takeaways. For viewers who require a more thorough explanation of the data, pie charts fall short in their ability to display complex information.
2. Bar Chart

The classic bar chart , or bar graph, is another common and easy-to-use method of data visualization. In this type of visualization, one axis of the chart shows the categories being compared, and the other, a measured value. The length of the bar indicates how each group measures according to the value.
One drawback is that labeling and clarity can become problematic when there are too many categories included. Like pie charts, they can also be too simple for more complex data sets.
3. Histogram

Unlike bar charts, histograms illustrate the distribution of data over a continuous interval or defined period. These visualizations are helpful in identifying where values are concentrated, as well as where there are gaps or unusual values.
Histograms are especially useful for showing the frequency of a particular occurrence. For instance, if you’d like to show how many clicks your website received each day over the last week, you can use a histogram. From this visualization, you can quickly determine which days your website saw the greatest and fewest number of clicks.
4. Gantt Chart

Gantt charts are particularly common in project management, as they’re useful in illustrating a project timeline or progression of tasks. In this type of chart, tasks to be performed are listed on the vertical axis and time intervals on the horizontal axis. Horizontal bars in the body of the chart represent the duration of each activity.
Utilizing Gantt charts to display timelines can be incredibly helpful, and enable team members to keep track of every aspect of a project. Even if you’re not a project management professional, familiarizing yourself with Gantt charts can help you stay organized.
5. Heat Map
A heat map is a type of visualization used to show differences in data through variations in color. These charts use color to communicate values in a way that makes it easy for the viewer to quickly identify trends. Having a clear legend is necessary in order for a user to successfully read and interpret a heatmap.
There are many possible applications of heat maps. For example, if you want to analyze which time of day a retail store makes the most sales, you can use a heat map that shows the day of the week on the vertical axis and time of day on the horizontal axis. Then, by shading in the matrix with colors that correspond to the number of sales at each time of day, you can identify trends in the data that allow you to determine the exact times your store experiences the most sales.
6. A Box and Whisker Plot

A box and whisker plot , or box plot, provides a visual summary of data through its quartiles. First, a box is drawn from the first quartile to the third of the data set. A line within the box represents the median. “Whiskers,” or lines, are then drawn extending from the box to the minimum (lower extreme) and maximum (upper extreme). Outliers are represented by individual points that are in-line with the whiskers.
This type of chart is helpful in quickly identifying whether or not the data is symmetrical or skewed, as well as providing a visual summary of the data set that can be easily interpreted.
7. Waterfall Chart

A waterfall chart is a visual representation that illustrates how a value changes as it’s influenced by different factors, such as time. The main goal of this chart is to show the viewer how a value has grown or declined over a defined period. For example, waterfall charts are popular for showing spending or earnings over time.
8. Area Chart

An area chart , or area graph, is a variation on a basic line graph in which the area underneath the line is shaded to represent the total value of each data point. When several data series must be compared on the same graph, stacked area charts are used.
This method of data visualization is useful for showing changes in one or more quantities over time, as well as showing how each quantity combines to make up the whole. Stacked area charts are effective in showing part-to-whole comparisons.
9. Scatter Plot

Another technique commonly used to display data is a scatter plot . A scatter plot displays data for two variables as represented by points plotted against the horizontal and vertical axis. This type of data visualization is useful in illustrating the relationships that exist between variables and can be used to identify trends or correlations in data.
Scatter plots are most effective for fairly large data sets, since it’s often easier to identify trends when there are more data points present. Additionally, the closer the data points are grouped together, the stronger the correlation or trend tends to be.
10. Pictogram Chart
Pictogram charts , or pictograph charts, are particularly useful for presenting simple data in a more visual and engaging way. These charts use icons to visualize data, with each icon representing a different value or category. For example, data about time might be represented by icons of clocks or watches. Each icon can correspond to either a single unit or a set number of units (for example, each icon represents 100 units).
In addition to making the data more engaging, pictogram charts are helpful in situations where language or cultural differences might be a barrier to the audience’s understanding of the data.
11. Timeline

Timelines are the most effective way to visualize a sequence of events in chronological order. They’re typically linear, with key events outlined along the axis. Timelines are used to communicate time-related information and display historical data.
Timelines allow you to highlight the most important events that occurred, or need to occur in the future, and make it easy for the viewer to identify any patterns appearing within the selected time period. While timelines are often relatively simple linear visualizations, they can be made more visually appealing by adding images, colors, fonts, and decorative shapes.
12. Highlight Table

A highlight table is a more engaging alternative to traditional tables. By highlighting cells in the table with color, you can make it easier for viewers to quickly spot trends and patterns in the data. These visualizations are useful for comparing categorical data.
Depending on the data visualization tool you’re using, you may be able to add conditional formatting rules to the table that automatically color cells that meet specified conditions. For instance, when using a highlight table to visualize a company’s sales data, you may color cells red if the sales data is below the goal, or green if sales were above the goal. Unlike a heat map, the colors in a highlight table are discrete and represent a single meaning or value.
13. Bullet Graph

A bullet graph is a variation of a bar graph that can act as an alternative to dashboard gauges to represent performance data. The main use for a bullet graph is to inform the viewer of how a business is performing in comparison to benchmarks that are in place for key business metrics.
In a bullet graph, the darker horizontal bar in the middle of the chart represents the actual value, while the vertical line represents a comparative value, or target. If the horizontal bar passes the vertical line, the target for that metric has been surpassed. Additionally, the segmented colored sections behind the horizontal bar represent range scores, such as “poor,” “fair,” or “good.”
14. Choropleth Maps

A choropleth map uses color, shading, and other patterns to visualize numerical values across geographic regions. These visualizations use a progression of color (or shading) on a spectrum to distinguish high values from low.
Choropleth maps allow viewers to see how a variable changes from one region to the next. A potential downside to this type of visualization is that the exact numerical values aren’t easily accessible because the colors represent a range of values. Some data visualization tools, however, allow you to add interactivity to your map so the exact values are accessible.
15. Word Cloud

A word cloud , or tag cloud, is a visual representation of text data in which the size of the word is proportional to its frequency. The more often a specific word appears in a dataset, the larger it appears in the visualization. In addition to size, words often appear bolder or follow a specific color scheme depending on their frequency.
Word clouds are often used on websites and blogs to identify significant keywords and compare differences in textual data between two sources. They are also useful when analyzing qualitative datasets, such as the specific words consumers used to describe a product.
16. Network Diagram

Network diagrams are a type of data visualization that represent relationships between qualitative data points. These visualizations are composed of nodes and links, also called edges. Nodes are singular data points that are connected to other nodes through edges, which show the relationship between multiple nodes.
There are many use cases for network diagrams, including depicting social networks, highlighting the relationships between employees at an organization, or visualizing product sales across geographic regions.
17. Correlation Matrix

A correlation matrix is a table that shows correlation coefficients between variables. Each cell represents the relationship between two variables, and a color scale is used to communicate whether the variables are correlated and to what extent.
Correlation matrices are useful to summarize and find patterns in large data sets. In business, a correlation matrix might be used to analyze how different data points about a specific product might be related, such as price, advertising spend, launch date, etc.
Other Data Visualization Options
While the examples listed above are some of the most commonly used techniques, there are many other ways you can visualize data to become a more effective communicator. Some other data visualization options include:
- Bubble clouds
- Circle views
- Dendrograms
- Dot distribution maps
- Open-high-low-close charts
- Polar areas
- Radial trees
- Ring Charts
- Sankey diagram
- Span charts
- Streamgraphs
- Wedge stack graphs
- Violin plots

Tips For Creating Effective Visualizations
Creating effective data visualizations requires more than just knowing how to choose the best technique for your needs. There are several considerations you should take into account to maximize your effectiveness when it comes to presenting data.
Related : What to Keep in Mind When Creating Data Visualizations in Excel
One of the most important steps is to evaluate your audience. For example, if you’re presenting financial data to a team that works in an unrelated department, you’ll want to choose a fairly simple illustration. On the other hand, if you’re presenting financial data to a team of finance experts, it’s likely you can safely include more complex information.
Another helpful tip is to avoid unnecessary distractions. Although visual elements like animation can be a great way to add interest, they can also distract from the key points the illustration is trying to convey and hinder the viewer’s ability to quickly understand the information.
Finally, be mindful of the colors you utilize, as well as your overall design. While it’s important that your graphs or charts are visually appealing, there are more practical reasons you might choose one color palette over another. For instance, using low contrast colors can make it difficult for your audience to discern differences between data points. Using colors that are too bold, however, can make the illustration overwhelming or distracting for the viewer.
Related : Bad Data Visualization: 5 Examples of Misleading Data
Visuals to Interpret and Share Information
No matter your role or title within an organization, data visualization is a skill that’s important for all professionals. Being able to effectively present complex data through easy-to-understand visual representations is invaluable when it comes to communicating information with members both inside and outside your business.
There’s no shortage in how data visualization can be applied in the real world. Data is playing an increasingly important role in the marketplace today, and data literacy is the first step in understanding how analytics can be used in business.
Are you interested in improving your analytical skills? Learn more about Business Analytics , our eight-week online course that can help you use data to generate insights and tackle business decisions.
This post was updated on January 20, 2022. It was originally published on September 17, 2019.

About the Author
We're sorry but you will need to enable Javascript to access all of the features of this site.
Stanford Online
Probabilistic graphical models 1: representation.
Stanford School of Engineering
- Engineering
- Artificial Intelligence
- Computer Science & Security
- Business & Management
- Energy & Sustainability
- Data Science
- Medicine & Health
- Explore All
- Technical Support
- Master’s Application FAQs
- Master’s Student FAQs
- Master's Tuition & Fees
- Grades & Policies
- HCP History
- Graduate Application FAQs
- Graduate Student FAQs
- Graduate Tuition & Fees
- Community Standards Review Process
- Academic Calendar
- Exams & Homework FAQs
- Enrollment FAQs
- Tuition, Fees, & Payments
- Custom & Executive Programs
- Free Online Courses
- Free Content Library
- School of Engineering
- Graduate School of Education
- Stanford Doerr School of Sustainability
- School of Humanities & Sciences
- Stanford Human Centered Artificial Intelligence (HAI)
- Graduate School of Business
- Stanford Law School
- School of Medicine
- Learning Collaborations
- Stanford Credentials
- What is a digital credential?
- Grades and Units Information
- Our Community
- Get Course Updates
Introduction to Graphical Models
A gentle introduction to graphical models, probabilistic programming, and mcmc using a simple linear regression example., will freyman, last modified on june 2, 2024.
Overview Prerequisites Getting Started with RevBayes and Rev Language Syntax
Data files and scripts Other linear_regression.Rev linear_regression_generative.Rev x.csv y.csv
RevBayes uses a graphical model framework in which all probabilistic models, including phylogenetic models, are comprised of modular components that can be assembled in a myriad of ways. RevBayes provides a highly flexible language called Rev that users employ to specify their own custom graphical models.
This tutorial is intended to be a gentle introduction on how to use Rev to specify graphical models. Additionally we’ll cover how to use Rev to specify the Markov chain Monte Carlo (MCMC) algorithms used to perform inference with the model. We will focus on a simple linear regression example, and use RevBayes to estimate the posterior distributions of our parameters.
Why Graphical Models?
RevBayes is a fundamental reconception of phylogenetic software. Most phylogenetic software have default settings that allow a user to run an analysis without truly understanding the assumptions of the model. RevBayes , on the other hand, has no defaults and is a complete blank slate when started. RevBayes requires users to fully specify the model they want to use for their analysis. This means the learning curve is steep, however there are a number of benefits:
Transparency: All the modeling assumptions are explicitly specified in RevBayes . The Rev script that runs an analysis makes these assumptions clear and can be easily shared. The assumptions can easily be modified in the Rev script and then the analysis can be rerun to see how changes affect the results. There is no reliance on “defaults” that may change with different versions of the software.
Flexibility: Users are not limited by a small set of models the programmers hard coded, instead users can specify their own custom models uniquely tailored to their hypotheses and datasets.
Modularity: Each model component can be combined with others in an endless number of new ways like a LEGO kit. Testing many complex evolutionary hypotheses require tying different models together. For example, suppose you wish to test how the effect of biographic range on trait evolution changes through time. In RevBayes you could simultaneously infer a time-calibrated phylogeny and estimate biogeography-dependent trait evolution using molecular data, biogeographic range data, and morphological data from both fossils and extant lineages.
What is a Graphical Model?

A graphical model is a way to represent a joint multivariate probability distribution as a graph. Here we mean graph in the mathematical sense of a set of nodes (vertices) and edges. In a graphical model, the nodes represent variables and the edges represent conditional dependencies among the variables. There are three important types of variables:
- Constant nodes: represents a fixed value that will not change.
- Stochastic nodes: represents a random variable with a value drawn from a probability distribution.
- Deterministic nodes: represents a deterministic transformation of the values of other nodes.
In the graphical modeling framework observed data is simply a variable with an observed value. To specify that a node has an observed value associated with it we say that the node is clamped , or fixed, to the observed value. illustrates the graphical model that represents the joint probability distribution
where \(\mathcal{D}\) is the vector of observed data points \(X_1,\dots,X_N\).
Nearly any probabilistic model can be represented as a graphical model: neural networks, classification models, time series models, and of course phylogenetic models! In some literature the terms Bayesian networks, belief networks, or causal networks are sometimes used to refer to graphical models.
Visual Representation
The statistics literature has developed a rich visual representation for graphical models. Visually representing graphical models can be useful for communication and pedagogy. We explain the notation used in the visual representation of these models only briefly (see ), and enourage readers to see Höhna et al. (2014) for more details. As we will discuss below, representing graphical models in computer code (using the Rev language) will likely be the most useful aspect of graphical models to most readers.

Phylogenetic Graphical Models
In phylogenetics, observations about different species are not considered independent data points due to their shared evolutionary history. So in a phylogenetic probabilistic model the topology of the tree determines the conditional dependencies among variables. This can be represented as a graphical model as in (left).
Phylogenetic models are often highly complex with hundreds of variables. Not only do we model the conditional dependencies due to shared evolutionary history (the tree topology), but we also commonly model character evolution (nucleotide substitution models, etc.), branching processes that determine the times between speciation events (birth-death processes), and many other aspects of the evolutionary process. With graphical models we can think of each part of these models as discrete components that can be combined in a myriad of ways to assemble different phylogenetic models ( right).

Probabilistic Programming
To describe complex probabilistic models and perform computational tasks with them, we need a way to formally specify the models in a computer. Probabilistic programming languages were designed exactly for this purpose. A probabilistic programming language is a tool for probabilistic inference that:
- formally specifies graphical models, and
- specifies the inference algorithms used with the model.
Probabilistic programming languages are being actively developed within the statistics and machine learning communities. Some of the most common are Stan , JAGS , Edward , and PyMC3 . While these are all excellent tools, they are all unsuitable for phylogenetic models since the tree topology itself must be treated as a random variable to be inferred.
The Rev Probabilistic Programming Language
RevBayes provides its own probabilistic programming language called Rev . While Rev focuses on phylogenetic models, nearly any type of probabilistic model can be programmed in Rev making it a highly flexible probabilistic computing environment. Most Rev scripts consist of two different parts:
- Model specification. This part of the script defines the constant, stochastic, and determinstic nodes that make up the model.
- Inference algorithm specification. This part of the script specifies what sort of inference algorithm we want to use with the model. Typically this is a Markov chain Monte Carlo algorithm, and we need to specify what sort of proposals (or moves) will operate on each variable.
In more complex Rev scripts, these two different elements (model specification and infernence algorithm specification) will be woven together. In the example for this tutorial we will keep the two parts separate.
Linear Regression Example
To demonstrate how to use the Rev language to specify a graphical model, we will start with a simple non-phylogenetic model. This tutorial will show both how to specify linear regression as a graphical model, and how to perform Bayesian inference over the model using MCMC.
Tutorial Format
All command-line text, including all Rev syntax, are given in monotype font . Furthermore, blocks of Rev code that are needed to build the model, specify the analysis, or execute the run are given in separate shaded boxes. For example, we will instruct you to create a new variable called n that is equal to 10 using the <- operator like this:
Setup Your Files
Make yourself familiar with the example script called linear_regression.Rev which shows the code for the following sections. Then, start a new and empty script in your text editor and follow each step provided as below. Name the script file my_linear_regression.Rev or anything you’d like.
You’ll also want to download the x.csv and y.csv data files and place them in a data directory.
Linear Regression as a Graphical Model

Suppose we observed the data shown in . We might hypothesize that $x$ and $y$ are related through the linear regression model
In this model $\beta$ and $\alpha$ are the regression variables (slope and y-intercept, respectively) and $\epsilon$ is an error or noise term. We can formulate this as the graphical model
Here $\mu_y$ is a deterministic variable, it is determined by whatever the values of $\beta$ and $\alpha$ are. We use the $:=$ assignment operator to designate that $\mu_y$ is deterministic. The error or noise term $\epsilon$ is represented as a normal distribution where the mean equals $\mu_y$ and the standard deviation is $\sigma_{\epsilon}$. $y$ is a stochastic variable, it has a value that is drawn from a probability distribution. This is designated by using the $\sim$ assignment operator. Since we have observed values for $y$, we will clamp $y$ to those observed values.
Bayesian Linear Regression
In our linear regression model $\beta$, $\alpha$, and $\sigma_{\epsilon}$ are the free variables we wish to estimate. To perform Bayesian inference, we need some priors!
Again, these are stochastic variables, so we use the $\sim$ assignment operator. For now we will accept these as decent uninformative priors. Later in the tutorial we will discuss how the choice of a prior can affect the outcome of the analysis.
Exercise: Using the sticks-and-arrows visual symbols explained in , draw the linear regression graphical model. See the answer in the expandable box below.
Answer: Visual Representation of the Linear Regression Model Visual representation of the linear regression graphical model. The plate (dashed rectangle) around $x_i$, $\mu_{yi}$ and $y_i$ represent the repeated variables for all the observed points. $y_i$ is a clamped (observed) stochastic node, so it is shaded. $\mu_{yi}$ is a deterministic node, so it is dashed. Here we treat $x_i$ as a constant node, so it is square. $\alpha$, $\beta$, and $\sigma$ are the stochastic variables we wish to estimate, and each of them are assigned priors distributions which have constant parameter values (the squares on the top row of the figure).
Specifying the Model in Rev
Remember that graphical models are made up of three types of nodes: stochastic, constant, and deterministic nodes. In Rev we specify the type of node by using a specific assignment operator:
- Stochastic node: n ~ dnNormal(0, 1)
- Constant node: n <- 5
- Deterministic node: n := m + 5
We will use each of these assignment operators to set up the linear regression model.
First, we read in the observed data as constant nodes:
Take a look at x_obs :
This is the vector of x-coordinates for the points plotted in .
Now we will specify the prior distributions for the stochastic nodes. These are the variables that we will estimate:
Now, for each observed value in x_obs we will create a deterministic node for mu_y and a stochastic node for y :
Take a look at y :
This produces a vector of simulated values of y ! We have specified a model that describes the process that generates y conditioned on the observed values of x . We have not clamped, or fixed, the observed values y_obs to the stochastic nodes y . In Rev all models can be used to both simulate new values and, when clamped to observed values, perform parameter inference.
In this case we are not interested in simulating new values of y , but instead we want to estimate our linear regression parameters. So let’s modify the above code to clamp the observed values to y :
Note that we have now clamped each observed value y_obs to each stochastic node y .
We have now fully specified the model, so we can begin specifying the inference algorithm.
Setting up MCMC in Rev
Here we will use the Metropolis-Hastings MCMC algorithm (Metropolis et al. 1953; Hastings 1970) to perform parameter estimation. We focus here on providing a simple overview of how to set up and tweak MCMC in RevBayes, for a more in depth introduction to MCMC please see the Introduction to Markov chain Monte Carlo (MCMC) Sampling tutorial.
The first step in setting up our MCMC algorithm is wrapping the entire model into a single variable:
Since our model is a graph in which all the model nodes are connected, we can use any model variable and RevBayes will traverse the graph to copy the entire model into the variable mymodel .
Note that we used the = assignment operator. This means that the variable mymodel is not part of the graphical model – it is not a stochastic, constant, or deterministic node. We call this a Rev workspace variable. Workspace variables are utility variables that we use for any programming task that is not specifically defining the model. Note, that unlike in R , in Rev the = and <- assignment operators have very different functions!
To sample different values of each variable, we must assign an MCMC move to each variable. Each MCMC move will propose new values of each parameter. We have three variables, so we will have three moves which we will save in a vector called moves :
Here we used simple slide moves for each variable. The slide move proposes new values for the variable by “sliding” its value within a small window determined by the delta argument. RevBayes provides many other types of moves that you will see in other tutorials. We set the weight of each move to 1, which means that each move will be performed on average once per MCMC iteration.
Next, we need to set up some monitors that will sample values during the MCMC. We will use two monitors which we save into a vector called monitors . The first monitor mnScreen prints out values to the screen, and the second monitor mnModel prints a log file.
RevBayes provides many other monitors that can be useful for different types of analyses, but these are sufficient for this example.
We can now pass the model, moves, and monitors into the mcmc function to finalize our analysis. Then we use the run member method to run the MCMC for 10000 iterations.
Note that we included the quit() command so that RevBayes will automatically quit after the MCMC has finished running.
Improving MCMC Mixing
Exercise: Now open the file output/linear_regression.log in Tracer.
You will notice that the MCMC analysis did not converge well:

We can fix this by modifying the MCMC moves we use. Let’s use a larger sliding window (the delta argument in mvSlide ). We will also increase the weight of each move to 5. This means that each move will be now be performed on average 5 times per MCMC iteration.
Exercise: Rerun the MCMC analysis with these new moves and view the log file in Tracer.
This analysis looks much better:

Prior Sensitivity

Prior distributions are a way to mathematically formalize our prior knowledge. We used normal distributions as priors for $\alpha$ and $\beta$. How did we pick these distributions? illustrates the normal distribution with different values for the standard deviation. Using a smaller standard deviation (0.1) places most of the density close to 0. This sort of prior is appropriate only if we have prior information that the parameter’s true value is close to 0, so we can call this an informative prior. Using a large standard deviation (10.0) is a highly uninformative prior. The density is diffuse and nearly uniform, allowing for a wide range of values. This is appropriate if we have very little idea what the true value of the parameter is.
In RevBayes it is easy to modify the priors used in an analysis and rerun the analysis.
Exercise: Try rerunning the linear regression exercise using highly informative priors (standard deviation set to 0.1) on beta and alpha as shown below.
shows the posterior estimates when using these priors. Compare those results with those shown in . Using informative priors that are incorrect can badly bias the results.

Exercise: Try running the analysis again with highly uninformative priors (10.0).
These results are highly similar to our original estimates shown in . Our original priors (that had a standard deviation of 1.0) did not introduce any bias. Typically the trade off is between informative priors that may introduce bias and uninformative priors that may increase the variance (uncertainty) of our estimates.
Generative vs Discriminative Models
Probabilistic models can be understood as either discriminative or generative models. The distinction between the two can be useful in phylogenetics where different analyses often make use of these different types of models. RevBayes enables us to specify both types of models.
Discriminative Models
Discriminative (or conditional) models involve a response variable conditioned on a predictor variable. The model represents the conditional distribution $p(y|x)$ and so makes fewer assumptions about the data: it is not necessary to specify $p(x)$. The linear regression example we coded in Rev was a discriminative model because it conditioned on the observed values of $x$. In other words the model could simulate values of $y$ conditioned on the observed values of $x$, but it could not simulate values of $x$.
In phylogenetics we often use discriminative models when we condition over a fixed tree (or set of trees):
- estimating divergence times over a fixed topology
- estimating ancestral states on a fixed tree
- estimating shifts in diversification rates over a fixed tree
We can set up all these discriminative models in RevBayes .
Generative Models
Generative models model the entire process used to generate the data. So these models represent the joint distribution $p(x, y)$, and therefore they must make more assumptions about the data: we need to define $p(x)$. This allows for a richer representation of the relations between variables. Additionally these models are more powerful; they allow us to compute $p(y|x)$ or $p(x|y)$ and to simulate both $x$ and $y$.
In phylogenetics we use fully generative models when we:
- jointly estimate divergence times and the tree topology
- jointly estimate ancestral states and the tree
- jointly estimate shifting diversification rates and the tree
RevBayes is unique because it allows us to specify both highly complex fully generative models as well as their more simple discriminative forms.
A Generative Linear Regression Model
A fully generative linear regression model enables us to learn something about $x$, for example the mean and standard deviation, which we don’t get from the discriminative form. With the generative model:
- we can simulate values of both $x$ and $y$,
- both $x$ and $y$ will need to be clamped to the observed data,
- and we will need to specify a prior distribution for $x$.
Exercise: Reformulate our linear regression example so that it is a fully generative model: Draw the sticks-and-arrows diagram for a generative model and compare it to the discriminative form. See the expandable box for one solution. Code up the model in Rev and run MCMC. A solution is provided in linear_regression_generative.Rev if you get stuck.
Answer: Visual Representation of the Generative Linear Regression Model Visual representation of the generative linear regression graphical model. Compare this to . The major difference is we now treat $x_i$ as a clamped (observed) stochastic node. Additionally, we now estimate $\mu_x$ and $\sigma_x$ as stochastic variables.
RevBayes gives evolutionary biologists the tools to formalize their hypotheses as custom graphical models that represent the specific process that generated their data. This enables many evolutionary hypotheses to now be tested in a rigorous and quantitative approach. Hopefully this tutorial will help readers develop their own custom models and not use defaults ever again!
- Hastings W.K. 1970. Monte Carlo Sampling Methods Using Markov Chains and Their Applications. Biometrika. 57:97–109.
- Höhna S., Heath T.A., Boussau B., Landis M.J., Ronquist F., Huelsenbeck J.P. 2014. Probabilistic Graphical Model Representation in Phylogenetics. Systematic Biology. 63:753–771. 10.1093/sysbio/syu039
- Metropolis N., Rosenbluth A.W., Rosenbluth M.N., Teller A.H., Teller E. 1953. Equation of State Calculations by Fast Computing Machines. Journal of Chemical Physics. 21:1087–1092. 10.1063/1.1699114
Thank you for visiting nature.com. You are using a browser version with limited support for CSS. To obtain the best experience, we recommend you use a more up to date browser (or turn off compatibility mode in Internet Explorer). In the meantime, to ensure continued support, we are displaying the site without styles and JavaScript.
- View all journals
- Explore content
- About the journal
- Publish with us
- Sign up for alerts
- Published: 15 March 2018
A graphical and computational modeling platform for biological pathways
- Alessandra Livigni 1 na1 ,
- Laura O'Hara ORCID: orcid.org/0000-0001-7790-9261 1 , 2 na1 ,
- Marta E Polak 3 , 4 ,
- Tim Angus 1 ,
- Derek W Wright ORCID: orcid.org/0000-0003-0411-4497 1 ,
- Lee B Smith 2 , 5 &
- Tom C Freeman ORCID: orcid.org/0000-0001-5235-8483 1
Nature Protocols volume 13 , pages 705–722 ( 2018 ) Cite this article
4101 Accesses
21 Citations
14 Altmetric
Metrics details
- Bioinformatics
- Computational biology and bioinformatics
- Computer modelling
- Stochastic modelling
A major endeavor of systems biology is the construction of graphical and computational models of biological pathways as a means to better understand their structure and function. Here, we present a protocol for a biologist-friendly graphical modeling scheme that facilitates the construction of detailed network diagrams, summarizing the components of a biological pathway (such as proteins and biochemicals) and illustrating how they interact. These diagrams can then be used to simulate activity flow through a pathway, thereby modeling its dynamic behavior. The protocol is divided into four sections: (i) assembly of network diagrams using the modified Edinburgh Pathway Notation (mEPN) scheme and yEd network editing software with pathway information obtained from published literature and databases of molecular interaction data; (ii) parameterization of the pathway model within yEd through the placement of 'tokens' on the basis of the known or imputed amount or activity of a component; (iii) model testing through visualization and quantitative analysis of the movement of tokens through the pathway, using the network analysis tool Graphia Professional and (iv) optimization of model parameterization and experimentation. This is the first modeling approach that combines a sophisticated notation scheme for depicting biological events at the molecular level with a Petri net–based flow simulation algorithm and a powerful visualization engine with which to observe the dynamics of the system being modeled. Unlike many mathematical approaches to modeling pathways, it does not require the construction of a series of equations or rate constants for model parameterization. Depending on a model's complexity and the availability of information, its construction can take days to months, and, with refinement, possibly years. However, once assembled and parameterized, a simulation run, even on a large model, typically takes only seconds. Models constructed using this approach provide a means of knowledge management, information exchange and, through the computation simulation of their dynamic activity, generation and testing of hypotheses, as well as prediction of a system's behavior when perturbed.
This is a preview of subscription content, access via your institution
Access options
Access Nature and 54 other Nature Portfolio journals
Get Nature+, our best-value online-access subscription
24,99 € / 30 days
cancel any time
Subscribe to this journal
Receive 12 print issues and online access
251,40 € per year
only 20,95 € per issue
Buy this article
- Purchase on SpringerLink
- Instant access to full article PDF
Prices may be subject to local taxes which are calculated during checkout
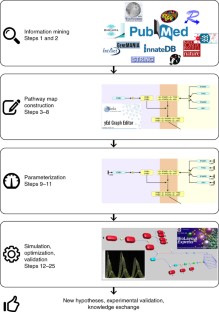
Similar content being viewed by others
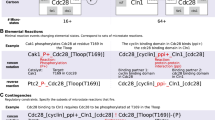
A scalable method for parameter-free simulation and validation of mechanistic cellular signal transduction network models
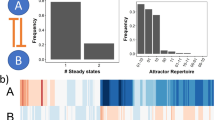
Assessing biological network dynamics: comparing numerical simulations with analytical decomposition of parameter space
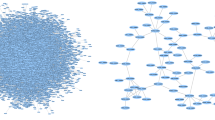
Automating parameter selection to avoid implausible biological pathway models
O'Hara, L. et al. Modelling the structure and dynamics of biological pathways. PLoS Biol. 14 , e1002530 (2016).
Article Google Scholar
Raza, S. et al. A logic-based diagram of signalling pathways central to macrophage activation. BMC Syst. Biol. 2 , 36 (2008).
Freeman, T.C., Raza, S., Theocharidis, A. & Ghazal, P. The mEPN scheme: an intuitive and flexible graphical system for rendering biological pathways. BMC Syst. Biol. 4 , 65 (2010).
Kitano, H., Funahashi, A., Matsuoka, Y. & Oda, K. Using process diagrams for the graphical representation of biological networks. Nat. Biotechnol. 23 , 961–966 (2005).
Article CAS Google Scholar
Kohn, K.W., Aladjem, M.I., Weinstein, J.N. & Pommier, Y. Molecular interaction maps of bioregulatory networks: a general rubric for systems biology. Mol. Biol. Cell 17 , 1–13 (2006).
Moodie, S.L., Sorokin, A., Goryanin, I. & Ghazal, P. A graphical notation to describe the logical interactions of biological pathways. J. Integr. Bioinform. 3 , 11 (2006).
Novere, N.L. et al. The systems biology graphical notation. Nat. Biotechnol. 27 , 735–741 (2009).
Lopez, C.F., Muhlich, J.L., Bachman, J.A. & Sorger, P.K. Programming biological models in Python using PySB. Mol. Syst. Biol. 9 , 646 (2013).
Beltrame, L. et al. The Biological Connection Markup Language: a SBGN-compliant format for visualization, filtering and analysis of biological pathways. Bioinformatics 27 , 2127–2133 (2011).
Calzone, L., Gelay, A., Zinovyev, A., Radvanyi, F. & Barillot, E. A comprehensive modular map of molecular interactions in RB/E2F pathway. Mol. Syst. Biol. 4 , 173 (2008).
Kuperstein, I. et al. Atlas of Cancer Signalling Network: a systems biology resource for integrative analysis of cancer data with Google Maps. Oncogenesis 4 , e160 (2015).
Oda, K. & Kitano, H. A comprehensive map of the toll-like receptor signaling network. Mol. Syst. Biol. 2 , 2006.0015 (2006).
Oda, K., Matsuoka, Y., Funahashi, A. & Kitano, H. A comprehensive pathway map of epidermal growth factor receptor signaling. Mol. Syst. Biol. 1 , 2005.0010 (2005).
Raza, S. et al. Construction of a large scale integrated map of macrophage pathogen recognition and effector systems. BMC Syst. Biol. 4 , 63 (2010).
Wentker, P. et al. An interactive macrophage signal transduction map facilitates comparative analyses of high-throughput data. J. Immunol. 198 , 2191–2201 (2017).
Matsuoka, Y., Funahashi, A., Ghosh, S. & Kitano, H. Modeling and simulation using CellDesigner. Methods Mol. Biol. 1164 , 121–145 (2014).
Demir, E. et al. The BioPAX community standard for pathway data sharing. Nat. Biotechnol. 28 , 935–942 (2010).
Kanehisa, M., Goto, S., Sato, Y., Furumichi, M. & Tanabe, M. KEGG for integration and interpretation of large-scale molecular data sets. Nucleic Acids Res. 40 , D109–D114 (2012).
Wu, G., Dawson, E., Duong, A., Haw, R. & Stein, L. ReactomeFIViz: a cytoscape app for pathway and network-based data analysis. F1000Res 3 , 146 (2014).
PubMed PubMed Central Google Scholar
Yamada, T., Letunic, I., Okuda, S., Kanehisa, M. & Bork, P. iPath2.0: interactive pathway explorer. Nucleic Acids Res. 39 , W412–W415 (2011).
Czauderna, T., Klukas, C. & Schreiber, F. Editing, validating and translating of SBGN maps. Bioinformatics 26 , 2340–2341 (2010).
Croft, D. et al. The reactome pathway knowledgebase. Nucleic Acids Res. 42 , D472–D477 (2014).
Joshi-Tope, G. et al. Reactome: a knowledgebase of biological pathways. Nucleic Acids Res. 33 , D428–432 (2005).
Kuperstein, I. et al. NaviCell: a web-based environment for navigation, curation and maintenance of large molecular interaction maps. BMC Syst. Biol. 7 , 100 (2013).
Mi, H. & Thomas, P. PANTHER pathway: an ontology-based pathway database coupled with data analysis tools. Methods Mol. Biol. 563 , 123–140 (2009).
Hucka, M. et al. The systems biology markup language (SBML): a medium for representation and exchange of biochemical network models. Bioinformatics 19 , 524–531 (2003).
Bause, F. & Kritzinger, P.S. Stochastic Petri Nets: An Introduction to the Theory (Vieweg & Teubner, 1996).
Reddy, V.N., Mavrovouniotis, M.L. & Liebman, M.N. Petri net representations in metabolic pathways. Proc. Int. Conf. Intell. Syst. Mol. Biol. 1 , 328–336 (1993).
CAS PubMed Google Scholar
Bahi-Jaber, N. & Pontier, D. Modeling transmission of directly transmitted infectious diseases using colored stochastic Petri nets. Math. Biosci. 185 , 1–13 (2003).
Chaouiya, C. Petri net modelling of biological networks. Brief Bioinform. 8 , 210–219 (2007).
Heiner, M., Koch, I. & Will, R. Model validation of biological pathways using Petri nets – demonstrated for apoptosis. Biosystems 75 , 15–28 (2004).
Peleg, M., Rubin, D. & Altman, R.B. Using Petri net tools to study properties and dynamics of biological systems. J. Am. Med. Inform. Assoc. 12 , 181–199 (2005).
Taubner, C., Mathiak, B., Kupfer, A., Fleischer, N. & Eckstein, S. Modelling and simulation of the TLR4 pathway with coloured Petri nets. Conf. Proc. IEEE Eng. Med. Biol. Soc. 1 , 2009–2012 (2006).
Balazki, P., Lindauer, K., Einloft, J., Ackermann, J. & Koch, I. MONALISA for stochastic simulations of Petri net models of biochemical systems. BMC Bioinform. 16 , 215 (2015).
Marwan, W., Rohr, C. & Heiner, M. Petri nets in Snoopy: a unifying framework for the graphical display, computational modelling, and simulation of bacterial regulatory networks. Bact. Mol. Netw.: Methods Protoc. 804 , 409–437 (2012).
Ramos, H. et al. The protein information and property explorer 2: gaggle-like exploration of biological proteomic data within one webpage. Proteomics 11 , 154–158 (2011).
Ruths, D., Muller, M., Tseng, J.T., Nakhleh, L. & Ram, P.T. The signaling petri net-based simulator: a non-parametric strategy for characterizing the dynamics of cell-specific signaling networks. PLoS Comput. Biol. 4 , e1000005 (2008).
Li, C. et al. Structural modeling and analysis of signaling pathways based on Petri nets. J. Bioinform. Comput. Biol. 4 , 1119–1140 (2006).
David, R. & Alla, H. Discrete, Continuous, and Hybrid Petri Nets, 2nd edn. (Springer, 2010).
Theocharidis, A., van Dongen, S., Enright, A.J. & Freeman, T.C. Network visualization and analysis of gene expression data using BioLayout Express(3D). Nat. Protoc. 4 , 1535–1550 (2009).
Polak, M.E., Ung, C.Y., Masapust, J., Freeman, T.C. & Ardern-Jones, M.R. Petri net computational modelling of Langerhans cell interferon regulatory factor network predicts their role in T cell activation. Sci. Rep. 7 , 668 (2017).
Di Ventura, B., Lemerle, C., Michalodimitrakis, K. & Serrano, L. From in vivo to in silico biology and back. Nature 443 , 527–533 (2006).
de Jong, H. Modeling and simulation of genetic regulatory systems: a literature review. J. Comput. Biol. 9 , 67–103 (2002).
Friesen, W.O. & Block, G.D. What is a biological oscillator? Am. J. Physiol. 246 , R847–853 (1984).
Pertsovskaya, I., Abad, E., Domedel-Puig, N., Garcia-Ojalvo, J. & Villoslada, P. Transient oscillatory dynamics of interferon beta signaling in macrophages. BMC Syst. Biol. 7 , 59 (2013).
Download references
Acknowledgements
We are grateful to P. Digard and D. Hume for helpful discussions and advice; we thank A. Theocharidis for all his work on developing Graphia Professional. The Hedgehog signaling pathway model ( Supplementary Data ) was generated by M. Graute, a University of Edinburgh final-year B.Sc. student, as part of a 10-week elective course. We also thank the Biotechnology and Biological Sciences Research Council (BBSRC), which funded the development of BioLayout Express 3D , the academic forerunner of Graphia Professional (BB/F003722/1 and BB/I001107/1). T.C.F. was supported by an Institute Strategic Program Grant on Transcriptomes, Networks and Systems (BBS/E/D/20211552).
Author information
Alessandra Livigni and Laura O'Hara: These authors contributed equally to this work.
Authors and Affiliations
The Roslin Institute and Royal (Dick) School of Veterinary Studies, University of Edinburgh, Edinburgh, UK
Alessandra Livigni, Laura O'Hara, Tim Angus, Derek W Wright & Tom C Freeman
MRC Centre for Reproductive Health, Edinburgh, UK
Laura O'Hara & Lee B Smith
Clinical and Experimental Sciences, Sir Henry Wellcome Laboratories, Faculty of Medicine, University of Southampton, Southampton, UK
Marta E Polak
Institute for Life Sciences, University of Southampton, Southampton, UK
Faculty of Science, University of Newcastle, Callaghan, Australia
Lee B Smith
You can also search for this author in PubMed Google Scholar
Corresponding author
Correspondence to Tom C Freeman .
Ethics declarations
Competing interests.
There is now a commercial and supported version of BioLayout Express 3D called Graphia Professional, produced by Kajeka Ltd., (Edinburgh, UK) that possesses all the functionality described here for pathway modeling. T.C.F. is a founder and director of Kajeka, and T.A. is employed by the company as a software engineer. The other authors declared no competing financial interests.
Supplementary information
Supplementary data.
mEPN palette for loading within yEd software; interferon-β signaling pathway components; primary network motifs drawn in Petri net style for testing the SPN algorithm; interferon-β signaling pathway; example of a more complex model, the Hedgehog signaling pathway; and examples of changing parameters—interferon-β signaling pathway. (ZIP 162 kb)
Video of the interferon-β signaling pathway simulation run within BioLayout
The video shows the process of model loading, running the simulation, inspecting token accumulation on specific components and watching the flow of tokens run through the model as an animation. See the Supplementary Data. (MP4 3735 kb)
Rights and permissions
Reprints and permissions
About this article
Cite this article.
Livigni, A., O'Hara, L., Polak, M. et al. A graphical and computational modeling platform for biological pathways. Nat Protoc 13 , 705–722 (2018). https://doi.org/10.1038/nprot.2017.144
Download citation
Published : 15 March 2018
Issue Date : April 2018
DOI : https://doi.org/10.1038/nprot.2017.144
Share this article
Anyone you share the following link with will be able to read this content:
Sorry, a shareable link is not currently available for this article.
Provided by the Springer Nature SharedIt content-sharing initiative
This article is cited by
Transcriptomic analysis of caecal tissue in inbred chicken lines that exhibit heritable differences in resistance to campylobacter jejuni.
- Kay M. Russell
- Jacqueline Smith
- Mark P. Stevens
BMC Genomics (2021)
PlantSimLab - a modeling and simulation web tool for plant biologists
- E. Dimitrova
- R. Laubenbacher
BMC Bioinformatics (2019)
A genomic analysis and transcriptomic atlas of gene expression in Psoroptes ovis reveals feeding- and stage-specific patterns of allergen expression
- Stewart T. G. Burgess
- Edward J. Marr
- Alasdair J. Nisbet
BMC Genomics (2019)
Modelling steroidogenesis: a framework model to support hypothesis generation and testing across endocrine studies
- Laura O’Hara
- Peter J. O’Shaughnessy
- Lee B. Smith
BMC Research Notes (2018)
By submitting a comment you agree to abide by our Terms and Community Guidelines . If you find something abusive or that does not comply with our terms or guidelines please flag it as inappropriate.
Quick links
- Explore articles by subject
- Guide to authors
- Editorial policies
Sign up for the Nature Briefing newsletter — what matters in science, free to your inbox daily.

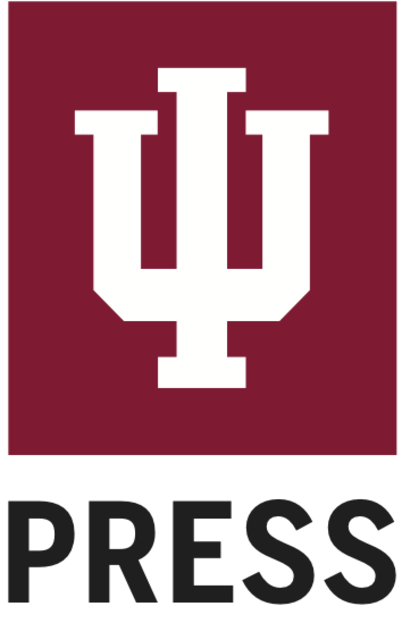
Graphic Representation of Models in Linguistic Theory
Approaching linguistic science from the combined viewpoint of the philosophy of science and the theory of graphic design, Graphic Representation of Models in Linguistic Theory offers a radically new treatment of linguistic theory and the history of linguistics. The author analyzes graphic representation, or diagramming, in terms of form and meaning, pro- poses a taxonomy of linguistic models that cuts across other classifications of linguistic theory, and shows that, like models in the biological and physical sciences, graphic representation has performed and continues to perform a heuristic function for the development of theory in linguistics. This view leads to a reinterpretation not only of the place and function of graphic representation in theoretical linguistics today but also of the history of linguistics.
The book suggests new directions for models in linguistics: by making use of the tools furnished by the principles of graphic design, within the context of the principles of the philosophy of science, linguistics can exploit the influence of graphic representation on theory. This is illustrated by two new three-dimensional models for linguistics designed to replace the two principal models now current—the matrix and the tree—both two-dimensional. An analysis of the ways in which new models grow out of old ones and improve upon them demonstrates how graphic representation can furnish linguistics with models and how the design of such models can be improved by applying the principles of graphic design. The view presented here has particular significance for the development of transformational-generative theory.
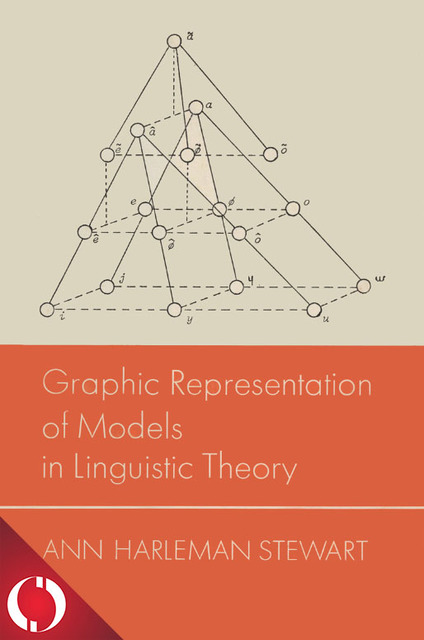
Table of Contents
- isbn 978-0-253-05069-4
- publisher Indiana University Press
- publisher place Bloomington, Indiana USA
- restrictions CC-BY-NC-ND
- rights Copyright © Trustees of Indiana University
- rights holder Indiana University Press
- rights territory World
- doi https://doi.org/10.2979/GraphicRepresentatio
We use cookies to analyze our traffic. Please decide if you are willing to accept cookies from our website. You can change this setting anytime in Privacy Settings .
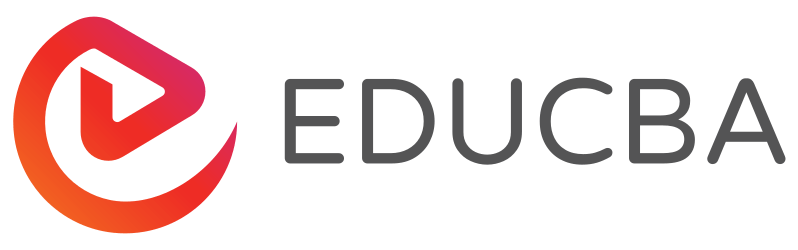
- Linear Regression Analysis
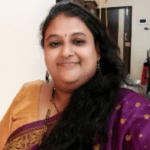
Introduction to Linear Regression Analysis
Linear regression analysis is among the most widely used statistical analysis technique as it involves the study of additive and linear relationships between single and multiple variables techniques. The analysis using a single variable is termed the simple linear analysis, while multiple variables are termed multiple linear analysis. Basically, in linear regression analysis, we try to figure out the relationship of the independent and the dependent variables, and that’s why it has multiple advantages such as being simple and powerful in making better business decisions, etc.
3 Types of Regression Analysis
These three Regression analyses have maximum use cases in the real world; otherwise, there are more than 15 types of regression analysis.
Start Your Free Data Science Course
Hadoop, Data Science, Statistics & others
Given below are 3 types of regression analysis:
- Multiple Linear Regression Analysis
- Logistic Regression
In this article, we will focus on Simple Linear Regression analysis. This analysis helps us to identify the relationship between the independent factor and the dependent factor. In simpler words, the Regression model helps us find how the independent factor changes affect the dependent factor.
This model helps us in multiple ways like:
- It is a simple and powerful statistical model.
- It will help us in making prediction and forecasts.
- It will help us to make a better business decision.
- It will help us to analyze the results and correcting errors.
Equation of Linear Regression and Split it into relevant parts:
- Β1 in the mathematical terminology known as intercept and β2 in the mathematical terminology is known as a slope. They are also known as regression coefficients. ϵ is the error term, and it is the part of Y the regression model is unable to explain.
- Y is a dependent variable (other terms which are interchangeably used for dependent variables are response variable, regressand, measured variable, observed variable, responding variable, explained variable, outcome variable, experimental variable, and/or output variable).
- X is an independent variable (regressors, controlled variable, manipulated a variable, explanatory variable, exposure variable, and/or input variable).
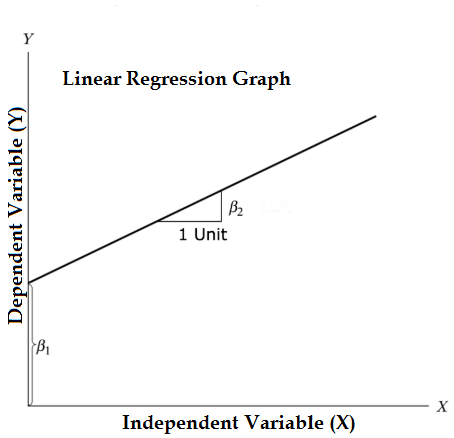
Problem: For understanding what is linear regression analysis, we are taking the “Cars” dataset, which comes by default in R directories. In this dataset, there are 50 observations (basically rows) and 2 variables (columns). Columns names are “Dist” and “Speed”. Here we have to see the impact on distance variables due to change speed variables. To see the structure of the data, we can run a code Str(dataset). This code helps us to understand the structure of the dataset. These functionalities help us make better decisions because we have a better picture of the dataset structure. This code helps us to identify the type of datasets.

Similarly, to check the statistics checkpoints of the dataset, we can use code Summary(cars). This Code provides the mean, median, range of the dataset in a go, which the researcher can use while dealing with the problem.
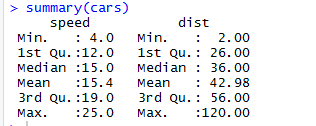
Here we can see the statistical output of every variable we have in our dataset.
Graphical Representation of Datasets
Types of graphical representation which will cover here are and why:
- Scatter Plot: With the help of the graph, we can see in which direction our linear regression model is going, whether there is any strong evidence to prove our model or not.
- Box Plot: Helps us to find outliers.
- Density Plot: Help us understand the independent variable’s distribution; in our case, the independent variable is “Speed”.
Advantages of Graphical Representation
Given below are advantages mentioned:
- Easy to understand.
- It helps us to take quick decision.
- Comparative analysis.
- Less effort and time.
1. Scatter Plot: It will help visualize any relationships between the independent and dependent variables.

We can see from the graph a linearly increasing relationship between the dependent variable (Distance) and the independent variable (Speed).
2. Box Plot: Box plot helps us to identify the outliers in the datasets.
Advantages of using a box plot are:
- Graphical display of variables location and spread.
- It helps us to understand the data’s skewness and symmetry.

3. Density Plot (to check the normality of the distribution)
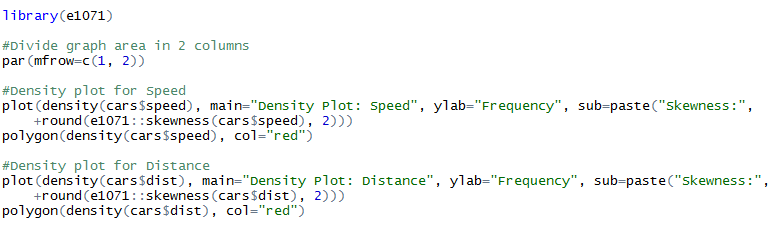

Correlation Analysis
This Analysis helps us to find the relationship between the variables.
There are mainly six types of correlation analysis.
- Positive Correlation (0.01 to 0.99)
- Negative Correlation (-0.99 to -0.01)
- No Correlation
- Perfect Correlation
- Strong Correlation (a value closer to ± 0.99)
- Weak Correlation (a value closer to 0)
A Scatter plot helps us to identify which types of correlation datasets have among them, and the code for finding the correlation is

Here we have a strong positive correlation between Speed and Distance, which means they directly relate to them.
Linear Regression Model
This is the core component of the analysis; earlier, we were just trying and testing things whether the dataset we have is logical enough to run such analysis or not. The function we are planning to use is lm(). This function contains two elements which are Formula and Data. Before assigning that which variable is dependent or independent, we have to be very sure about that because our whole formula depends on that.
The formula looks like this:

As we can recall from the above segment of the article, the equation of linear regression is:
Now we will fit in the information which we got from the above code in this equation.
Only finding the equation of linear regression is not sufficient; we have to check its statistic significance also. For this, we have to pass a code “Summary” on our linear regression model.

There are multiple ways of checking the statistic significance of a model, and here we are using the P-value method. We can consider a model statistically fit when the P-value is less than the pre-determined statistical significant level, which is ideally 0.05. In our table of summary(linear_regression), we can see that P-value is below the 0.05 level, so we can conclude that our model is statistically significant. Once we are sure about our model, we can use our dataset to predict things.
Recommended Articles
This is a guide to Linear Regression Analysis. Here we discuss the three types of linear regression analysis, the graphical representation of datasets with advantages and linear regression models. You can also go through our other related articles to learn more-
- Linear Regression in R
- What is Regression Analysis?
- Guide to Simple Linear Regression in R
- What is Linear Regression?

*Please provide your correct email id. Login details for this Free course will be emailed to you
By signing up, you agree to our Terms of Use and Privacy Policy .
Forgot Password?
This website or its third-party tools use cookies, which are necessary to its functioning and required to achieve the purposes illustrated in the cookie policy. By closing this banner, scrolling this page, clicking a link or continuing to browse otherwise, you agree to our Privacy Policy
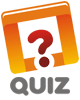
Explore 1000+ varieties of Mock tests View more
Submit Next Question
Early-Bird Offer: ENROLL NOW

Published: 27 June, 2024 Contributors: Ivan Belcic, Cole Stryker
Process modeling is the practice of creating data-driven visual representations of key business processes. It gives organizations a common language with which they can understand and optimize workflows
If an organization wants to earn strong returns on its research and development investments, resolve IT issues with minimal downtime or create an accurate lead qualification workflow, it needs to understand these processes on an objective and comprehensive level. Even the business users directly involved in these processes might lack total transparency into exactly what happens every step of the way.
Business analysts and other stakeholders can gain end-to-end views of the current state of their business process lifecycle through process modeling. It is a business process management (BPM) technique that creates data-driven visualizations of workflows. These process models help organizations document workflows, surface key metrics, pinpoint potential problems and intelligently automate processes.
Download the study to explore Forrester’s findings and discover why continuous process optimization should be a business imperative for your organization.
Automate to elevate
A process model is a graphical representation of a business process or workflow and its related subprocesses. Process modeling generates comprehensive, quantitative activity diagrams and flowcharts containing critical insights into the functioning of a particular process, including:
Events and activities that occur within a workflow.
Who owns or initiates those events and activities.
Decision points and the different paths workflows can take based on their outcomes.
Devices involved in the process.
Timelines of the overall process and each step in the process.
Success and failure rates of the process.
Algorithm-driven: Process models are produced by data mining algorithms that use the data contained within event logs to construct models of the workflows as they exist.
Objective: Because process models are based on quantitative data, they offer genuinely objective views of workflows as they exist in practice and include key data, metrics or events for more thorough process analysis.
By creating a flow diagram of its new account creation process, a software company might discover that a significant number of customers are abandoning the sign-up process because it takes too long. A model can even help the company pinpoint the exact stage at which these drop-offs occur.
Standardized: Process models typically use one of two standardized styles of graphical business process notation: business process modeling notation (BPMN) —also called business process model and notation—or unified modeling language (UML).
Within these notation systems, certain visual elements have universally recognized meanings when used in a process model. Whether an organization uses UML diagrams or BPMN diagrams, these standardized notation methodologies allow process models to be readily shared and read by anyone. Here's how different elements are represented within these diagrams:
Arrows represent sequence flows.
Diamonds represent decision points or gateways.
Ovals represent the beginnings and endpoints of processes.
Rectangles represent specific activities within a workflow.
Swimlanes identify who owns which components of a process.
Event logs and process mining are essential business process modeling tools that underpin modern business process modeling techniques.
Most enterprise IT systems maintain event logs . These event logs are digital records that automatically track state changes and activities, known as events , within the system. Anything that happens within a system can be an event. Here are some common event examples:
A user logs in.
A user updates a record.
A user submits a form.
Information is transferred between systems.
Event logs track both the occurrence of events and information surrounding these events, such as the device performing an activity and how long the activity takes. Event logs act as the inputs during the production of process models.
Process mining is the application of a data-mining algorithm to all of this event log data. The algorithm identifies trends in the data and uses the results of its analysis to generate a visual representation of the process flow within the system.
This visual representation is the process model. Depending on the process targeted for modeling, process-mining algorithms can be applied to a single system, multiple systems or entire technological ecosystems and departments.
Business process modeling shouldn’t be confused with process mapping and process mining . Process maps are manually created based on employee reports and provide higher-level workflow process diagrams. Process mining analyzes organizational data to produce process models, which use that data to create present more objective workflow diagrams .
Process models offer transparency into company workflows, making them a key business process management tool. Process models can be used in any scenario that requires analyzing business processes. These are some of the most common use cases:
A single process model can contain a wealth of workflow data, allowing team members to analyze a workflow from multiple perspectives. Business analysts often use process modeling to highlight these workflow components:
- Control flow: The order in which steps and commands occur within a process is known as its control flow. A process model depicts a flowchart of a process so that a team can see what steps are taken and when. This perspective also helps the team identify any dependencies between steps.
- Organization: A process model can capture who is involved in a process—including people, teams, systems and devices—and how they interact with each other. This perspective illuminates the connections between people and systems that form the organizational social network. In this way, a process model offers insight into how various components of a business function together.
- Time: A process model can record how long a process takes, overall and how long each step takes, allowing the team to identify delays, slowdowns and bottlenecks within the workflow.
- Case: A process model can offer a general view of how a workflow plays out, or it can reflect a particular case—or instance—of a workflow. Teams often use this case perspective to analyze anomalous process outcomes. For example, if a specific instance of a workflow results in lower-than-average outcome quality, teams can isolate exactly what went wrong.
Process models accurately reflect existing workflow inefficiencies and redundancies, simplifying the identification of opportunities for process optimization. When workflows have been optimized, businesses can use process modeling to standardize workflows across the entire enterprise.
The model acts as a template for how processes should play out, helping to ensure that every team and employee approaches the same process in the same way. This leads to more predictable workflows and outcomes overall.
Process models can take the guesswork out of implementing and evaluating new business processes. By creating a model of a new process, business users can get a real-time look at how that workflow is performing, allowing them to make adjustments as necessary to achieve process optimization.
Process models can help companies track whether money and resource investments produce suitable returns. For example, by creating a model of the standard sales process, an organization can see how sales representatives are using the tools and systems at their disposal.
It might turn out that a certain tool is used much less frequently in the process steps than anticipated, in which case, the organization can choose to disinvest from the tool and spend that money on a solution the sales team uses throughout the entire process.
Process models transform complex processes into concrete images, simplifying the dissemination and discussion of processes throughout the organization, especially useful for standardizing project management. For example, if one department has an efficient process for troubleshooting technical problems, the business can create a model of this process to guide implementation on an organization-wide scale.
Process modeling arms an enterprise with objective business intelligence that supports more informed decisions for resource allocation, process improvement and overall business strategy. With a clear view of processes, enterprise teams can ensure that workflows consistently yield optimal results. As a result, operating costs are lower, revenue is higher and business outcomes are stronger.
With process modeling, companies can:
Without a process model, teams are limited to discussing and analyzing workflows in qualitative and subjective terms.
As a result, teams might not accurately understand their workflows. They might make business decisions based on misunderstandings, assumptions or incomplete knowledge.
Process modeling grants access to quantitative workflow data, including success and error rates, allowing for a more rigorous analysis of business processes.
Before a process can be automated, an organization needs a clear understanding of how that process plays out in reality, including the business logic underpinning each decision point.
A process model illuminates both the way that a workflow unfolds and the relationships between events, actors, tools and systems within and between processes.
This viewpoint helps a team document the process itself and the business rules that guide its execution. This information simplifies the process of effectively automating workflows the first time, and then iterating for continuous improvement.
Process models provide organizations with a simpler way to identify opportunities for process optimization. As a result, business processes require less investment to maintain and generate positive outcomes at a lower cost.
Discover solutions that deliver intelligent automation quickly with low-code tools.
IBM Process Mining helps businesses make faster, more informed decisions for process improvement through data-driven insights.
Boost ROI with full-featured AI-driven Robotic Process Automation.
Learn how restaurant chain Primanti Brothers is modernizing back-office processes with a Robotic Process Automation bot.
Discover how organizations are employing predictive approaches, process mining tools and implementing tech-infused workflows to achieve data-driven innovation.
Simplify the identification of opportunities for process optimization by providing organizations with process models.
Discover how high-impact automation can help make your IT systems more proactive, business processes more efficient and people more productive.
- Contact Your Regional Manager
- News and Blogs
- Staff Portal

What are logic models, and when should you use them?
Written by Leressa Suber, PHR, SHRM-CP
Apr 3, 2019 | Blog , Grants & Evaluation

There are several key components of logic models that are standard best practice. This blog will discuss these various components and provide an example of a logic model template. You may access the free logic model template here .
When developing a new program, or trying to figure out what aspects of an existing program need to be evaluated, a logic model may be helpful in showing the various components, activities and goals of the program.
A logic model is a graphical representation of your program, from the resources (inputs) and activities that will take place, to the deliverables (outputs) and goals( outcomes) that the program will produce.
Three reasons to consider developing a logic model:
- A funder (e.g. grantor) requires a logic model as part of an evaluation plan in your proposal.
- Program stakeholders are requesting details on your measurable goals and objectives, and you are looking for ways to visually display this information.
- You want to see a quick snapshot of how your program operates and what it intends to accomplish.
To further clarify the significance and components of a logic model, let’s walk through an example which will break out each component separately and finish with a complete logic model.
Example: Your internal evaluation team has been tasked with identifying issues with student persistence at a local community college. What are some examples of inputs, activities and goals which will be shown in the logic model?
Inputs | Activities | Outputs | Outcomes |
Project Team, Student Support Staff, Advisory Board |
Inputs | Activities (High Level) | Outputs | Outcomes |
Project Team, Student Support Staff, Advisory Board | intensive/intrusive advising |
Inputs | Activities (High Level) | Outputs | Outcomes |
Project Team, Student Support Staff, Advisory Board | intensive/intrusive advising | # of intensive/intrusive advising sessions |
Inputs | Activity | Outputs | Short-Term | Mid-Term | Long Term Outcomes |
Students, Student Support Staff, | intensive / intrusive advising | # of intensive/intrusive advising sessions | # of completers | Increase in student persistence | Increase in graduation |
As you work through your program implementation, you may determine that changes need to be made along the way. If this occurs, update the components of the logic model accordingly. Overall, the logic model will serve as a guide for your evaluation plan design. Stay tuned for more information on Evaluation Plan Design in our next blog. If we can help with your logic model development, consider dropping us a line .

Leressa Suber is the Evaluation Coordinator with NC State University Industry Expansion Solutions and the IES Evaluation Solutions Group . Leressa works with program and grant evaluations related to workforce development, community college, STEM, and the Department of Defense (DOD). Leressa has a background in human resources and adult education. She has an MS Degree in Occupational and Technical Studies from Old Dominion University and a BS in Business Education from North Carolina A&T State University.
919.515.2358
Contact Form
Quick Links
Questions? Email or call 919.515.8584 .
Questions? Email or call 919.515.5358 .
On-Site Availability
Our open enrollment classes can be customized for your organization and provided on location at your facility. Please Contact Your Industry Expansion Solutions Regional Manager for more information.
We Can Help
Regional Managers are located across the state to help you with customized onsite training programs!
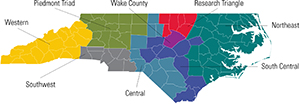
Piedmont Triad Email: Kami Baggett 919.830.7292
Northeast Email: Lori Benn 919.988.7475
Wake County Email: Robert Crew 919.830.2941
Southwest Email: Jennifer Fielder 704.380.0063
South Central Email: Anna Mangum 919.210.6050
Western Email: Chris McGraw 828.329.3119
Military - Statewide Email: Michael Mullins 919.515.8812
Research Triangle Email: Mitch Poteat 919.607.0684
Central Email: Mary Tillery 910.622.5849

Navigate Blog
- Resource Library
Recent Posts
- Steps for Communicating Evaluation Design to PIs
- The Secret Weakness the CrowdStrike Disaster Revealed
- Connecting the Dots: Summer 2024 Newsletter
IES Solutions Overview
Want to quickly browse all our solutions? Click here for the complete IES Solutions Overview brochure.
Reserving-Masking-Reconstruction Model for Self-Supervised Heterogeneous Graph Representation
New citation alert added.
This alert has been successfully added and will be sent to:
You will be notified whenever a record that you have chosen has been cited.
To manage your alert preferences, click on the button below.
New Citation Alert!
Please log in to your account
Information & Contributors
Bibliometrics & citations, view options, supplemental material, index terms.
Computing methodologies
Machine learning
Network algorithms
Recommendations
Unsupervised heterogeneous graph rewriting attack via node clustering.
Self-supervised learning (SSL) has become one of the most popular learning paradigms and has achieved remarkable success in the graph field. Recently, a series of pre-training studies on heterogeneous graphs (HGs) using SSL have been proposed considering ...
Node and edge dual-masked self-supervised graph representation
Self-supervised graph representation learning has been widely used in many intelligent applications since labeled information can hardly be found in these data environments. Currently, masking and reconstruction-based (MR-based) methods lead the ...
Self-supervised contrastive graph representation with node and graph augmentation
Graph representation is a critical technology in the field of knowledge engineering and knowledge-based applications since most knowledge bases are represented in the graph structure. Nowadays, contrastive learning has become a prominent way for ...
Information
Published in.

- General Chairs:

Northeastern University, USA

CENTAI / Eurecat, Italy
- SIGMOD: ACM Special Interest Group on Management of Data
- SIGKDD: ACM Special Interest Group on Knowledge Discovery in Data
Association for Computing Machinery
New York, NY, United States
Publication History
Check for updates, author tags.
- graph representation learning
- heterogeneous graph neural network
- self-supervised learning
- Research-article
Funding Sources
- National Natural Foundation of China
- The Key Scientific and Technological Project of Yunnan Province
Acceptance Rates
Contributors, other metrics, bibliometrics, article metrics.
- 0 Total Citations
- 50 Total Downloads
- Downloads (Last 12 months) 50
- Downloads (Last 6 weeks) 58
View options
View or Download as a PDF file.
View online with eReader .
Login options
Check if you have access through your login credentials or your institution to get full access on this article.
Full Access
Share this publication link.
Copying failed.
Share on social media
Affiliations, export citations.
- Please download or close your previous search result export first before starting a new bulk export. Preview is not available. By clicking download, a status dialog will open to start the export process. The process may take a few minutes but once it finishes a file will be downloadable from your browser. You may continue to browse the DL while the export process is in progress. Download
- Download citation
- Copy citation
We are preparing your search results for download ...
We will inform you here when the file is ready.
Your file of search results citations is now ready.
Your search export query has expired. Please try again.
Temporal knowledge graph reasoning based on evolutional representation and contrastive learning
- Published: 31 August 2024
Cite this article
- Qiuying Ma 1 ,
- Xuan Zhang ORCID: orcid.org/0000-0003-2929-2126 1 , 2 ,
- ZiShuo Ding 7 ,
- Chen Gao 4 ,
- Weiyi Shang 3 ,
- Qiong Nong 1 ,
- Yubin Ma 1 &
- Zhi Jin 5 , 6
Temporal knowledge graphs (TKGs) are a form of knowledge representation constructed based on the evolution of events at different time points. It provides an additional perspective by extending the temporal dimension for a range of downstream tasks. Given the evolving nature of events, it is essential for TKGs to reason about non-existent or future events. Most of the existing models divide the graph into multiple time snapshots and predict future events by modeling information within and between snapshots. However, since the knowledge graph inherently suffers from missing data and uneven data distribution, this time-based division leads to a drastic reduction in available data within each snapshot, which makes it difficult to learn high-quality representations of entities and relationships. In addition, the contribution of historical information changes over time, distinguishing its importance to the final results when capturing information that evolves over time. In this paper, we introduce CH-TKG (Contrastive Learning and Historical Information Learning for TKG Reasoning) to addresses issues related to data sparseness and the ambiguity of historical information weights. Firstly, we obtain embedding representations of entities and relationships with evolutionary dependencies by R-GCN and GRU. On this foundation, we introduce a novel contrastive learning method to optimize the representation of entities and relationships within individual snapshots of sparse data. Then we utilize self-attention and copy mechanisms to learn the effects of different historical data on the final inference results. We conduct extensive experiments on four datasets, and the experimental results demonstrate the effectiveness of our proposed model with sparse data.
This is a preview of subscription content, log in via an institution to check access.
Access this article
Subscribe and save.
- Get 10 units per month
- Download Article/Chapter or eBook
- 1 Unit = 1 Article or 1 Chapter
- Cancel anytime
Price includes VAT (Russian Federation)
Instant access to the full article PDF.
Rent this article via DeepDyve
Institutional subscriptions
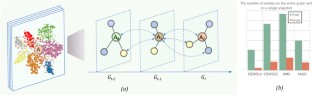
Similar content being viewed by others
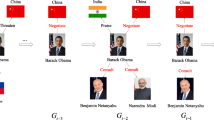
GLANet: temporal knowledge graph completion based on global and local information-aware network
tHR-Net: A Hybrid Reasoning Framework for Temporal Knowledge Graph
LogE-Net: Logic Evolution Network for Temporal Knowledge Graph Forecasting
Explore related subjects.
- Artificial Intelligence
Data Availability
The data that support the findings of this study are openly available at https://github.com/mqygit/TKGs
Chen X, Jia S, Xiang Y (2020) A review: Knowledge reasoning over knowledge graph. Expert Syst Appl 141:112948
Article Google Scholar
Chen IY, Agrawal M, Horng S, Sontag D (2019) Robustly extracting medical knowledge from ehrs: a case study of learning a health knowledge graph. In: Pacific symposium on biocomputing 2020, pp 19–30. World Scientific
Jiang Z, Chi C, Zhan Y (2021) Research on medical question answering system based on knowledge graph. IEEE Access 9:21094–21101
Ahmed IA, AL-Aswadi FN, Noaman KM et al (2022) Arabic knowledge graph construction: A close look in the present and into the future. J King Saud University-Comput Inf Sci 34(9):6505–6523
Bordes A, Usunier N, Garcia-Duran A, Weston J, Yakhnenko O (2013) Translating embeddings for modeling multi-relational data. Advances in Neural Information Processing Systems 26
Jin W, Qu M, Jin X, Ren X (2020) Recurrent event network: Autoregressive structure inference over temporal knowledge graphs. In: Proceedings of the 2020 conference on empirical methods in natural language processing (EMNLP)
Park N, Liu F, Mehta P, Cristofor D, Faloutsos C, Dong Y (2022) Evokg: Jointly modeling event time and network structure for reasoning over temporal knowledge graphs. In: Proceedings of the fifteenth ACM international conference on web search and data mining, pp 794–803
Li Z, Jin X, Li W, Guan S, Guo J, Shen H, Wang Y, Cheng X (2021) Temporal knowledge graph reasoning based on evolutional representation learning. In: Proceedings of the 44th international ACM SIGIR conference on research and development in information retrieval, pp 408–417
Lin Z, Tian C, Hou Y, Zhao WX (2022) Improving graph collaborative filtering with neighborhood-enriched contrastive learning. Proceedings of the ACM web conference 2022:2320–2329
Google Scholar
Wang Z, Zhang J, Feng J, Chen Z (2014) Knowledge graph embedding by translating on hyperplanes. In: Proceedings of the AAAI conference on artificial intelligence, vol 28
Lin Y, Liu Z, Sun M, Liu Y, Zhu X (2015) Learning entity and relation embeddings for knowledge graph completion. In: Proceedings of the AAAI conference on artificial intelligence, vol 29
Nickel M, Tresp V, Kriegel H-P et al (2011) A three-way model for collective learning on multi-relational data. Icml 11:3104482–3104584
Bishan Yang and Wen-tau Yih and Xiaodong He and Jianfeng Gao and Li Deng (2014) embedding entities and relations for learning and inference in knowledge bases. In: International conference on learning representations
Trouillon T, Welbl J, Riedel S, Gaussier É, Bouchard G (2016) Complex embeddings for simple link prediction. In: International conference on machine learning, pp 2071–2080. PMLR
Bordes A, Glorot X, Weston J, Bengio Y (2014) A semantic matching energy function for learning with multi-relational data: Application to word-sense disambiguation. Mach Learn 94:233–259
Article MathSciNet Google Scholar
Zhang S, Liu Y, Sun Y, Shah N (2021) Graph-less neural networks: Teaching old mlps new tricks via distillation. In: International conference on learning representations
Socher R, Chen D, Manning CD, Ng A (2013) Reasoning with neural tensor networks for knowledge base completion. Adv Neural Inf Process Syst 26
Schlichtkrull M, Kipf TN, Bloem P, Van Den Berg R, Titov I, Welling M (2018) Modeling relational data with graph convolutional networks. In: The Semantic Web: 15th international conference, ESWC 2018, Heraklion, Crete, Greece, June 3–7, 2018, Proceedings 15, pp 593–607. Springer
Vashishth S, Sanyal S, Nitin V, Talukdar P (2019) Composition-based multi-relational graph convolutional networks. In: International conference on learning representations
Zhang M, Xia Y, Liu Q, Wu S, Wang L (2023) Learning long-and short-term representations for temporal knowledge graph reasoning. In: Proceedings of the ACM web conference vol 2023, pp 2412–2422
Garcia-Duran A, Dumančić S, Niepert M (2018) Learning sequence encoders for temporal knowledge graph completion. In: Proceedings of the 2018 conference on empirical methods in natural language processing, pp 4816–4821
Leblay J, Chekol MW (2018) Deriving validity time in knowledge graph. In: Companion proceedings of the the web conference vol 2018, pp 1771–1776
Kazemi SM, Goel R, Jain K, Kobyzev I, Sethi A, Forsyth P, Poupart P (2020) Representation learning for dynamic graphs: A survey. J Mach Learn Res 21(1):2648–2720
MathSciNet Google Scholar
Zhu C, Chen M, Fan C, Cheng G, Zhang Y (2021) Learning from history: Modeling temporal knowledge graphs with sequential copy-generation networks. In: Proceedings of the AAAI conference on artificial intelligence vol 35, pp 4732–4740
Li Z, Jin X, Guan S, Li W, Guo J, Wang Y, Cheng X (2021) Search from history and reason for future: Two-stage reasoning on temporal knowledge graphs. In: Proceedings of the 59th annual meeting of the association for computational linguistics and the 11th international joint conference on natural language processing (vol 1: Long Papers), pp 4732–4743
Wang J, Lin X, Huang H, Ke X, Wu R, You C, Guo K (2023) Glanet: temporal knowledge graph completion based on global and local information-aware network. Appl Intell, pp 1–17
Trivedi R, Dai H, Wang Y, Song L (2017) Know-evolve: deep temporal reasoning for dynamic knowledge graphs. In: International conference on machine learning, pp 3462–3471. PMLR
Sun H, Geng S, Zhong J, Hu H, He K (2022) Graph hawkes transformer for extrapolated reasoning on temporal knowledge graphs. In: Proceedings of the 2022 conference on empirical methods in natural language processing, pp 7481–7493
Bai L, Chai D, Zhu L (2023) Rlat: Multi-hop temporal knowledge graph reasoning based on reinforcement learning and attention mechanism. Knowl-Based Syst 269:110514
Zhang H, Bai L (2023) Few-shot link prediction for temporal knowledge graphs based on time-aware translation and attention mechanism. Neural Netw 161:371–381
Zhang D, Feng W, Wu Z, Li G, Ning B (2024) Cdrgn-sde: Cross-dimensional recurrent graph network with neural stochastic differential equation for temporal knowledge graph embedding. Expert Syst Appl 247:123295
Han Z, Ding Z, Ma Y, Gu Y, Tresp V (2021) Learning neural ordinary equations for forecasting future links on temporal knowledge graphs. In: Proceedings of the 2021 conference on empirical methods in natural language processing
You Y, Chen T, Sui Y, Chen T, Wang Z, Shen Y (2020) Graph contrastive learning with augmentations. Adv Neural Info Process Syst 33:5812–5823
Chen X, Fan H, Girshick R, He K (2020) Improved baselines with momentum contrastive learning. arXiv:2003.04297
Gao T, Yao X, Chen D (2021) Simcse: Simple contrastive learning of sentence embeddings. In: Proceedings of the 2021 conference on empirical methods in natural language processing
You Y, Chen T, Wang Z, Shen Y (2022) Bringing your own view: Graph contrastive learning without prefabricated data augmentations. In: Proceedings of the fifteenth ACM international conference on web search and data mining, pp 1300–1309
Chen Y, Liu Z, Li J, McAuley J, Xiong C (2022) Intent contrastive learning for sequential recommendation. In: Proceedings of the ACM web conference vol 2022, pp 2172–2182
Zhu Y, Xu Y, Yu F, Liu Q, Wu S, Wang L (2021) Graph contrastive learning with adaptive augmentation. In: Proceedings of the web conference vol 2021, pp 2069–2080
Sun F-Y, Hoffman J, Verma V, Tang J (2019) Infograph: Unsupervised and semi-supervised graph-level representation learning via mutual information maximization. In: International conference on learning representations
Xu M, Wang H, Ni B, Guo H, Tang J (2021) Self-supervised graph-level representation learning with local and global structure. In: International conference on machine learning, pp 11548–11558. PMLR
Wang L, Zhao W, Wei Z, Liu J (2022) Simkgc: Simple contrastive knowledge graph completion with pre-trained language models. In: Proceedings of the 60th annual meeting of the association for computational linguistics (vol 1: Long Papers). https://aclanthology.org/2022.acl-long.295
Zhang D, Rong Z, Xue C, Li G (2024) Simre: Simple contrastive learning with soft logical rule for knowledge graph embedding
Xu Y, Ou J, Xu H, Fu L (2023) Temporal knowledge graph reasoning with historical contrastive learning. In: Proceedings of the AAAI conference on artificial intelligence vol 37, pp 4765–4773
Mahdisoltani F, Biega J, Suchanek FM (2013) Yago3: A knowledge base from multilingual wikipedias. In: CIDR
Dettmers T, Minervini P, Stenetorp P, Riedel S (2018) Convolutional 2d knowledge graph embeddings. In: Proceedings of the AAAI conference on artificial intelligence, vol 32
Shang C, Tang Y, Huang J, Bi J, He X, Zhou B (2019) End-to-end structure-aware convolutional networks for knowledge base completion. In: Proceedings of the AAAI conference on artificial intelligence vol 33, pp 3060–3067
Sun Z, Deng Z-H, Nie J-Y, Tang J (2019) Rotate: Knowledge graph embedding by relational rotation in complex space. In: International conference on learning representations. https://openreview.net/forum?id=HkgEQnRqYQ
Dasgupta SS, Ray SN, Talukdar P (2018) Hyte: Hyperplane-based temporally aware knowledge graph embedding. In: Proceedings of the 2018 conference on empirical methods in natural language processing, pp 2001–2011
Li Z, Guan S, Jin X, Peng W, Lyu Y, Zhu Y, Bai L, Li W, Guo J, Cheng X (2022) Complex evolutional pattern learning for temporal knowledge graph reasoning. In: Proceedings of the 60th annual meeting of the association for computational linguistics (vol 2: Short Papers), pp 290–296
Download references
Acknowledgements
National Natural Science Foundation of China under Grant Nos. 62192731; Science Foundation of Young and Middle-aged Academic and Technical Leaders of Yunnan under Grant No. 202205AC160040; Science Foundation of Yunnan Jinzhi Expert Workstation under Grant No. 202205AF150006; Major Project of Yunnan Natural Science Foundation under Grant No. 202302AE09002003; Knowledge-driven Smart Energy Science and Technology Innovation Team of Yunnan Provincial Department of Education; Open Foundation of Yunnan Key Laboratory of Software Engineering under Grant No. 2023SE101.
Author information
Authors and affiliations.
School of Software, Yunnan University, Yunnan, 650091, China
Qiuying Ma, Xuan Zhang, Qiong Nong & Yubin Ma
Yunnan Key Laboratory of Software Engineering, Yunnan, 650091, China
Department of Electrical and Computer Engineering, University of Waterloo, Ontario, N2L 3G1, Canada
Weiyi Shang
School of Information Science and Engineering, Yunnan University, Yunnan, 650091, China
School of Computer Science, Peking University, Beijing, 10087, China
Key Lab. of High-Confidence Software Technologies (PKU), Ministry of Education, Beijing, 10087, China
Data Science and Anaytics Thrust, Hong Kong University of Science and Technology (Guangzhou), Guangzhou, Guangdong, 511442, China
ZiShuo Ding
You can also search for this author in PubMed Google Scholar
Contributions
\(\bullet \) Conceptualization: [Qiuying Ma]; \(\bullet \) Methodology: [Qiuying Ma]; \(\bullet \) Formal analysis and investigation: [Qiuying Ma], [Xuan Zhang]; \(\bullet \) Writing - original draft preparation: [Qiuying Ma]; \(\bullet \) Writing - review and editing: [Xuan Zhang],[Chen Gao],[ZiShuo Ding],[Qiong Nong],[Yubin Ma]; \(\bullet \) Funding acquisition: [Xuan Zhang]; \(\bullet \) Resources: [Xuan Zhang],[Weiyi Shang],[Zhi Jin]; \(\bullet \) Supervision: [Xuan Zhang]
Corresponding author
Correspondence to Xuan Zhang .
Ethics declarations
Competing of interest.
The authors declare that they have no known competing financial interests or personal relationships that could have appeared to influence the work reported in this paper.
Additional information
Publisher's note.
Springer Nature remains neutral with regard to jurisdictional claims in published maps and institutional affiliations.
Rights and permissions
Springer Nature or its licensor (e.g. a society or other partner) holds exclusive rights to this article under a publishing agreement with the author(s) or other rightsholder(s); author self-archiving of the accepted manuscript version of this article is solely governed by the terms of such publishing agreement and applicable law.
Reprints and permissions
About this article
Ma, Q., Zhang, X., Ding, Z. et al. Temporal knowledge graph reasoning based on evolutional representation and contrastive learning. Appl Intell (2024). https://doi.org/10.1007/s10489-024-05767-6
Download citation
Accepted : 11 August 2024
Published : 31 August 2024
DOI : https://doi.org/10.1007/s10489-024-05767-6
Share this article
Anyone you share the following link with will be able to read this content:
Sorry, a shareable link is not currently available for this article.
Provided by the Springer Nature SharedIt content-sharing initiative
- Temporal knowledge graph reasoning
- Contrastive learning
- Graph convolutional network
- Knowledge weight learning
- Find a journal
- Publish with us
- Track your research
Downlink CCM Estimation via Representation Learning with Graph Regularization
In this paper, we propose an algorithm for downlink (DL) channel covariance matrix (CCM) estimation for frequency division duplexing (FDD) massive multiple-input multiple-output (MIMO) communication systems with base station (BS) possessing a uniform linear array (ULA) antenna structure. We consider a setting where the UL CCM is mapped to DL CCM by a mapping function. We first present a theoretical error analysis of learning a nonlinear embedding by constructing a mapping function, which points to the importance of the Lipschitz regularity of the mapping function for achieving high estimation performance. Then, based on the theoretical ground, we propose a representation learning algorithm as a solution for the estimation problem, where Gaussian RBF kernel interpolators are chosen to map UL CCMs to their DL counterparts. The proposed algorithm is based on the optimization of an objective function that fits a regression model between the DL CCM and UL CCM samples in the training dataset and preserves the local geometric structure of the data in the UL CCM space, while explicitly regulating the Lipschitz continuity of the mapping function in light of our theoretical findings. The proposed algorithm surpasses benchmark methods in terms of three error metrics as shown by simulations.
Index Terms:
I introduction.
Massive MIMO is a favorable technology for 5G and beyond networks in terms of achieving high spectral efficiency and energy efficiency [ 1 ] . In this technology, the base station (BS) has a high number of antennas, which is much more than the number of active user terminals [ 2 ] . However, there is a drawback of this technology for FDD systems: the excessive impractical pilot and feedback overhead [ 3 , 4 ] . Since the uplink and downlink channels are not reciprocal in FDD systems, the channel estimation process consumes too much resource for pilot and feedback symbols due to the high number of antennas at the base station [ 3 ] .
Cellular systems operate in time division duplexing (TDD) mode or frequency division duplexing (FDD) mode. Sharing the same wireless medium and frequency band, uplink and downlink channels are said to be reciprocal in TDD systems [ 5 ] , which means that learning the uplink channel state information (CSI), the base station can infer the downlink CSI as well, and does not require any additional pilot training for downlink channel estimation. However, the reciprocity of uplink and downlink channels does not hold for FDD systems, because even though they share the same wireless medium, they operate using different carrier frequencies [ 6 ] . Thus, one cannot learn the DL CSI from its uplink counterpart in FDD systems, which is a huge disadvantage for the implementation of massive MIMO in these systems. As the number of base station antennas increases, the dimension of the downlink channel to be estimated also increases. Therefore, one needs to send more pilot signals to the users and receive feedback from them, in order to learn the DL CSI.
One solution to loosen the pilot and feedback overhead is to use the DL CCM instead of the DL CSI [ 4 ] . The channel covariance matrix provides second order channel statistics, which may be useful for channel estimation and beamforming [ 7 ] . Therefore, it is an important parameter to know for the implementation of massive MIMO in FDD systems and knowing it as accurately as possible is highly important. In literature, there are numerous studies where the DL CCM is estimated by using the UL CCM, as in [ 8 , 9 , 10 , 11 , 12 , 13 , 14 ] . The motivation behind this choice is the following: Even though there is no channel reciprocity between the uplink and downlink channels, there is spatial reciprocity between them [ 15 ] . Therefore, their power distribution in the angular domain, i.e., their power angular spectrum (PAS), is commonly taken as the same. Thus, one can say that the UL CCM is quite informative about the DL CCM. Many of the fundamental approaches for the solution of this problem benefits from this property.
Some of the earlier works propose simple signal processing methods for the DL CCM estimation problem, such as [ 8 , 9 , 14 ] . On the other hand, much more complex tools like deep learning can also be incorporated into the solution of the UL-to-DL CCM transformation problem, as in [ 13 ] . Signal processing-based methods may experience performance degradation in practical cases where the UL CCM is not perfectly known. Their performance heavily depends on the availability of an accurate UL CCM and error propagation may occur in the cases where these methods are used to predict the DL CCM from noisy UL CCM estimates. Deep learning solutions are more robust to noise compared to classical methods due to the process of learning from the data. Nonetheless, in order to learn an accurate deep learning model with good generalization ability, one needs to use excessive amounts of data. Especially, as the number of base station antennas increases, the size of the matrix to be learned will increase, which increases the need for more training data.
In this paper, for the DL CCM estimation problem, we propose to learn a nonlinear interpolation function which maps an arbitrary user’s UL CCM to its DL CCM. In view of the above discussions, our idea is to seek a trade-off between simple signal processing-based methods and the complex deep learning solutions. We thus propose to learn a nonlinear interpolator that possesses the rich representation power of nonlinear methods with successful generalization capabilities, while involving fewer parameters to be optimized compared to neural networks in order to require much less training data. To the best of our knowledge, the estimation of DL CCMs from their UL counterparts via nonlinear interpolators has not yet been studied thoroughly in the current literature, due to which we aim to address both the theoretical and methodological aspects of this problem.
We first present a detailed theoretical analysis, where we study the performance of mapping the UL CCM to the DL CCM via a mapping function. Our analysis shows us that under certain assumptions, the norm of the difference between two points in the DL CCM space is upper-bounded by a scale of the norm of the difference between their corresponding points in the UL CCM space. This theoretical result motivates us to enforce a constraint that preserves the local neighborhood relationships of the UL CCM space in the mapping to be learnt. Our theoretical analysis also indicates that the smaller the Lipschitz constant of the mapping function is, the smaller the upper bound for the error of an arbitrary test point is. Therefore, we also impose a constraint of keeping the Lipschitz constant of the mapping function to be learnt sufficiently small. Besides, we notice that the upper bound gets smaller as the average estimation error of the test point’s nearest neighbors in the training dataset decreases. Hence, we aim to map the training samples to points close to their true values as well.
Next, in the light of our theoretical findings, we propose an objective function, where a term related to the preservation of the local neighborhood structure and two terms related to the Lipschitz constant of the interpolator are optimized together with a data fitting term. We choose Gaussian RBF kernels for our interpolator, which provides a smooth interpolation of training data points by preventing sudden changes in the embedding space, i.e., overfitting, thanks to the Lipschitz regularity of the Gaussian kernel. We use an alternating optimization method to minimize the objective function in an iterative fashion, in order to jointly learn the embedding and the parameters of the RBF interpolation function.
In this paper, our main contributions to the field of DL CCM estimation from UL CCM are the following:
We first present a theoretical analysis of learning interpolation functions that map UL CCMs to their DL counterparts, with the purpose of identifying the main factors that affect the estimation error of the DL CCM. Our analysis shows that the error is essentially influenced by: (i) the average estimation error of the nearest neighbors of the point in the training dataset, (ii) the Lipschitz constant of the interpolation function, and (iii) the maximum value of the ratio of the distance between two DL CCMs to the distance between their UL counterparts.
We next propose a novel representation learning method for DL CCM estimation, which builds on our theoretical results and relies on a model with much fewer parameters compared to other methods such as deep-learning based ones. The proposed method thus achieves considerably higher estimation performance in settings with limited availability of training data. Meanwhile, the nonlinear structure of the learnt model allows for successfully capturing the particular geometry of the data, making it favorable against simpler solutions such as linear transformations.
The paper is organized as follows: Section II summarizes the significant earlier works in the literature for the UL-DL CCM conversion problem. In Section III , the system model for the communication scenario is explained. In Section IV , the theoretical motivation behind our method is presented. A representation learning method for the problem of DL CCM estimation from UL CCMs is proposed in Section V . In Section VI , the performance of the proposed algorithm is compared to benchmark methods via simulations in terms of several error metrics, and a stability and sensitivity analysis is presented for the proposed algorithm. Finally, the concluding remarks are given in Section VII .
A bold lower case letter such as a denotes a vector, while a bold upper case letter as in A denotes a matrix. If A is a square matrix, A − 1 superscript A 1 \textbf{A}^{-1} A start_POSTSUPERSCRIPT - 1 end_POSTSUPERSCRIPT and t r ( A ) 𝑡 𝑟 A tr(\textbf{A}) italic_t italic_r ( A ) denote the inverse and the trace of A , respectively. ( . ) T (.)^{T} ( . ) start_POSTSUPERSCRIPT italic_T end_POSTSUPERSCRIPT and ( . ) H (.)^{H} ( . ) start_POSTSUPERSCRIPT italic_H end_POSTSUPERSCRIPT denote transpose and Hermitian operators, respectively.
II Related Work
There are several creative solutions that try to estimate the DL channel state information (CSI) in an efficient way such as [ 16 , 17 ] , or to design feedback signals in an efficient manner as in [ 18 , 19 , 20 ] . In [ 21 ] , a joint user grouping, scheduling and precoding design is developed based on CCMs of users in a multi-user environment. In [ 22 ] , a joint pilot, feedback and precoder design is developed as a solution to the problematic FDD massive MIMO implementation issue. In [ 23 ] , authors design an algorithm to find a pilot weighting matrix to shrink the feasible set of DL CCMs and find the center of the set in an FDD massive MIMO system with limited feedback and Type I codebook. In [ 24 ] , a neural network architecture is trained for DL CSI estimation and DL beamforming by extracting the joint long term properties of a wireless channel that is shared by both the UL and the DL channels due to the ”partial reciprocity” of UL/DL channels.
As the DL CSI estimation problem, DL CCM estimation is also a well-studied problem with numerous solutions proposed in the literature. In [ 8 ] , a frequency calibration matrix is suggested to convert the UL CCM to its DL counterpart by taking the carrier frequency gap between UL and DL into account. In [ 9 ] , a cubic splines method is suggested to interpolate the magnitude and the phase of DL CCM’s elements from the corresponding UL CCM’s elements. In [ 10 ] , a dictionary is formed from UL/DL CCM pairs. When the DL CCM is to be estimated at an arbitrary point, its UL CCM is first represented as a weighted average of the UL CCMs in the dictionary, and then the same weights are used to interpolate the DL CCM from the DL CCMs in the dictionary.
There are several works in the literature that explicitly exploit the angular reciprocity concept by estimating the PAS from the UL CCM and using this estimate to form the corresponding DL CCM. [ 7 , 11 , 12 ] and [ 25 ] suggest methods to estimate the DL CCM in this manner, where the PAS is discretized for the estimation process. In [ 7 , 11 ] , the power distribution is estimated at certain angles, which corresponds to taking samples of the PAS in certain angles. In [ 12 ] and [ 25 ] , the UL CCM is expressed by a system of equations, from which a discrete PAS is estimated. Then, the PAS estimation is used to find the DL CCM of the corresponding UL CCM.
On the other hand, using UL CCM to directly estimate DL CCM without explicitly finding the PAS is also an option, which is studied in several works such as [ 8 , 10 , 13 , 14 ] . The method in [ 10 ] suggests using a dictionary of UL/DL CCM pairs for deduction of a new user’s DL CCM with the help of its UL CCM and the dictionary created. A conditional generative adversarial networks (CGAN)-based method in [ 13 ] uses the image-to-image translation approach proposed in [ 26 ] by converting UL CCMs and DL CCMs into RGB images. In [ 14 ] , the elements of UL CCM are considered as a non-linear transformed version of the common PAS of the UL and DL channels. Based on this property, a linear transformation method is proposed that maps an UL CCM to its DL CCM.
In this paper, the direct approach is followed without finding a PAS estimate explicitly. In literature, the machine learning-based studies that address the UL-to-DL CCM mapping problem generally use deep neural networks for this task. Although deep learning methods are able to learn highly complicated functions, they require tremendous amount of data for successful generalization, in contrast to simpler nonlinear interpolator structures with fewer parameters as chosen in our work.
In [ 27 ] , the performance of learning a supervised nonlinear embedding via a mapping function is examined for a classification setup, where particular attention is paid to the generalization of the learned embedding to previously unseen data. [ 27 ] inspires us to develop a representation learning algorithm with a similar motivation to a distinct problem. Different from [ 27 ] , we are interested in a regression setup tailored to tackling a specific wireless communications problem, i.e., UL-to-DL CCM transformation. Our theoretical analysis aims to provide performance bounds for this particular problem setting.
III System Model
We consider an FDD single cell massive MIMO system, in which a base station (BS) containing M 𝑀 M italic_M antennas forming a uniform linear array (ULA) serves single-antenna user equipments (UE). The UL channel operates at the carrier frequency of f U L subscript 𝑓 𝑈 𝐿 f_{UL} italic_f start_POSTSUBSCRIPT italic_U italic_L end_POSTSUBSCRIPT , and the DL channel operates at the carrier frequency of f D L subscript 𝑓 𝐷 𝐿 f_{DL} italic_f start_POSTSUBSCRIPT italic_D italic_L end_POSTSUBSCRIPT . Their respective wavelengths are denoted as λ U L subscript 𝜆 𝑈 𝐿 \lambda_{UL} italic_λ start_POSTSUBSCRIPT italic_U italic_L end_POSTSUBSCRIPT and λ D L subscript 𝜆 𝐷 𝐿 \lambda_{DL} italic_λ start_POSTSUBSCRIPT italic_D italic_L end_POSTSUBSCRIPT . The ratio of carrier frequencies is denoted by f R = f D L f U L = λ U L λ D L subscript 𝑓 𝑅 subscript 𝑓 𝐷 𝐿 subscript 𝑓 𝑈 𝐿 subscript 𝜆 𝑈 𝐿 subscript 𝜆 𝐷 𝐿 f_{R}=\frac{f_{DL}}{f_{UL}}=\frac{\lambda_{UL}}{\lambda_{DL}} italic_f start_POSTSUBSCRIPT italic_R end_POSTSUBSCRIPT = divide start_ARG italic_f start_POSTSUBSCRIPT italic_D italic_L end_POSTSUBSCRIPT end_ARG start_ARG italic_f start_POSTSUBSCRIPT italic_U italic_L end_POSTSUBSCRIPT end_ARG = divide start_ARG italic_λ start_POSTSUBSCRIPT italic_U italic_L end_POSTSUBSCRIPT end_ARG start_ARG italic_λ start_POSTSUBSCRIPT italic_D italic_L end_POSTSUBSCRIPT end_ARG . The UL and the DL channels are considered to be frequency-flat.
The UL and the DL channel vectors ( h U L subscript h 𝑈 𝐿 \textbf{h}_{UL} h start_POSTSUBSCRIPT italic_U italic_L end_POSTSUBSCRIPT and h D L subscript h 𝐷 𝐿 \textbf{h}_{DL} h start_POSTSUBSCRIPT italic_D italic_L end_POSTSUBSCRIPT , respectively) are formulated as follows [ 13 ] , [ 14 ] :
(1) |
where γ ( ϕ ) 𝛾 italic-ϕ \gamma(\phi) italic_γ ( italic_ϕ ) is the complex channel gain corresponding to the angle of arrival (AoA) ϕ italic-ϕ \phi italic_ϕ and a x ( ϕ ) subscript a 𝑥 italic-ϕ \textbf{a}_{x}(\phi) a start_POSTSUBSCRIPT italic_x end_POSTSUBSCRIPT ( italic_ϕ ) is the array response vector at the angle ϕ italic-ϕ \phi italic_ϕ . The array response vectors of the UL and the DL channels ( a U L ( ϕ ) subscript a 𝑈 𝐿 italic-ϕ \textbf{a}_{UL}(\phi) a start_POSTSUBSCRIPT italic_U italic_L end_POSTSUBSCRIPT ( italic_ϕ ) and a D L ( ϕ ) subscript a 𝐷 𝐿 italic-ϕ \textbf{a}_{DL}(\phi) a start_POSTSUBSCRIPT italic_D italic_L end_POSTSUBSCRIPT ( italic_ϕ ) , respectively) are given by:
(2) |
where d = λ U L 2 𝑑 subscript 𝜆 𝑈 𝐿 2 d=\frac{\lambda_{UL}}{2} italic_d = divide start_ARG italic_λ start_POSTSUBSCRIPT italic_U italic_L end_POSTSUBSCRIPT end_ARG start_ARG 2 end_ARG .
We consider the wide sense stationary uncorrelated scattering (WSSUS) model for our communication scenario as in [ 14 ] . In this model, the autocorrelation function (acf) of the channel gain is time-invariant, and scattering at different angle of arrivals (AoA’s) are uncorrelated. Considering the UL and the DL channels as zero mean channels, the UL CCM and the DL CCM ( R U L subscript R 𝑈 𝐿 \textbf{R}_{UL} R start_POSTSUBSCRIPT italic_U italic_L end_POSTSUBSCRIPT and R D L subscript R 𝐷 𝐿 \textbf{R}_{DL} R start_POSTSUBSCRIPT italic_D italic_L end_POSTSUBSCRIPT , respectively) can then be formulated as [ 14 ] :
(3) |
¯ 𝑣 Δ 𝑝 italic-ϕ differential-d italic-ϕ 1 \int_{\bar{v}-\Delta}^{\bar{v}+\Delta}p(\phi)d\phi=1 ∫ start_POSTSUBSCRIPT over¯ start_ARG italic_v end_ARG - roman_Δ end_POSTSUBSCRIPT start_POSTSUPERSCRIPT over¯ start_ARG italic_v end_ARG + roman_Δ end_POSTSUPERSCRIPT italic_p ( italic_ϕ ) italic_d italic_ϕ = 1 . From ( 3 ) and ( 2 ), one can conclude that CCMs are Hermitian, i.e., R x = R x H subscript R 𝑥 superscript subscript R 𝑥 𝐻 \textbf{R}_{x}=\textbf{R}_{x}^{H} R start_POSTSUBSCRIPT italic_x end_POSTSUBSCRIPT = R start_POSTSUBSCRIPT italic_x end_POSTSUBSCRIPT start_POSTSUPERSCRIPT italic_H end_POSTSUPERSCRIPT , for x ∈ { U L , D L } 𝑥 𝑈 𝐿 𝐷 𝐿 x\in\{{UL,DL}\} italic_x ∈ { italic_U italic_L , italic_D italic_L } . The ULA antenna structure and the WSSUS model lead CCMs to be Toeplitz. Due to its Hermitian and Toeplitz structure, the R x subscript R 𝑥 \textbf{R}_{x} R start_POSTSUBSCRIPT italic_x end_POSTSUBSCRIPT matrix given in ( 3 ) can be represented by its first row.
IV Performance Bounds for DL CCM Estimation via Gaussian RBF kernels
In this section, first, the representation learning setting considered for the problem of DL CCM estimation from UL CCM is presented. Then, an upper bound on the error of an arbitrary test sample is provided.
IV-A Notation and Setting
Let { r U L i , r D L i } i = 1 N superscript subscript superscript subscript r 𝑈 𝐿 𝑖 superscript subscript r 𝐷 𝐿 𝑖 𝑖 1 𝑁 \{{\textbf{r}_{UL}}^{i},{\textbf{r}_{DL}}^{i}\}_{i=1}^{N} { r start_POSTSUBSCRIPT italic_U italic_L end_POSTSUBSCRIPT start_POSTSUPERSCRIPT italic_i end_POSTSUPERSCRIPT , r start_POSTSUBSCRIPT italic_D italic_L end_POSTSUBSCRIPT start_POSTSUPERSCRIPT italic_i end_POSTSUPERSCRIPT } start_POSTSUBSCRIPT italic_i = 1 end_POSTSUBSCRIPT start_POSTSUPERSCRIPT italic_N end_POSTSUPERSCRIPT be a training dataset with N 𝑁 N italic_N training UL/DL CCM sample pairs, where r x i ∈ ℝ 1 × 2 M − 1 superscript subscript r 𝑥 𝑖 superscript ℝ 1 2 𝑀 1 {\textbf{r}_{x}}^{i}\in\mathbb{R}^{1\times 2M-1} r start_POSTSUBSCRIPT italic_x end_POSTSUBSCRIPT start_POSTSUPERSCRIPT italic_i end_POSTSUPERSCRIPT ∈ blackboard_R start_POSTSUPERSCRIPT 1 × 2 italic_M - 1 end_POSTSUPERSCRIPT is a row vector obtained by the concatenation of the real and imaginary parts of the first row vector of the i t h superscript 𝑖 𝑡 ℎ i^{th} italic_i start_POSTSUPERSCRIPT italic_t italic_h end_POSTSUPERSCRIPT CCM in the training dataset for x ∈ { U L , D L } 𝑥 𝑈 𝐿 𝐷 𝐿 x\in\{UL,DL\} italic_x ∈ { italic_U italic_L , italic_D italic_L } . The first element of the first row of a CCM is always real, so it has no imaginary part. Hence, the vectors in the dataset are of length 2 M − 1 2 𝑀 1 2M-1 2 italic_M - 1 . Let the UL data samples be drawn i.i.d. from a probability measure υ 𝜐 \upsilon italic_υ on ℝ 1 × 2 M − 1 superscript ℝ 1 2 𝑀 1 \mathbb{R}^{1\times 2M-1} blackboard_R start_POSTSUPERSCRIPT 1 × 2 italic_M - 1 end_POSTSUPERSCRIPT . The training samples are embedded into ℝ 1 × 2 M − 1 superscript ℝ 1 2 𝑀 1 \mathbb{R}^{1\times 2M-1} blackboard_R start_POSTSUPERSCRIPT 1 × 2 italic_M - 1 end_POSTSUPERSCRIPT such that each training sample r U L i superscript subscript r 𝑈 𝐿 𝑖 {\textbf{r}_{UL}}^{i} r start_POSTSUBSCRIPT italic_U italic_L end_POSTSUBSCRIPT start_POSTSUPERSCRIPT italic_i end_POSTSUPERSCRIPT is mapped to a vector r ^ D L i ∈ ℝ 1 × 2 M − 1 superscript subscript ^ r 𝐷 𝐿 𝑖 superscript ℝ 1 2 𝑀 1 {{\hat{\textbf{r}}}_{DL}}^{i}\in\mathbb{R}^{1\times 2M-1} over^ start_ARG r end_ARG start_POSTSUBSCRIPT italic_D italic_L end_POSTSUBSCRIPT start_POSTSUPERSCRIPT italic_i end_POSTSUPERSCRIPT ∈ blackboard_R start_POSTSUPERSCRIPT 1 × 2 italic_M - 1 end_POSTSUPERSCRIPT .The mapping is assumed to be extended to the whole data space through an interpolation function f : ℝ 1 × 2 M − 1 → ℝ 1 × 2 M − 1 : 𝑓 → superscript ℝ 1 2 𝑀 1 superscript ℝ 1 2 𝑀 1 f:\mathbb{R}^{1\times 2M-1}\rightarrow\mathbb{R}^{1\times 2M-1} italic_f : blackboard_R start_POSTSUPERSCRIPT 1 × 2 italic_M - 1 end_POSTSUPERSCRIPT → blackboard_R start_POSTSUPERSCRIPT 1 × 2 italic_M - 1 end_POSTSUPERSCRIPT such that each training sample is mapped to its embedding as f ( r U L i ) = r ^ D L i 𝑓 superscript subscript r 𝑈 𝐿 𝑖 superscript subscript ^ r 𝐷 𝐿 𝑖 f({\textbf{r}_{UL}}^{i})={{\hat{\textbf{r}}}_{DL}}^{i} italic_f ( r start_POSTSUBSCRIPT italic_U italic_L end_POSTSUBSCRIPT start_POSTSUPERSCRIPT italic_i end_POSTSUPERSCRIPT ) = over^ start_ARG r end_ARG start_POSTSUBSCRIPT italic_D italic_L end_POSTSUBSCRIPT start_POSTSUPERSCRIPT italic_i end_POSTSUPERSCRIPT . Let r U L t e s t superscript subscript r 𝑈 𝐿 𝑡 𝑒 𝑠 𝑡 {\textbf{r}_{UL}}^{test} r start_POSTSUBSCRIPT italic_U italic_L end_POSTSUBSCRIPT start_POSTSUPERSCRIPT italic_t italic_e italic_s italic_t end_POSTSUPERSCRIPT be the concatenated vector of an arbitrary UL CCM test point and B δ ( r U L t e s t ) subscript 𝐵 𝛿 superscript subscript r 𝑈 𝐿 𝑡 𝑒 𝑠 𝑡 B_{\delta}({\textbf{r}_{UL}}^{test}) italic_B start_POSTSUBSCRIPT italic_δ end_POSTSUBSCRIPT ( r start_POSTSUBSCRIPT italic_U italic_L end_POSTSUBSCRIPT start_POSTSUPERSCRIPT italic_t italic_e italic_s italic_t end_POSTSUPERSCRIPT ) be an open ball of radius δ 𝛿 \delta italic_δ around it:
(4) |
Let A U L superscript 𝐴 𝑈 𝐿 A^{UL} italic_A start_POSTSUPERSCRIPT italic_U italic_L end_POSTSUPERSCRIPT be the set of the training samples within a δ 𝛿 \delta italic_δ -neighborhood of r U L t e s t superscript subscript r 𝑈 𝐿 𝑡 𝑒 𝑠 𝑡 {\textbf{r}_{UL}}^{test} r start_POSTSUBSCRIPT italic_U italic_L end_POSTSUBSCRIPT start_POSTSUPERSCRIPT italic_t italic_e italic_s italic_t end_POSTSUPERSCRIPT in ℝ 1 × 2 M − 1 superscript ℝ 1 2 𝑀 1 \mathbb{R}^{1\times 2M-1} blackboard_R start_POSTSUPERSCRIPT 1 × 2 italic_M - 1 end_POSTSUPERSCRIPT
(5) |
Denoting the support of the probability measure υ 𝜐 \upsilon italic_υ as ℳ ⊂ ℝ 1 × 2 M − 1 ℳ superscript ℝ 1 2 𝑀 1 \mathcal{M}\subset\mathbb{R}^{1\times 2M-1} caligraphic_M ⊂ blackboard_R start_POSTSUPERSCRIPT 1 × 2 italic_M - 1 end_POSTSUPERSCRIPT , we define
(6) |
which is a lower bound on the measure of the open ball B δ ( r U L t e s t ) subscript 𝐵 𝛿 superscript subscript r 𝑈 𝐿 𝑡 𝑒 𝑠 𝑡 B_{\delta}\left({\textbf{r}_{UL}}^{test}\right) italic_B start_POSTSUBSCRIPT italic_δ end_POSTSUBSCRIPT ( r start_POSTSUBSCRIPT italic_U italic_L end_POSTSUBSCRIPT start_POSTSUPERSCRIPT italic_t italic_e italic_s italic_t end_POSTSUPERSCRIPT ) around any test point.
IV-B Theoretical Analysis for Motivation Behind the Proposed Method
We now present a theoretical analysis of the regression problem of UL-to-DL CCM conversion via a mapping function f ( ⋅ ) 𝑓 ⋅ f(\cdot) italic_f ( ⋅ ) . We consider a setting with the following assumptions:
- subscript r 1 subscript r 2 superscript ℝ 1 2 𝑀 1 \textbf{r}_{1},\textbf{r}_{2}\in\mathbb{R}^{1\times 2M-1} r start_POSTSUBSCRIPT 1 end_POSTSUBSCRIPT , r start_POSTSUBSCRIPT 2 end_POSTSUBSCRIPT ∈ blackboard_R start_POSTSUPERSCRIPT 1 × 2 italic_M - 1 end_POSTSUPERSCRIPT , ‖ f ( r 1 ) − f ( r 2 ) ‖ ≤ L ‖ r 1 − r 2 ‖ norm 𝑓 subscript r 1 𝑓 subscript r 2 𝐿 norm subscript r 1 subscript r 2 \|f(\textbf{r}_{1})-f(\textbf{r}_{2})\|\leq L\|\textbf{r}_{1}-\textbf{r}_{2}\| ∥ italic_f ( r start_POSTSUBSCRIPT 1 end_POSTSUBSCRIPT ) - italic_f ( r start_POSTSUBSCRIPT 2 end_POSTSUBSCRIPT ) ∥ ≤ italic_L ∥ r start_POSTSUBSCRIPT 1 end_POSTSUBSCRIPT - r start_POSTSUBSCRIPT 2 end_POSTSUBSCRIPT ∥ .
The probability measure υ 𝜐 \upsilon italic_υ has a bounded support ℳ ⊂ ℝ 1 × 2 M − 1 ℳ superscript ℝ 1 2 𝑀 1 \mathcal{M}\subset\mathbb{R}^{1\times 2M-1} caligraphic_M ⊂ blackboard_R start_POSTSUPERSCRIPT 1 × 2 italic_M - 1 end_POSTSUPERSCRIPT .
For any δ > 0 𝛿 0 \delta>0 italic_δ > 0 , the probability measure lower bound η δ subscript 𝜂 𝛿 \eta_{\delta} italic_η start_POSTSUBSCRIPT italic_δ end_POSTSUBSCRIPT is strictly positive, i.e., η δ > 0 subscript 𝜂 𝛿 0 \eta_{\delta}>0 italic_η start_POSTSUBSCRIPT italic_δ end_POSTSUBSCRIPT > 0 .
We examine the relation between the local geometries of the UL CCM and the DL CCM spaces with the following theorem:
Theorem 1 .
Let p U L i ∈ ℝ 1 × 2 M − 1 superscript subscript p 𝑈 𝐿 𝑖 superscript ℝ 1 2 𝑀 1 {\textbf{p}_{UL}}^{i}\in\mathbb{R}^{1\times 2M-1} p start_POSTSUBSCRIPT italic_U italic_L end_POSTSUBSCRIPT start_POSTSUPERSCRIPT italic_i end_POSTSUPERSCRIPT ∈ blackboard_R start_POSTSUPERSCRIPT 1 × 2 italic_M - 1 end_POSTSUPERSCRIPT and p U L j ∈ ℝ 1 × 2 M − 1 superscript subscript p 𝑈 𝐿 𝑗 superscript ℝ 1 2 𝑀 1 {\textbf{p}_{UL}}^{j}\in\mathbb{R}^{1\times 2M-1} p start_POSTSUBSCRIPT italic_U italic_L end_POSTSUBSCRIPT start_POSTSUPERSCRIPT italic_j end_POSTSUPERSCRIPT ∈ blackboard_R start_POSTSUPERSCRIPT 1 × 2 italic_M - 1 end_POSTSUPERSCRIPT be obtained by concatenating the real and imaginary parts of the first rows of two arbitrary UL CCMs, i.e., let p U L i superscript subscript p 𝑈 𝐿 𝑖 {\textbf{p}_{UL}}^{i} p start_POSTSUBSCRIPT italic_U italic_L end_POSTSUBSCRIPT start_POSTSUPERSCRIPT italic_i end_POSTSUPERSCRIPT and p U L j superscript subscript p 𝑈 𝐿 𝑗 {\textbf{p}_{UL}}^{j} p start_POSTSUBSCRIPT italic_U italic_L end_POSTSUBSCRIPT start_POSTSUPERSCRIPT italic_j end_POSTSUPERSCRIPT be drawn i.i.d. from the probability measure υ 𝜐 \upsilon italic_υ . Let p D L i superscript subscript p 𝐷 𝐿 𝑖 {\textbf{p}_{DL}}^{i} p start_POSTSUBSCRIPT italic_D italic_L end_POSTSUBSCRIPT start_POSTSUPERSCRIPT italic_i end_POSTSUPERSCRIPT and p D L j superscript subscript p 𝐷 𝐿 𝑗 {\textbf{p}_{DL}}^{j} p start_POSTSUBSCRIPT italic_D italic_L end_POSTSUBSCRIPT start_POSTSUPERSCRIPT italic_j end_POSTSUPERSCRIPT be their DL counterparts. If ‖ p U L i − p U L j ‖ ≤ 2 δ norm superscript subscript p 𝑈 𝐿 𝑖 superscript subscript p 𝑈 𝐿 𝑗 2 𝛿 \left\|{\textbf{p}_{UL}}^{i}-{\textbf{p}_{UL}}^{j}\right\|\leq 2\delta ∥ p start_POSTSUBSCRIPT italic_U italic_L end_POSTSUBSCRIPT start_POSTSUPERSCRIPT italic_i end_POSTSUPERSCRIPT - p start_POSTSUBSCRIPT italic_U italic_L end_POSTSUBSCRIPT start_POSTSUPERSCRIPT italic_j end_POSTSUPERSCRIPT ∥ ≤ 2 italic_δ , then, there exists a constant K > 0 𝐾 0 K>0 italic_K > 0 such that ‖ p D L i − p D L j ‖ ≤ K ‖ p U L i − p U L j ‖ ≤ 2 K δ norm superscript subscript p 𝐷 𝐿 𝑖 superscript subscript p 𝐷 𝐿 𝑗 𝐾 norm superscript subscript p 𝑈 𝐿 𝑖 superscript subscript p 𝑈 𝐿 𝑗 2 𝐾 𝛿 \left\|{\textbf{p}_{DL}}^{i}-{\textbf{p}_{DL}}^{j}\right\|\leq K\left\|{% \textbf{p}_{UL}}^{i}-{\textbf{p}_{UL}}^{j}\right\|\leq 2K\delta ∥ p start_POSTSUBSCRIPT italic_D italic_L end_POSTSUBSCRIPT start_POSTSUPERSCRIPT italic_i end_POSTSUPERSCRIPT - p start_POSTSUBSCRIPT italic_D italic_L end_POSTSUBSCRIPT start_POSTSUPERSCRIPT italic_j end_POSTSUPERSCRIPT ∥ ≤ italic_K ∥ p start_POSTSUBSCRIPT italic_U italic_L end_POSTSUBSCRIPT start_POSTSUPERSCRIPT italic_i end_POSTSUPERSCRIPT - p start_POSTSUBSCRIPT italic_U italic_L end_POSTSUBSCRIPT start_POSTSUPERSCRIPT italic_j end_POSTSUPERSCRIPT ∥ ≤ 2 italic_K italic_δ , under the following assumptions:
The PAS, p ( ϕ ) 𝑝 italic-ϕ p(\phi) italic_p ( italic_ϕ ) , is uniform.
δ 𝛿 \delta italic_δ is so small that two points in a δ 𝛿 \delta italic_δ ball of a test point, say point i 𝑖 i italic_i and point j 𝑗 j italic_j , have very close mean Angle of Arrival (AoA) values, i.e., v i ¯ − v j ¯ ≈ 0 ¯ subscript 𝑣 𝑖 ¯ subscript 𝑣 𝑗 0 \bar{v_{i}}-\bar{v_{j}}\approx 0 over¯ start_ARG italic_v start_POSTSUBSCRIPT italic_i end_POSTSUBSCRIPT end_ARG - over¯ start_ARG italic_v start_POSTSUBSCRIPT italic_j end_POSTSUBSCRIPT end_ARG ≈ 0 .
The spread of AoA, Δ Δ \Delta roman_Δ , of each data point in the dataset is constant and the same.
Proof of Theorem 1 .
The square of the norm of the difference between p U L i ∈ ℝ 1 × 2 M − 1 superscript subscript p 𝑈 𝐿 𝑖 superscript ℝ 1 2 𝑀 1 {\textbf{p}_{UL}}^{i}\in\mathbb{R}^{1\times 2M-1} p start_POSTSUBSCRIPT italic_U italic_L end_POSTSUBSCRIPT start_POSTSUPERSCRIPT italic_i end_POSTSUPERSCRIPT ∈ blackboard_R start_POSTSUPERSCRIPT 1 × 2 italic_M - 1 end_POSTSUPERSCRIPT and p U L j ∈ ℝ 1 × 2 M − 1 superscript subscript p 𝑈 𝐿 𝑗 superscript ℝ 1 2 𝑀 1 {\textbf{p}_{UL}}^{j}\in\mathbb{R}^{1\times 2M-1} p start_POSTSUBSCRIPT italic_U italic_L end_POSTSUBSCRIPT start_POSTSUPERSCRIPT italic_j end_POSTSUPERSCRIPT ∈ blackboard_R start_POSTSUPERSCRIPT 1 × 2 italic_M - 1 end_POSTSUPERSCRIPT , which are drawn i.i.d. from υ 𝜐 \upsilon italic_υ , is given as follows:
(7) |
¯ subscript 𝑣 𝑖 Δ \phi\in\left[\bar{v_{j}}+\Delta,\bar{v_{i}}+\Delta\right] italic_ϕ ∈ [ over¯ start_ARG italic_v start_POSTSUBSCRIPT italic_j end_POSTSUBSCRIPT end_ARG + roman_Δ , over¯ start_ARG italic_v start_POSTSUBSCRIPT italic_i end_POSTSUBSCRIPT end_ARG + roman_Δ ] ,
| (8) |
subscript 𝛼 1 italic-ϕ subscript 𝛽 1 \theta\approx\alpha_{1}\phi+\beta_{1} italic_θ ≈ italic_α start_POSTSUBSCRIPT 1 end_POSTSUBSCRIPT italic_ϕ + italic_β start_POSTSUBSCRIPT 1 end_POSTSUBSCRIPT , where
| (9) |
| (10) |
Similarly, for ϕ ∈ [ v j ¯ − Δ , v i ¯ − Δ ] italic-ϕ ¯ subscript 𝑣 𝑗 Δ ¯ subscript 𝑣 𝑖 Δ \phi\in\left[\bar{v_{j}}-\Delta,\bar{v_{i}}-\Delta\right] italic_ϕ ∈ [ over¯ start_ARG italic_v start_POSTSUBSCRIPT italic_j end_POSTSUBSCRIPT end_ARG - roman_Δ , over¯ start_ARG italic_v start_POSTSUBSCRIPT italic_i end_POSTSUBSCRIPT end_ARG - roman_Δ ] ,
(11) |
subscript 𝛼 2 italic-ϕ subscript 𝛽 2 \theta\approx\alpha_{2}\phi+\beta_{2} italic_θ ≈ italic_α start_POSTSUBSCRIPT 2 end_POSTSUBSCRIPT italic_ϕ + italic_β start_POSTSUBSCRIPT 2 end_POSTSUBSCRIPT , where
(12) |
(13) |
Using the approximations in Appendix A , one can write | [ p U L i ] m − [ p U L j ] m | 2 superscript subscript delimited-[] superscript subscript p 𝑈 𝐿 𝑖 𝑚 subscript delimited-[] superscript subscript p 𝑈 𝐿 𝑗 𝑚 2 \left|[{\textbf{p}_{UL}}^{i}]_{m}-[{\textbf{p}_{UL}}^{j}]_{m}\right|^{2} | [ p start_POSTSUBSCRIPT italic_U italic_L end_POSTSUBSCRIPT start_POSTSUPERSCRIPT italic_i end_POSTSUPERSCRIPT ] start_POSTSUBSCRIPT italic_m end_POSTSUBSCRIPT - [ p start_POSTSUBSCRIPT italic_U italic_L end_POSTSUBSCRIPT start_POSTSUPERSCRIPT italic_j end_POSTSUPERSCRIPT ] start_POSTSUBSCRIPT italic_m end_POSTSUBSCRIPT | start_POSTSUPERSCRIPT 2 end_POSTSUPERSCRIPT as the following:
(14) |
(15) |
| (16) |
Note that for v i ¯ − v j ¯ ≈ 0 ¯ subscript 𝑣 𝑖 ¯ subscript 𝑣 𝑗 0 \bar{v_{i}}-\bar{v_{j}}\approx 0 over¯ start_ARG italic_v start_POSTSUBSCRIPT italic_i end_POSTSUBSCRIPT end_ARG - over¯ start_ARG italic_v start_POSTSUBSCRIPT italic_j end_POSTSUBSCRIPT end_ARG ≈ 0 , by using first order Taylor expansion, we arrive at sin ( ( m − 1 ) α 1 ( v i ¯ − v j ¯ ) 2 ) ≈ ( m − 1 ) α 1 ( v i ¯ − v j ¯ ) 2 𝑚 1 subscript 𝛼 1 ¯ subscript 𝑣 𝑖 ¯ subscript 𝑣 𝑗 2 𝑚 1 subscript 𝛼 1 ¯ subscript 𝑣 𝑖 ¯ subscript 𝑣 𝑗 2 \sin\left((m-1)\frac{\alpha_{1}(\bar{v_{i}}-\bar{v_{j}})}{2}\right)\approx(m-1% )\frac{\alpha_{1}(\bar{v_{i}}-\bar{v_{j}})}{2} roman_sin ( ( italic_m - 1 ) divide start_ARG italic_α start_POSTSUBSCRIPT 1 end_POSTSUBSCRIPT ( over¯ start_ARG italic_v start_POSTSUBSCRIPT italic_i end_POSTSUBSCRIPT end_ARG - over¯ start_ARG italic_v start_POSTSUBSCRIPT italic_j end_POSTSUBSCRIPT end_ARG ) end_ARG start_ARG 2 end_ARG ) ≈ ( italic_m - 1 ) divide start_ARG italic_α start_POSTSUBSCRIPT 1 end_POSTSUBSCRIPT ( over¯ start_ARG italic_v start_POSTSUBSCRIPT italic_i end_POSTSUBSCRIPT end_ARG - over¯ start_ARG italic_v start_POSTSUBSCRIPT italic_j end_POSTSUBSCRIPT end_ARG ) end_ARG start_ARG 2 end_ARG and sin ( ( m − 1 ) α 2 ( v i ¯ − v j ¯ ) 2 ) ≈ ( m − 1 ) α 2 ( v i ¯ − v j ¯ ) 2 𝑚 1 subscript 𝛼 2 ¯ subscript 𝑣 𝑖 ¯ subscript 𝑣 𝑗 2 𝑚 1 subscript 𝛼 2 ¯ subscript 𝑣 𝑖 ¯ subscript 𝑣 𝑗 2 \sin\left((m-1)\frac{\alpha_{2}(\bar{v_{i}}-\bar{v_{j}})}{2}\right)\approx(m-1% )\frac{\alpha_{2}(\bar{v_{i}}-\bar{v_{j}})}{2} roman_sin ( ( italic_m - 1 ) divide start_ARG italic_α start_POSTSUBSCRIPT 2 end_POSTSUBSCRIPT ( over¯ start_ARG italic_v start_POSTSUBSCRIPT italic_i end_POSTSUBSCRIPT end_ARG - over¯ start_ARG italic_v start_POSTSUBSCRIPT italic_j end_POSTSUBSCRIPT end_ARG ) end_ARG start_ARG 2 end_ARG ) ≈ ( italic_m - 1 ) divide start_ARG italic_α start_POSTSUBSCRIPT 2 end_POSTSUBSCRIPT ( over¯ start_ARG italic_v start_POSTSUBSCRIPT italic_i end_POSTSUBSCRIPT end_ARG - over¯ start_ARG italic_v start_POSTSUBSCRIPT italic_j end_POSTSUBSCRIPT end_ARG ) end_ARG start_ARG 2 end_ARG for all m ∈ { 1 , … , M } 𝑚 1 … 𝑀 m\in\{1,...,M\} italic_m ∈ { 1 , … , italic_M } . The expression in ( 16 ) can then be approximated as
|
|
|
superscript subscript ¯ 𝑣 Δ \Delta_{\sin}:=\sin\left(\bar{v}_{\Delta}^{+}\right)-\sin\left(\bar{v}_{\Delta% }^{-}\right) roman_Δ start_POSTSUBSCRIPT roman_sin end_POSTSUBSCRIPT := roman_sin ( over¯ start_ARG italic_v end_ARG start_POSTSUBSCRIPT roman_Δ end_POSTSUBSCRIPT start_POSTSUPERSCRIPT + end_POSTSUPERSCRIPT ) - roman_sin ( over¯ start_ARG italic_v end_ARG start_POSTSUBSCRIPT roman_Δ end_POSTSUBSCRIPT start_POSTSUPERSCRIPT - end_POSTSUPERSCRIPT ) . Then, one can write
(17) |
Similarly, one can approximate ‖ p D L i − p D L j ‖ 2 superscript norm superscript subscript p 𝐷 𝐿 𝑖 superscript subscript p 𝐷 𝐿 𝑗 2 \|{\textbf{p}_{DL}}^{i}-{\textbf{p}_{DL}}^{j}\|^{2} ∥ p start_POSTSUBSCRIPT italic_D italic_L end_POSTSUBSCRIPT start_POSTSUPERSCRIPT italic_i end_POSTSUPERSCRIPT - p start_POSTSUBSCRIPT italic_D italic_L end_POSTSUBSCRIPT start_POSTSUPERSCRIPT italic_j end_POSTSUPERSCRIPT ∥ start_POSTSUPERSCRIPT 2 end_POSTSUPERSCRIPT as the following:
(18) |
(19) |
Let us denote the sine ratio as R sin := ∑ m = 1 M ( sin ( f R ( m − 1 ) π Δ sin 2 ) ) 2 ∑ m = 1 M ( sin ( ( m − 1 ) π Δ sin 2 ) ) 2 assign subscript 𝑅 superscript subscript 𝑚 1 𝑀 superscript subscript 𝑓 𝑅 𝑚 1 𝜋 subscript Δ 2 2 superscript subscript 𝑚 1 𝑀 superscript 𝑚 1 𝜋 subscript Δ 2 2 R_{\sin}:=\frac{\sum_{m=1}^{M}(\sin(f_{R}(m-1)\pi\frac{\Delta_{\sin}}{2}))^{2}% }{\sum_{m=1}^{M}(\sin((m-1)\pi\frac{\Delta_{\sin}}{2}))^{2}} italic_R start_POSTSUBSCRIPT roman_sin end_POSTSUBSCRIPT := divide start_ARG ∑ start_POSTSUBSCRIPT italic_m = 1 end_POSTSUBSCRIPT start_POSTSUPERSCRIPT italic_M end_POSTSUPERSCRIPT ( roman_sin ( italic_f start_POSTSUBSCRIPT italic_R end_POSTSUBSCRIPT ( italic_m - 1 ) italic_π divide start_ARG roman_Δ start_POSTSUBSCRIPT roman_sin end_POSTSUBSCRIPT end_ARG start_ARG 2 end_ARG ) ) start_POSTSUPERSCRIPT 2 end_POSTSUPERSCRIPT end_ARG start_ARG ∑ start_POSTSUBSCRIPT italic_m = 1 end_POSTSUBSCRIPT start_POSTSUPERSCRIPT italic_M end_POSTSUPERSCRIPT ( roman_sin ( ( italic_m - 1 ) italic_π divide start_ARG roman_Δ start_POSTSUBSCRIPT roman_sin end_POSTSUBSCRIPT end_ARG start_ARG 2 end_ARG ) ) start_POSTSUPERSCRIPT 2 end_POSTSUPERSCRIPT end_ARG . The K 𝐾 K italic_K constant introduced in Theorem 1 can then be defined as the maximum value that R sin subscript 𝑅 R_{\sin} italic_R start_POSTSUBSCRIPT roman_sin end_POSTSUBSCRIPT can take. ∎
Motivated by Theorem 1 , for the given special case where the PAS is uniform and the angular spread of each user in a dataset is the same, one can say that if two points are close to each other in the UL CCM space, they should be close to each other in the DL CCM space as well. In practice, the constant K 𝐾 K italic_K takes values close to f R subscript 𝑓 𝑅 f_{R} italic_f start_POSTSUBSCRIPT italic_R end_POSTSUBSCRIPT in realistic settings. We demonstrate this with a numerical analysis in Appendix D . Overall, Theorem 1 provides useful insight for settings where a mapping function is to be learned between the spaces of UL CCMs and DL CCMs.
For a sufficiently large dataset, i.e., for a sufficiently high N 𝑁 N italic_N value, the distance between a point in the dataset and its nearest neighbors becomes considerably small, so that one can think of the ball radius parameter δ 𝛿 \delta italic_δ as a small constant. In Theorem 2 , we consider such a setting and provide an upper bound on the test error of the estimate of an arbitrary test point obtained via the interpolation function f ( ⋅ ) 𝑓 ⋅ f(\cdot) italic_f ( ⋅ ) .
Theorem 2 .
Let the training sample set contain at least N 𝑁 N italic_N training samples { r U L i } i = 1 N superscript subscript superscript subscript r 𝑈 𝐿 𝑖 𝑖 1 𝑁 \{{\textbf{r}_{UL}}^{i}\}_{i=1}^{N} { r start_POSTSUBSCRIPT italic_U italic_L end_POSTSUBSCRIPT start_POSTSUPERSCRIPT italic_i end_POSTSUPERSCRIPT } start_POSTSUBSCRIPT italic_i = 1 end_POSTSUBSCRIPT start_POSTSUPERSCRIPT italic_N end_POSTSUPERSCRIPT with r U L i ∼ υ similar-to superscript subscript r 𝑈 𝐿 𝑖 𝜐 {\textbf{r}_{UL}}^{i}\sim\upsilon r start_POSTSUBSCRIPT italic_U italic_L end_POSTSUBSCRIPT start_POSTSUPERSCRIPT italic_i end_POSTSUPERSCRIPT ∼ italic_υ . Let r U L t e s t superscript subscript r 𝑈 𝐿 𝑡 𝑒 𝑠 𝑡 {{\textbf{r}}_{UL}}^{test} r start_POSTSUBSCRIPT italic_U italic_L end_POSTSUBSCRIPT start_POSTSUPERSCRIPT italic_t italic_e italic_s italic_t end_POSTSUPERSCRIPT be a test sample drawn from υ 𝜐 \upsilon italic_υ independently of the training samples. Assume that the interpolation function f : ℝ 1 × 2 M − 1 → ℝ 1 × 2 M − 1 : 𝑓 → superscript ℝ 1 2 𝑀 1 superscript ℝ 1 2 𝑀 1 f:\mathbb{R}^{1\times 2M-1}\rightarrow\mathbb{R}^{1\times 2M-1} italic_f : blackboard_R start_POSTSUPERSCRIPT 1 × 2 italic_M - 1 end_POSTSUPERSCRIPT → blackboard_R start_POSTSUPERSCRIPT 1 × 2 italic_M - 1 end_POSTSUPERSCRIPT is a Lipschitz continuous function with Lipschitz constant L 𝐿 L italic_L . Let ϵ > 0 italic-ϵ 0 \epsilon>0 italic_ϵ > 0 , 1 N η δ ≤ a < 1 1 𝑁 subscript 𝜂 𝛿 𝑎 1 \frac{1}{N\eta_{\delta}}\leq a<1 divide start_ARG 1 end_ARG start_ARG italic_N italic_η start_POSTSUBSCRIPT italic_δ end_POSTSUBSCRIPT end_ARG ≤ italic_a < 1 and δ > 0 𝛿 0 \delta>0 italic_δ > 0 be arbitrary constants. Then, for a dataset with users having uniform PAS ( p ( ϕ ) 𝑝 italic-ϕ p(\phi) italic_p ( italic_ϕ ) ) with the same AS ( Δ Δ \Delta roman_Δ ), for sufficiently large N 𝑁 N italic_N , with probability at least
(20) |
the following inequality holds
(21) |
The proof of Theorem 2 is given in Appendix B .
Fixing the probability parameters δ > 0 𝛿 0 \delta>0 italic_δ > 0 and ϵ > 0 italic-ϵ 0 \epsilon>0 italic_ϵ > 0 to sufficiently small constant values, one can see that the probability expression given in ( 20 ) approaches 1 at an exponential rate, as N → ∞ → 𝑁 N\rightarrow\infty italic_N → ∞ . Thus, it can be concluded that as N → ∞ → 𝑁 N\rightarrow\infty italic_N → ∞ , with probability approaching 1, the difference between a test point’s estimation error and the average estimation error of training points within the δ 𝛿 {\delta} italic_δ -neighborhood of the test point can be made as small as desired. (One can choose the δ 𝛿 \delta italic_δ parameter sufficiently close to 0 0 , as N → ∞ → 𝑁 N\rightarrow\infty italic_N → ∞ .) From this result, one can conclude the following:
The smaller the average estimation error of the training points in the δ 𝛿 {\delta} italic_δ -neighborhood of the test point can be made via the algorithm used to learn the f ( ⋅ ) 𝑓 ⋅ f(\cdot) italic_f ( ⋅ ) function, the smaller the upper bound on the estimation error of the test point gets. This can be achieved by arranging the objective function of the algorithm accordingly.
Learning a function f ( ⋅ ) 𝑓 ⋅ f(\cdot) italic_f ( ⋅ ) with a low Lipschitz constant L 𝐿 L italic_L leads to a faster decrease in the upper bound. This can also be achieved by proper adjustments in the objective function of the algorithm. In practice, our result puts forward the following trade-off between the Lipschitz constant L 𝐿 L italic_L and the training error: While one may reduce the training error to arbitrarily small values by increasing the complexity of f ( ⋅ ) 𝑓 ⋅ f(\cdot) italic_f ( ⋅ ) , this may come at the cost of learning a too irregular function with high Lipschitz constant L 𝐿 L italic_L . Consequently, this causes poor generalization to new test data. A better strategy is to seek a trade-off between the minimization of the training error and the regularity of the learned interpolator f ( ⋅ ) 𝑓 ⋅ f(\cdot) italic_f ( ⋅ ) .
V DL CCM Estimation
In this section, we propose a representation learning algorithm motivated by the theoretical analysis in the previous section for the problem of DL CCM estimation from UL CCM.
V-A Problem Formulation
Let X = [ ( r U L 1 ) T … ( r U L N ) T ] T ∈ ℝ N × ( 2 M − 1 ) X superscript delimited-[] superscript superscript subscript r 𝑈 𝐿 1 𝑇 … superscript superscript subscript r 𝑈 𝐿 𝑁 𝑇 𝑇 superscript ℝ 𝑁 2 𝑀 1 \textbf{X}=[\left({\textbf{r}_{UL}}^{1}\right)^{T}...\left({\textbf{r}_{UL}}^{% N}\right)^{T}]^{T}\in\mathbb{R}^{N\times(2M-1)} X = [ ( r start_POSTSUBSCRIPT italic_U italic_L end_POSTSUBSCRIPT start_POSTSUPERSCRIPT 1 end_POSTSUPERSCRIPT ) start_POSTSUPERSCRIPT italic_T end_POSTSUPERSCRIPT … ( r start_POSTSUBSCRIPT italic_U italic_L end_POSTSUBSCRIPT start_POSTSUPERSCRIPT italic_N end_POSTSUPERSCRIPT ) start_POSTSUPERSCRIPT italic_T end_POSTSUPERSCRIPT ] start_POSTSUPERSCRIPT italic_T end_POSTSUPERSCRIPT ∈ blackboard_R start_POSTSUPERSCRIPT italic_N × ( 2 italic_M - 1 ) end_POSTSUPERSCRIPT be the input training data matrix. Let R ^ = [ ( r ^ D L 1 ) T … ( r ^ D L N ) T ] T ∈ ℝ N × ( 2 M − 1 ) ^ R superscript delimited-[] superscript superscript subscript ^ r 𝐷 𝐿 1 𝑇 … superscript superscript subscript ^ r 𝐷 𝐿 𝑁 𝑇 𝑇 superscript ℝ 𝑁 2 𝑀 1 \hat{\textbf{R}}=[\left({\hat{\textbf{r}}_{DL}}^{1}\right)^{T}...\left({\hat{% \textbf{r}}_{DL}}^{N}\right)^{T}]^{T}\in\mathbb{R}^{N\times(2M-1)} over^ start_ARG R end_ARG = [ ( over^ start_ARG r end_ARG start_POSTSUBSCRIPT italic_D italic_L end_POSTSUBSCRIPT start_POSTSUPERSCRIPT 1 end_POSTSUPERSCRIPT ) start_POSTSUPERSCRIPT italic_T end_POSTSUPERSCRIPT … ( over^ start_ARG r end_ARG start_POSTSUBSCRIPT italic_D italic_L end_POSTSUBSCRIPT start_POSTSUPERSCRIPT italic_N end_POSTSUPERSCRIPT ) start_POSTSUPERSCRIPT italic_T end_POSTSUPERSCRIPT ] start_POSTSUPERSCRIPT italic_T end_POSTSUPERSCRIPT ∈ blackboard_R start_POSTSUPERSCRIPT italic_N × ( 2 italic_M - 1 ) end_POSTSUPERSCRIPT be the embedding matrix, where r ^ D L i = f ( r U L i ) superscript subscript ^ r 𝐷 𝐿 𝑖 𝑓 superscript subscript r 𝑈 𝐿 𝑖 {\hat{\textbf{r}}_{DL}}^{i}=f({\textbf{r}_{UL}}^{i}) over^ start_ARG r end_ARG start_POSTSUBSCRIPT italic_D italic_L end_POSTSUBSCRIPT start_POSTSUPERSCRIPT italic_i end_POSTSUPERSCRIPT = italic_f ( r start_POSTSUBSCRIPT italic_U italic_L end_POSTSUBSCRIPT start_POSTSUPERSCRIPT italic_i end_POSTSUPERSCRIPT ) . Let R = [ ( r D L 1 ) T … ( r D L N ) T ] T ∈ ℝ N × ( 2 M − 1 ) R superscript delimited-[] superscript superscript subscript r 𝐷 𝐿 1 𝑇 … superscript superscript subscript r 𝐷 𝐿 𝑁 𝑇 𝑇 superscript ℝ 𝑁 2 𝑀 1 \textbf{R}=[\left({\textbf{r}_{DL}}^{1}\right)^{T}...\left({\textbf{r}_{DL}}^{% N}\right)^{T}]^{T}\in\mathbb{R}^{N\times(2M-1)} R = [ ( r start_POSTSUBSCRIPT italic_D italic_L end_POSTSUBSCRIPT start_POSTSUPERSCRIPT 1 end_POSTSUPERSCRIPT ) start_POSTSUPERSCRIPT italic_T end_POSTSUPERSCRIPT … ( r start_POSTSUBSCRIPT italic_D italic_L end_POSTSUBSCRIPT start_POSTSUPERSCRIPT italic_N end_POSTSUPERSCRIPT ) start_POSTSUPERSCRIPT italic_T end_POSTSUPERSCRIPT ] start_POSTSUPERSCRIPT italic_T end_POSTSUPERSCRIPT ∈ blackboard_R start_POSTSUPERSCRIPT italic_N × ( 2 italic_M - 1 ) end_POSTSUPERSCRIPT be the output training data matrix. R is the DL counterpart of X .
Our aim is to find a function f ( ⋅ ) 𝑓 ⋅ f(\cdot) italic_f ( ⋅ ) that approximates the training data sufficiently well, i.e., f ( r U L i ) = r ^ D L i ≈ r D L i 𝑓 superscript subscript r 𝑈 𝐿 𝑖 superscript subscript ^ r 𝐷 𝐿 𝑖 superscript subscript r 𝐷 𝐿 𝑖 f({\textbf{r}_{UL}}^{i})={\hat{\textbf{r}}_{DL}}^{i}\approx{\textbf{r}_{DL}}^{i} italic_f ( r start_POSTSUBSCRIPT italic_U italic_L end_POSTSUBSCRIPT start_POSTSUPERSCRIPT italic_i end_POSTSUPERSCRIPT ) = over^ start_ARG r end_ARG start_POSTSUBSCRIPT italic_D italic_L end_POSTSUBSCRIPT start_POSTSUPERSCRIPT italic_i end_POSTSUPERSCRIPT ≈ r start_POSTSUBSCRIPT italic_D italic_L end_POSTSUBSCRIPT start_POSTSUPERSCRIPT italic_i end_POSTSUPERSCRIPT , and preserves the nearest neighbors of each input vector in the embedding space, while mapping previously unseen UL CCMs (test data) to DL CCMs with low error. The interpolation problem can be formulated considering the following objectives.
Lipschitz regularity of the interpolation function: The interpolation function is of the form
(22) |
Here f ( ⋅ ) 𝑓 ⋅ f(\cdot) italic_f ( ⋅ ) is chosen as a radial basis function (RBF) interpolator due to its well-studied properties [ 28 ] . Specifically, the Gaussian RBF kernel is chosen for the extension of the embedding, where
(23) |
is the k t h superscript 𝑘 𝑡 ℎ k^{th} italic_k start_POSTSUPERSCRIPT italic_t italic_h end_POSTSUPERSCRIPT element of f ( r U L ) 𝑓 subscript r 𝑈 𝐿 f({\textbf{r}_{UL}}) italic_f ( r start_POSTSUBSCRIPT italic_U italic_L end_POSTSUBSCRIPT ) , for k ∈ { 1 , … , 2 M − 1 } 𝑘 1 … 2 𝑀 1 k\in\{1,...,2M-1\} italic_k ∈ { 1 , … , 2 italic_M - 1 } . C i k subscript 𝐶 𝑖 𝑘 C_{ik} italic_C start_POSTSUBSCRIPT italic_i italic_k end_POSTSUBSCRIPT are the interpolator coefficients, and σ 𝜎 \sigma italic_σ is the scale parameter of the Gaussian RBF kernel.
The Lipschitz constant of the Gaussian RBF interpolation function is provided in [ 27 ] as
(24) |
where C ∈ ℝ N × ( 2 M − 1 ) C superscript ℝ 𝑁 2 𝑀 1 \textbf{C}\in\mathbb{R}^{N\times(2M-1)} C ∈ blackboard_R start_POSTSUPERSCRIPT italic_N × ( 2 italic_M - 1 ) end_POSTSUPERSCRIPT is the matrix containing the interpolator coefficient C i k subscript 𝐶 𝑖 𝑘 C_{ik} italic_C start_POSTSUBSCRIPT italic_i italic_k end_POSTSUBSCRIPT in its ( i , k ) t h superscript 𝑖 𝑘 𝑡 ℎ (i,k)^{th} ( italic_i , italic_k ) start_POSTSUPERSCRIPT italic_t italic_h end_POSTSUPERSCRIPT element, i ∈ { 1 , … , N } 𝑖 1 … 𝑁 i\in\{1,...,N\} italic_i ∈ { 1 , … , italic_N } , k ∈ { 1 , … , 2 M − 1 } 𝑘 1 … 2 𝑀 1 k\in\{1,...,2M-1\} italic_k ∈ { 1 , … , 2 italic_M - 1 } . The matrix C is obtained as
(25) |
by learning a mapping R ^ ^ R \hat{\textbf{R}} over^ start_ARG R end_ARG from the training data matrix X , where 𝚿 ∈ ℝ N × N 𝚿 superscript ℝ 𝑁 𝑁 \boldsymbol{\Psi}\in\mathbb{R}^{N\times N} bold_Ψ ∈ blackboard_R start_POSTSUPERSCRIPT italic_N × italic_N end_POSTSUPERSCRIPT is the RBF kernel matrix, whose ( i , j ) t h superscript 𝑖 𝑗 𝑡 ℎ (i,j)^{th} ( italic_i , italic_j ) start_POSTSUPERSCRIPT italic_t italic_h end_POSTSUPERSCRIPT element is e − ‖ r U L i − r U L j ‖ 2 σ 2 superscript 𝑒 superscript norm superscript subscript r 𝑈 𝐿 𝑖 superscript subscript r 𝑈 𝐿 𝑗 2 superscript 𝜎 2 e^{-\frac{\|{\textbf{r}_{UL}}^{i}-{\textbf{r}_{UL}}^{j}\|^{2}}{\sigma^{2}}} italic_e start_POSTSUPERSCRIPT - divide start_ARG ∥ r start_POSTSUBSCRIPT italic_U italic_L end_POSTSUBSCRIPT start_POSTSUPERSCRIPT italic_i end_POSTSUPERSCRIPT - r start_POSTSUBSCRIPT italic_U italic_L end_POSTSUBSCRIPT start_POSTSUPERSCRIPT italic_j end_POSTSUPERSCRIPT ∥ start_POSTSUPERSCRIPT 2 end_POSTSUPERSCRIPT end_ARG start_ARG italic_σ start_POSTSUPERSCRIPT 2 end_POSTSUPERSCRIPT end_ARG end_POSTSUPERSCRIPT .
From Theorem 2 , the Lipschitz constant, L 𝐿 L italic_L , of the interpolator, f ( ⋅ ) 𝑓 ⋅ f(\cdot) italic_f ( ⋅ ) , should be small so as to reduce the error upper bound in ( 21 ), which leads to a good generalization of the embedding to the test data. With that in mind, according to ( 24 ), the following terms should be minimized while learning embedding coordinates and the function parameters of the RBF interpolator:
σ − 2 superscript 𝜎 2 \sigma^{-2} italic_σ start_POSTSUPERSCRIPT - 2 end_POSTSUPERSCRIPT
‖ C ‖ F 2 = ‖ 𝚿 − 1 R ^ ‖ F 2 = t r ( R ^ T 𝚿 − 2 R ^ ) superscript subscript norm C 𝐹 2 superscript subscript norm superscript 𝚿 1 ^ R 𝐹 2 𝑡 𝑟 superscript ^ R 𝑇 superscript 𝚿 2 ^ R \|\textbf{C}\|_{F}^{2}=\|\boldsymbol{\Psi}^{-1}\hat{\textbf{R}}\|_{F}^{2}=tr(% \hat{\textbf{R}}^{T}\boldsymbol{\Psi}^{-2}\hat{\textbf{R}}) ∥ C ∥ start_POSTSUBSCRIPT italic_F end_POSTSUBSCRIPT start_POSTSUPERSCRIPT 2 end_POSTSUPERSCRIPT = ∥ bold_Ψ start_POSTSUPERSCRIPT - 1 end_POSTSUPERSCRIPT over^ start_ARG R end_ARG ∥ start_POSTSUBSCRIPT italic_F end_POSTSUBSCRIPT start_POSTSUPERSCRIPT 2 end_POSTSUPERSCRIPT = italic_t italic_r ( over^ start_ARG R end_ARG start_POSTSUPERSCRIPT italic_T end_POSTSUPERSCRIPT bold_Ψ start_POSTSUPERSCRIPT - 2 end_POSTSUPERSCRIPT over^ start_ARG R end_ARG )
Preservation of the local geometry between the UL/DL CCM spaces: Due to the angular reciprocity, there is an inherent similarity between the UL CCM and DL CCM of the same user, even though there is no explicit function that relates one another. On the other hand, from Theorem 1 , one can see that with enough training data, the points in the UL space become so close that the distance between nearest neighbors is bounded proportionally to the distance between their corresponding points in the DL space. Therefore, in order to preserve the local geometry of UL CCMs in the embedding space, the following term should be minimized
(26) |
UL/DL CCM pairs in the training dataset: Since the task is to learn a function that maps UL CCMs to their corresponding DL CCMs, the UL-DL CCM pairs in the training dataset are also incorporated into our optimization problem. Instead of employing hard data fidelity constraints, in order to achieve better noise tolerance we prefer the quadratic penalty term given by
Overall problem: We finally combine the above terms to form our overall objective function as
(27) |
where μ 1 subscript 𝜇 1 \mu_{1} italic_μ start_POSTSUBSCRIPT 1 end_POSTSUBSCRIPT , μ 2 subscript 𝜇 2 \mu_{2} italic_μ start_POSTSUBSCRIPT 2 end_POSTSUBSCRIPT and μ 3 subscript 𝜇 3 \mu_{3} italic_μ start_POSTSUBSCRIPT 3 end_POSTSUBSCRIPT are positive weights to determine the relative importance of each term in the objective function.
V-B Solution of the Problem
The optimization problem defined above is not jointly convex in R ^ ^ R \hat{\textbf{R}} over^ start_ARG R end_ARG and σ 𝜎 \sigma italic_σ . We employ an alternating optimization method, where one of the parameters is fixed while the other one is optimized in an alternative fashion at each iteration. This alternation is continued until convergence or the maximum number of iterations is reached.
Optimization of R ^ ^ R \hat{\textbf{R}} over^ start_ARG R end_ARG : When σ 𝜎 \sigma italic_σ is fixed, the optimization problem in ( 27 ) becomes the following:
(28) |
This minimization problem is a quadratic and convex problem. The closed form solution of the problem in ( 28 ) is given by
(29) |
A subscript 𝜇 3 I \left(\textbf{A}+\mu_{3}\textbf{I}\right) ( A + italic_μ start_POSTSUBSCRIPT 3 end_POSTSUBSCRIPT I ) is always invertible.
Optimization of σ 𝜎 \sigma italic_σ : When R ^ ^ R \hat{\textbf{R}} over^ start_ARG R end_ARG is fixed, the optimization problem is the following:
(30) |
Although nonconvex, this problem involves the optimization of a single scalar variable σ 𝜎 \sigma italic_σ , which can be solved via an exhaustive search of σ 𝜎 \sigma italic_σ in a reasonable interval.
Our solution algorithm is summarized in Algorithm 1.
After learning the embedding matrix R ^ ^ R \hat{\textbf{R}} over^ start_ARG R end_ARG and the kernel scale parameter σ 𝜎 \sigma italic_σ with Algorithm 1, one can calculate the interpolator coefficient matrix C by using equation ( 25 ). Thus, using ( 22 ) and ( 23 ), one can estimate the DL CCM of a new test sample that is not in the training dataset by using its UL CCM.
The integral of PAS over all angles is known to be 1 1 1 1 ; however we have no constraints forcing such a normalization while learning the embedding and the kernel scale parameter. For this reason, once we obtain the estimate r ^ D L subscript ^ r 𝐷 𝐿 \hat{\textbf{r}}_{DL} over^ start_ARG r end_ARG start_POSTSUBSCRIPT italic_D italic_L end_POSTSUBSCRIPT , we normalize it such that its first entry becomes r ^ D L ( 1 ) = 1 subscript ^ r 𝐷 𝐿 1 1 \hat{\textbf{r}}_{DL}(1)=1 over^ start_ARG r end_ARG start_POSTSUBSCRIPT italic_D italic_L end_POSTSUBSCRIPT ( 1 ) = 1 .
V-C Complexity Analysis
The main factors that determine the complexity of our algorithm are the optimization problems given in ( 28 ) and ( 30 ), which are solved in an alternating fashion. The complexity of constructing the matrices L and 𝚿 𝚿 \boldsymbol{\Psi} bold_Ψ is 𝒪 ( M N 2 ) 𝒪 𝑀 superscript 𝑁 2 \mathcal{O}(MN^{2}) caligraphic_O ( italic_M italic_N start_POSTSUPERSCRIPT 2 end_POSTSUPERSCRIPT ) , where N 𝑁 N italic_N is the number of training data in the dataset. The matrix inversion operations in ( 29 ) and ( 30 ) are of complexity 𝒪 ( N 3 ) 𝒪 superscript 𝑁 3 \mathcal{O}(N^{3}) caligraphic_O ( italic_N start_POSTSUPERSCRIPT 3 end_POSTSUPERSCRIPT ) , which is the determining part of the complexity analysis, in a typical scenario where M < N 𝑀 𝑁 M<N italic_M < italic_N . Hence, the overall complexity of our algorithm is 𝒪 ( N 3 ) 𝒪 superscript 𝑁 3 \mathcal{O}(N^{3}) caligraphic_O ( italic_N start_POSTSUPERSCRIPT 3 end_POSTSUPERSCRIPT ) .
After the training is completed, the Gaussian RBF interpolation function can be directly used to find the DL CCMs of new data. The complexity of finding an estimate of the DL CCM using our function is 𝒪 ( M 2 N ) 𝒪 superscript 𝑀 2 𝑁 \mathcal{O}(M^{2}N) caligraphic_O ( italic_M start_POSTSUPERSCRIPT 2 end_POSTSUPERSCRIPT italic_N ) since for each element of the mapping vector of size ( 2 M − 1 ) 2 𝑀 1 \left(2M-1\right) ( 2 italic_M - 1 ) , ( 2 M − 1 ) 2 𝑀 1 \left(2M-1\right) ( 2 italic_M - 1 ) -dimensional vectors are used for calculation at N 𝑁 N italic_N center locations. [ 30 ] .
VI Simulations
In this section, we evaluate the performance of our algorithm with simulations, based on the simulation setup reported in Table I . We first observe the behavior of the objective function and that of the estimation performance of our method throughout the iterations. Next, we conduct tests to study how the performance of our method varies with algorithm hyperparameters. Finally, we compare the performance of our method to that of some baseline methods in the literature.
Carrier Frequencies | GHz, GHz |
---|---|
Base Station Antenna Number (M) | One of the following: |
Dataset Size (Train and Test) | |
Train/Test Data Ratio | |
, , | |
SNR | 20 dB |
Users are considered to have uniform PAS with mean AoAs uniformly distributed in [ − π , π ] 𝜋 𝜋 [-\pi,\pi] [ - italic_π , italic_π ] . The spread of AoAs of users are drawn from [ 5 ° , 15 ° ] 5 ° 15 ° [5\degree,15\degree] [ 5 ° , 15 ° ] uniformly. The carrier frequencies of uplink and downlink channels in Table I are chosen according to [ 31 ] .
Let us denote the true value of a DL CCM by R D L subscript R 𝐷 𝐿 \textbf{R}_{DL} R start_POSTSUBSCRIPT italic_D italic_L end_POSTSUBSCRIPT and its estimate by R ^ D L subscript ^ R 𝐷 𝐿 \hat{\textbf{R}}_{DL} over^ start_ARG R end_ARG start_POSTSUBSCRIPT italic_D italic_L end_POSTSUBSCRIPT . The following three error metrics are used to compare the performance of the proposed algorithm with benchmark methods:
Normalized Mean Square Error (NMSE): NMSE is used to measure the average error in each entry of a CCM, which is defined as
(31) |
Correlation Matrix Distance (CMD): This metric defined in [ 32 ] is used to quantify the deviation between the direction of the true DL CCM and that of its estimate. The CMD is given by
(32) |
Deviation Metric (DM): In [ 14 ] , the following deviation metric is used to measure the deviation in the principal eigenvector of the estimated DL CCM, which is useful in beamforming applications:
(33) |
where Γ m a x subscript Γ 𝑚 𝑎 𝑥 \Gamma_{max} roman_Γ start_POSTSUBSCRIPT italic_m italic_a italic_x end_POSTSUBSCRIPT is the largest eigenvalue of R D L subscript R 𝐷 𝐿 \textbf{R}_{DL} R start_POSTSUBSCRIPT italic_D italic_L end_POSTSUBSCRIPT and v is the eigenvector corresponding to the largest eigenvalue of R ^ D L subscript ^ R 𝐷 𝐿 \hat{\textbf{R}}_{DL} over^ start_ARG R end_ARG start_POSTSUBSCRIPT italic_D italic_L end_POSTSUBSCRIPT .
VI-A Simulation Setup
The dataset is constructed similarly to the setting in [ 14 ] as described below. The following steps are followed for all UL CCMs in the dataset and for DL CCMs in the training dataset. DL CCMs in the test set are constructed via only Step 1, so that they form an ideal ground truth data set for performance comparisons of our algorithm with the benchmark methods.
CCMs are calculated using the formula in ( 3 ).
Using the generated CCMs, UL and DL channel realizations are constructed as follows:
(34) |
where ( w x k ) c ∼ 𝒞 𝒩 ( 0 , I ) similar-to superscript superscript subscript w 𝑥 𝑘 𝑐 𝒞 𝒩 0 I \left(\textbf{w}_{x}^{k}\right)^{c}\sim\mathcal{CN}(\textbf{0},\,\textbf{I}) ( w start_POSTSUBSCRIPT italic_x end_POSTSUBSCRIPT start_POSTSUPERSCRIPT italic_k end_POSTSUPERSCRIPT ) start_POSTSUPERSCRIPT italic_c end_POSTSUPERSCRIPT ∼ caligraphic_C caligraphic_N ( 0 , I ) , R x k superscript subscript R 𝑥 𝑘 \textbf{R}_{x}^{k} R start_POSTSUBSCRIPT italic_x end_POSTSUBSCRIPT start_POSTSUPERSCRIPT italic_k end_POSTSUPERSCRIPT is the CCM of user k 𝑘 k italic_k (either UL or DL, specified by x 𝑥 x italic_x ) and N c h subscript 𝑁 𝑐 ℎ N_{ch} italic_N start_POSTSUBSCRIPT italic_c italic_h end_POSTSUBSCRIPT is the number of channel realizations. N c h subscript 𝑁 𝑐 ℎ N_{ch} italic_N start_POSTSUBSCRIPT italic_c italic_h end_POSTSUBSCRIPT is taken as 2 M 2 𝑀 2M 2 italic_M in the simulations.
The noisy channel estimates obtained after the training phase with pilot signals are modeled and generated as follows:
(35) |
where ( n x k ) c ∼ 𝒞 𝒩 ( 0 , σ 2 I ) similar-to superscript superscript subscript n 𝑥 𝑘 𝑐 𝒞 𝒩 0 superscript 𝜎 2 I \left(\textbf{n}_{x}^{k}\right)^{c}\sim\mathcal{CN}(\textbf{0},\,\sigma^{2}% \textbf{I}) ( n start_POSTSUBSCRIPT italic_x end_POSTSUBSCRIPT start_POSTSUPERSCRIPT italic_k end_POSTSUPERSCRIPT ) start_POSTSUPERSCRIPT italic_c end_POSTSUPERSCRIPT ∼ caligraphic_C caligraphic_N ( 0 , italic_σ start_POSTSUPERSCRIPT 2 end_POSTSUPERSCRIPT I ) and ( h ^ x k ) c superscript superscript subscript ^ h 𝑥 𝑘 𝑐 \left(\hat{\textbf{h}}_{x}^{k}\right)^{c} ( over^ start_ARG h end_ARG start_POSTSUBSCRIPT italic_x end_POSTSUBSCRIPT start_POSTSUPERSCRIPT italic_k end_POSTSUPERSCRIPT ) start_POSTSUPERSCRIPT italic_c end_POSTSUPERSCRIPT is the noisy channel estimate of the c t h superscript 𝑐 𝑡 ℎ c^{th} italic_c start_POSTSUPERSCRIPT italic_t italic_h end_POSTSUPERSCRIPT channel realization. The signal-to-noise ratio (SNR) for this pilot signaling setup is taken to be the same as in [ 14 ] , which is t r ( R U L k ) σ 2 = 20 𝑡 𝑟 superscript subscript R 𝑈 𝐿 𝑘 superscript 𝜎 2 20 \frac{tr(\textbf{R}_{UL}^{k})}{\sigma^{2}}=20 divide start_ARG italic_t italic_r ( R start_POSTSUBSCRIPT italic_U italic_L end_POSTSUBSCRIPT start_POSTSUPERSCRIPT italic_k end_POSTSUPERSCRIPT ) end_ARG start_ARG italic_σ start_POSTSUPERSCRIPT 2 end_POSTSUPERSCRIPT end_ARG = 20 dB, unless it is explicitly said to be taken differently.
The sample covariance for user k 𝑘 k italic_k is then given by
(36) |
Due to the ULA antenna structure at the BS and the WSSUS model, the CCMs are Toeplitz, Hermitian and PSD, which is used for the correction of the sample covariance found in ( 36 ). The projection of the sample covariance onto the set of Toeplitz, Hermitian and PSD matrices is done by the alternative projection method proposed in [ 33 ] . The projection method solves the following optimization problem:
| (37) |
𝑀 T_{+}^{M} italic_T start_POSTSUBSCRIPT + end_POSTSUBSCRIPT start_POSTSUPERSCRIPT italic_M end_POSTSUPERSCRIPT is the set of M × M 𝑀 𝑀 M\times M italic_M × italic_M Toeplitz, Hermitian and PSD matrices.
The matrices estimated in the previous step are normalized so that their ( 1 , 1 ) t h superscript 1 1 𝑡 ℎ (1,1)^{th} ( 1 , 1 ) start_POSTSUPERSCRIPT italic_t italic_h end_POSTSUPERSCRIPT element is 1. This is done due to the fact that the PAS of the CCMs are normalized to 1.
VI-B Stability and Sensitivity Analysis
First, we study the change in the objective function and the change in the average NMSE of DL CCMs learned by our algorithm throughout the iterations. For M = 64 𝑀 64 M=64 italic_M = 64 base station antennas, we repeat the experiments for 25 i.i.d. datasets. The average objective function and error values are presented in Figure 1 . From Figure 1 , one can see that the objective function decreases throughout the iterations, which is expected because the algorithm updates both the embedding and the kernel scale parameter in such a way that the objective function never increases. The average NMSE, CMD and DM follow a similar trend to decrease as the objective function, which suggests that our proposed objective function well captures the performance goal of our algorithm.

Table III reports the performance for different weight combinations for the Lipschitz continuity of the interpolator and the data fidelity. The ratio between μ 1 subscript 𝜇 1 \mu_{1} italic_μ start_POSTSUBSCRIPT 1 end_POSTSUBSCRIPT and μ 2 subscript 𝜇 2 \mu_{2} italic_μ start_POSTSUBSCRIPT 2 end_POSTSUBSCRIPT is fixed to a suitable number chosen based on Table II . Looking at Table III , one can see that as μ 3 subscript 𝜇 3 \mu_{3} italic_μ start_POSTSUBSCRIPT 3 end_POSTSUBSCRIPT gets smaller, the average NMSE increases drastically. However, it also shows that μ 1 subscript 𝜇 1 \mu_{1} italic_μ start_POSTSUBSCRIPT 1 end_POSTSUBSCRIPT (and also μ 2 subscript 𝜇 2 \mu_{2} italic_μ start_POSTSUBSCRIPT 2 end_POSTSUBSCRIPT ) should be chosen as positive numbers to improve the performance. The performance seems to be more sensitive to the data fidelity term than the Lipschitz continuity terms.
μ 2 subscript 𝜇 2 \mu_{2} italic_μ start_POSTSUBSCRIPT 2 end_POSTSUBSCRIPT μ 1 subscript 𝜇 1 \mu_{1} italic_μ start_POSTSUBSCRIPT 1 end_POSTSUBSCRIPT 0 0 10 − 4 superscript 10 4 10^{-4} 10 start_POSTSUPERSCRIPT - 4 end_POSTSUPERSCRIPT 10 − 3 superscript 10 3 10^{-3} 10 start_POSTSUPERSCRIPT - 3 end_POSTSUPERSCRIPT 10 − 2 superscript 10 2 10^{-2} 10 start_POSTSUPERSCRIPT - 2 end_POSTSUPERSCRIPT 10 − 1 superscript 10 1 10^{-1} 10 start_POSTSUPERSCRIPT - 1 end_POSTSUPERSCRIPT 1 1 1 1 10 1 superscript 10 1 10^{1} 10 start_POSTSUPERSCRIPT 1 end_POSTSUPERSCRIPT 10 2 superscript 10 2 10^{2} 10 start_POSTSUPERSCRIPT 2 end_POSTSUPERSCRIPT 0 0 0.0463 0.0390 0.0390 0.0389 0.0376 0.0345 0.0309 0.0402 3 × 10 − 1 3 superscript 10 1 3\times 10^{-1} 3 × 10 start_POSTSUPERSCRIPT - 1 end_POSTSUPERSCRIPT 0.0463 0.0348 0.0378 0.0387 0.0376 0.0345 0.0309 0.0402 3 × 10 1 3 superscript 10 1 3\times 10^{1} 3 × 10 start_POSTSUPERSCRIPT 1 end_POSTSUPERSCRIPT 0.0463 0.0313 0.0320 0.0344 0.0361 0.0343 0.0309 0.0402 3 × 10 3 3 superscript 10 3 3\times 10^{3} 3 × 10 start_POSTSUPERSCRIPT 3 end_POSTSUPERSCRIPT 0.0463 0.0349 0.0325 0.0307 0.0297 0.0298 0.0300 0.0403 3 × 10 5 3 superscript 10 5 3\times 10^{5} 3 × 10 start_POSTSUPERSCRIPT 5 end_POSTSUPERSCRIPT 0.0463 0.0265 0.0221 0.0194 0.0201 0.0238 0.0319 0.0452 3 × 10 7 3 superscript 10 7 3\times 10^{7} 3 × 10 start_POSTSUPERSCRIPT 7 end_POSTSUPERSCRIPT 0.0463 0.0265 0.0221 0.0194 0.0208 0.0313 0.0540 0.0796 3 × 10 9 3 superscript 10 9 3\times 10^{9} 3 × 10 start_POSTSUPERSCRIPT 9 end_POSTSUPERSCRIPT 0.0463 0.0265 0.0221 0.0194 0.0208 0.0313 0.0540 0.1022 3 × 10 11 3 superscript 10 11 3\times 10^{11} 3 × 10 start_POSTSUPERSCRIPT 11 end_POSTSUPERSCRIPT 0.0463 0.0265 0.0221 0.0194 0.0208 0.0313 0.0540 0.1022
μ 3 subscript 𝜇 3 \mu_{3} italic_μ start_POSTSUBSCRIPT 3 end_POSTSUBSCRIPT μ 1 subscript 𝜇 1 \mu_{1} italic_μ start_POSTSUBSCRIPT 1 end_POSTSUBSCRIPT 0 0 10 − 4 superscript 10 4 10^{-4} 10 start_POSTSUPERSCRIPT - 4 end_POSTSUPERSCRIPT 10 − 3 superscript 10 3 10^{-3} 10 start_POSTSUPERSCRIPT - 3 end_POSTSUPERSCRIPT 10 − 2 superscript 10 2 10^{-2} 10 start_POSTSUPERSCRIPT - 2 end_POSTSUPERSCRIPT 10 − 1 superscript 10 1 10^{-1} 10 start_POSTSUPERSCRIPT - 1 end_POSTSUPERSCRIPT 1 1 1 1 10 1 superscript 10 1 10^{1} 10 start_POSTSUPERSCRIPT 1 end_POSTSUPERSCRIPT 10 2 superscript 10 2 10^{2} 10 start_POSTSUPERSCRIPT 2 end_POSTSUPERSCRIPT 10 − 1 superscript 10 1 10^{-1} 10 start_POSTSUPERSCRIPT - 1 end_POSTSUPERSCRIPT 0.2093 0.2248 0.2448 0.2550 0.2739 0.3643 0.6225 0.8106 1 1 1 1 0.0722 0.0704 0.0682 0.0730 0.0858 0.1252 0.2766 0.6016 10 1 superscript 10 1 10^{1} 10 start_POSTSUPERSCRIPT 1 end_POSTSUPERSCRIPT 0.0357 0.0315 0.0277 0.0241 0.0347 0.0566 0.1043 0.2657 10 2 superscript 10 2 10^{2} 10 start_POSTSUPERSCRIPT 2 end_POSTSUPERSCRIPT 0.0463 0.0324 0.0325 0.0275 0.0201 0.0311 0.0540 0.1022 10 3 superscript 10 3 10^{3} 10 start_POSTSUPERSCRIPT 3 end_POSTSUPERSCRIPT 0.0496 0.0330 0.0331 0.0335 0.0283 0.0199 0.0308 0.0538 10 4 superscript 10 4 10^{4} 10 start_POSTSUPERSCRIPT 4 end_POSTSUPERSCRIPT 0.0500 0.0331 0.0331 0.0332 0.0336 0.0284 0.0198 0.0307 10 5 superscript 10 5 10^{5} 10 start_POSTSUPERSCRIPT 5 end_POSTSUPERSCRIPT 0.0500 0.0331 0.0331 0.0331 0.0332 0.0336 0.0284 0.0198

VI-C Algorithm Performance
In this section, we compare the average errors of our method to those of the following three benchmark methods: (1) The dictionary based method in [ 10 ] , (2) the sinc transformation-based method in [ 14 ] , (3) the CGAN-based method in [ 13 ] . We conduct three different experiments. First, we calculate the DL CCM estimation errors with a perfect dataset to study the performances of the compared methods. Then, we calculate the DL CCM estimation errors for different SNR values. Finally, we compare the error values of the algorithms for different numbers of base station antennas, M 𝑀 M italic_M .
In Figure 2 , we compare the performances of all benchmark methods for M = 256 𝑀 256 M=256 italic_M = 256 base station antennas where the CCMs in both the training and the test datasets are perfectly known. The results are averaged over 10 i.i.d. datasets. For this experiment, the hyperparameters are taken as μ 1 = 10 , μ 2 = 3 × 10 8 formulae-sequence subscript 𝜇 1 10 subscript 𝜇 2 3 superscript 10 8 \mu_{1}=10,\ \mu_{2}=3\times 10^{8} italic_μ start_POSTSUBSCRIPT 1 end_POSTSUBSCRIPT = 10 , italic_μ start_POSTSUBSCRIPT 2 end_POSTSUBSCRIPT = 3 × 10 start_POSTSUPERSCRIPT 8 end_POSTSUPERSCRIPT and μ 3 = 10 7 subscript 𝜇 3 superscript 10 7 \mu_{3}=10^{7} italic_μ start_POSTSUBSCRIPT 3 end_POSTSUBSCRIPT = 10 start_POSTSUPERSCRIPT 7 end_POSTSUPERSCRIPT . One can see from Figure 2 that our algorithm mostly outperforms the dictionary based method and the sinc transformation method, while the CGAN-based method has relatively higher error values than the other methods. In particular, our method yields the smallest average NMSE value of 6.1 × 10 − 3 6.1 superscript 10 3 6.1\times 10^{-3} 6.1 × 10 start_POSTSUPERSCRIPT - 3 end_POSTSUPERSCRIPT among all methods, while its closest competitor algorithms dictionary and sinc transformation methods result in average NMSE values of 0.0324 0.0324 0.0324 0.0324 and 0.0112 0.0112 0.0112 0.0112 , respectively. On the other hand, the average NMSE of the CGAN based method for this setup is 0.0761 0.0761 0.0761 0.0761 . One can interpret this finding as follows: Even though deep learning based methods can learn highly complex functions quite well, they need a large amount of data to achieve this. In settings with a limited availability of training data, such methods may fail to learn a network that can generalize to new data well. Considering also the long training processes, in the rest of our experiments we compare our algorithm with the dictionary based method and the sinc transformation method, since they are closer to our method in terms of performance.

Figure 3 demonstrates the average error values of each algorithm for the base station antenna numbers of M ∈ { 32 , 64 , 128 , 256 } 𝑀 32 64 128 256 M\in\{32,64,128,256\} italic_M ∈ { 32 , 64 , 128 , 256 } . For 25 25 25 25 i.i.d. datasets, the experiments are repeated and the average errors are presented in Figure 3 . One can see that the proposed algorithm surpasses the performance of dictionary-based method for each error metric for all antenna numbers. However, the sinc transformation method has better average error performance than our method for high number of antennas, for example M = 256 𝑀 256 M=256 italic_M = 256 antennas. This result is expected, since the methods that rely on training data, i.e., the dictionary method and our algorithm, both try to estimate more matrix parameters with the same dataset size, which gets more difficult as the number of base station antennas increases. On the other hand, the sinc transformation method has an error upper bound that decreases with the antenna number, M 𝑀 M italic_M , which is presented in [ 14 ] . Even though the average error of the sinc transformation method is lower than that of our method for M = 256 𝑀 256 M=256 italic_M = 256 antennas, we have observed the standard deviations of the NMSE values for our method, dictionary method and the sinc transformation method to be 0.0161, 0.0377 and 0.0343, respectively. One can deduce from these results that even though our algorithm may yield higher average error than the sinc transformation method at a high number of antennas, its error performance is more stable than that of the sinc transformation method, i.e., it is less likely to encounter outliers with significantly high error values.
Figure 4 shows the performances of the algorithms when the base station has M = 64 𝑀 64 M=64 italic_M = 64 antennas. The experiments are repeated for 25 i.i.d. datasets. In this scenario, the CCMs have been constructed for several different SNR values ranging from 0 dB 0 dB 0\ \text{dB} 0 dB to 40 dB 40 dB 40\ \text{dB} 40 dB and the effect of the SNR on the performance is observed. One can see that all algorithms yield high estimation error at 0 dB SNR as expected, where the CCMs are corrupted with severe noise. As the SNR increases, the estimates obtained from each algorithm improves and our algorithm outperforms the benchmark methods in all performance metrics.

VII Conclusion
In this paper, we have proposed a novel DL CCM estimation method for FDD massive MIMO systems where the base station is equipped with ULA antennas. We have first presented a theoretical analysis that gives an upper bound on the estimation error of the DL CCM from UL CCMs. We have then proposed a representation learning-based method in order to learn a mapping function from UL CCMs to their DL CCM counterparts. The proposed method aims at learning an interpolation function from datasets relatively smaller than those needed for training deep neural networks, while capturing the richness of nonlinear learning methods so that the learned mapping is more robust to nonlinearities/discrepancies in the system parameters than simple signal processing based methods. The experimental results show that the proposed algorithm achieves better estimation performance than the benchmark methods in most of the scenarios. The proposed method can especially be useful in practical applications with limited access to training data. Our algorithm shows promising performance in such applications as it provides quite accurate downlink channel covariance estimates with a simple nonlinear learning setup. The extension of our method to other base station antenna structures, such as a uniform rectangular array (URA) is left as a future research direction.
Acknowledgments
The authors would like to thank Dr. -Ing. Bitan Banerjee for offering his help for the implementation of the CGAN-based benchmark method in [ 13 ] .
Appendix A Approximations Used in the Proof of Theorem 1
Based on the first order Taylor approximations given in ( 8 ) and ( 11 ), one can make the following approximations, which are useful for the proof of Theorem 1 :
¯ subscript 𝑣 𝑖 ¯ subscript 𝑣 𝑗 2 Δ \frac{\sin(\bar{v_{i}}+\Delta)+\sin(\bar{v_{j}}+\Delta)}{2}\approx\sin\left(% \frac{\bar{v_{i}}+\bar{v_{j}}}{2}+\Delta\right) divide start_ARG roman_sin ( over¯ start_ARG italic_v start_POSTSUBSCRIPT italic_i end_POSTSUBSCRIPT end_ARG + roman_Δ ) + roman_sin ( over¯ start_ARG italic_v start_POSTSUBSCRIPT italic_j end_POSTSUBSCRIPT end_ARG + roman_Δ ) end_ARG start_ARG 2 end_ARG ≈ roman_sin ( divide start_ARG over¯ start_ARG italic_v start_POSTSUBSCRIPT italic_i end_POSTSUBSCRIPT end_ARG + over¯ start_ARG italic_v start_POSTSUBSCRIPT italic_j end_POSTSUBSCRIPT end_ARG end_ARG start_ARG 2 end_ARG + roman_Δ )
¯ subscript 𝑣 𝑖 ¯ subscript 𝑣 𝑗 2 Δ \frac{\sin(\bar{v_{i}}-\Delta)+\sin(\bar{v_{j}}-\Delta)}{2}\approx\sin\left(% \frac{\bar{v_{i}}+\bar{v_{j}}}{2}-\Delta\right) divide start_ARG roman_sin ( over¯ start_ARG italic_v start_POSTSUBSCRIPT italic_i end_POSTSUBSCRIPT end_ARG - roman_Δ ) + roman_sin ( over¯ start_ARG italic_v start_POSTSUBSCRIPT italic_j end_POSTSUBSCRIPT end_ARG - roman_Δ ) end_ARG start_ARG 2 end_ARG ≈ roman_sin ( divide start_ARG over¯ start_ARG italic_v start_POSTSUBSCRIPT italic_i end_POSTSUBSCRIPT end_ARG + over¯ start_ARG italic_v start_POSTSUBSCRIPT italic_j end_POSTSUBSCRIPT end_ARG end_ARG start_ARG 2 end_ARG - roman_Δ )
¯ subscript 𝑣 𝑗 Δ subscript 𝛼 1 ¯ subscript 𝑣 𝑖 ¯ subscript 𝑣 𝑗 \pi\left(\sin(\bar{v_{i}}+\Delta)-\sin(\bar{v_{j}}+\Delta)\right)\approx\alpha% _{1}(\bar{v_{i}}-\bar{v_{j}}) italic_π ( roman_sin ( over¯ start_ARG italic_v start_POSTSUBSCRIPT italic_i end_POSTSUBSCRIPT end_ARG + roman_Δ ) - roman_sin ( over¯ start_ARG italic_v start_POSTSUBSCRIPT italic_j end_POSTSUBSCRIPT end_ARG + roman_Δ ) ) ≈ italic_α start_POSTSUBSCRIPT 1 end_POSTSUBSCRIPT ( over¯ start_ARG italic_v start_POSTSUBSCRIPT italic_i end_POSTSUBSCRIPT end_ARG - over¯ start_ARG italic_v start_POSTSUBSCRIPT italic_j end_POSTSUBSCRIPT end_ARG )
π ( sin ( v i ¯ − Δ ) − sin ( v j ¯ − Δ ) ) ≈ α 2 ( v i ¯ − v j ¯ ) 𝜋 ¯ subscript 𝑣 𝑖 Δ ¯ subscript 𝑣 𝑗 Δ subscript 𝛼 2 ¯ subscript 𝑣 𝑖 ¯ subscript 𝑣 𝑗 \pi\left(\sin(\bar{v_{i}}-\Delta)-\sin(\bar{v_{j}}-\Delta)\right)\approx\alpha% _{2}(\bar{v_{i}}-\bar{v_{j}}) italic_π ( roman_sin ( over¯ start_ARG italic_v start_POSTSUBSCRIPT italic_i end_POSTSUBSCRIPT end_ARG - roman_Δ ) - roman_sin ( over¯ start_ARG italic_v start_POSTSUBSCRIPT italic_j end_POSTSUBSCRIPT end_ARG - roman_Δ ) ) ≈ italic_α start_POSTSUBSCRIPT 2 end_POSTSUBSCRIPT ( over¯ start_ARG italic_v start_POSTSUBSCRIPT italic_i end_POSTSUBSCRIPT end_ARG - over¯ start_ARG italic_v start_POSTSUBSCRIPT italic_j end_POSTSUBSCRIPT end_ARG )
Appendix B Proof of Theorem 2
The norm of the difference between an arbitrary test point in the DL CCM dataset and its estimate obtained by the mapping of its UL counterpart via the interpolation function f ( . ) f\left(.\right) italic_f ( . ) can be bounded as the following:
Let us name ‖ f ( r U L t e s t ) − 1 | A U L | ∑ r U L i ∈ A U L f ( r U L i ) ‖ norm 𝑓 superscript subscript r 𝑈 𝐿 𝑡 𝑒 𝑠 𝑡 1 superscript 𝐴 𝑈 𝐿 subscript superscript subscript r 𝑈 𝐿 𝑖 superscript 𝐴 𝑈 𝐿 𝑓 superscript subscript r 𝑈 𝐿 𝑖 \left\|f({\textbf{r}_{UL}}^{test})-\frac{1}{|A^{UL}|}\sum_{{{\textbf{r}_{UL}}^% {i}}\in A^{UL}}f({\textbf{r}_{UL}}^{i})\right\| ∥ italic_f ( r start_POSTSUBSCRIPT italic_U italic_L end_POSTSUBSCRIPT start_POSTSUPERSCRIPT italic_t italic_e italic_s italic_t end_POSTSUPERSCRIPT ) - divide start_ARG 1 end_ARG start_ARG | italic_A start_POSTSUPERSCRIPT italic_U italic_L end_POSTSUPERSCRIPT | end_ARG ∑ start_POSTSUBSCRIPT r start_POSTSUBSCRIPT italic_U italic_L end_POSTSUBSCRIPT start_POSTSUPERSCRIPT italic_i end_POSTSUPERSCRIPT ∈ italic_A start_POSTSUPERSCRIPT italic_U italic_L end_POSTSUPERSCRIPT end_POSTSUBSCRIPT italic_f ( r start_POSTSUBSCRIPT italic_U italic_L end_POSTSUBSCRIPT start_POSTSUPERSCRIPT italic_i end_POSTSUPERSCRIPT ) ∥ as (UB-1) , ‖ r D L t e s t − 1 | A U L | ∑ i : r U L i ∈ A U L r D L i ‖ norm superscript subscript r 𝐷 𝐿 𝑡 𝑒 𝑠 𝑡 1 superscript 𝐴 𝑈 𝐿 subscript : 𝑖 superscript subscript r 𝑈 𝐿 𝑖 superscript 𝐴 𝑈 𝐿 superscript subscript r 𝐷 𝐿 𝑖 \left\|{{\textbf{r}}_{DL}}^{test}-\frac{1}{|A^{UL}|}\sum_{i:{{\textbf{r}_{UL}}% ^{i}}\in A^{UL}}{\textbf{r}_{DL}}^{i}\right\| ∥ r start_POSTSUBSCRIPT italic_D italic_L end_POSTSUBSCRIPT start_POSTSUPERSCRIPT italic_t italic_e italic_s italic_t end_POSTSUPERSCRIPT - divide start_ARG 1 end_ARG start_ARG | italic_A start_POSTSUPERSCRIPT italic_U italic_L end_POSTSUPERSCRIPT | end_ARG ∑ start_POSTSUBSCRIPT italic_i : r start_POSTSUBSCRIPT italic_U italic_L end_POSTSUBSCRIPT start_POSTSUPERSCRIPT italic_i end_POSTSUPERSCRIPT ∈ italic_A start_POSTSUPERSCRIPT italic_U italic_L end_POSTSUPERSCRIPT end_POSTSUBSCRIPT r start_POSTSUBSCRIPT italic_D italic_L end_POSTSUBSCRIPT start_POSTSUPERSCRIPT italic_i end_POSTSUPERSCRIPT ∥ as (UB-2) and 1 | A U L | ∑ i : r U L i ∈ A U L ‖ r D L i − f ( r U L i ) ‖ 1 superscript 𝐴 𝑈 𝐿 subscript : 𝑖 superscript subscript r 𝑈 𝐿 𝑖 superscript 𝐴 𝑈 𝐿 norm superscript subscript r 𝐷 𝐿 𝑖 𝑓 superscript subscript r 𝑈 𝐿 𝑖 \frac{1}{|A^{UL}|}\sum_{i:{{\textbf{r}_{UL}}^{i}}\in A^{UL}}\left\|{\textbf{r}% _{DL}}^{i}-f({\textbf{r}_{UL}}^{i})\right\| divide start_ARG 1 end_ARG start_ARG | italic_A start_POSTSUPERSCRIPT italic_U italic_L end_POSTSUPERSCRIPT | end_ARG ∑ start_POSTSUBSCRIPT italic_i : r start_POSTSUBSCRIPT italic_U italic_L end_POSTSUBSCRIPT start_POSTSUPERSCRIPT italic_i end_POSTSUPERSCRIPT ∈ italic_A start_POSTSUPERSCRIPT italic_U italic_L end_POSTSUPERSCRIPT end_POSTSUBSCRIPT ∥ r start_POSTSUBSCRIPT italic_D italic_L end_POSTSUBSCRIPT start_POSTSUPERSCRIPT italic_i end_POSTSUPERSCRIPT - italic_f ( r start_POSTSUBSCRIPT italic_U italic_L end_POSTSUBSCRIPT start_POSTSUPERSCRIPT italic_i end_POSTSUPERSCRIPT ) ∥ as (UB-3).
(UB-1) can be upper bounded by using Lemma 1 , which is the adaptation of Lemma 1 in [ 34 ] to our study. The proof of Lemma 1 is presented in Appendix C .
Let the training sample set contain at least N 𝑁 N italic_N training samples { r U L i } i = 1 N superscript subscript superscript subscript r 𝑈 𝐿 𝑖 𝑖 1 𝑁 \{{\textbf{r}_{UL}}^{i}\}_{i=1}^{N} { r start_POSTSUBSCRIPT italic_U italic_L end_POSTSUBSCRIPT start_POSTSUPERSCRIPT italic_i end_POSTSUPERSCRIPT } start_POSTSUBSCRIPT italic_i = 1 end_POSTSUBSCRIPT start_POSTSUPERSCRIPT italic_N end_POSTSUPERSCRIPT with r U L i ∼ υ similar-to superscript subscript r 𝑈 𝐿 𝑖 𝜐 {\textbf{r}_{UL}}^{i}\sim\upsilon r start_POSTSUBSCRIPT italic_U italic_L end_POSTSUBSCRIPT start_POSTSUPERSCRIPT italic_i end_POSTSUPERSCRIPT ∼ italic_υ . Assume that the interpolation function f : ℝ 1 × 2 M − 1 → ℝ 1 × 2 M − 1 : 𝑓 → superscript ℝ 1 2 𝑀 1 superscript ℝ 1 2 𝑀 1 f:\mathbb{R}^{1\times 2M-1}\rightarrow\mathbb{R}^{1\times 2M-1} italic_f : blackboard_R start_POSTSUPERSCRIPT 1 × 2 italic_M - 1 end_POSTSUPERSCRIPT → blackboard_R start_POSTSUPERSCRIPT 1 × 2 italic_M - 1 end_POSTSUPERSCRIPT is Lipschitz continuous with constant L.
Let r U L t e s t superscript subscript r 𝑈 𝐿 𝑡 𝑒 𝑠 𝑡 {{\textbf{r}}_{UL}}^{test} r start_POSTSUBSCRIPT italic_U italic_L end_POSTSUBSCRIPT start_POSTSUPERSCRIPT italic_t italic_e italic_s italic_t end_POSTSUPERSCRIPT be a test sample drawn from υ 𝜐 \upsilon italic_υ independently of the training samples. Let A U L superscript 𝐴 𝑈 𝐿 A^{UL} italic_A start_POSTSUPERSCRIPT italic_U italic_L end_POSTSUPERSCRIPT be defined as in ( 5 ).
Then, for any ϵ > 0 italic-ϵ 0 \epsilon>0 italic_ϵ > 0 , for some 1 N η δ ≤ a < 1 1 𝑁 subscript 𝜂 𝛿 𝑎 1 \frac{1}{N\eta_{\delta}}\leq a<1 divide start_ARG 1 end_ARG start_ARG italic_N italic_η start_POSTSUBSCRIPT italic_δ end_POSTSUBSCRIPT end_ARG ≤ italic_a < 1 and δ > 0 𝛿 0 \delta>0 italic_δ > 0 , with probability at least
the set A U L superscript 𝐴 𝑈 𝐿 A^{UL} italic_A start_POSTSUPERSCRIPT italic_U italic_L end_POSTSUPERSCRIPT contains at least a N η δ 𝑎 𝑁 subscript 𝜂 𝛿 aN\eta_{\delta} italic_a italic_N italic_η start_POSTSUBSCRIPT italic_δ end_POSTSUBSCRIPT samples and the distance between the embedding of r U L t e s t superscript subscript r 𝑈 𝐿 𝑡 𝑒 𝑠 𝑡 \textbf{r}_{UL}^{test} r start_POSTSUBSCRIPT italic_U italic_L end_POSTSUBSCRIPT start_POSTSUPERSCRIPT italic_t italic_e italic_s italic_t end_POSTSUPERSCRIPT and the sample mean of the embeddings of its neighboring training samples is bounded as
(38) |
Next, (UB-2) can be bounded by using Theorem 1 as follows:
(39) |
for some constant K > 0 𝐾 0 K>0 italic_K > 0 .
Finally, (UB-3) is the average training error of the points in A U L superscript 𝐴 𝑈 𝐿 A^{UL} italic_A start_POSTSUPERSCRIPT italic_U italic_L end_POSTSUPERSCRIPT . Thus, by finding upper bounds on (UB-1) and (UB-2) as in ( 38 ) and ( 39 ) respectively, the difference between the test error of any point and the average training error of its neighboring training points can be upper bounded as given in Theorem 2 . ∎
Appendix C Proof of Lemma 1
A training sample r U L i superscript subscript r 𝑈 𝐿 𝑖 {\textbf{r}_{UL}}^{i} r start_POSTSUBSCRIPT italic_U italic_L end_POSTSUBSCRIPT start_POSTSUPERSCRIPT italic_i end_POSTSUPERSCRIPT drawn independently from r U L t e s t superscript subscript r 𝑈 𝐿 𝑡 𝑒 𝑠 𝑡 {\textbf{r}_{UL}}^{test} r start_POSTSUBSCRIPT italic_U italic_L end_POSTSUBSCRIPT start_POSTSUPERSCRIPT italic_t italic_e italic_s italic_t end_POSTSUPERSCRIPT lies in a δ 𝛿 \delta italic_δ -neighborhood of r U L t e s t superscript subscript r 𝑈 𝐿 𝑡 𝑒 𝑠 𝑡 {\textbf{r}_{UL}}^{test} r start_POSTSUBSCRIPT italic_U italic_L end_POSTSUBSCRIPT start_POSTSUPERSCRIPT italic_t italic_e italic_s italic_t end_POSTSUPERSCRIPT with probability
From [ 34 ] and the references therein, one can show that
for 1 ≤ Q < N η δ 1 𝑄 𝑁 subscript 𝜂 𝛿 1\leq Q<N\eta_{\delta} 1 ≤ italic_Q < italic_N italic_η start_POSTSUBSCRIPT italic_δ end_POSTSUBSCRIPT . Assuming that | A U L | ≥ Q superscript 𝐴 𝑈 𝐿 𝑄 \left|A^{UL}\right|\geq Q | italic_A start_POSTSUPERSCRIPT italic_U italic_L end_POSTSUPERSCRIPT | ≥ italic_Q , from [ 34 ] and the references therein, one can show that, with probability at least
the distance between the embedding of r U L t e s t superscript subscript r 𝑈 𝐿 𝑡 𝑒 𝑠 𝑡 {\textbf{r}_{UL}}^{test} r start_POSTSUBSCRIPT italic_U italic_L end_POSTSUBSCRIPT start_POSTSUPERSCRIPT italic_t italic_e italic_s italic_t end_POSTSUPERSCRIPT and the sample average of the embeddings of training samples lying inside the δ 𝛿 \delta italic_δ -neighborhood of r U L t e s t superscript subscript r 𝑈 𝐿 𝑡 𝑒 𝑠 𝑡 {\textbf{r}_{UL}}^{test} r start_POSTSUBSCRIPT italic_U italic_L end_POSTSUBSCRIPT start_POSTSUPERSCRIPT italic_t italic_e italic_s italic_t end_POSTSUPERSCRIPT is bounded as
(40) |
Let B 1 subscript 𝐵 1 B_{1} italic_B start_POSTSUBSCRIPT 1 end_POSTSUBSCRIPT be the event that the inequality in ( 40 ) holds. Combining the probability expressions above,
(41) |
Thus, one can see that with probability at least
| A U L | ≥ Q superscript 𝐴 𝑈 𝐿 𝑄 \left|A^{UL}\right|\geq Q | italic_A start_POSTSUPERSCRIPT italic_U italic_L end_POSTSUPERSCRIPT | ≥ italic_Q and B 1 subscript 𝐵 1 B_{1} italic_B start_POSTSUBSCRIPT 1 end_POSTSUBSCRIPT occurs. Setting Q = a N η δ 𝑄 𝑎 𝑁 subscript 𝜂 𝛿 Q=aN\eta_{\delta} italic_Q = italic_a italic_N italic_η start_POSTSUBSCRIPT italic_δ end_POSTSUBSCRIPT for 0 < a < 1 0 𝑎 1 0<a<1 0 < italic_a < 1 , one can reach the statement given in Lemma 1 . ∎
Appendix D Numerical Analysis About the Constant K 𝐾 K italic_K :
¯ subscript 𝑣 𝑖 ¯ subscript 𝑣 𝑗 2 C:=\cos\left(\frac{\bar{v_{i}}+\bar{v_{j}}}{2}\right) italic_C := roman_cos ( divide start_ARG over¯ start_ARG italic_v start_POSTSUBSCRIPT italic_i end_POSTSUBSCRIPT end_ARG + over¯ start_ARG italic_v start_POSTSUBSCRIPT italic_j end_POSTSUBSCRIPT end_ARG end_ARG start_ARG 2 end_ARG ) and b := C sin ( Δ ) assign 𝑏 𝐶 Δ b:=C\sin\left(\Delta\right) italic_b := italic_C roman_sin ( roman_Δ ) . Then, Δ s i n subscript Δ 𝑠 𝑖 𝑛 \Delta_{sin} roman_Δ start_POSTSUBSCRIPT italic_s italic_i italic_n end_POSTSUBSCRIPT can be written as
(42) |
Since − 1 ≤ C ≤ 1 1 𝐶 1 -1\leq C\leq 1 - 1 ≤ italic_C ≤ 1 , we have − sin ( Δ ) ≤ b ≤ sin ( Δ ) Δ 𝑏 Δ -\sin\left(\Delta\right)\leq b\leq\sin\left(\Delta\right) - roman_sin ( roman_Δ ) ≤ italic_b ≤ roman_sin ( roman_Δ ) . Since sin 2 ( ⋅ ) superscript 2 ⋅ \sin^{2}(\cdot) roman_sin start_POSTSUPERSCRIPT 2 end_POSTSUPERSCRIPT ( ⋅ ) is an even function, it is enough to examine only the positive side of the interval, i.e., 0 ≤ b ≤ sin ( Δ ) 0 𝑏 Δ 0\leq b\leq\sin\left(\Delta\right) 0 ≤ italic_b ≤ roman_sin ( roman_Δ ) . We evaluate the K 𝐾 K italic_K constant for different Δ Δ \Delta roman_Δ values (hence, different maximum values of b 𝑏 b italic_b ) for a range of base station antenna numbers, M 𝑀 M italic_M . Table IV reports the values that K 𝐾 K italic_K takes for 2 ≤ M ≤ 1000 2 𝑀 1000 2\leq M\leq 1000 2 ≤ italic_M ≤ 1000 and for different Δ Δ \Delta roman_Δ values, where f R = 1.0974 subscript 𝑓 𝑅 1.0974 f_{R}=1.0974 italic_f start_POSTSUBSCRIPT italic_R end_POSTSUBSCRIPT = 1.0974 is taken as in our communication scenario.
Corresponding Value | |
---|---|
5 | 1.0974 |
10 | 1.0974 |
15 | 1.0974 |
35 | 1.0974 |
45 | 1.1317 |
60 | 1.1893 |
- [1] E. Björnson, J. Hoydis, L. Sanguinetti, et al. , “Massive mimo networks: Spectral, energy, and hardware efficiency,” Foundations and Trends® in Signal Processing , vol. 11, no. 3-4, pp. 154–655, 2017.
- [2] F. Rusek, D. Persson, B. K. Lau, E. G. Larsson, T. L. Marzetta, O. Edfors, and F. Tufvesson, “Scaling up mimo: Opportunities and challenges with very large arrays,” IEEE signal processing magazine , vol. 30, no. 1, pp. 40–60, 2012.
- [3] E. Björnson, E. G. Larsson, and T. L. Marzetta, “Massive mimo: Ten myths and one critical question,” IEEE Communications Magazine , vol. 54, no. 2, pp. 114–123, 2016.
- [4] Y. Xu, G. Yue, and S. Mao, “User grouping for massive mimo in fdd systems: New design methods and analysis,” IEEE Access , vol. 2, pp. 947–959, 2014.
- [5] E. Björnson, J. Hoydis, M. Kountouris, and M. Debbah, “Massive mimo systems with non-ideal hardware: Energy efficiency, estimation, and capacity limits,” IEEE Transactions on information theory , vol. 60, no. 11, pp. 7112–7139, 2014.
- [6] Z. Zhong, L. Fan, and S. Ge, “Fdd massive mimo uplink and downlink channel reciprocity properties: Full or partial reciprocity?,” in GLOBECOM 2020-2020 IEEE Global Communications Conference , pp. 1–5, IEEE, 2020.
- [7] H. Xie, F. Gao, S. Jin, J. Fang, and Y.-C. Liang, “Channel estimation for tdd/fdd massive mimo systems with channel covariance computing,” IEEE Transactions on Wireless Communications , vol. 17, no. 6, pp. 4206–4218, 2018.
- [8] Y.-C. Liang and F. P. S. Chin, “Downlink channel covariance matrix (dccm) estimation and its applications in wireless ds-cdma systems,” IEEE Journal on Selected Areas in Communications , vol. 19, no. 2, pp. 222–232, 2001.
- [9] M. Jordan, A. Dimofte, X. Gong, and G. Ascheid, “Conversion from uplink to downlink spatio-temporal correlation with cubic splines,” in VTC Spring 2009-IEEE 69th Vehicular Technology Conference , pp. 1–5, IEEE, 2009.
- [10] A. Decurninge, M. Guillaud, and D. T. Slock, “Channel covariance estimation in massive mimo frequency division duplex systems,” in 2015 IEEE Globecom Workshops (GC Wkshps) , pp. 1–6, IEEE, 2015.
- [11] M. B. Khalilsarai, S. Haghighatshoar, X. Yi, and G. Caire, “Fdd massive mimo via ul/dl channel covariance extrapolation and active channel sparsification,” IEEE Transactions on Wireless Communications , vol. 18, no. 1, pp. 121–135, 2018.
- [12] L. Miretti, R. L. G. Cavalcante, and S. Stańczak, “Channel covariance conversion and modelling using infinite dimensional hilbert spaces,” IEEE Transactions on Signal Processing , vol. 69, pp. 3145–3159, 2021.
- [13] B. Banerjee, R. C. Elliott, W. A. Krzymień, and H. Farmanbar, “Downlink channel estimation for fdd massive mimo using conditional generative adversarial networks,” IEEE Transactions on Wireless Communications , vol. 22, no. 1, pp. 122–137, 2022.
- [14] S. Bameri, K. A. Almahrog, R. H. Gohary, A. El-Keyi, and Y. A. E. Ahmed, “Uplink to downlink channel covariance transformation in fdd systems,” IEEE Transactions on Signal Processing , vol. 71, pp. 3196–3212, 2023.
- [15] K. Hugl, K. Kalliola, J. Laurila, et al. , “Spatial reciprocity of uplink and downlink radio channels in fdd systems,” in Proc. COST , vol. 273, p. 066, Citeseer, 2002.
- [16] Y. Yang, F. Gao, G. Y. Li, and M. Jian, “Deep learning-based downlink channel prediction for fdd massive mimo system,” IEEE Communications Letters , vol. 23, no. 11, pp. 1994–1998, 2019.
- [17] W. Utschick, V. Rizzello, M. Joham, Z. Ma, and L. Piazzi, “Learning the csi recovery in fdd systems,” IEEE Transactions on Wireless Communications , vol. 21, no. 8, pp. 6495–6507, 2022.
- [18] J. Zeng, J. Sun, G. Gui, B. Adebisi, T. Ohtsuki, H. Gacanin, and H. Sari, “Downlink csi feedback algorithm with deep transfer learning for fdd massive mimo systems,” IEEE Transactions on Cognitive Communications and Networking , vol. 7, no. 4, pp. 1253–1265, 2021.
- [19] J. Wang, G. Gui, T. Ohtsuki, B. Adebisi, H. Gacanin, and H. Sari, “Compressive sampled csi feedback method based on deep learning for fdd massive mimo systems,” IEEE Transactions on Communications , vol. 69, no. 9, pp. 5873–5885, 2021.
- [20] M. Nerini, V. Rizzello, M. Joham, W. Utschick, and B. Clerckx, “Machine learning-based csi feedback with variable length in fdd massive mimo,” IEEE Transactions on Wireless Communications , vol. 22, no. 5, pp. 2886–2900, 2022.
- [21] A. Adhikary, J. Nam, J.-Y. Ahn, and G. Caire, “Joint spatial division and multiplexing—the large-scale array regime,” IEEE transactions on information theory , vol. 59, no. 10, pp. 6441–6463, 2013.
- [22] F. Sohrabi, K. M. Attiah, and W. Yu, “Deep learning for distributed channel feedback and multiuser precoding in fdd massive mimo,” IEEE Transactions on Wireless Communications , vol. 20, no. 7, pp. 4044–4057, 2021.
- [23] K. Li, Y. Li, L. Cheng, Q. Shi, and Z.-Q. Luo, “Downlink channel covariance matrix reconstruction for fdd massive mimo systems with limited feedback,” IEEE Transactions on Signal Processing , 2024.
- [24] Y. Liu and O. Simeone, “Learning how to transfer from uplink to downlink via hyper-recurrent neural network for fdd massive mimo,” IEEE Transactions on Wireless Communications , vol. 21, no. 10, pp. 7975–7989, 2022.
- [25] L. Miretti, R. L. G. Cavalcante, and S. Stanczak, “Fdd massive mimo channel spatial covariance conversion using projection methods,” in 2018 IEEE International Conference on Acoustics, Speech and Signal Processing (ICASSP) , pp. 3609–3613, IEEE, 2018.
- [26] P. Isola, J.-Y. Zhu, T. Zhou, and A. A. Efros, “Image-to-image translation with conditional adversarial networks,” in Proceedings of the IEEE conference on computer vision and pattern recognition , pp. 1125–1134, 2017.
- [27] C. Örnek and E. Vural, “Nonlinear supervised dimensionality reduction via smooth regular embeddings,” Pattern Recognition , vol. 87, pp. 55–66, 2019.
- [28] C. Piret, Analytical and numerical advances in radial basis functions . PhD thesis, University of Colorado at Boulder, 2007.
- [29] M. Belkin and P. Niyogi, “Laplacian eigenmaps for dimensionality reduction and data representation,” Neural computation , vol. 15, no. 6, pp. 1373–1396, 2003.
- [30] G. Roussos and B. J. Baxter, “Rapid evaluation of radial basis functions,” Journal of Computational and Applied Mathematics , vol. 180, no. 1, pp. 51–70, 2005.
- [31] “LTE; Evolved Universal Terrestrial Radio Access (E-UTRA); User Equipment (UE) Radio Transmission and Reception (3GPP TS 36.101 Version 14.3.0 Release 14), document ETSI TS 136 101 V14.3.0,” Apr 2017.
- [32] M. Herdin and E. Bonek, A MIMO correlation matrix based metric for characterizing non-stationarity . na, 2004.
- [33] K. M. Grigoriadis, A. E. Frazho, and R. E. Skelton, “Application of alternating convex projection methods for computation of positive toeplitz matrices,” IEEE transactions on signal processing , vol. 42, no. 7, pp. 1873–1875, 1994.
- [34] S. Kaya and E. Vural, “Learning multi-modal nonlinear embeddings: Performance bounds and an algorithm,” CoRR , vol. abs/2006.02330, 2020.
Information
- Author Services
Initiatives
You are accessing a machine-readable page. In order to be human-readable, please install an RSS reader.
All articles published by MDPI are made immediately available worldwide under an open access license. No special permission is required to reuse all or part of the article published by MDPI, including figures and tables. For articles published under an open access Creative Common CC BY license, any part of the article may be reused without permission provided that the original article is clearly cited. For more information, please refer to https://www.mdpi.com/openaccess .
Feature papers represent the most advanced research with significant potential for high impact in the field. A Feature Paper should be a substantial original Article that involves several techniques or approaches, provides an outlook for future research directions and describes possible research applications.
Feature papers are submitted upon individual invitation or recommendation by the scientific editors and must receive positive feedback from the reviewers.
Editor’s Choice articles are based on recommendations by the scientific editors of MDPI journals from around the world. Editors select a small number of articles recently published in the journal that they believe will be particularly interesting to readers, or important in the respective research area. The aim is to provide a snapshot of some of the most exciting work published in the various research areas of the journal.
Original Submission Date Received: .
- Active Journals
- Find a Journal
- Proceedings Series
- For Authors
- For Reviewers
- For Editors
- For Librarians
- For Publishers
- For Societies
- For Conference Organizers
- Open Access Policy
- Institutional Open Access Program
- Special Issues Guidelines
- Editorial Process
- Research and Publication Ethics
- Article Processing Charges
- Testimonials
- Preprints.org
- SciProfiles
- Encyclopedia
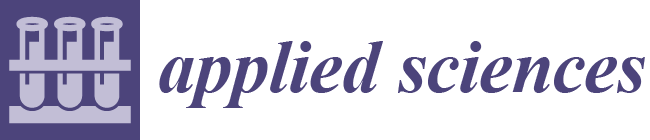
Article Menu

- Subscribe SciFeed
- Recommended Articles
- Google Scholar
- on Google Scholar
- Table of Contents
Find support for a specific problem in the support section of our website.
Please let us know what you think of our products and services.
Visit our dedicated information section to learn more about MDPI.
JSmol Viewer
Knowledge graph and personalized answer sequences for programming knowledge tracing.

1. Introduction
- We construct a knowledge graph in the programming field to constrain the embedding of knowledge concepts by perceiving their types. The resulting embedding vectors can effectively connect the KCs in the exercises.
- In response to the phenomenon of students answering consecutive programming exercises, we explore students’ learning behavior and learning ability, and a gating mechanism is introduced to balance their historical and current knowledge states. This approach better reflects the personalized knowledge mastery of students.
- We conduct extensive experiments on two real-world programming datasets, which shows that GPPKT outperforms state-of-the-art models, with an average AUC improvement of 9.0%. Ablation experiments are also performed to ensure the reliability and effectiveness of each component in the study.
2. Related Work
3.1. presentation of the problem, 3.2. overall architecture, 3.3. knowledge graph to represent exercise vectors, 3.3.1. constructing knowledge graph.
- Knowledge concept acquisition : We gather information about KCs from the OI-Wiki website. Specifically, hierarchical and relational information about programming concepts, such as “Dynamic Programming” and its associated subtopics, like “Depth First Search” and “Recurrence”, is extracted. To ensure the completeness and correctness of these concepts, the obtained KCs are categorized and organized with the help of information from CSDN and Wikipedia. Additionally, three authoritative textbooks, Data Structure Programming Practice, Introduction to Competitive Programming (2nd Edition) , and Introduction to Algorithms , are used for validation. This process results in a knowledge graph with 11 topics and 204 KCs.
- Knowledge graph design : Introductory KCs in the field of programming are first identified based on various knowledge sources. For instance, under the “Fundamentals” category, concepts such as “Divide and Conquer” and “Function” are categorized as basic KCs. Next, predecessor–successor relationships are established, linking more advanced KCs like “Dynamic Programming” to foundational concepts. These relationships are labeled with references to content from Data Structure Programming Practice and OI-Wiki. Further validation of the initial knowledge graph is carried out using Introduction to Competitive Programming (2nd Edition) and Introduction to Algorithms to minimize potential subjective biases. The graph is then optimized by retrieving additional hierarchical relationships for KCs from Wikipedia, ensuring a more comprehensive coverage.
- Knowledge storage: After KC acquisition and knowledge graph design, we obtain the nodes and relationships required for the knowledge graph and finally use Neo4j for knowledge storage.
3.3.2. Knowledge Graph Embedding Model: RotatE-TA
- Entity and Relation Definition : We define predecessor KC as head entities c i and successor KC as tail entities c j , with the relation denoted as r . These two KCs, along with the relation, are represented as a triple ( c i , r , c j ) .
- Scoring Function : We define a scoring function for the triple as d r ( c i , c j ) , which evaluates the importance of a candidate triple.
- Type Comparison : The knowledge topics for all KCs in the knowledge graph are defined as T = { t 1 , t 2 , … , t m } , where each KC c i is associated with a knowledge topic c i t ∈ T , and each KC belongs to only one knowledge topic. The comparison of the two knowledge topic types is calculated as follows: t y p e ( c i , c j ) = 1 , if c i t = c j t 0 , else (1)
- Distance Scoring with Type-Awareness : Based on the type comparison function, we introduce type-aware weights into the distance scoring function as follows: d r ( c i , c j ) = | | c i ∘ r − c j | | + λ ∗ | | m c i − m c j | | ∗ t y p e ( c i , c j ) . (2) In Equation ( 2 ), ∘ represents the Hadamard product, m c i and m c j represent the modular lengths of the head entity and tail entity, respectively. λ is a hyperparameter representing the magnitude of type-aware weights.
3.4. Modeling Answer Sequences with VAE
3.4.1. input representation, 3.4.2. model architecture, 3.4.3. objective function.
- Reconstruction Loss : This loss measures the difference between the original input r t s e q and the output of the decoder y . It is defined as: L r e c o n = − ∑ i = 1 N r t s e q i log ( y i ) + ( 1 − r t s e q i ) log ( 1 − y i ) , (5) where N represents the dimensions of the student’s answer sequence, r t s e q i and y i represent the i -th element of the input sample r t s e q and the decoder output y , respectively.
- KL Divergence Loss : This loss encourages the learned latent variable distribution to be close to a standard normal distribution. It is defined as: L K L = − 1 2 ∑ j = 1 D 1 + log ( ( σ j ) 2 ) − ( μ j ) 2 − ( σ j ) 2 , (6) where D represents the dimensions of the latent variable z t , and μ j and σ j represent the mean and standard deviation of the j -th dimension of the latent variable z t , respectively.
3.5. Students’ Personalized Learning Abilities
3.5.1. first-order irt for ability assessment, 3.5.2. inverting ability parameters, 3.6. lstm framework, 3.6.1. modeling knowledge states with lstm, 3.6.2. attention mechanism, 3.6.3. gating mechanism, 3.7. prediction, 4. experiments, 4.1. dataset, 4.1.1. data source, 4.1.2. data preprocess.
- Filtering Invalid Submissions : We filtered out invalid submission records, such as those labeled “Time limit exceeded”, “Runtime error”, or “Unanswered”, to retain only valid and meaningful submission data. These invalid records were considered noise and could skew the analysis if included.
- Removing Irrelevant Users : We excluded users with an insufficient number of answer sequences, as these would not provide enough data to model effectively. Additionally, non-student users, including administrators and virtual users, were removed to ensure that the dataset reflected genuine student learning behavior.
- Normalizing Sequence Lengths : Since the number of exercises answered by each student varied significantly, directly modeling answer sequences of different lengths would be challenging. To address this, we standardized the sequence length to a fixed value, ensuring that all input sequences were of uniform length for the model. This involved truncating longer sequences and padding shorter ones to the specified length.
4.2. Environment
4.3. evaluation metrics and baseline methods, 4.3.1. evaluation metrics, 4.3.2. baseline methods.
- DKT [ 1 ]: A pioneering knowledge tracing method using recurrent neural networks.
- DKT+ [ 2 ]: An improved DKT model addressing input reconstruction and state fluctuations.
- DKVMN [ 3 ]: A memory network-based model with interpretable knowledge and student state representation.
- Deep-IRT [ 13 ]: Combines IRT with DKVMN for an interpretable deep knowledge tracing model.
- AKT [ 6 ]: Introduces the Rasch model and self-attention for better exercise and interaction modeling.
- ATKT [ 15 ]: Adds adversarial perturbations to LSTM-based sequences to reduce overfitting.
- IEKT [ 5 ]: Combines individual cognition and learning processes for accurate knowledge state tracing.
- SAKT [ 4 ]: Uses self-attention mechanisms in Transformer to handle sparse data in knowledge tracing.
- SAINT [ 35 ]: Extends SAKT with additional attention modules for deeper exercise–answer relationship modeling.
4.4. Model Training and Parameter Selection
4.5. experiment results, 4.5.1. comparative experiment analysis, 4.5.2. ablation experiment analysis, 4.6. visualization, 4.6.1. knowledge graph visualization, 4.6.2. student learning abilities visualization, 4.6.3. personalized answer sequence visualization, 5. conclusions, author contributions, institutional review board statement, informed consent statement, data availability statement, acknowledgments, conflicts of interest.
- Piech, C.; Bassen, J.; Huang, J.; Ganguli, S.; Sahami, M.; Guibas, L.J.; Sohl-Dickstein, J. Deep knowledge tracing. In Proceedings of the Advances in Neural Information Processing Systems, Montreal, QC, Canada, 7–12 December 2015; Volume 28. [ Google Scholar ]
- Yeung, C.K.; Yeung, D.Y. Addressing two problems in deep knowledge tracing via prediction-consistent regularization. In Proceedings of the Fifth Annual ACM Conference on Learning at Scale, London, UK, 26–28 June 2018; pp. 1–10. [ Google Scholar ]
- Zhang, J.; Shi, X.; King, I.; Yeung, D.Y. Dynamic key-value memory networks for knowledge tracing. In Proceedings of the 26th International Conference on World Wide Web, Perth, Australia, 3–7 April 2017; pp. 765–774. [ Google Scholar ]
- Pandey, S.; Karypis, G. A self-attentive model for knowledge tracing. arXiv 2019 , arXiv:1907.06837. [ Google Scholar ]
- Long, T.; Liu, Y.; Shen, J.; Zhang, W.; Yu, Y. Tracing knowledge state with individual cognition and acquisition estimation. In Proceedings of the 44th International ACM SIGIR Conference on Research and Development in Information Retrieval, Virtual, 11–15 July 2021; pp. 173–182. [ Google Scholar ]
- Ghosh, A.; Heffernan, N.; Lan, A.S. Context-aware attentive knowledge tracing. In Proceedings of the 26th ACM SIGKDD International Conference on Knowledge Discovery & Data Mining, Virtual, 23–27 August 2020; pp. 2330–2339. [ Google Scholar ]
- Nakagawa, H.; Iwasawa, Y.; Matsuo, Y. Graph-based knowledge tracing: Modeling student proficiency using graph neural network. In Proceedings of the IEEE/WIC/ACM International Conference on Web Intelligence, Thessaloniki, Greece, 14–17 October 2019; pp. 156–163. [ Google Scholar ]
- Kingma, D.P.; Welling, M. Auto-encoding variational bayes. arXiv 2013 , arXiv:1312.6114. [ Google Scholar ]
- Hochreiter, S.; Schmidhuber, J. Long short-term memory. Neural Comput. 1997 , 9 , 1735–1780. [ Google Scholar ] [ CrossRef ] [ PubMed ]
- Su, Y.; Liu, Q.; Liu, Q.; Huang, Z.; Yin, Y.; Chen, E.; Ding, C.; Wei, S.; Hu, G. Exercise-enhanced sequential modeling for student performance prediction. In Proceedings of the AAAI Conference on Artificial Intelligence, New Orleans, LA, USA, 2–7 February 2018; Volume 32. [ Google Scholar ]
- Minn, S.; Yu, Y.; Desmarais, M.C.; Zhu, F.; Vie, J.J. Deep knowledge tracing and dynamic student classification for knowledge tracing. In Proceedings of the 2018 IEEE International conference on data mining (ICDM), Singapore, 17–20 November 2018; pp. 1182–1187. [ Google Scholar ]
- Lee, J.; Yeung, D.Y. Knowledge query network for knowledge tracing: How knowledge interacts with skills. In Proceedings of the 9th International Conference on Learning Analytics & Knowledge, Tempe, AZ, USA, 4–8 March 2019; pp. 491–500. [ Google Scholar ]
- Yeung, C.K. Deep-IRT: Make deep learning based knowledge tracing explainable using item response theory. arXiv 2019 , arXiv:1904.11738. [ Google Scholar ]
- Abdelrahman, G.; Wang, Q. Knowledge tracing with sequential key-value memory networks. In Proceedings of the 42nd International ACM SIGIR Conference on Research and Development in Information Retrieval, Paris, France, 21–25 July 2019; pp. 175–184. [ Google Scholar ]
- Guo, X.; Huang, Z.; Gao, J.; Shang, M.; Shu, M.; Sun, J. Enhancing knowledge tracing via adversarial training. In Proceedings of the 29th ACM International Conference on Multimedia, Virtual, 20–24 October 2021; pp. 367–375. [ Google Scholar ]
- Pandey, S.; Srivastava, J. RKT: Relation-aware self-attention for knowledge tracing. In Proceedings of the 29th ACM International Conference on Information & Knowledge Management, Virtual, 19–23 October 2020; pp. 1205–1214. [ Google Scholar ]
- Shen, S.; Liu, Q.; Chen, E.; Wu, H.; Huang, Z.; Zhao, W.; Su, Y.; Ma, H.; Wang, S. Convolutional knowledge tracing: Modeling individualization in student learning process. In Proceedings of the 43rd International ACM SIGIR Conference on Research and Development in Information Retrieval, Virtual, 25–30 July 2020; pp. 1857–1860. [ Google Scholar ]
- Wang, W.; Liu, T.; Chang, L.; Gu, T.; Zhao, X. Convolutional recurrent neural networks for knowledge tracing. In Proceedings of the 2020 International Conference on Cyber-Enabled Distributed Computing and Knowledge Discovery (CyberC), Chongqing, China, 29–30 October 2020; pp. 287–290. [ Google Scholar ]
- Liu, Q.; Huang, Z.; Yin, Y.; Chen, E.; Xiong, H.; Su, Y.; Hu, G. Ekt: Exercise-aware knowledge tracing for student performance prediction. IEEE Trans. Knowl. Data Eng. 2019 , 33 , 100–115. [ Google Scholar ] [ CrossRef ]
- Ebbinghaus, H. Memory: A contribution to experimental psychology. Ann. Neurosci. 2013 , 20 , 155–156. [ Google Scholar ] [ CrossRef ] [ PubMed ]
- Nagatani, K.; Zhang, Q.; Sato, M.; Chen, Y.Y.; Chen, F.; Ohkuma, T. Augmenting knowledge tracing by considering forgetting behavior. In Proceedings of the World Wide Web Conference, San Francisco, CA, USA, 13–17 May 2019; pp. 3101–3107. [ Google Scholar ]
- Yang, Y.; Shen, J.; Qu, Y.; Liu, Y.; Wang, K.; Zhu, Y.; Zhang, W.; Yu, Y. GIKT: A graph-based interaction model for knowledge tracing. In Proceedings of the Machine Learning and Knowledge Discovery in Databases: European Conference, ECML PKDD 2020, Ghent, Belgium, 14–18 September 2020; Proceedings, Part I. Springer: Berlin/Heidelberg, Germany, 2021; pp. 299–315. [ Google Scholar ]
- Song, Q.; Luo, W. SFBKT: A Synthetically Forgetting Behavior Method for Knowledge Tracing. Appl. Sci. 2023 , 13 , 7704. [ Google Scholar ] [ CrossRef ]
- Kasurinen, J.; Nikula, U. Estimating programming knowledge with Bayesian knowledge tracing. ACM SIGCSE Bull. 2009 , 41 , 313–317. [ Google Scholar ] [ CrossRef ]
- Wang, L.; Sy, A.; Liu, L.; Piech, C. Learning to Represent Student Knowledge on Programming Exercises Using Deep Learning. In Proceedings of the International Educational Data Mining Society, Paper Presented at the International Conference on Educational Data Mining (EDM), Wuhan, China, 25–28 June 2017. [ Google Scholar ]
- Piech, C.; Huang, J.; Nguyen, A.; Phulsuksombati, M.; Sahami, M.; Guibas, L. Learning program embeddings to propagate feedback on student code. In Proceedings of the International Conference on Machine Learning, PMLR, Lille, France, 6–11 July 2015; pp. 1093–1102. [ Google Scholar ]
- Swamy, V.; Guo, A.; Lau, S.; Wu, W.; Wu, M.; Pardos, Z.; Culler, D. Deep knowledge tracing for free-form student code progression. In Proceedings of the Artificial Intelligence in Education: 19th International Conference, AIED 2018, London, UK, 27–30 June 2018; Proceedings, Part II 19. Springer: Berlin/Heidelberg, Germany, 2018; pp. 348–352. [ Google Scholar ]
- Shi, Y.; Chi, M.; Barnes, T.; Price, T. Code-dkt: A code-based knowledge tracing model for programming tasks. arXiv 2022 , arXiv:2206.03545. [ Google Scholar ]
- Jiang, B.; Wu, S.; Yin, C.; Zhang, H. Knowledge tracing within single programming practice using problem-solving process data. IEEE Trans. Learn. Technol. 2020 , 13 , 822–832. [ Google Scholar ] [ CrossRef ]
- Liang, Y.; Peng, T.; Pu, Y.; Wu, W. HELP-DKT: An interpretable cognitive model of how students learn programming based on deep knowledge tracing. Sci. Rep. 2022 , 12 , 4012. [ Google Scholar ] [ CrossRef ] [ PubMed ]
- Sun, Z.; Deng, Z.H.; Nie, J.Y.; Tang, J. Rotate: Knowledge graph embedding by relational rotation in complex space. arXiv 2019 , arXiv:1902.10197. [ Google Scholar ]
- Le Cun, Y.; Fogelman-Soulié, F. Modèles connexionnistes de l’apprentissage. Intellectica 1987 , 2 , 114–143. [ Google Scholar ] [ CrossRef ]
- Koedinger, K.R.; Baker, R.S.; Cunningham, K.; Skogsholm, A.; Leber, B.; Stamper, J. A data repository for the EDM community: The PSLC DataShop. Handb. Educ. Data Min. 2010 , 43 , 43–56. [ Google Scholar ]
- Feng, M.; Heffernan, N.; Koedinger, K. Addressing the assessment challenge with an online system that tutors as it assesses. User Model. User-Adapt. Interact. 2009 , 19 , 243–266. [ Google Scholar ] [ CrossRef ]
- Choi, Y.; Lee, Y.; Cho, J.; Baek, J.; Kim, B.; Cha, Y.; Shin, D.; Bae, C.; Heo, J. Towards an appropriate query, key, and value computation for knowledge tracing. In Proceedings of the Seventh ACM Conference on Learning@ Scale, Virtual, 12–14 August 2020; pp. 341–344. [ Google Scholar ]
- Liu, Z.; Liu, Q.; Chen, J.; Huang, S.; Tang, J.; Luo, W. pyKT: A python library to benchmark deep learning based knowledge tracing models. Adv. Neural Inf. Process. Syst. 2022 , 35 , 18542–18555. [ Google Scholar ]
Click here to enlarge figure
Data Sources | Students | Exercises | Interactions | Knowledge Concepts |
---|---|---|---|---|
Luogu | 2081 | 3329 | 299,168 | 190 |
Codeforces | 1685 | 8237 | 630,724 | 204 |
Configuration Environment | Configuration Parameters |
---|---|
Operating System | Windows 10 64-bit |
GPU | RTX 3070ti |
CPU | i7-13700H |
Memory | 16 GB |
Programming language | Python 3.7 |
Deep learning framework | tensorflow 2.1.0 |
Python library | Scikit-learn, Numpy, Pandas |
Methods | Luogu | Codeforces | ||
---|---|---|---|---|
AUC | ACC | AUC | ACC | |
DKT | 0.8114 | 0.8397 | 0.6804 | 0.8574 |
DKT+ | 0.8104 | 0.8388 | 0.7037 | 0.8589 |
Deep-IRT | 0.8020 | 0.8271 | 0.6702 | 0.8526 |
DKVMN | 0.8058 | 0.8300 | 0.6828 | 0.8573 |
IEKT | 0.8230 | 0.8309 | 0.7308 | 0.8567 |
SAKT | 0.8301 | 0.8307 | 0.6708 | 0.8550 |
SAINT | 0.8263 | 0.8254 | 0.6763 | 0.8577 |
AKT | 0.8313 | 0.8394 | 0.7491 | 0.8595 |
ATKT | 0.8380 | 0.8418 | 0.7543 | 0.8614 |
Methods | AUC | ACC |
---|---|---|
(1) LSTM | 0.8097 | 0.7954 |
(2) LSTM + VAE | 0.8576 | 0.8343 |
(3) LSTM + KG | 0.8654 | 0.8361 |
(4) LSTM + ABI | 0.8679 | 0.8380 |
(5) LSTM + VAE + ABI | 0.8762 | 0.8425 |
(6) LSTM + VAE + KG | 0.8749 | 0.8392 |
(7) LSTM + KG + ABI | 0.8703 | 0.8411 |
The statements, opinions and data contained in all publications are solely those of the individual author(s) and contributor(s) and not of MDPI and/or the editor(s). MDPI and/or the editor(s) disclaim responsibility for any injury to people or property resulting from any ideas, methods, instructions or products referred to in the content. |
Share and Cite
Pan, J.; Dong, Z.; Yan, L.; Cai, X. Knowledge Graph and Personalized Answer Sequences for Programming Knowledge Tracing. Appl. Sci. 2024 , 14 , 7952. https://doi.org/10.3390/app14177952
Pan J, Dong Z, Yan L, Cai X. Knowledge Graph and Personalized Answer Sequences for Programming Knowledge Tracing. Applied Sciences . 2024; 14(17):7952. https://doi.org/10.3390/app14177952
Pan, Jianguo, Zhengyang Dong, Lijun Yan, and Xia Cai. 2024. "Knowledge Graph and Personalized Answer Sequences for Programming Knowledge Tracing" Applied Sciences 14, no. 17: 7952. https://doi.org/10.3390/app14177952
Article Metrics
Further information, mdpi initiatives, follow mdpi.
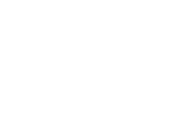
Subscribe to receive issue release notifications and newsletters from MDPI journals

IMAGES
VIDEO
COMMENTS
Generally, probabilistic graphical models use a graph-based representation as the foundation for encoding a distribution over a multi-dimensional space and a graph that is a compact or factorized representation of a set of independences that hold in the specific distribution. Two branches of graphical representations of distributions are commonly used, namely, Bayesian networks and Markov ...
Why do we need graphical models? • Graphs are an intuitive way of representing and visualising the relationships between many variables. (Examples: family trees, electric circuit diagrams, neural networks) • A graph allows us to abstract out the conditional independence relationships between the variables from the details of their parametric forms.
in graphical models, including the factorial and nested structures that occur in experimental designs. A simple example of a plate is shown in Figure 1, which can be viewed as a graphical model representation of the de Finetti exchangeability theorem. Directed graphical models are familiar as represen-tations of hierarchical Bayesian models. An ...
Visualizing a PyTorch Model. By Adrian Tam on April 8, 2023 in Deep Learning with PyTorch 2. PyTorch is a deep learning library. You can build very sophisticated deep learning models with PyTorch. However, there are times you want to have a graphical representation of your model architecture. In this post, you will learn:
A graphical model is a family of probability distributions defined in terms of a directed or. undirected graph. The nodes in the graph are identified with random variables, and joint probability distributions are defined by taking products over functions defined on connected subsets of nodes. By exploiting the graph-theoretic representation ...
How to develop a graphical framework to chart your research. Graphic representations or frameworks can be powerful tools to explain research processes and outcomes. David Waller explains how researchers can develop effective visual models to chart their work. Outreach and communication. Writing guides.
Graphical models are a class of statistical models which combine the rigour of a probabilistic approach with the intuitive representation of relationships given by graphs. They are composed by two parts: ... Graph representations meet our earlier requirements of explicitness, saliency, and stability. The links in the graph permit us to express
Graphical models provide a more economic representation of the joint distribution by taking advantage of local relationships between random variables. Directed graphical models ‚ A directed graphical model is a directed acyclic graph. The vertices are random variables X 1;:::;X n; edges denote the "parent of" relationship, where ˇ
Probabilistic graphical models (PGMs) are a rich framework for encoding probability distributions over complex domains: joint (multivariate) distributions over large numbers of random variables that interact with each other. These representations sit at the intersection of statistics and computer science, relying on concepts from probability ...
The underlying graph structure is an undirected graph G = (V, E). Definition 3. The joint distribution over x. ∈ X V is a Markov Random Field on G = (V, F, E) if there exists a vector of functions ψ = {ψC}C indexed by the cliques in C. G, ψC : X → R+, and a constant Z such that.
Bullet Graph. Choropleth Map. Word Cloud. Network Diagram. Correlation Matrices. 1. Pie Chart. Pie charts are one of the most common and basic data visualization techniques, used across a wide range of applications. Pie charts are ideal for illustrating proportions, or part-to-whole comparisons.
Probabilistic graphical models (PGMs) are a rich framework for encoding probability distributions over complex domains: joint (multivariate) distributions over large numbers of random variables that interact with each other. These representations sit at the intersection of statistics and computer science, relying on concepts from probability ...
Definition 2.22. The treewidth of a chordal graph is the size of the largest clique minus 1. The treewidth of an untriangulated graph is the minimum treewidth of all of its trian-gulations. Note that the treewidth of a chain in figure 2.4(a) is 1 and the treewidth of the grid in figure 2.4(b) is 4.
Jul 15, 2020. --. 1. created by author to illustrate the nodes and edges in a Bayesian network. Probabilistic graphical model (PGM) provides a graphical representation to understand the complex relationship between a set of random variables (RVs). RVs represent the nodes and the statistical dependency between them is called an edge.
In subject area: Computer Science. A Graphical Model is defined as a representation of probabilistic relationships among variables, where nodes correspond to variables and the absence of edges indicates conditional independence. AI generated definition based on: International Encyclopedia of the Social & Behavioral Sciences, 2001.
As we will discuss below, representing graphical models in computer code (using the Rev language) will likely be the most useful aspect of graphical models to most readers. The symbols for a visual representation of a graphical model. a) Solid squares represent constant nodes, which specify fixed- valued variables.
Representation of biological systems as graphical models, i.e., diagrams, can in principle circumvent these issues by presenting a system in a visually intuitive manner using a standardized ...
The author analyzes graphic representation, or diagramming, in terms of form and meaning, pro- poses a taxonomy of linguistic models that cuts across other classifications of linguistic theory, and shows that, like models in the biological and physical sciences, graphic representation has performed and continues to perform a heuristic function ...
Graphical Representation of Datasets. Types of graphical representation which will cover here are and why: Scatter Plot: With the help of the graph, we can see in which direction our linear regression model is going, whether there is any strong evidence to prove our model or not. Box Plot: Helps us to find outliers.
Oct 13, 2017. 2. Probabilistic graphical models or PGM are frameworks used to create probabilistic models of complex real world scenarios and represent them in compact graphical representation. This definition in itself is very abstract and involves many terms that needs it's own space, so lets take these terms one by one.
A process model is a graphical representation of a business process or workflow and its related subprocesses. Process modeling generates comprehensive, quantitative activity diagrams and flowcharts containing critical insights into the functioning of a particular process, including:. Events and activities that occur within a workflow.
A logic model is a graphical representation of your program, from the resources (inputs) and activities that will take place, to the deliverables (outputs) and goals( outcomes) that the program will produce. Three reasons to consider developing a logic model:
Self-supervised Heterogeneous Graph Representation (SSHGRL) learning is widely used in data mining. The latest SSHGRL methods normally use metapaths to describe the heterogeneous information (multiple relations and node types) to learn the heterogeneous graph representation and achieve impressive results. ... (RMR) model that can fully consider ...
Temporal knowledge graphs (TKGs) are a form of knowledge representation constructed based on the evolution of events at different time points. It provides an additional perspective by extending the temporal dimension for a range of downstream tasks. Given the evolving nature of events, it is essential for TKGs to reason about non-existent or future events. Most of the existing models divide ...
Z1 Z2 Z3 ZN θ N θ Zn (a) (b) Figure 1: The diagram in (a) is a shorthand for the graphical model in (b). This model asserts that the variables Zn are conditionally independent and identically distributed given θ, and can be viewed as a graphical model representation of the De Finetti theorem.
In Section III, the system model for the communication scenario is explained. In Section IV, the theoretical motivation behind our method is presented. A representation learning method for the problem of DL CCM estimation from UL CCMs is proposed in Section V.
Knowledge tracing is a significant research area in educational data mining, aiming to predict future performance based on students' historical learning data. In the field of programming, several challenges are faced in knowledge tracing, including inaccurate exercise representation and limited student information. These issues can lead to biased models and inaccurate predictions of students ...